DOI:
10.1039/C9AN02026H
(Paper)
Analyst, 2020,
145, 1236-1249
Rapid differentiation of Campylobacter jejuni cell wall mutants using Raman spectroscopy, SERS and mass spectrometry combined with chemometrics†
Received
10th October 2019
, Accepted 17th November 2019
First published on 18th November 2019
Abstract
The Gram-negative bacterial pathogen Campylobacter jejuni is a major cause of foodborne gastroenteritis worldwide. Rapid detection and identification of C. jejuni informs timely prescription of appropriate therapeutics and epidemiological investigations. Here, for the first time, we report the applicability of Raman spectroscopy, surface-enhanced Raman scattering (SERS) and matrix-assisted laser desorption/ionisation mass spectrometry (MALDI-TOF-MS) combined with chemometrics, for rapid differentiation and characterisation of mutants of a single isogenic C. jejuni strain that disrupt the production of prominent surface features (capsule, flagella and glycoproteins) of the bacterium. Multivariate analysis of the spectral data obtained from these different physicochemical tools revealed distinctive biochemical differences which consistently discriminated between these mutants. In order to generate biochemical and phenotypic information from different locations in the cell–cell wall versus cytoplasm – we developed two different in situ methods for silver nanoparticle (AgNP) production, and compared this with simple mixing of bacteria with pre-synthesised AgNPs. This SERS trilogy (simple mixing with premade AgNPs and two in situ AgNP production methods) presents an integrated platform with potential for rapid, accurate and confirmatory detection of pathogenic bacteria based on cell envelope or intracellular molecular dynamics. Our spectral findings demonstrate that Raman, SERS and MALDI-TOF-MS are powerful metabolic fingerprinting techniques capable of discriminating clinically relevant cell wall mutants of a single isogenic bacterial strain.
Introduction
Foodborne bacterial diseases are a global health challenge associated with high morbidity and mortality.1 Common bacterial pathogens which are responsible for foodborne infections include Escherichia coli O157, Listeria monocytogenes, Campylobacter and Salmonella spp.2 Human campylobacteriosis is considered the leading form of food poisoning worldwide.1,3 Several foodborne infection cases predominantly implicate C. jejuni strains as the leading etiological agents of campylobacteriosis.4,5 Recently, a survey of foodborne infections in the European Union reported a notification rate of 64.8 per 100
000 population for C. jejuni infections,6 which has increased substantially within the recent decade.3 Although specific outbreaks of Campylobacter food poisoning are uncommon, the many apparently sporadic cases are a significant public health problem causing a substantial global economic burden.4
Numerous epidemiological studies have identified contaminated chicken as the major source of Campylobacter infection,1,6 although other poultry are also reservoirs.7 The widespread consumption of chicken, a relatively inexpensive protein source, undoubtedly contributes to the scale of human C. jejuni infections. Whilst C. jejuni infection typically results in self-limiting gastroenteritis in humans, it can also lead to more serious outcomes such as reactive arthritis and Guillain-Barré syndrome involving acute neuromuscular paralysis.8,9 Thus, rapid detection of C. jejuni in real time is important for quick and effective surveillance, remediation and timely prescription of appropriate medication.
During infection, C. jejuni colonises the mucus layer in the gut and can also invade epithelial cells. Important virulence factors include the capsular polysaccharide (CPS), bipolar flagella that mediate cell motility, and the N-linked general protein glycosylation system that modifies a large number of surface located proteins.9,10 Accurate characterisation of C. jejuni based on biochemical dynamics and associated phenotypes may improve our understanding of the pathogenesis of campylobacteriosis and how C. jejuni interact with the human gut.11
Culture, along with morphological and biochemical tests, are the gold standard tools for the detection, isolation and identification of pathogens in healthcare systems, though alternative analytical techniques have been proposed.12 However, traditional microbiological methods are time consuming, and biochemical tests are prone to false negative results,12,13 since they do not detect biochemically unreactive clinical isolates.14 Although real-time polymerase chain reaction (PCR) can overcome these limitations by providing more sensitive and reliable taxonomy of microbes rapidly,15 it does not characterise real bacterial phenotypes and cannot differentiate between viable and non-viable microbes.12,16
Recently, Raman spectroscopy has emerged as an alternative molecularly specific tool for rapid, accurate, non-destructive and label-free characterisation of pathogens down to the strain level.17 A Raman spectrum can be described as a typical whole-organism biochemical fingerprint derived from intact bacterial cells, consisting of vibrational modes from molecules including metabolites, nucleic acids, proteins, lipids and carbohydrates.18 Consequently, Raman spectra can be utilised to infer strain-specific physiological, metabolic and phenotypic states of bacterial cells.19,20 However, Raman spectroscopy has limited sensitivity owing to its inherently poor quantum efficiency.21 Surface-enhanced Raman scattering (SERS) can be used to amplify Raman signals up to several orders of magnitude when a sample is in the close vicinity or adsorbed onto plasmonic materials such as metallic nanoparticles (NP).22 In addition to improving the sensitivity, SERS allows for selective deposition of NPs onto the bacterial cell wall or inside the cells.23 Thus, when Raman and SERS are applied together, they potentially provide complementary biomolecular information capable of differentiating bacterial classes as well as inferring functional capability. That is to say, whilst Raman spectroscopy provides whole-bacterium biochemical information and phenotype, SERS may selectively unravel and amplify structural moieties and bond linkages located specifically on bacterial cell surface including lipopolysaccharides (LPS) and peptidoglycan, or intracellular biomolecules such as amino acids, carbohydrates and nucleobases.24
Matrix-assisted laser desorption/ionisation time-of-flight mass spectrometry (MALDI-TOF-MS) has also gained significant attention for routine phenotyping of clinical isolates such as C. jejuni,25–27Bacillus28,29 and Enterococcus30,31 spp. Interestingly, in recent years, MALDI-TOF-MS has been applied in parallel with Raman and SERS fingerprints for comprehensive biochemical characterisation of bacteria.30 Similar to vibrational spectroscopy, MALDI-TOF-MS is rapid, robust and cost-effective, and elucidates structural dynamics specifically in physiologically essential cellular proteins, peptides and lipids biomarkers.32 However, to achieve clinically desirable reproducibility for bacterial detection, MALDI-TOF-MS also requires carefully optimised procedures including the identification of appropriate matrix preparation and deposition methods for bacterial samples.33
In this study, we applied Raman spectroscopy, SERS and MALDI-TOF-MS for rapid and accurate differentiation of a panel of mutants within the otherwise isogenic C. jejuni strain that disrupted the production of key surface biochemical features (CPS, flagella and N-glycosylated proteins). For the first time, we employed label-free SERS involving simple mixing and in situ synthesis of AgNPs to probe molecular dynamics of pathogenic bacteria to complement Raman and MALDI-TOF-MS fingerprints with the help of chemometrics.
Experimental methods
Bacterial strains and cultivation conditions
The C. jejuni NCTC 11168H strain and derived mutants (for details see Table 1) were grown on Columbia agar (Oxoid) plates containing 5% horse blood (TCS Biosciences), obtained from a single batch. Inoculated agar plates were incubated in a MACS Variable Atmosphere Incubator (Don Whitley Scientific Ltd) containing a gas mixture of 85% N2, 10% CO2 and 5% O2.
Table 1 List of C. jejuni strain and derivative mutants investigated in this study, including descriptions and sources
Strain |
Description |
Mutations |
Source/reference |
11168H |
Wild type |
None |
National collection of type cultures, U.K. Karlyshev et al.99 |
11168H pglB− |
Cj1126::kn |
Surface proteins lack heptasaccharide N-linked glycans |
Linton laboratory |
11168H kpsM− |
Cj1448c::kn |
Disrupted cytoplasmic CPS biosynthesis |
Karlyshev et al.42 |
11168H kpsS− |
Cj1413c::kn |
Disrupted cytoplasmic CPS biosynthesis |
Karlyshev et al.42 |
11168H kpsC− |
Cj1414c::kn |
Disrupted CPS transport to cell surface |
Karlyshev et al.42 |
11168H flaA− |
Cj1339c::kn |
Lacks flagella |
Linton laboratory |
Sample preparation
Biomass for C. jejuni NCTC 11168H and derived mutants was harvested from the surface of agar plates after 24 h of incubation. Following harvesting using sterile inoculating loops, biomass was resuspended in physiological saline (0.9% NaCl) in Falcon tubes (Greiner Bio-One, Germany) and stored on ice throughout the sample preparation steps. Each bacterial strain or mutant was grown on four separate agar plates and these were used as biological replicates per sample (n = 4). Bacterial cell samples were washed twice in 0.9% NaCl by centrifugation at 4000g for 5 min at 4 °C using a bench top Eppendorf Centrifuge 5810 R (Hamburg, Germany), and resuspended in 2 mL of 0.9% NaCl. Optical densities of all cell suspensions were measured spectrophotometrically at 600 nm using an Eppendorf BioSpectrometer (Eppendorf, Cambridge, UK) and used to normalise biomass. All bacterial samples with normalised final OD600 were stored at −80 °C prior to spectral analysis.
Transmission Electron Microscopy
The samples were fixed with 4% formaldehyde and 2.5% glutaraldehyde in 0.1 M HEPES buffer (pH 7.2). Then they were post-fixed with 1% osmium tetroxide and 1.5% potassium ferrocynaide in 0.1 M cacodylate buffer (pH 7.2) for 1 h and in 1% uranyl acetate in water for 1 h. The samples were dehydrated in an ethanol series infiltrated with TAAB 812 resin and polymerized for 24 h at 60 °C. Finally, 70 nm sections were cut with Reichert Ultracut ultramicrotome and observed with a FEI Tecnai 12 Biotwin microscope at 80 kV accelerating voltage. Images were taken with a Gatan Orius SC1000 CCD camera.
Synthesis of colloidal silver NPs (AgNPs)
All glassware was washed with aqua regia (1 part of HNO3 and 3 parts of HCl), rinsed in deionised water and dried in a fume hood prior to AgNPs synthesis.
The synthetic routes for in situ methods followed our previously reported protocols,34 similar to those described in detail by Efrima and Zeiri.23 For the in situ SERS method involving deposition of AgNPs around cell walls (in situ external), cell pellets were suspended in ice-cold 0.09 M NaBH4 solution acting as a reductant, followed by 5 min centrifugation at 2800g at 4 °C. After discarding supernatants, each pellet was resuspended in ice-cold 0.04 M AgNO3 solution. At this point, a redox reaction occurred resulting in the production of AgNPs on the cell wall as demonstrated recently.34 To effect the in situ protocol for deposition of AgNPs inside cells (in situ internal), ice-cold reductant and oxidant were added in reverse order; that is to say, the pelleted biomass initially suspended in 0.04 M AgNO3 solution and centrifuged for 5 min at 2800g at 4 °C, were re-dissolved in 0.09 M NaBH4 solution.
In the simple mixing SERS, AgNPs were synthesised prior to mixing with cell suspensions. The method described by Creighton,35 was modified as follows: aqueous solutions of 0.09 M NaBH4 and 0.04 M AgNO3 were separately prepared and kept on an ice-bath for 1 h. Ice-cold AgNO3 solution was slowly added dropwise to ice-cold NaBH4 with vigorous stirring in the fume hood. The reaction mixture changed colour from clear solution to milky yellow and finally dark green, confirming successful AgNP production. The resulting solution was left stirring for 15 min to stabilise colloidal NP sizes. The flasks containing colloidal suspensions were wrapped in aluminium foils and stored in the dark prior to SERS analysis. Using UV/Vis spectrophotometry, the absorption maximum for borohydride-reduced AgNPs was detected at 390 nm. These pre-formed AgNPs were mixed with bacterial cell suspension in an optimal volume (μL) ratio of 20
:
1 that resulted in the best SERS signal enhancement.
Raman and SERS spectroscopic analysis
Prior to Raman and SERS measurements, 2 μL sample aliquots were spotted, in a random order, on to clean CaF2 discs and dried for 30 min at room temperature. Spectral analysis was performed in confocal mode on an InVia confocal Raman spectrometer (Renishaw Plc., Gloucestershire, UK) equipped with a 785 nm near infrared diode laser and 600 lines per mm grating, giving a spectral resolution of 6 cm−1 as we reported previously.36 The Raman instrument was calibrated with the phonon band of a Si wafer visualised under a 50× objective (NA = 0.9) and collected as a static spectrum centered at 520 cm−1. For Raman spectroscopy, the laser power was adjusted to 30 mW on the sample with an exposure time of 15 s and 4 spectral accumulations. SERS analysis was carried out by irradiating laser power of 0.3 mW onto dried bacteria-AgNPs samples for 2 s with single spectral accumulation. To account for biological and spectral variations, three spectra were acquired from different positions of each sample spot chosen manually.
MALDI-TOF-MS analysis
Sample preparation for MALDI-TOF-MS followed the protocol reported by AlMasoud and co-workers.30 The matrix was prepared by dissolving 10 mg of sinapinic acid in 500 μL of 2% trifluoroacetic acid (TFA) and 500 μL acetonitrile aqueous solutions. Cell pellets were resuspended in 0.1% TFA aqueous solution and mixed with an equal volume (10 μL) of the matrix and vortexed for 2 s to obtain homogenous solutions. For each matrix-bacteria mixture, 2 μL was immediately spotted on to a MALDI stainless steel plate and air-dried for 1 h before spectral acquisition.
An AXIMA-Confidence MALDI-TOF mass spectrometer (Shimadzu Biotech, Manchester, UK) equipped with a nitrogen pulsed UV laser with 337 nm wavelength was used for data acquisition. The instrument was calibrated with a homogenous mixture of proteins, viz. insulin (5735 Da), cytochrome c (12
362 Da) and apomyoglobin (16
952 Da),25 prior to spectral data measurements from dried bacterial-matrix samples. A total of 100 profiles, each containing 20 shots, were collected using a circular raster pattern and the laser head power set to 120 mV. The MALDI-TOF-MS instrument was operated in the positive ionisation mode (EI) and linear TOF set up, and mass spectra were acquired between 400–12
000 mass-to-charge ratios (m/z). Spectral collection time was ∼3 min per sample.
Data processing and analysis
MATLAB software version 2017a (The MathWorks Inc., Natwick, US) was used for all multivariate statistical analyses. A typical procedure for pre-processing of spectral data was conducted as follows. All acquired Raman, SERS and MALDI-TOF-MS data were separately baseline corrected using the asymmetric least squares (AsLS) algorithm.37 Raman and SERS spectra were scaled using the extended multiplicative signal correction (EMSC),38 whilst mass spectra were log10 scaled prior to multivariate cluster analysis.
The unsupervised modelling of principal component analysis (PCA) was employed to reduce the dimensionality of data, and explore natural differences and similarities between bacteria using Raman and SERS data.39 However, due to the complexity of MALDI-TOF-MS data (101
000 data points × 6 samples × 3 replicates), discriminant function analysis (DFA) was used to discriminate between C. jejuni strain and mutants on the basis of 30 retained principal components (PCs) and a priori knowledge from spectral replicates and strain metadata. DFA minimises within-class and simultaneously maximising between-class variance in a dataset.40 To simplify the interpretation of scores plots, hierarchical cluster analysis (HCA),41 based on mean scores for PCA (Raman and SERS) and DFA (MALDI-TOF-MS) using the Ward's linkage, were generated to visualise the relatedness of mutants through dendrograms.
Results
Understanding biochemical composition and structural dynamics in pathogens is undoubtedly essential for elucidating roles of biomolecules in the development of human gastroenteritis and for probing molecular targets to guide therapeutic development. In this study, we applied Raman spectroscopy, SERS and MALDI-TOF-MS platforms to differentiate and characterise structurally diverse bacterial mutants derived from a single C. jejuni wild type strain based on chemical capabilities and phenotypes. Amongst the investigated bacteria, the kpsC−, kpsS− and kpsM− mutants exhibited closely related phenotypes, which are mediated by the changes in CPS. The CPS is an essential and structurally unique extracellular biomolecule, which is the major determinant of C. jejuni serotype.10 The KpsC, KpsS and KpsM proteins are involved in the production and transportation of CPS to C. jejuni cell surface.42 Another vital extracellular virulence factor is the flagellum,43 which enables cell locomotion. The flagellum consists of a basal body, hook and long filament protruding from the cell surface. The flagellar filament contains several proteins, including FlaA, which facilitates the invasion of epithelial cells and plays a key role in the activation of host immune response.44 Unlike other mutants tested in this study, the flaA− does not have flagellar filaments, due to lack of FlaA protein. In contrast, plgB− expresses unique phenotype driven by the absence of heptasaccharide N-linked glycans in surface proteins. All bacterial mutations are clearly depicted in Fig. 1.
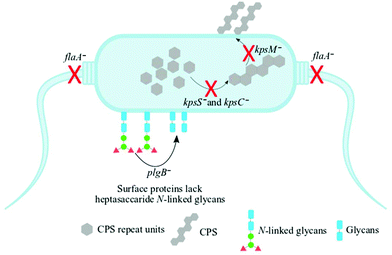 |
| Fig. 1 A schematic representation of the mutants of C. jejuni 11168H strain examined in this study. The kpsS− and kpsC− mutants have disrupted cytoplasmic CPS biosynthesis while kpsM− is capable of biosynthesising cytoplasmic CPS but lacks the transport machinery of cytoplasmic CPS to the cell surface. The flaA− mutant lacks the flagellar filament whilst the plgB− strain contains numerous surface proteins which lack heptasaccharide N-linked glycans. Black arrows and red crosses indicate processes disrupted by the indicated mutations. | |
Fig. 2 shows transmission electron microscope (TEM) images of thin sections of C. jejuni and spectra for AgNPs. Clearly AgNPs were located on the cell envelope when the in situ production method for external AgNPs and simple mixing SERS methods were applied (Fig. 2a and b). By contrast, when AgNPs were synthesised in situ and located internally, the AgNPs were generated by silver ion reduction inside cells to produce Ag(0).
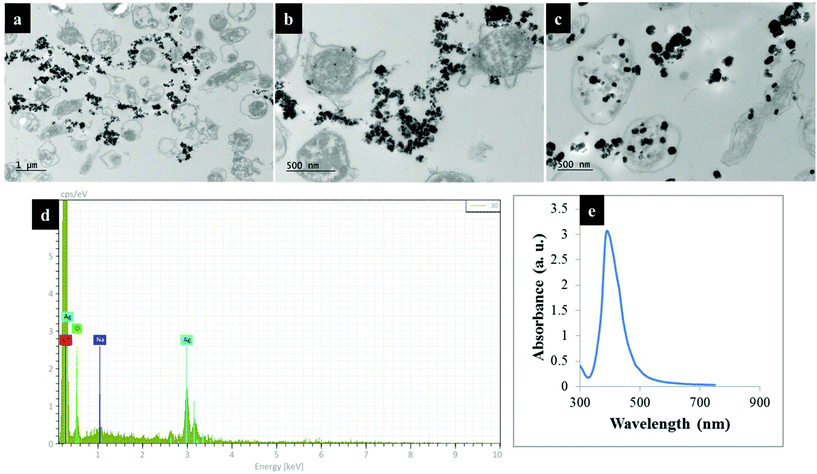 |
| Fig. 2 TEM images of thin section of C. jejuni cells and AgNPs prepared via (a) in situ external, (b) simple mixing and (c) in situ internal SERS protocols; (d) the energy-dispersive X-ray (EDX) spectrum of bacteria-AgNPs indicating the presence of AgNPs in the sample mixtures and (e) UV/Vis plasmon spectrum for pre-formed AgNPs employed for the simple mixing protocols. Bacteria-AgNPs were diluted to reduce the density of NPs and bacterial cells. Thin sections of bacteria were used for TEM imaging to assist with clearer visualisation of AgNPs on bacterial cells. | |
Raman fingerprints
Fig. 3a shows typical information-rich Raman spectral features arising from predominant biochemical components which were used to discriminate bacterial samples. Each Raman spectrum represents a mean of at least 8 spectra recorded from bacterial slurries examined in this study. The prominent Raman band at 1004 cm−1 is assigned to the ring breathing modes of phenylalanine.16 The Raman band at 1340 cm−1 was also observed which is attributed to adenine ring vibrations displayed peak splitting pattern.21 The amide I (C
O, C–N stretching and N–H bending) and amide III (C–N stretching and N–H bending) also showed intense peaks at 1661 and 1250 cm−1, respectively.45 Other bands of low intensity but diagnostically useful were recorded at 1575 cm−1 (ring breathing in guanine and adenine), 1143 cm−1 (C–N and C–C stretching in proteins), 1090 and 540 cm−1 (C–C skeletal and C–O–C stretching modes in glycosidic linkages in polysaccharides) and 783 cm−1 (ring breathing in DNA/RNA) and 965 cm−1 (lipids and polysaccharide modes).45,46
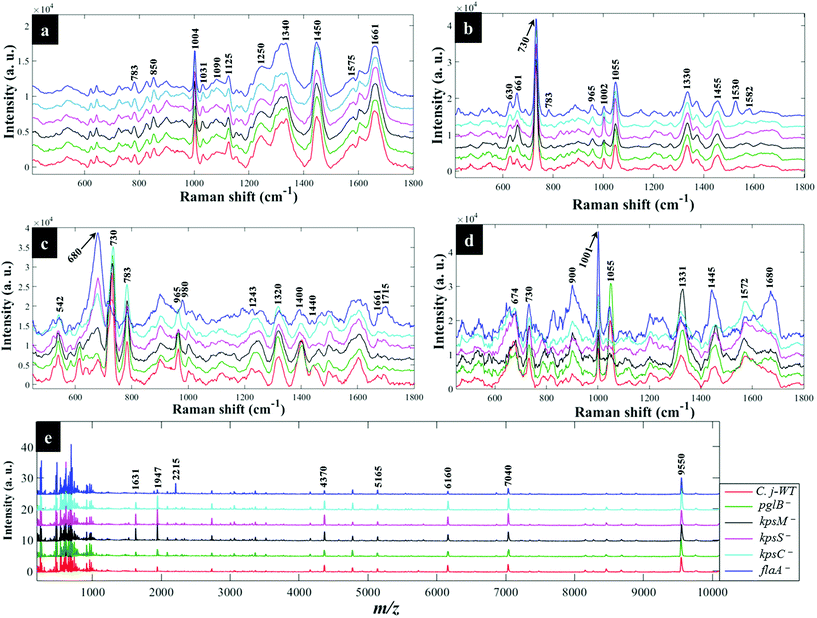 |
| Fig. 3 Typical average spectral data showing characteristic bands measured from C. jejuni and mutants examined in this study: (a) Raman spectra (b) in situ external SERS (c) SERS by simple mixing method (d) in situ internal SERS and (e) MALDI-TOF-MS spectra. The colour of each mean spectrum represents individual strains. Spectra are stacked for clarity. Numbers above spectral peaks represent wavenumbers for the major vibrations associated with differentiation of bacteria. | |
Fig. 4a illustrates PCA scores for C. jejuni NCTC 11168H strain and five derived (near isogenic) mutants (Table 1). Coloured clusters represent individual strain classes listed in Table 1. According to PC1 and PC2, all the strains clustered separately and away from each other; moreover, replicate samples clustered together illustrating excellent reproducibility. Notably, the flaA− mutant was clearly discriminated from the parental wild type C. jejuni (C.j-WT) and other derived mutants along PC1 (Fig. 4a). On the other hand, the C.j-WT and pglB− were clearly separated from other mutants according to PC2. These observations were consistent with HCA dendrograms in Fig. 5a.
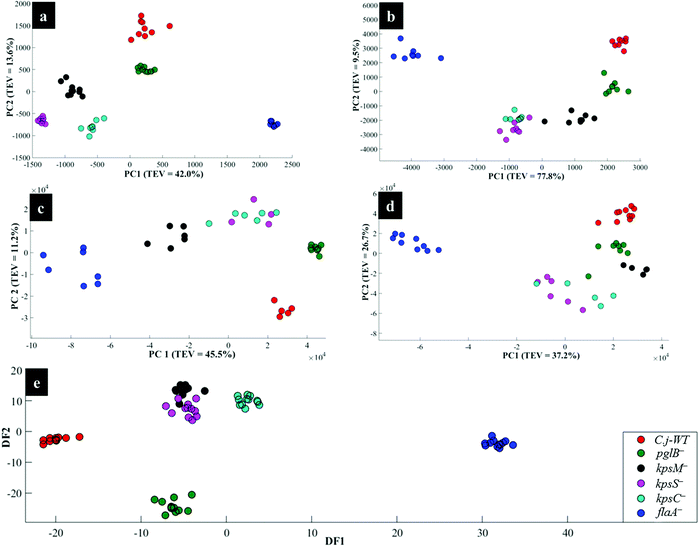 |
| Fig. 4 PCA (a, b, c and d) and DFA (e) scores of spectral data generated by: (a) Raman spectroscopy, (b) in situ external SERS, (c) SERS by simple mixing method (d), in situ internal SERS, and (e) MALDI-TOF-MS of investigated bacteria. Coloured circular symbols represent individual strains, and their designations are provided within the figure. | |
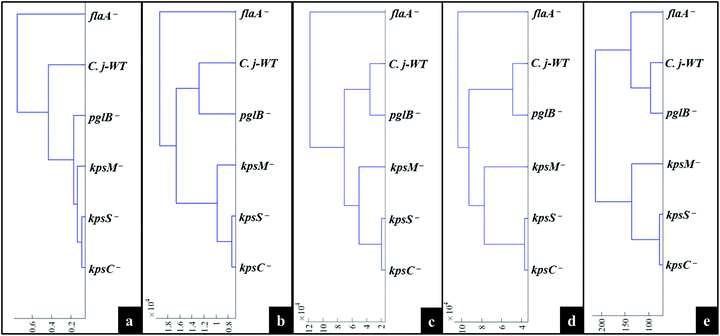 |
| Fig. 5 Representative dendrograms generated by HCA using the averages of PCA (a, b, c and d) and DFA (e) scores of spectral data of bacteria for: (a) Raman spectroscopy, (b) in situ external SERS, (c) SERS spectra by simple mixing method, (d) in situ internal SERS, and (e) MALDI-TOF-MS spectral data. | |
Interestingly, the kpsM−, kpsS− and kpsC− mutants clustered close to each other and clearly separated away from the rest of the bacterial strains along PC1. The clusters for kpsS− and kpsC− are closer to each other and more distant from the kpsM−. This discrimination between these three mutants was explored further by plotting PCA scores (Fig. 6). As shown on Fig. 6a, PC1 clearly separated kpsM− from kpsS− and kpsC− whilst PC2 differentiated between kpsS− and kpsC−. According to the PC1 and PC2 loadings (Fig. S1†), the dominant variable associated with the separation patterns for these three mutants were a redshift (the peak shifted to a lower frequency) for symmetric ring breathing in phenylalanine (1006 to 999 cm−1), adenine (1331 cm−1), polysaccharides (1020–1065 cm−1) and lipids (1461 cm−1). A spectral band detected at 1091 cm−1, which is assigned to C–C skeletal and C–O–C stretching for 1,4-glycosidic linkages in polysaccharides,47 was more intense for kpsM− than the other kps mutants according to PC1 loadings (Fig. S1a†).
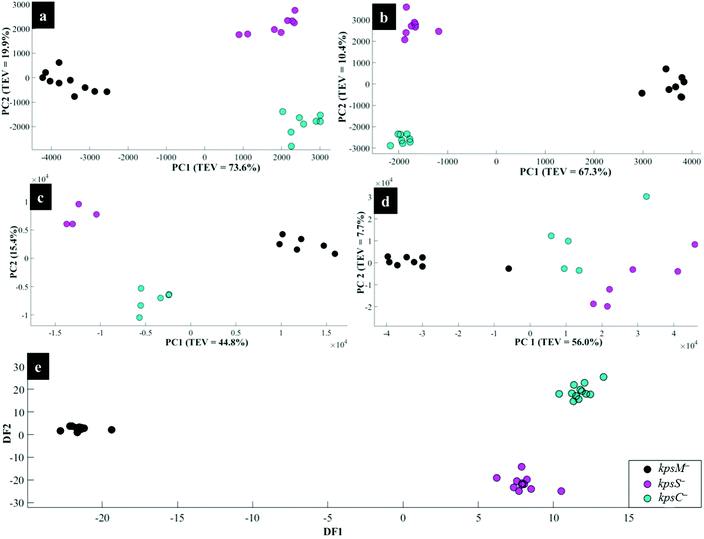 |
| Fig. 6 PCA (a, b, c and d) and DFA (e) scores of spectral data generated using: (a) Raman spectroscopy, (b) in situ external SERS, (c) SERS by simple mixing method, (d) in situ internal SERS, and (e) MALDI-TOF-MS of the kps mutants: kpsM−, kpsS− and kpsC−. The coloured symbols represent individual bacterial classes. | |
Next, the PC loadings plots (Fig. S2†) for Raman data of all the strains examined in this study were constructed to assess the significant variants associated with the clustering trends in the PCA scores plot (Fig. 4a). Examination of PC1 loadings (Fig. S2a†) revealed mainly intensity differences in phenylalanine (1004 cm−1), alkyl CH2 deformations in lipids and proteins (1450 cm−1), and C–C and C–O–C stretching mode of 1,4-glycosidic linkage (1209, 1099 and 856 cm−1) vibrations,21,45 which were higher for C.j-WT, pglB− and flaA−. Other vibrations: C–N and C–C stretching in proteins (1143 cm−1), adenine (1338 cm−1), and adenine and guanine (1575 cm−1) ring modes contributed significantly to strain differentiation on PC1. In addition to PC1 variables, PC2 loadings identified a redshift in phenylalanine ring symmetric breathing (1004–998 cm−1) and COO− stretching in proteins (1400 cm−1) as other explanatory inputs linked to PCA scores distribution in Fig. 4a.
SERS fingerprints
In situ production where nanoparticles are externally located.
For in situ external SERS, AgNPs formed layers around bacterial cells as shown in Fig. 2a. Fig. 3b shows SERS spectra for the in situ external mode which is typically characterised by sharp bands at 661, 730, 1055 and 1330 cm−1 that are usually weak in Raman spectra (Fig. 3a). PCA scores (Fig. 4b) demonstrate similar clustering patterns as the Raman data (Fig. 4a), revealing complete separation of flaA− from the rest of the strains, whilst kpsM−, kpsS− and kpsC− clustered closely and away from C.j-WT and pglB− along PC1 axis. By contrast, C.j-WT, pglB− and flaA− strains were separated from the other mutants based on PC2 axis. By visual inspection, the SERS spectra for flaA− exhibited extra vibrational modes for DNA/RNA nucleobases at 783 cm−1, proteins at 1149 and 1582 cm−1 (C–N stretching), lipid modes at 1530 cm−1 (C–H bending and C
C stretching) and combination modes in the deep fingerprint region (420–700 cm−1) which are dominated by carbohydrates and nucleic acids.48,49 The PCA (Fig. 4b) were consistent with HCA grouping patterns on Fig. 5b.
As in Raman data analysis (Fig. 6a), kps mutants were analysed separately by PCA scores (Fig. 6b); where PC1 plane, again, separated kpsM− from kpsS− and kpsC− mutants whilst PC2 discriminated between kpsS− and kpsC−. The PC1 loadings (Fig. S3a†) particularly for the three mutants highlighted more intense SERS bands for kpsS− and kpsC− at 730 cm−1 (adenine moiety in flavin adenine dinucleotide (FAD),23,50 and glycosidic ring breathing in N-acetylmuramic acid (NAM) and N-acetyl-D-glucosamine (NAG) located in the cell wall),51 1002 cm−1 (ring breathing in phenylalanine) and 1049 cm−1 (PO2− stretching in FAD, phosphodiester and adenosine triphosphate (ATP)),48,52 whilst the kpsM− mutant produced a more intense band at 661 cm−1 (COO− and C–C bending modes in carbohydrates/proteins) and lowest level of phenylalanine as suggested by a weak band at 999 cm−1 (Fig. 3b).53–55 Additionally, PC2 loadings (Fig. S3b†) detected the bands at 661 and 730 cm−1 which were rather more intense for kpsS− than kpsC−, as significant variables associated with separation trends on PC2. These band intensity differences especially for carbohydrates and proteins for in situ external SERS spectra are consistent with Raman spectra discussed above.
Assessment of PC1 and PC2 loadings for all in situ external SERS data showed interesting trends. PC1 loadings (Fig. S4a†) revealed intensity differences for the bands at 661 cm−1 (carbohydrates/proteins), 783 cm−1 (DNA/RNA bases), and 860 cm−1 (C–O–C ring modes in ribose sugars) exhibited greater intensities in kpsM− and flaA− than the remaining strains. However, kpsS− displayed more intense signals at 999 cm−1 (phenylalanine) and 1055 cm−1 (PO2− stretching in ATP, FAD).56 PC2 loadings (Fig. S4b†) also indicated that kpsM− and flaA− had relatively intense vibrations at 661 cm−1 (carbohydrates/proteins) and a blueshift (shift to higher frequency) at 730–738 cm−1 (FAD, NAM, NAG). By comparison, intense peaks at 1149, 1530 and 1582 cm−1 on PC2 loadings (Fig. S4b†) were only present on the spectrum of flaA−, and these peaks are clearly visible on SERS spectra in Fig. 3b.
SERS by simple mixing method of bacteria with pre-synthesised AgNPs.
The PCA (Fig. 4c) for SERS data involving simple mixing (Fig. 3c) revealed distinctive clustering patterns mediated mainly by cellular constituents on the cell surface (Fig. 2b). The flaA− strain clustered alone, and away from the remaining samples along PC1. Similarly, kps mutants clustered tightly whilst C.j-WT and pglB− mutants were separated along PC1. By contrast, PC2 discriminated C.j-WT, pglB− and flaA− from kps mutants (Fig. 4c). The HCA dendrograms (Fig. 5c) confirmed these relationships, and were also in agreement with in situ external SERS data (Fig. 5b).
When the kps mutants were analysed separately by PCA (Fig. 6c), kpsM− separated from kpsS− and kpsC− on PC 1, whilst PC2 differentiated kpsS− from kpsC−. The clustering trends on PCA were mainly based on 680 cm−1 (guanine ring and C–S stretching) that was stronger in kpsS− and kpsC− than kpsM− mutant whilst the 730 cm−1 band (FAD, NAM, NAG) was less intense in kpsS− than the other kps bacteria as shown by PC1 loadings (Fig. S5a†). Moreover, a blueshift at 965–980 cm−1 (C–N stretching in proteins),56 was detected. PC2 included strong modes at 783 cm−1 (DNA/RNA), 1400 cm−1 (COO− stretching in proteins), 1440 cm−1 (CH2 deformation in lipids), and a redshift at 730–738 cm−1 as the differential variants for the PCA clusters (Fig. 6c).
Based on PC1 loadings (Fig. S6a†) for all spectral data measured via simple mixing, flaA−, kpsS− and kpsC− cells registered an appreciable band at 680 cm−1, whilst flaA− also exhibited a unique band at 1715 cm−1 (C
O in esters).55 Unlike flaA− mutant, the remaining five strains exhibited an intense discriminatory peak related to adenine vibrations at 730 cm−1. Other significant bands shown by PC1 and PC2 loadings (Fig. S6†) were 783 cm−1 (ring breathing in nucleobases), 1243 cm−1 (C–N stretching, N–H bending in amide III), 1320 cm−1 (ring modes in adenine), 1400 (COO− in proteins) and 1661 cm−1 (C
O, C–N stretching, and N–H bending in amide I), a spectral blueshift at 965–980 cm−1 (C–N stretching in proteins) as well as redshift at 730–738 cm−1 (adenine ring modes).48,57
In situ production where nanoparticles are located internally in the bacteria.
Interestingly, PCA scores for the in situ internal protocol (Fig. 4d) displayed the same clustering patterns as SERS methods discussed above (in situ external production and simple mixing), in spite of detecting different discriminatory peaks: external bacterial components compared with internal molecules. PC1 separated all the strains (Fig. 4), with flaA− strain forming a single member cluster distinct from C.j-WT and the other derived mutants. PC2 isolated three kps mutants from other strains. All these clusters of investigated C. jejuni cells were grouped accordingly by HCA dendrograms shown in Fig. 5d.
Separate PCA (Fig. 6d) for in situ internal SERS data of kps mutants were also generated to assess any unique differential bands present for intracellular molecules (Fig. 2c). It was discovered that PC1 loadings (Fig. S7a†) were dominated by strong vibrations at 674 cm−1 (amino acids) and 1001 cm−1 (phenylalanine) bands, which were stronger for kpsS− and kpsC− compared to kpsM− cells. Conversely, the band at 1331 cm−1 (adenine modes) was apparently much stronger for kpsM−. Other explanatory variants detected at 730 cm−1 (FAD, NAM and NAG), 1445 cm−1 (CH2 in lipids),23,24 were demonstrated in PC2 loadings (Fig. S7b†). The internal spectrum of flaA− mutant displayed a unique band at 1680 cm−1 (C
O, C–N stretching and N–H bending in proteins), which was clearly absent from all other strains.
For all the in situ internal SERS data combined, the PC1 loadings (Fig. S8a†) identified the salient peak at 998 (phenylalanine) that was most intense in flaA− compared to the rest of the strains, whereas the band at 1055 cm−1 (PO2− in ATP, phosphodiester and FAD) was apparently not detected in kps mutants. Besides the bands revealed by PC1 loadings, peaks at 734 and 1331 cm−1 (FAD, NAG, NAM) with varying intensities were detected, with the highest peaks being recorded for kpsM− according to PC2 loadings on Fig. S8b.†
MALDI-TOF-MS analysis.
In addition, a different physicochemical method was used which targets the proteins found within bacteria. As shown in Fig. 4e, DFA scores of MALDI-TOF-MS data revealed clear differentiation of C. jejuni NCTC 11168H and the five derived isogenic mutants. Consistent with Raman and SERS spectra, DFA scores displayed clear separation of the flaA− strain from the remaining bacteria according to DF1. By contrast, DF2 revealed the separation of kps mutants from the parental wild type strain and other mutants, which again is in complete agreement with vibrational spectroscopy. Similar to the Raman and SERS data, the HCA dendrograms for the mean DFA scores generated from MALDI-TOF-MS data (Fig. 5e) clustered the kps samples together and separately from the wild type and other mutants. However, as opposed to vibrational spectral data, MALDI-TOF-MS data classified C.j-WT, pglB− and flaA− within the same clade but on completely different branches (Fig. 5e).
The DFA scores generated from MALDI-TOF-MS data for kps cells (Fig. 6e) separated kpsM− from kpsS− and kpsC− along DF1, and between kpsS− and kpsC− along DF2. DF1 loadings (Fig. S9†) for kps mutants only indicated more intense peaks at 1631, 1947 and 7040 m/z ratios32 for kpsS− and kpsC−. Conversely, the molecular ions at 4778 and 9550 m/z were more intense in kpsM−. DF2 loadings (Fig. S9a†) showed stronger bands at m/z 2734, 4370 and 6160 for the kpsS− and kpsC− relative to kpsM−.
The DFA loadings for MALDI-TOF-MS data of all bacterial cells (Fig. S10†) identified ions of varying intensities in the m/z 1631–9550 range. The peaks for molecular ions with m/z 4370 and 6160 were very weak in the flaA− mutant but strongly intense in the rest of the strains. By contrast, the band with m/z 2215 was very intense in flaA− mutant but extremely weak in the other five strains. However, biomarker bands detected at m/z 5165, 7040 and 9550, which are tentatively linked to various bacterial ribosomal and membrane proteins,58 displayed similar intensities in C. jejuni and mutants.
Discussions
The aim of this study was to characterise bacterial mutants of a single C. jejuni strain that express different phenotypes. The five mutants exhibited molecular changes in extracellular biocomponents (viz. polysaccharides and proteins) on the cell wall surface as a consequence of gene mutations as described in Fig. 2. Thus, three rapid fingerprinting tools (Raman, SERS and MALDI-TOF-MS) aimed to access this intracellular and extracellular biochemical information in C. jejuni strains. In particular, the simple mixing and in situ external SERS methods were designed to provide structural and abundance dynamics of molecules present on the cell wall (e.g. FAD, LPS, etc.), whilst in situ internal SERS revealed complementary spectral information from molecules located in the cytoplasm (e.g. amino acids, DNA/RNA) of cells. In addition, since gene mutations resulted in changes in the abundance, identity and structure of specific cell surface proteins; MALDI-TOF-MS was specially applied to give deeper insights into changes in proteins in the examined mutants. The SERS trilogy (simple mixing and two in situ methods) and MALDI-TOF-MS tools were further complemented by Raman spectroscopy, which provides whole-organism biochemical fingerprint. However, the spectra obtained from the applied techniques are multidimensional by nature, and so the discrimination of bacteria by visual screening only was difficult. This emphasised the need to apply unsupervised (PCA) and supervised (DFA) analyses for dimensionality reduction to guide differentiation of bacteria. Despite exhibiting different selection rules for biochemical detection,59,60 spectral data acquired from Raman, SERS and MALDI-TOF-MS differentiated between the parental strain and derived mutants examined in this study. The PCA and DFA scores generated from respective spectral data consistently separated flaA− from the remaining mutants (Fig. 4). The HCA dendrograms constructed from the averages of PCA and DFA scores also confirmed separate groupings; where flaA− formed a single isolated group, whilst the kps mutants consistently formed a separate three-member grouping which clustered away from C.j-WT and pglB−. Raman differentiated C.j-WT from pglB− (Fig. 5a) as opposed to SERS and MALDI-TOF-MS, which classified these two strains in the same group. Although C.j-WT, pglB− and flaA− were separated based on phenotypic dynamics, MALDI-TOF-MS data assigned these strains under one grouping as shown by HCA dendrograms (Fig. 5e).
As evidenced by dynamics in spectral features, the clustering trends on PCA and DFA scores and HCA grouping patterns were as a result of chemical and structural differences between the wild type strain and five derived isogenic mutants. The flaA− mutant lacks single bipolar unsheathed helical flagella (Fig. 1) making it non-motile, and clearly different from other strains. The flaA− mutant lacks the flagellar filament FlaA protein, and so it can assemble a basal body type structure in the inner and outer cell membrane but no long filament type structure is produced. MALDI-TOF-MS spectra and corresponding loadings plots revealed diagnostic peaks at m/z 4370 and 6160 that are very weak in the spectrum of flaA− mutant but, by far, strongly intense in C.j-WT, plgB− and kps strains. These molecular ions contributed significantly to the clustering patterns observed on the PCA and DFA (Fig. 4). In contrast, the mutants in kps genes (kpsC−, kpsM− and kpsS−) that abolish capsule formation consistently clustered closely together. Further investigation of these three mutants by PCA (Raman and SERS) and DFA (MALDI-TOF-MS) scores (Fig. 6) highlighted clear separations among them due to phenotypic variations. That is to say, whilst KpsS and KpsC proteins play vital functions in the biosynthesis and assembly of cytoplasmic CPS before transport, the KpsM protein is a component of the export machinery for transporting CPS across the bacterial inner membrane as depicted in Fig. 1.11,61 To be more specific, KpsS and KpsC are 3-deoxy-D-manno-oct-2-ulosonic acid (Kdo) transferases that add initial Kdo residues to the phospholipid carrier on the cytoplasmic face of inner membrane prior to addition of CPS and transport to the cell surface.11,62 Thus, kpsM− mutant is expected to accumulate cytoplasmic phospholipid-linked CPS which is only blocked in transport but not in biosynthesis.42 Conversely, kpsS− and kpsC− strains would, to a large extent, not accumulate phospholipid-linked CPS since lipid acceptors need priming with Kdo residues, though they may accumulate unlinked CPS in the cytoplasm,63 as evidenced by the presence of polysaccharide Raman and SERS bands in the fingerprint region (400–900 cm−1). The Raman (Fig. S1†) and in situ internal SERS (Fig. S7†) data also revealed diagnostic peaks measured from intracellular polysaccharides and proteins for kps cells that contributed to the separations observed on PCA and DFA (Fig. 4). These bands were predominantly differentiated via intensity variations between 400 and 900 cm−1, and at 1050 cm−1; the spectral region linked to polysaccharide vibrations.45,64 The simple mixing and in situ external SERS also displayed similar bands in the same frequency range (Fig. S3 and S5†); however, these bands were predominantly derived from cell wall molecules (Fig. 2a and b). On the other hand, C.j-WT exhibits natural phenotype as C. jejuni is found in nature, which is why it formed a distinctive cluster. By contrast, pglB− clustered separately by virtue of having non-functional N-linked oligosaccharyltransferase enzyme, resulting in numerous cell surface proteins lacking one or more heptasaccharide N-linked glycans (Fig. 1).65 The mutants investigated in this study were constructed through the insertion of a kanamycin resistance gene, and this may potentially contribute to the discrimination from the C.j-WT strain, which does not contain this cassette. Nonetheless, the kanamycin resistance gene was present in all the mutants and thus it should not contribute to differentiation amongst them.
Previous research indicates that several tools such as pyrolysis MS,66–68 Fourier-transform infrared (FT-IR),25,69–72 and UV resonance Raman (UVRR)73–75 are widely used in parallel with multivariate analysis for accurate identification of bacteria. Among spectroscopic tools, Raman and SERS are powerful sampling platforms capable of generating phenotypes from slurry and single cells non-destructively,41,47,76–78 and in situ in aqueous biological systems where pathogens are naturally found.79–81 By combining molecular sensitivity, selectivity and specificity, Raman and SERS are perhaps ideally suitable for rapid detection of fastidious microbes, including Campylobacter spp.16,25,82 Like Raman spectroscopy, SERS methods applied in the present study are label-free, implying that plasmonic substrates were in direct contact with cellular biomolecules. Hence, SERS spectra represent real bacterial phenotypes determined by underlying biological processes, which differs from indirect SERS based on Raman reporters and recognition elements e.g. dyes, antibodies.83 Despite showing potential for bacterial detection, label-free SERS demands careful optimisation of protocols to ensure clinically desirable robustness and reproducibility,84,85 and especially so when differentiating closely related pathogens. In this study, we designed SERS approaches in which AgNPs were synthesised in situ (in the presence of cells) to improve chemical interactions with molecules, resulting in uniform spectra of negligible fluorescence backgrounds.24
SERS involving in situ assays employing various reductants and oxidants as precursors have been exploited for bacterial detection,34,86,87 since pioneering work by Efrima and Zeiri.23 Recently, Chen and co-workers also reported in situ near infrared-SERS probes designed to access cell wall and intracellular biomolecules for clinical isolates.64 A key limitation of this method was that the SERS spectra for in situ internal strategy displayed only two weak spectral bands at 890 and 1040 cm−1.64 By contrast, three SERS assays reported here using the same precursors for AgNPs synthesis recorded multi-variable spectra consisting of intense peaks which proved to be practical for discriminating C. jejuni pathogens.
The strains examined in this study exhibited varied molecular composition and chemical bond linkages in biomolecules present in the cytoplasm and cell envelope as a result of mutations.11 Our SERS assays with NPs localised within extra or intracellular parts of cells were meant, in principle, to capture this characteristic chemical information and associated phenotypes in an untargeted way, given that AgNPs have high affinity for electronegative atoms (N, O, S, etc.) naturally present in biomolecules.88 For example, our SERS protocols proved to be sensitive towards nitrogen and oxygen-rich FAD and adenine-related vibrations detected at 730, 1320 and 1330 cm−1 (Fig. 3b and c). FAD is a co-enzyme which regulates enzymatic redox metabolic reactions such as respiration,89 and N- or O-linked glycosylation processes related to virulence factors of the pathogens.65,90 In addition, SERS complemented Raman data by providing insights into molecular dynamics in LPS; the major component of the outer membrane of Gram-negative cells, which mediates adhesion of bacteria to human epithelial cells.91 Considering that the cell wall is the part of a bacterium which is in direct contact with the human gut during microbial infection, elucidating surface molecular composition and structural dynamics of pathogens by SERS may be crucial for predicting the induction and extent of gastroenteritis, differential diagnostics and choice of therapeutics for infections induced by closely related etiological agents,92 as demonstrated for methicillin-resistant and sensitive Staphylococcus aureus.93 The SERS assays used in this study generated similar PCA scores and HCA dendrograms, though the spectra were derived from different molecular moieties as evidenced by dynamic and diverse bands. This not only indicated improved reproducibility of these techniques, and robust classification accuracy at strain level, which are comparable to other biosensors.12,94 Whilst MALDI-TOF-MS is destructive, it has established workflow, rapid library search and identification of protein masses measured from clinical isolates.95 Importantly, MALDI-TOF-MS offers a snapshot of essential protein biomarkers in the proteome and transcriptome that regulate physiological and metabolic functions of bacteria,96 with similar accuracy level as conventional techniques such as 16S rRNA sequencing,25 and Vitek 2™ systems.97
Therefore, in clinical diagnostics Raman, SERS and MALDI-TOF-MS optical tools represent an exciting holistic approach with considerable potential for conveying simultaneously, complementary and confirmatory phenotypes for C. jejuni,13 and how such cellular properties may affect the interactions with the human gut. It is also worth noting that all analytical tools applied in this study showed distinctive advantages over traditional and molecular methods. Firstly, they are rapid (spectral acquisition time: 15 s, 2 s and 3 min for Raman, SERS and MALDI-TOF-MS, respectively), making them ideal for high-throughput screening of many infectious pathogens be it at genus, species or subspecies level. Secondly, they employ simple sample preparation methods, and obtain information-rich biochemical information from small sample volumes, as low as 1 pL (Raman and SERS). Since Raman and SERS may preserve cell integrity, bacteria can potentially be re-cultivated and used for further downstream studies such as genomics, lipidomics and metabolomics.25,98
Conclusions
We have demonstrated the applicability of Raman spectroscopy, SERS and MALDI-TOF-MS for intra-strain level discrimination of mutants of the bacterium C. jejuni analysis. SERS involving in situ external and internal deposition of AgNPs were successfully used to probe extra- and intracellular polysaccharides and proteins present in the parental C. jejuni strain and its mutants. Interestingly, all analytical techniques applied in this study clearly differentiated among near isogenic mutants of the foodborne pathogen C. jejuni. Multivariate analysis of spectra via PCA (Raman and SERS) and DFA (MALDI-TOF-MS) scores revealed distinctive clusters indicating phenotypic dynamics for individual mutants, which were confirmed by HCA dendrograms. In addition, using Raman, SERS and MALDI-TOF-MS, we discriminated between three kps mutants which express similar phenotypes. The major MALDI-TOF-MS biomarkers that are, perhaps, related to flagellin protein (FlaA) fragments were detected at m/z 4370 and 6160. These peaks were very weak in flaA− mutant compared to C.j-WT, plgB− and kps strains. Other molecular ions detected on MALDI-TOF-MS spectra had similar intensities for all the strains examined in this study, suggesting that capsular polysaccharides did not contribute significantly to the differentiation of mutants. Nevertheless, various polysaccharides signals were detected by SERS measured via in situ approaches, and these were important variables in the characterisation of mutants. Based on the biochemical variations of near isogenic mutants and subsequent spectral findings, Raman and SERS exhibited greater specificity and rapidity. Collectively, our fingerprinting platforms represent powerful integrated techniques which may be suitable for rapid and accurate mutant verification and testing of clinically relevant pathogens for spontaneous loss of flagella or capsule within clinical and epidemiological food poisoning research.
Conflicts of interest
The authors have no conflicts of interest to declare.
Acknowledgements
MC thanks the Commonwealth Scholarship Commission (ZMCA-2016-152), UK for PhD funding. HM and RG thank the European Commission's Seventh Framework Program for funding (STREPSYNTH; Project No. 613877). RG is indebted to UK BBSRC (BB/L014823/1) for funding for Raman spectroscopy. HM thanks the University of Liverpool for funding and support. We thank Emma Enston in the Department of Chemistry at the University of Manchester for assisting with MALDI-TOF-MS sample measurements.
References
- World Health Organisation, Available online: https://www.who.int/en/news-room/fact-sheets/detail/campylobacter (accessed on 13 August, 2019).
- D. I. Ellis, H. Muhamadali, M. Chisanga and R. Goodacre, Encycl. Food Chem., 2019, 1, 364–370 CrossRef.
- D. Y. He, Z. Z. Wu, B. Cui, E. B. Xu and Z. Y. Jin, Food Chem., 2019, 289, 708–713 CrossRef CAS PubMed.
- European Food Safety and European Centre for Disease Prevention and Control, EFSA J., 2018, 16, 5500–5761 Search PubMed.
- European Food Safety and European Centre for Disease Prevention and Control, EFSA J., 2016, 14, 4634–1664 Search PubMed.
- European Food Safety and European Centre for Disease Prevention and Control, EFSA J., 2015, 13, 4329–4518 Search PubMed.
- E. Frirdich, J. Biboy, M. Pryjma, J. Lee, S. Huynh, C. T. Parker, S. E. Girardin, W. Vollmer and E. C. Gaynor, Mol. Microbiol., 2019, 112, 280–301 CrossRef CAS PubMed.
- J. Parkhill, B. W. Wren, K. Mungall, J. M. Ketley, C. Churcher, D. Basham, T. Chillingworth, R. M. Davies, T. Feltwell, S. Holroyd, K. Jagels, A. V. Karlyshev, S. Moule, M. J. Pallen, C. W. Penn, M. A. Quail, M. A. Rajandream, K. M. Rutherford, A. H. M. van Vliet, S. Whitehead and B. G. Barrell, Nature, 2000, 403, 665–668 CrossRef CAS PubMed.
- J. Tian, C. F. Cao, T. Li, K. Zhang, P. F. Li, Y. L. Liu and X. Y. Liu, Front. Neurol., 2019, 10, 714–720 CrossRef PubMed.
- O. Sahin, S. A. Terhorst, E. R. Burrough, Z. Q. Shen, Z. W. Wu, L. Dai, Y. Z. Tang, P. J. Plummer, J. Ji, M. J. Yaeger and Q. J. Zhang, Infect. Immun., 2017, 85, 1–15 CrossRef PubMed.
- L. M. Willis and C. Whitfield, Proc. Natl. Acad. Sci. U. S. A., 2013, 110, 20753–20758 CrossRef CAS PubMed.
- O. Lazcka, F. J. Del Campo and F. X. Munoz, Biosens. Bioelectron., 2007, 22, 1205–1217 CrossRef CAS PubMed.
- Y. H. Liu, W. Yamazaki, Y. T. Huang, C. H. Liao, W. H. Sheng and P. R. Hsueh, J. Microbiol., Immunol. Infect., 2019, 52, 122–131 CrossRef PubMed.
- D. Martiny, A. Dediste, L. Debruyne, L. Vlaes, N. B. Haddou, P. Vandamme and O. Vandenberg, Clin. Microbiol. Infect., 2011, 17, 1001–1006 CrossRef CAS PubMed.
- D. Q. Zhong and W. Q. He, J. Nanosci. Nanotechnol., 2019, 19, 5517–5521 CrossRef PubMed.
- M. Chisanga, H. Muhamadali, D. I. Ellis and R. Goodacre, Appl. Spectrosc., 2018, 72, 987–1000 CrossRef CAS PubMed.
- O. Samek, H. H. Telle, L. G. Harris, M. Bloomfield and D. Mack, Laser Phys. Lett., 2008, 5, 465–470 CrossRef CAS.
- M. J. Tang, G. D. McEwen, Y. Z. Wu, C. D. Miller and A. H. Zhou, Anal. Bioanal. Chem., 2013, 405, 1577–1591 CrossRef CAS PubMed.
- J. B. Xu, L. Preciado-Llanes, A. Aulicino, C. M. Decker, M. Depke, M. G. Salazar, F. Schmidt, A. Simmons and W. E. Huang, Anal. Chem., 2019, 91, 7729–7737 CrossRef CAS PubMed.
- K. S. Lee, M. Palatinszky, F. C. Pereira, J. Nguyen, V. I. Fernandez, A. J. Mueller, F. Menolascina, H. Daims, D. Berry, M. Wagner and R. Stocker, Nat. Microbiol., 2019, 4, 1035–1048 CrossRef CAS PubMed.
-
W. E. Huang, M. Q. Li, R. M. Jarvis, R. Goodacre and S. A. Banwart, in Advances in Applied Microbiology, ed. A. I. Laskin, S. Sariaslani and G. M. Gadd, Elsevier Academic Press Inc, San Diego, 2010, vol. 70, pp. 153–186 Search PubMed.
- S. Schlucker, Angew. Chem., Int. Ed., 2014, 53, 4756–4795 CrossRef PubMed.
- S. Efrima and L. Zeiri, J. Raman Spectrosc., 2009, 40, 277–288 CrossRef CAS.
- L. Zeiri, B. V. Bronk, Y. Shabtai, J. Eichler and S. Efrima, Appl. Spectrosc., 2004, 58, 33–40 CrossRef CAS PubMed.
- H. Muhamadali, D. Weaver, A. Subaihi, N. AlMasoud, D. K. Trivedi, D. I. Ellis, D. Linton and R. Goodacre, Analyst, 2016, 141, 111–122 RSC.
- R. E. Mandrell, L. A. Harden, A. Bates, W. G. Miller, W. F. Haddon and C. K. Fagerquist, Appl. Environ. Microbiol., 2005, 71, 6292–6307 CrossRef CAS PubMed.
- M. Alispahic, K. Hummel, D. Jandreski-Cvetkovic, K. Nobauer, E. Razzazi-Fazeli, M. Hess and C. Hess, J. Med. Microbiol., 2010, 59, 295–301 CrossRef CAS PubMed.
- N. AlMasoud, Y. Xu, D. K. Trivedi, S. Salivo, T. Abban, N. J. W. Rattray, E. Szula, H. AlRabiah, A. Sayqal and R. Goodacre, Anal. Bioanal. Chem., 2016, 408, 7865–7878 CrossRef CAS PubMed.
- T. Krishnamurthy, P. L. Ross and U. Rajamani, Rapid Commun. Mass Spectrom., 1996, 10, 883–888 CrossRef CAS PubMed.
- N. AlMasoud, Y. Xu, D. I. Ellis, P. Rooney, J. F. Turton and R. Goodacre, Anal. Methods, 2016, 8, 7603–7613 RSC.
- S. Savas, G. Hazirolan, A. Karagoz and M. Parlak, J. Microbiol. Methods, 2019, 162, 62–68 CrossRef CAS PubMed.
- P. Lasch, D. Jacob, R. Grunow, T. Schwecke and J. Doellinger, TrAC, Trends Anal. Chem., 2016, 85, 103–111 CrossRef CAS.
- N. AlMasoud, Y. Xu, N. Nicolaou and R. Goodacre, Anal. Chim. Acta, 2014, 840, 49–57 CrossRef CAS PubMed.
- M. Chisanga, H. Muhamadali, R. Kimber and R. Goodacre, Faraday Discuss., 2017, 205, 331–343 RSC.
- J. A. Creighton, C. G. Blatchford and M. G. Albrecht, J. Chem. Soc., Faraday Trans. 2, 1979, 75, 790–798 RSC.
- H. Muhamadali, M. Chisanga, A. Subaihi and R. Goodacre, Anal. Chem., 2015, 87, 4578–4586 CrossRef CAS PubMed.
- P. H. C. Eilers, Anal. Chem., 2004, 76, 404–411 CrossRef CAS PubMed.
- H. Martens, J. P. Nielsen and S. B. Engelsen, Anal. Chem., 2003, 75, 394–404 CrossRef CAS PubMed.
- P. Geladi, B. Sethson, J. Nystrom, T. Lillhonga, T. Lestander and J. Burger, Spectrochim. Acta, Part B, 2004, 59, 1347–1357 Search PubMed.
- H. J. H. Macfie, C. S. Gutteridge and J. R. Norris, J. Gen. Microbiol., 1978, 104, 67–74 CrossRef CAS PubMed.
- Z. Q. Lin, X. Zhao, J. Q. Huang, W. Liu, Y. T. Zheng, X. Yang, Y. Zhang, M. L. de la Chapelle and W. L. Fu, Analyst, 2019, 144, 2803–2810 RSC.
- A. V. Karlyshev, D. Linton, N. A. Gregson, A. J. Lastovica and B. W. Wren, Mol. Microbiol., 2000, 35, 529–541 CrossRef CAS PubMed.
- M. E. Konkel, J. D. Klena, V. Rivera-Amill, M. R. Monteville, D. Biswas, B. Raphael and J. Mickelson, J. Bacteriol., 2004, 186, 3296–3303 CrossRef CAS PubMed.
- I. A. Hajam, P. A. Dar, I. Shahnawaz, J. C. Jaume and J. H. Lee, Exp. Mol. Med., 2017, 49, 373–387 CrossRef PubMed.
- K. Maquelin, C. Kirschner, L. P. Choo-Smith, N. van den Braak, H. P. Endtz, D. Naumann and G. J. Puppels, J. Microbiol. Methods, 2002, 51, 255–271 CrossRef CAS PubMed.
- J. De Gelder, K. De Gussem, P. Vandenabeele and L. Moens, J. Raman Spectrosc., 2007, 38, 1133–1147 CrossRef CAS.
- S. Jaafreh, O. Valler, J. Kreyenschmidt, K. Gunther and P. Kaul, Talanta, 2019, 202, 411–425 CrossRef CAS PubMed.
- A. Walter, A. Marz, W. Schumacher, P. Rosch and J. Popp, Lab Chip, 2011, 11, 1013–1021 RSC.
- M. Kahraman, M. M. Yazici, F. Sahin, O. F. Bayrak and M. Culha, Appl. Spectrosc., 2007, 61, 479–485 CrossRef CAS PubMed.
- P. Kubryk, R. Niessner and N. P. Ivleva, Analyst, 2016, 141, 2874–2878 RSC.
- R. M. Jarvis and R. Goodacre, Anal. Chem., 2004, 76, 40–47 CrossRef CAS PubMed.
- W. R. Premasiri, J. C. Lee, A. Sauer-Budge, R. Theberge, C. E. Costello and L. D. Ziegler, Anal. Bioanal. Chem., 2016, 408, 4631–4647 CrossRef CAS PubMed.
- M. Graff and J. Bukowska, Vib. Spectrosc., 2010, 52, 103–107 CrossRef CAS.
- K. Kneipp, A. S. Haka, H. Kneipp, K. Badizadegan, N. Yoshizawa, C. Boone, K. E. Shafer-Peltier, J. T. Motz, R. R. Dasari and M. S. Feld, Appl. Spectrosc., 2002, 56, 150–154 CrossRef CAS.
- Y. F. Xie, L. Xu, Y. Q. Wang, J. D. Shao, L. Wang, H. Y. Wang, H. Qian and W. R. Yao, Anal. Methods, 2013, 5, 946–952 RSC.
- M. Culha, A. Adiguzel, M. M. Yazici, M. Kahraman, F. Sahin and M. Gulluce, Appl. Spectrosc., 2008, 62, 1226–1232 CrossRef CAS PubMed.
- A. Sengupta, M. Mujacic and E. J. Davis, Anal. Bioanal. Chem., 2006, 386, 1379–1386 CrossRef CAS PubMed.
- D. Ziegler, J. F. Pothier, J. Ardley, R. K. Fossou, V. Pfluger, S. de Meyer, G. Vogel, M. Tonolla, J. Howieson, W. Reeve and X. Perret, Appl. Microbiol. Biotechnol., 2015, 99, 5547–5562 CrossRef CAS PubMed.
-
E. Smith and G. Dent, Modern Raman Spectroscopy: A Practical Approach, Blackwell Science Publ, Oxford, 2005 Search PubMed.
- K. S. Jang and Y. H. Kim, J. Microbiol., 2018, 56, 209–216 CrossRef CAS PubMed.
- L. M. Willis and C. Whitfield, Carbohydr. Res., 2013, 378, 35–44 CrossRef CAS PubMed.
- C. R. H. Raetz and C. Whitfield, Annu. Rev. Biochem., 2002, 71, 635–700 CrossRef CAS PubMed.
- G. P. Rigg, B. Barrett and I. S. Roberts, Microbiology, 1998, 144, 2905–2914 CrossRef CAS PubMed.
- L. Y. Chen, N. Mungroo, L. Daikuara and S. Neethirajan, J. Nanobiotechnol., 2015, 13, 45–53 CrossRef PubMed.
- J. Kelly, H. Jarrell, L. Millar, L. Tessier, L. M. Fiori, P. C. Lau, B. Allan and C. M. Szymanski, J. Bacteriol., 2006, 188, 2427–2434 CrossRef CAS PubMed.
- D. Melucci, S. Fedi, M. Locatelli, C. Locatelli, S. Montalbani and M. Cappelletti, Curr. Drug Targets, 2013, 14, 1023–1033 CrossRef CAS PubMed.
- R. Goodacre, E. M. Timmins, R. Burton, N. Kaderbhai, A. M. Woodward, D. B. Kell and P. J. Rooney, Microbiology, 1998, 144, 1157–1170 CrossRef CAS PubMed.
- X. Li, P. T. Lv, L. Wang, A. L. Guo, M. H. Ma and X. B. Qi, J. Chromatogr. B: Anal. Technol. Biomed. Life Sci., 2014, 971, 107–111 CrossRef CAS PubMed.
- D. J. M. Mouwen, M. Weijtens, R. Capita, C. Alonso-Calleja and M. Prieto, Appl. Environ. Microbiol., 2005, 71, 4318–4324 CrossRef CAS PubMed.
- D. J. M. Mouwen, R. Capita, C. Alonso-Calleja, J. Prieto-Gomez and M. Prieto, J. Microbiol. Methods, 2006, 67, 131–140 CrossRef CAS PubMed.
- Z. Cao, Z. J. Wang, Z. L. Shang and J. C. Zhao, PLoS One, 2017, 12, 172359–172373 Search PubMed.
- L. Mariey, J. P. Signolle, C. Amiel and J. Travert, Vib. Spectrosc., 2001, 26, 151–159 CrossRef CAS.
- E. C. Lopez-Diez and R. Goodacre, Anal. Chem., 2004, 76, 585–591 CrossRef CAS PubMed.
- R. M. Jarvis and R. Goodacre, FEMS Microbiol. Lett., 2004, 232, 127–132 CrossRef CAS PubMed.
- K. Gaus, P. Rosch, R. Petry, K. D. Peschke, O. Ronneberger, H. Burkhardt, K. Baumann and J. Popp, Biopolymers, 2006, 82, 286–290 CrossRef CAS PubMed.
- L. Z. Guo, C. S. Ye, L. Cui, K. Wan, S. Chen, S. H. Zhang and X. Yu, Environ. Int., 2019, 130, 104883–104893 CrossRef CAS PubMed.
- W. E. Huang, R. I. Griffiths, I. P. Thompson, M. J. Bailey and A. S. Whiteley, Anal. Chem., 2004, 76, 4452–4458 CrossRef CAS PubMed.
- R. Weiss, M. Palatinszky, M. Wagner, R. Niessner, M. Elsner, M. Seidel and N. P. Ivleva, Analyst, 2019, 144, 943–953 RSC.
- B. Lorenz, C. Wichmann, S. Stockel, P. Rosch and J. Popp, Trends Microbiol., 2017, 25, 413–424 CrossRef CAS PubMed.
- M. K. Song, L. F. Jiang, D. Y. Zhang, C. L. Luo, H. Yin, Y. T. Li and G. Zhang, J. Hazard. Mater., 2018, 353, 534–541 CrossRef CAS PubMed.
- Y. Z. Song, A. K. Kaster, J. Vollmers, Y. Q. Song, P. A. Davison, M. Frentrup, G. M. Preston, I. P. Thompson, J. C. Murrell, H. B. Yin, C. N. Hunter and W. E. Huang, Microb. Biotechnol., 2017, 10, 125–137 CrossRef CAS PubMed.
- X. N. Lu, Q. Huang, W. G. Miller, D. E. Aston, J. Xu, F. Xue, H. W. Zhang, B. A. Rasco, S. Wang and M. E. Konkel, J. Clin. Microbiol., 2012, 50, 2932–2946 CrossRef CAS PubMed.
- H. Kearns, R. Goodacre, L. E. Jamieson, D. Graham and K. Faulds, Anal. Chem., 2017, 89, 12666–12673 CrossRef CAS PubMed.
- M. Kahraman, M. M. Yazici, F. Sahin and M. Culha, J. Biomed. Opt., 2007, 12, 540151–540156 CrossRef PubMed.
- H. Fisk, C. Westley, N. J. Turner and R. Goodacre, J. Raman Spectrosc., 2016, 47, 59–66 CrossRef CAS PubMed.
- H. B. Zhou, D. T. Yang, N. P. Ivleva, N. E. Mircescu, S. Schubert, R. Niessner, A. Wieser and C. Haisch, Anal. Chem., 2015, 87, 6553–6561 CrossRef CAS PubMed.
- H. B. Zhou, D. T. Yang, N. P. Ivleva, N. E. Mircescu, R. Niessner and C. Haisch, Anal. Chem., 2014, 86, 1525–1533 CrossRef CAS PubMed.
- M. Chisanga, H. Muhamadali, D. I. Ellis and R. Goodacre, Appl. Sci., 2019, 9, 1163–1186 CrossRef.
- S. O. Mansoorabadi, C. J. Thibodeaux and H. W. Liu, J. Org. Chem., 2007, 72, 6329–6342 CrossRef CAS PubMed.
- C. Schaffer and P. Messner, FEMS Microbiol. Rev., 2017, 41, 49–91 CrossRef PubMed.
- G. Zhang, T. C. Meredith and D. Kahne, Curr. Opin. Microbiol., 2013, 16, 779–785 CrossRef CAS PubMed.
- A. M. Teixeira, A. Nemec and C. Sousa, Molecules, 2019, 24, 168–177 CrossRef PubMed.
- L. I. Llarrull, J. F. Fisher and S. Mobashery, Antimicrob. Agents Chemother., 2009, 53, 4051–4063 CrossRef CAS PubMed.
- V. Velusamy, K. Arshak, O. Korostynska, K. Oliwa and C. Adley, Biotechnol. Adv., 2010, 28, 232–254 CrossRef CAS PubMed.
- E. Nagy, S. Becker, M. Kostrzewa, N. Barta and E. Urban, J. Med. Microbiol., 2012, 61, 1393–1400 CrossRef CAS PubMed.
- A. Mellmann, J. Cloud, T. Maier, U. Keckevoet, I. Ramminger, P. Iwen, J. Dunn, G. Hall, D. Wilson, P. LaSala, M. Kostrzewa and D. Harmsen, J. Clin. Microbiol., 2008, 46, 1946–1954 CrossRef CAS PubMed.
- A. Kassim, V. Pfluger, Z. Premji, C. Daubenberger and G. Revathi, BMC Microbiol., 2017, 17, 128–135 CrossRef PubMed.
- M. R. Mashego, K. Rumbold, M. De Mey, E. Vandamme, W. Soetaert and J. J. Heijnen, Biotechnol. Lett., 2007, 29, 1–16 CrossRef CAS PubMed.
- A. V. Karlyshev, D. Linton, N. A. Gregson and B. W. Wren, Microbiology, 2002, 148, 473–480 CrossRef CAS PubMed.
Footnote |
† Electronic supplementary information (ESI) available. See DOI: 10.1039/c9an02026h |
|
This journal is © The Royal Society of Chemistry 2020 |
Click here to see how this site uses Cookies. View our privacy policy here.