DOI:
10.1039/C9AN01252D
(Critical Review)
Analyst, 2020,
145, 13-28
A systematic review of analytical methods for the detection and quantification of β-N-methylamino-L-alanine (BMAA)†
Received
4th July 2019
, Accepted 21st October 2019
First published on 19th November 2019
Abstract
Neurodegenerative diseases are influenced by environmental factors such as exposure to toxins including the cyanotoxin β-N-methylamino-L-alanine (BMAA) that can bioaccumulate in common food sources such as fish, mussels and crabs. Accurate and precise analytical methods are needed to detect and quantify BMAA to minimize human health risks. The objective of this review is to provide a comprehensive overview of the methods used for BMAA analysis from 2003 to 2019 and to evaluate the reported performance characteristics for each method to determine the consensus data for each analytical approach and different sample matrices. Detailed searches of the database Web of Science™ (WoS) were performed between August 21st, 2018 and April 5th, 2019. Eligible studies included analytical methods for the detection and quantification of BMAA in cyanobacteria and bioaccumulated BMAA in higher trophic levels, in phytoplankton and zooplankton and in human tissues and fluids. This systematic review has limitations in that only the English language literature is included and it did not include standard operating protocols nor any method validation data that have not been made public. We identified 148 eligible studies, of which a positive result for BMAA in one or more samples analyzed was reported in 84% (125 out of 148) of total studies, 57% of HILIC studies, 92% of RPLC studies and 71% of other studies. The largest discrepancy between different methods arose from the analysis of cyanobacteria samples, where BMAA was detected in 95% of RPLC studies but only in 25% of HILIC studies. Without sufficient published validation of each method's performance characteristics, it is difficult to establish each method as fit for purpose for each sample matrix. The importance of establishing methods as appropriate for their intended use is evidenced by the inconsistent reporting of BMAA across environmental samples, despite its prevalence in diverse ecosystems and food webs.
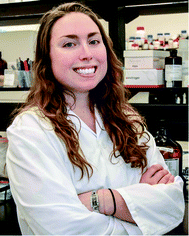 Stephanie L. Bishop | Stephanie Bishop is a PhD student in analytical chemistry at the University of British Columbia Okanagan. She studies the effects of different environments and ecosystems on cyanobacterial growth and metabolism, with a focus on lake systems in Canada and South America. Her research explores the relationships of non-protein amino acids including BMAA with other secondary metabolites and she uses validated analytical techniques to quantify the levels of non-protein amino acids in cyanobacteria and other organisms. |
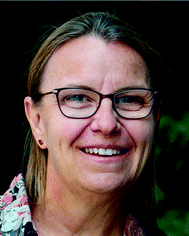 Susan J. Murch | Susan Murch investigates the chemicals produced by plants and how plant chemicals affect human health. Her current research uses metabolomics tools to understand the chemistry of foods including: (a) identification and quantification of the natural neurotoxic non-protein amino acid BMAA in food webs and aquatic ecosystems, (b) development of new foods and new food ingredients from the underutilized staple crop breadfruit and (c) metabolomics and targeted phytochemistry approaches to understand how plant chemistry responds to environmental cues and challenges. |
1 Rationale for a systematic review
1.1 Background
In the next decades, scientists will be challenged to find solutions to many public health issues including exposure to natural toxins associated with neurological and hepatotoxic diseases. Though the mechanisms of neurodegenerative diseases such as amyotrophic lateral sclerosis (ALS) and Alzheimer's disease (AD) are only partially understood, many factors including exposure to environmental toxins likely increase the risk of disease. Climate change and anthropogenic changes to aquatic environments such as nutrient enrichment lead to potentially harmful events such as cyanobacterial blooms.1 These blooms not only harm ecosystems and commercial industries but may impact human health, as cyanobacteria are known to produce a variety of toxic secondary metabolites.1,2 Currently, many research groups around the world are developing analytical methods to monitor toxin levels in ecosystems containing cyanobacteria and other toxin-producing microorganisms to help minimize the exposure risk and prevent disease.3,4 One such toxin, β-N-methylamino-L-alanine (BMAA), is a non-protein amino acid produced by diverse taxa of cyanobacteria and other microorganisms such as diatoms and dinoflagellates.5–7 Increased exposure to cyanobacterial biomass is correlated to the risk of developing ALS, demonstrating that accurate detection and quantification of BMAA in the environment may help prevent disease.8
Research on BMAA began after Marjorie Grant Whiting led ethnobotanical studies on the toxicity of cycad trees in Guam.9 Whiting had been studying the Chamorro people of Guam and hypothesized that their traditional diet may have been implicated in an epidemic of neurodegenerative disease known as amyotrophic lateral sclerosis/Parkinsonism dementia complex (ALS/PDC).10–12 Vega and Bell were able to isolate BMAA from cycad seeds and its dose-dependent modes of toxicity were demonstrated in animal models including rats and macaques.13–15 The Chamorro people, however, knew of the toxicity of cycads and washed the seeds thoroughly before consumption which lessened the likelihood that the ALS/PDC epidemic was correlated to BMAA exposure.16 Cox and Sacks (2002) hypothesized that BMAA could bioaccumulate in the Guam ecosystem including in traditional Chamorro foods and observed that the decreasing consumption of flying foxes on Guam after World War II was correlated to decreased rates of ALS/PDC.17,18 After a period of reduced interest in BMAA in the 1990s, the 2002 hypothesis stimulated an explosion of interest with more than 370 papers published and 9300 citations involving BMAA (Fig. 1; based on Web of Science™ search April 5, 2019). Several studies demonstrated multiple sources of BMAA in traditional Chamorro foods that exposed people to much higher rates than estimated previously.17,19,20 It was hypothesized that BMAA was produced by cyanobacteria and accumulated through the Guam ecosystem leading to the disease epidemic.19,21–23
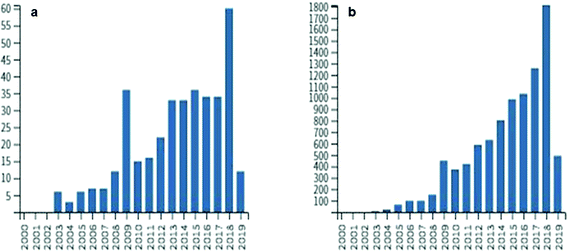 |
| Fig. 1 (a) Number of publications per year and (b) number of times cited per year from 2003–2019 based on the Web of Science search (April 5, 2019). Search terms included: BMAA, N-β-methylamino-L-alanine, β-N-methylamino-L-alanine; search results were filtered manually to remove unrelated entries. Total number of studies: 372. Total number of citations: 9304. | |
BMAA has been detected in ecosystems around the world in both cyanobacteria and other organisms and has bioaccumulated in common food sources including phytoplankton, fish, mussels, lobsters and blue crabs.5,6,24–27 This may have human health implications, as BMAA is known to cross the blood–brain barrier and has been found in the brain tissues of Chamorro as well as Canadian Alzheimer's patients.28,29 Upon the formation of a β-carbamate adduct in the presence of bicarbonate that is structurally similar to glutamate, BMAA acts as a glutamate receptor agonist and has been shown to bind to both metabotropic and ionotropic glutamate receptors.30,31 This may cause excitotoxic mechanisms to occur, leading to increased calcium influx and eventual motor neuron cell death. BMAA likely has several other modes of toxicity, as it has been found to incorporate into proteins in place of protein amino acids such as L-serine which can cause protein misfolding leading to detrimental processes in brain tissues.32,33 Vervets that consumed a chronic low dose of BMAA over 140 days developed the characteristic neurofibrillary tangles and β-amyloid plaques of Alzheimer's disease.34 β-Amyloid plaques were similarly found in the cerebral cortexes of deceased dolphins that contained high levels of BMAA.35 Other studies have shown that BMAA can interfere with the phosphorylation of tau proteins and can bind to neuromelanin in brain tissues indicating two potential mechanisms that could interfere with the brain's detoxification pathways.36,37
Understanding the potential risk of BMAA to human health requires validated, accurate and precise methods for the detection of BMAA in multiple sample matrices. The analysis of complex sample matrices such as human brain tissues, animal tissues, plant tissues, environmental samples and cyanobacteria is complicated by other naturally occurring amino acids, salts, sugars, proteins and a wide range of interfering compounds.
1.2 Analysis of BMAA
Since the onset of intensified BMAA research in the early 21st century, analytical chemists have struggled to find a suitable method for BMAA detection and quantification in food and environmental samples. The analysis of BMAA is complicated because it can exist (a) as a free amino acid,20 (b) in a protein-bound or associated form,19,38 (c) complexed with metal ions,39 or (d) as a salt, dimer or carbamate.39,40 Additionally, careful preparation of environmental samples is necessary as BMAA can adhere to glass and other surfaces and may be present in low concentration.41 BMAA is generally present in complex matrices which may interfere with analysis and contribute to Type II errors (false negatives). Analytical methods must adequately separate BMAA from its naturally occurring isomers. Two of these: N-(aminoethyl)glycine (AEG) and 2,4-diaminobutyric acid (2,4-DAB), are measured routinely by most researchers and one more: β-aminomethyl-L-alanine (BAMA), has recently been added to several methods42–46 (Fig. 2). Not all methods include all these compounds within their scope and as such may not have the required specificity (Fig. 3a). Additionally, several research groups have commented that not all analytical methods are capable of fully separating BMAA from one or more of its isomers and this must be taken into account during method design.6,25,47–49
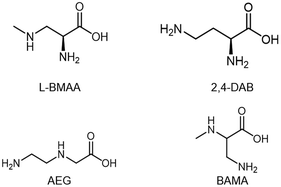 |
| Fig. 2 β-N-Methylamino-L-alanine (BMAA) and its isomers 2,4-diaminobutyric acid (2,4-DAB), N-2(amino)ethylglycine (AEG) and β-amino-N-methylalanine (BAMA). | |
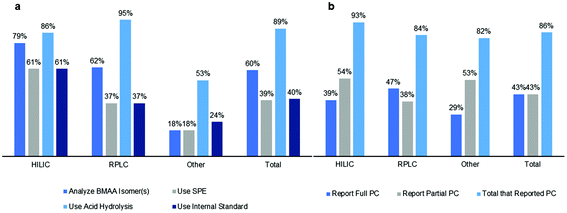 |
| Fig. 3 Overview of differences in (a) sample preparation/analysis and (b) reporting of performance characteristics (PC) between different methods. Bar graphs show percent of studies for each method. | |
The earliest methods of BMAA detection used modified standard techniques for amino acid analysis due to low sample concentration. Researchers used techniques such as gas chromatography coupled to mass spectrometry (GC-MS) and reverse-phase liquid chromatography (RPLC) using 9-fluorenylmethylchloroformate (FMOC) as a derivatizing agent.16,50 In 2003, Cox, Banack and Murch identified flying foxes (Pteropus mariannus) and cyanobacteria (Nostoc isolated from coralloid roots of Cycas micronesica Hill) as a source of BMAA using pre-column 6-aminoquinoyl-N-hydroxysuccinimidyl carbamate (AQC) derivatization and RPLC separation with fluorescence detection (FD).20,21 The presence of BMAA in these samples was confirmed orthogonally via LC-MS with atmospheric pressure ionization (API).20,28 Other common derivatizing agents used with liquid chromatography include propyl chloroformate (PCF) in the Phenomenex EZ:faast™ method with MS detection,51 ninhydrin (post-column derivatization) with fluorescence detection52 and dansyl chloride (DC) with MS/MS detection.53 Chromatographic techniques without derivatizing agents, such as hydrophilic interaction liquid chromatography (HILIC), have also been used for the analysis of BMAA.54 Alternative methods used include 1H nuclear magnetic resonance (NMR) spectroscopy,55 capillary electrophoresis56 and the commercially available enzyme linked immunosorbent assay (ELISA) kit,57 the results of which are often compared to orthogonal methods. There have been several studies directly comparing the results of chromatography methods utilizing different detection techniques, including MS/MS, FD and UV.52,58–60
Different sample preparation techniques have been shown to significantly influence the results. One study compared the results of 4 different labs using 4 different methods for analysis of samples of cycad flour prepared in Guam using the traditional Chamorro methods.19,61 The identical samples were analyzed by three methods that included fluorescence detection of FMOC50 or AQC derivatives,19 and GC-MS analysis of methyl ester derivatives,62 and reported BMAA values ranging from not detected (ND) to 146 μg g−1.19,63 A fourth study used LC-MS analysis and reported values in a similar range for samples that were either very similar or identical but not clearly identified.64 The variability in the results of analysis of the identical flours was attributed to the amount and duration of acid hydrolysis and the variability in the detection methods.19 As a result, Murch et al. (2004) hypothesized that BMAA could be present in tissues not only as a free amino acid, but it can also incorporate into or be associated with proteins19 and most subsequent studies use an acid hydrolysis step to release protein-associated BMAA (Fig. 3a).
Another common difference among recently published methods is the use of a solid phase extraction (SPE) cleanup step during sample preparation to eliminate interfering compounds from the sample matrix and concentrate the target analyte60 (Fig. 3a). Kubo et al. (2008) used a combination of a C18 cartridge (Sep-Pak C18), hydrophobic polymer cartridge (Oasis HLB) and cation-exchange resin (Oasis MCX) from Waters to concentrate BMAA in cyanobacteria matrices before analysis via HILIC.65 RPLC methods have also used Sep-Pak C18 as well as Isolute HCX-3 SPE cartridges for sample cleanup and concentration.66,67 Additionally, several RPLC studies use the Phenomenex EZ:faast™ physiological free amino acid kit which includes an SPE sorbent tip.26 However, some groups have chosen to eliminate this step as it may also result in reduced recoveries of BMAA and its isomers. Esterhuizen-Londt and Downing (2011) found that the use of SPE Giga™ tubes resulted in recoveries as low as 47% for BMAA in pond water, while Yan et al. (2017) found that the methods using SPE cleanup must be carefully optimized for adequate recovery and demonstrated that the use of tandem Oasis HLB-C18 and Oasis-MCX cartridges provided the best recoveries of BMAA in natural water sources.68,69 Differences between analytical methods are also seen in the use of external or internal standards for quantitation (Fig. 3a). Isotopically labelled internal standards for BMAA were not commercially available until about 2010 and some research teams have used custom synthesis to create deuterated BMAA.16 Availability has since become more widespread and labeled internal standards commonly used include D3-BMAA, 13C,15N2-BMAA and 13C3,15N2-BMAA.54,70 An international consensus of agreement for a standardized method has not been achieved and controversies exist in the literature arising from variable results from different methods. A single laboratory validation of AQC derivatization has been previously published71 and provides the foundation for method comparisons.
1.3 Validation of analytical methods for BMAA analysis
The process of method validation allows researchers to confirm that the analytical procedure employed for a specific use is suitable for that intended use – fit for purpose. These protocols include an initial assessment of performance characteristics along with continual re-assessment of method validity and quality control during routine analysis. The results from method validation can be used to determine the quality, reliability and consistency of analytical results and are necessary to ensure that the method is fit for purpose for an intended application. There are several generally accepted methodologies to evaluate and establish these performance characteristics, with different levels of validation ranging from a single laboratory validation, peer verification and a collaborative study. Some of the organizations involved in method validation include the International Union of Pure and Applied Chemistry (IUPAC), United States Pharmacopeia/National Formulary (USP/NF), the International Council for Harmonization (ICH), the U.S. Food and Drug Administration (FDA) and the Association of Official Analytical Chemists (AOAC™). Analytical method development for BMAA analysis should follow guidelines set forth by these agencies and include performance characteristics such as selectivity, linearity, accuracy, precision and sensitivity in order to show that the method is fit for purpose and achieves meaningful results for a specific matrix.
Though researchers have used and evaluated a great number of analytical methods, to date only the UPLC-MS/MS method using AQC derivatization71 has been validated according to the AOAC™ Guidelines for Single Laboratory Validation.72 Many other publications report some performance characteristics and validation data for detection and quantification of BMAA but are incomplete (Fig. 3b). Furthermore, many of these methods have not evaluated diverse sample matrices or provide validation data for a different matrix than the samples studied. This systematic review seeks to critically evaluate the literature from 2003 to 2019 to compare analytical methods used to detect and quantify BMAA in environmental or in vitro samples.
1.4 Goals and objectives
The overall objective of this study is to provide an overview of the different analytical methods used for BMAA analysis as well as evaluate the reported performance characteristics for each method to date. This will provide a basis of comparison for the current state of BMAA research and may provide insight into trends in BMAA data for each analytical method type and sample matrix. With better agreement and consensus between different laboratories using different methods, researchers will be able to accurately quantify BMAA in a wide range of samples and therefore provide important data that may be used by the public and health authorities to minimize the risk of neurotoxin exposure.
2 Methods and design
A systematic review of the literature was designed using the Preferred Reporting Items for Systematic Reviews and Meta-Analyses (PRISMA) criteria.73 This review is registered in the International Prospective Register of Systematic Reviews (PROSPERO) database, hosted by the National Institute for Health Research (NIHR), with ID CRD42019138300.
2.1 Information sources
Detailed searches of the database Web of Science™ (WoS) were performed between August 21th, 2018 and April 5th, 2019 (Fig. 4). The searches were conducted in English with the following terms: BMAA; N-β-methylamino-L-alanine; β-N-methylamino-L-alanine; β-methylamino-L-alanine. Additional materials containing the same search terms but not cited in WoS were collected from Google Scholar, ResearchGate and the senior author's collection of BMAA reprints collected from corresponding authors over the last 15 years.
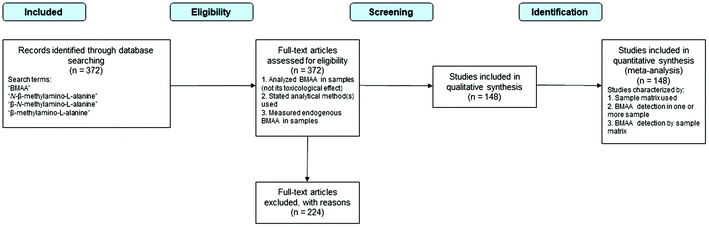 |
| Fig. 4 Schematic diagram of systematic method for literature review. Adapted from Moher et al. 2009.73 | |
2.2 Study selection and eligibility criteria
Of the 372 total studies analyzed (Fig. 1a), we selected the 148 studies that included analytical methods for detection and quantification of BMAA in cyanobacteria (from natural habitats or grown in vitro), BMAA that bioaccumulated in higher trophic levels (including typical Chamorro dietary items, seafood and mammals), in phytoplankton and zooplankton (including diatoms and dinoflagellates) and in human tissues and fluids (including brain tissues and urine). Studies that quantified exogenously applied BMAA in organisms, studies not appearing within the search terms or studies published in a language other than English were excluded. Studies that included multiple sample matrices or more than one analytical method were counted multiple times accordingly. Details of the literature selection process are shown in Fig. 4.
2.3 Data collection process
Data were collected from the Materials and methods and Results sections from each of the studies and collated in an Excel spreadsheet for analysis (ESI Tables†).
2.4 Risk of bias
To mitigate any risk of bias according to the authors’ previous publications, summary data of all publications are presented (ESI Tables†). Published data from research groups from 17 countries on 6 continents are included in the analysis.
2.5 Synthesis of results
Data were sorted into subcategories by methods and analyzed by method validation parameters. Data were analyzed by subgroups according to the chromatography and derivatization methods.
3 Synthesis of results
3.1 Methods used to detect and quantify BMAA
The majority of methods used for BMAA analysis employed chromatography (either gas or liquid) while a small percentage used other methods including capillary electrophoresis (CE) and ELISA kits among others (ESI Tables†).106–163 Of the 148 total studies analyzed, 70% used RPLC, 19% used HILIC and 11% used other methods (Fig. 5). The majority of RPLC studies used derivatives which included AQC, PCF, DC and others (Table S1;†Fig. 6c) with about 80% of the studies using AQC. For the HILIC studies, over 90% of the studies used a Tosoh Bioscience TSKgel amide-80 column or a SeQuant ZIC-HILIC column (Table S1;†Fig. 6b). Though the earlier studies used fluorescence detection, most of the studies since 2008 used mass spectrometry (MS) or tandem mass spectrometry (MS/MS) as the detection method (ESI Tables†).
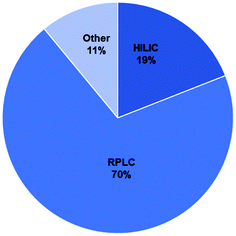 |
| Fig. 5 Overview of methods used for BMAA analysis by percent of studies using each method. | |
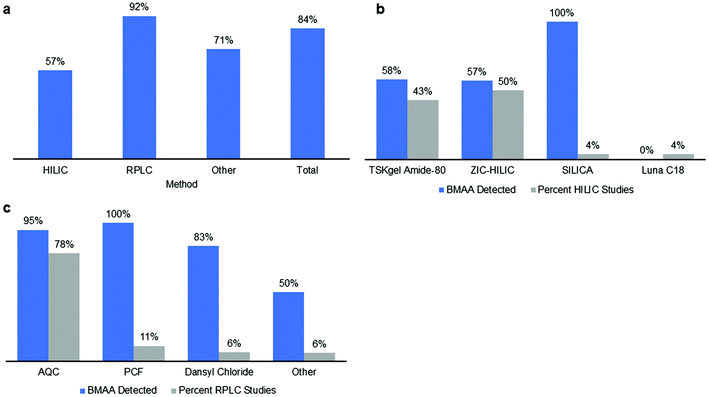 |
| Fig. 6 (a) Percent of studies that reported a positive result for BMAA detection in one or more samples using the method. (b) Percent of HILIC studies that reported a positive result for BMAA in one or more samples by column used (blue bar) and percent of HILIC studies by column used (grey bar). (c) Percent of RPLC that reported a positive result for BMAA in one or more samples by column used (blue bar) and percent of RPLC studies by column used (grey bar). | |
3.2 Sample matrices analyzed
Though many researchers have analyzed similar sample materials to those in the pioneering study in 2003 that analyzed cyanobacteria (Nostoc), cycad tissues, flying fox tissues and Chamorro brain tissues,20 several other sources of BMAA have been identified or examined. A global collaborative study in 2005 by Cox et al. determined that about 95% of free-living cyanobacteria genera tested produced BMAA as well as most symbionts.22 This study demonstrated that BMAA may be present in many ecosystems around the world including saline and freshwater and soil crusts, so many studies have examined cyanobacteria growing in natural habitats or in culture under different growth conditions (ESI Tables†). With the rise of cyanobacterial blooms due to nutrient overloading and increased global temperatures,1 many studies have also focused on quantifying BMAA in cyanobacterial blooms that could implicate human health (ESI Tables†). Around 2010, several publications examined various trophic levels in ecosystems in Florida and Sweden and identified zooplankton, fish, mussels, shrimp, blue crabs and oysters as sources of BMAA27,66,74,75 and in 2014, diatoms and shark tissues (cartilage) were also determined to contain BMAA.24,76 Brain tissues from deceased dolphins exposed to cyanobacterial blooms also contained BMAA.35 Apart from the superior frontal gyrus of Chamorro and AD patients in 2004, other studies have examined different brain tissues including the middle frontal gyrus, frontal cortex, temporal cortex, and cerebellum.28,29,77 Other human tissues or fluids examined include blood, cerebral spinal fluid (CSF), urine and human hair.78–81 This study demonstrates that BMAA may be produced by or present in organisms in various trophic levels of ecosystems around the world, indicating that methods used for BMAA analysis must be fit for purpose and designed to minimize losses of BMAA in complex matrices during sample preparation.
3.3 Sample preparation in studies
Sample preparation for BMAA analysis varied between studies, and has evolved since 2003 to include analysis of several isomers of BMAA including AEG, 2,4-DAB and BAMA43,46,82,83 (Fig. 2). Since about 2010, most studies included at least 2 of these isomers in their analysis but many of the earliest studies using RPLC with AQC derivatization only reported concentrations of BMAA. Overall, 79% of HILIC studies, 62% of RPLC studies and 60% of other studies analyzed isomer(s) of BMAA (Fig. 3a). The use of an SPE cleanup step during sample preparation was more common with HILIC methods (79%) than RPLC (37%) or other methods (18%) (Fig. 3a). Although most studies analyzed sample matrices for free, protein-bound or associated BMAA and/or total BMAA, some studies differed in their amino acid extraction techniques. It is generally accepted in the literature that in order to release protein bound or associated BMAA from a sample matrix, an acid hydrolysis step is required; however, some studies did not report this. An acid hydrolysis step for determination of the protein bound or associated and/or total BMAA was reported in 86% of HILIC studies, 95% of RPLC studies and 89% of other studies (Fig. 3a). Lastly, several studies differed in the use of an internal standard for BMAA analysis, which was reported in 61% of HILIC studies, 37% of RPLC studies and 24% of other studies (Fig. 3a).
3.4 Reported performance characteristics in studies
Method validation ensures that each method used for BMAA analysis is fit for purpose for a specific matrix. We evaluated whether each method analyzed reported the following performance characteristics: linearity (coefficient of determination or R2 value of standard curve), selectivity (the method separated BMAA from one or more of its isomers), accuracy (analyte % recovery), precision (intra- and/or inter-day %RSD values) and sensitivity (limit of detection (LOD) and/or limit of quantification (LOQ)). These performance characteristics demonstrate whether there are analyte losses during sample preparation and whether the results are valid and reproducible. Additionally, the reporting of LOD and LOQ values for a method indicates that the method is suitable for a certain concentration range of BMAA in a given matrix and the reporting of selectivity indicates whether interference from isobaric compounds may complicate analysis.
For HILIC methods, 39% of studies reported or referenced a validated method for all performance characteristics described above while 54% of studies reported only partial performance characteristics (Fig. 3b). Studies that used a ZIC-HILIC column were fully validated in 50% of studies, compared to only 25% of HILIC studies that used an amide column. 47% of RPLC studies reported full performance characteristics while 38% reported partial performance characteristics, with 48% of RPLC studies using AQC derivatization fully validated compared to 27% of studies with PCF derivatization or 58% of studies using other derivatizing agents (Fig. 3b). Fewer other method types were fully validated, with 29% of other studies reporting full performance characteristics and 53% reporting partial performance characteristics (Fig. 3b). Studies using ELISA kits were fully validated 43% of the time, compared to 33% of studies employing capillary electrophoresis. Overall, 43% of studies reported full performance characteristics while 43% reported partial performance characteristics with a total of 86% of studies reporting performance characteristics (Fig. 3b). One of the common performance characteristics that groups failed to report was linearity, which is defined as adequate by the AOAC™ when a calibration curve for the method achieved a coefficient of determination (R2 value) of 0.99 or greater.72 Only 64% of HILIC studies, 48% of RPLC studies and 29% of other studies reported R2 values of 0.99 or greater, with the remaining studies failing this criterion or not reporting linearity at all.
Representative values for performance characteristics for each method are shown in Table 1. Although most methods reported values that are considered generally acceptable by method validation organizations, some methods did not meet these criteria for a certain matrix. For example, the HILIC method using a ZIC-HILIC column reported a recovery of 7–78% for total BMAA and 21–103% recovery for free BMAA in a cyanobacteria matrix, the lower limits of which are unacceptably low (Table 1). Also, sensitivities between methods differed, with HILIC methods reporting LOD values between 0.08–2 ng mL−1, RPLC methods reporting LOD values between 0.05–5 ng mL−1 and other methods reporting LOD values between 0.8–5 ng mL−1 (Table 1). The differences between performance characteristic values demonstrate the need for each research group to carefully evaluate their method for a given matrix in order to produce valid and accurate results.
Table 1 Representative method validation performance characteristics reported for each method type. Performance characteristics analyzed included: linearity (coefficient of determination (R2 value)), selectivity (full separation of BMAA from its isomers and similar compounds), accuracy (% recovery of BMAA), precision (intra- and inter-day %RSD) and sensitivity (limit of detection (LOD) and limit of quantification (LOQ))
Method type |
Column/separation type |
Derivative |
Sample matrix |
Isomers fully separated |
Linearity (R2 value) |
BMAA recovery |
Intra-day precision (%RSD) |
Inter-day precision (%RSD) |
LOD |
LOQ |
Ref. |
Not reported.
|
HILIC |
ZIC-HILIC |
None |
Animal (seafood) |
BMAA, AEG, 2,4-DAB |
0.995 |
93–101% (free) |
2.5–7.8% (free) |
NRa |
34 fmol (2 ng mL−1) |
84 fmol (5 ng mL−1) |
94
|
64–78% (total soluble) |
1–11.4% (total soluble) |
86–110% (total) |
2.1–8% (total) |
Brain |
BMAA, AEG, 2,4-DAB |
0.995 |
77–80% (free) |
6–11.1% (free) |
NR |
34 fmol (2 ng mL−1) |
84 fmol (5 ng mL−1) |
94
|
82–84% (total) |
9–15.7% (total) |
Cyanobacteria |
BMAA, AEG, 2,4-DAB |
0.995 |
21–103% (free) |
7.4–61% (free) |
NR |
34 fmol (2 ng mL−1) |
84 fmol (5 ng mL−1) |
94
|
7–78% (total) |
2.3–41.5% (total) |
Waters Acquity BEH amide |
None |
Blue mussel |
BMAA, AEG, 2,4-DAB |
NR |
99–105% |
1.7–8.2% |
1.3–6.2% |
0.025 μg g−1 (ww); (0.05 ng mL−1) |
NR |
105
|
TSKgel amide-80 |
None |
Mussel, cycad, cyanobacteria |
BMAA, AEG, 2,4-DAB, 3,4-DAB, BAMA |
0.99991 |
NR |
NR |
NR |
20 ng g−1 (dw); (0.4 ng mL−1) |
NR |
45
|
RPLC |
Waters Acquity C18 BEH |
AQC |
Cyanobacteria |
BMAA, AEG, 2,4-DAB |
0.999 |
105–108% |
3–6% |
3–6% |
0.187 ng mL−1 |
0.746 ng mL−1 |
71
|
Phenomenex AAA-MS 250 |
PCF |
Cyanobacteria |
BMAA, 2,4-DAB |
0.989 |
87.9–98.9% |
0.6–2.8% |
NR |
0.042 pmol per injection; (5 ng mL−1) |
0.82 pmol per injection; (97 ng mL−1) |
51
|
Waters Acquity C18 BEH |
Dansyl chloride |
Mussel |
BMAA, AEG, 2,4-DAB |
0.99 |
83% |
2.2–12% |
6.8–15% |
16 ng g−1 (ww); (0.05 ng mL−1) |
0.15 μg g−1 (ww); (0.4 ng mL−1) |
53
|
Other |
ELISA kit |
None |
Fish, contaminated water basin |
Only BMAA |
NR |
70–83% |
<6% |
NR |
1.25 μg g−1 (dw); (5 ng mL−1) |
NR |
97
|
Capillary electrophoresis |
None |
Mussel, cycad, lobster, cyanobacteria |
BMAA, AEG, 2,4-DAB, 3,4-DAB, BAMA |
0.9994 |
NR |
NR |
NR |
16 ng g−1 (dw); (0.8 ng mL−1) |
NR |
102
|
3.5 BMAA detection in different sample matrices
BMAA was detected in 84% (125 out of 148) of studies included in this literature review (in one or more sample matrix and/or specimen though not necessarily in all samples examined) (Fig. 6a). When examined according to the method type, BMAA was detected in 57% of HILIC studies, 92% of RPLC studies and 71% of other studies (CE, ELISA, etc.) (Fig. 6a). In order to determine what percent of studies detected BMAA according to the sample matrix, the samples were categorized by either: (1) cyanobacteria (from natural habitats, grown in vitro or dietary supplements); (2) bioaccumulated sources (including Chamorro dietary items, seafoods and mammals); (3) phytoplankton or zooplankton (including diatoms and dinoflagellates); and (4) human tissues and fluids (including brain, hair, blood, CSF and urine) (Fig. 7). Studies that included more than one sample type were counted multiple times accordingly. When HILIC chromatography was used, BMAA was detected in: 25% of studies including cyanobacteria, 83% of studies including bioaccumulated sources, 100% of studies including phyto- or zooplankton and 25% of studies including human tissues/fluids (Fig. 7). With RPLC chromatography, BMAA was detected in: 95% of studies including cyanobacteria, 96% of studies including bioaccumulated sources, 100% of studies including phyto- or zooplankton and 57% of studies including human tissues/fluids (Fig. 7). Other types of studies detected BMAA in: 88% of studies including cyanobacteria, 67% of studies including bioaccumulated sources and 33% of studies including human tissues/fluids (no studies examined BMAA in phyto- or zooplankton) (Fig. 6). In total, BMAA was detected in: 78% of studies including cyanobacteria, 93% of studies including bioaccumulated sources, 100% of studies including phyto- or zooplankton and 48% of studies including human tissues/fluids (Fig. 7).
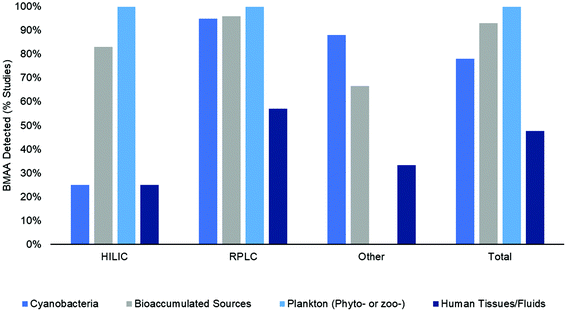 |
| Fig. 7 Percent of studies that reported a positive result for BMAA detection by sample type and method type. Cyanobacteria (medium blue bars) are from natural habitats, grown in vitro or dietary supplements. Bioaccumulated BMAA sources (grey bars) include human food sources and higher trophic level organisms. Plankton (light blue bars) include phytoplankton (diatoms and dinoflagellates) or zooplankton. Human tissues and fluids (dark blue bars) include brain (superior frontal gyrus, middle frontal gyrus, frontal cortex, temporal cortex or cerebellum), hair, blood, CSF and urine. | |
4 Discussion
4.1 Summary of evidence
The results of this systematic review show that BMAA has been detected in many sample matrices from diverse environments using a variety of analytical methods (Fig. 7). Therefore, monitoring of this toxin in environmental samples is warranted to ensure public safety during cyanobacterial blooms or other adverse events. While analytical results are similar between HILIC, RPLC and other methods for matrices from bioaccumulated sources, plankton and human tissues/fluids, there was a large discrepancy in results for cyanobacteria matrices in HILIC and RPLC studies. BMAA was detected in cyanobacteria in 95% of RPLC studies and only 25% of HILIC studies (Fig. 7). Additionally, there were several differences in sample preparation between HILIC and RPLC methods, where HILIC studies tended to use an SPE cleanup step (in 61% of studies) and an internal standard (in 61% of studies) more frequently than RPLC studies (37% and 37% respectively) (Fig. 3a). Only 43% of total studies performed full method validation, with 39% of HILIC studies, 47% of RPLC studies and 29% of other studies reporting full performance characteristics (Fig. 3b). This indicates that different methods may produce different results on similar samples, especially if a method is not fully validated for a specific matrix and sample preparation protocol.
4.2 Controversies in the literature
The results of this systematic literature review demonstrate that BMAA was found in one or more of the samples tested in the majority of publications from 2003–2019. While one may argue that this is derived from the well-known and documented “positive publication bias” which results in more papers with positive findings than negative results published,84 this seems unlikely as several of the publications reported negative results, often directly criticizing previous studies.48,54,57,60,77,85 Therefore, it appears that the analytical method used has a great influence on the results of the study. The first papers to use HILIC chromatography were published in 2008 and both failed to detect BMAA in cyanobacteria samples.54,65 This and other contradictory results in the literature have led to a series of controversial publications, some of which claimed many of the initial studies using a fluorescent tag were not selective or properly validated and questioned whether BMAA was actually present worldwide.60,86,87 Methods using HILIC generally fail to detect BMAA more often than RPLC techniques (Fig. 6a). However, the pervading idea in the literature that few HILIC studies have detected BMAA in samples seems unfounded considering that over half the studies examined reported a positive result for one or more samples tested (Fig. 6 & 7). This may be due to improved sample preparation procedures and separation/detection techniques in the past few years and an increased number of sample matrices analyzed. Furthermore, it appears that the main discrepancy in results stems from the analysis of BMAA in cyanobacteria and human tissues/fluids while the results for phyto- and zooplankton and seafoods seem to agree regardless of the method used. Interestingly, while 83–100% of HILIC studies reported a positive detection of BMAA in bioaccumulated sources and phyto- or zooplankton, only 25% reported a positive result for cyanobacteria or human tissues/fluids (Fig. 7). While RPLC studies only reported a moderate number of positive results for human tissues/fluids (57%), almost all studies (95%) detected BMAA in one more cyanobacterial sample (Fig. 7). Some of the possible explanations for the discrepancies in results in the cyanobacterial matrix include biological variability in samples, differences in sample preparation and instrumentation, and inherent matrix effects in cyanobacterial samples. Many of the other sample matrices analyzed in this review lack the large-scale variability in species and environment displayed by ubiquitous cyanobacteria, lessening analytical issues such as differing matrix effects and biological variability. However, in order to confidently move forward in BMAA research, we must determine the source(s) of large discrepancies in analytical results and ensure that all techniques can detect BMAA accurately regardless of the sample matrix.
4.3 Differences in sample matrices and sample preparation
One reasonable explanation for the differences in analytical results between different cyanobacteria studies is simply due to natural biological variability. Cyanobacteria are known in virtually every ecosystem on Earth, including extreme ones where they adapt accordingly to survive.88 Therefore, environmental factors including nutrient and light availability, microbial community structure and seasonal fluctuations may play a role in the amount of BMAA produced by cyanobacteria at a given time. For example, nutrient availability is thought to be an important factor in cyanobacterial metabolism and researchers have demonstrated that cyanobacteria (Microcystis and Synechocystis) may produce more BMAA under nitrogen limiting conditions, although this may not be universal to all strains of cyanobacteria.89,90 The microbial community structure and nutrient sources in a complex microbial ecosystem may also affect amino acid metabolism in cyanobacteria.91 Studies in several ecosystems have demonstrated that the BMAA levels within a bloom vary seasonally and with bloom intensity and stages of decline.44,92,93 Thus, high levels of metabolic variability even within a single bloom will necessarily lead to discrepancies in levels of BMAA reported in different strains of cyanobacteria and under different environmental conditions.
Perhaps the most important aspect of an analytical procedure is the sample preparation and a lack of reproducibility in results can often be explained by ineffective sample preparation. In 2004, Murch et al. determined that acid hydrolysis of protein-bound amino acids in samples could result in up to a 240-fold increase in BMAA compared to the analysis of free amino acids with trichloroacetic acid (TCA) extraction.19 This demonstrated that BMAA could be bound or associated with a protein in tissues and provided an explanation for the lack of BMAA found in cycad seeds using earlier methods. Researchers now routinely use acid hydrolysis or a combination of acid hydrolysis and TCA precipitation to quantify the total BMAA or fractions of free and bound/associated BMAA94 (Fig. 3a). Differences in the acid extraction of BMAA in biological tissues and failure to specify which fraction was used could result in discrepancies in analytical results. Furthermore, some studies report the use of a solid-phase extraction (SPE) cleanup step during sample preparation to reduce matrix interferences during analysis but this may result in poor recovery69,95 (Fig. 3a). Yan et al. found that adequate recoveries of BMAA in natural water could be achieved but only with the use of tandem C18 and MCX cartridges under optimized conditions.69 More of the HILIC studies used SPE and some of the HILIC methods report very low recovery of BMAA (<10%) in cyanobacterial matrices even without the use of SPE94 (Table 1). Poorly optimized SPE procedures could therefore result in analyte loss and underestimation of BMAA levels in samples.
The use of an internal standard can also increase accuracy and indicate analyte losses during sample preparation. More HILIC methods (61% of studies) used an internal standard for analysis than RPLC studies (37% of studies) or other types of studies (24%) (Fig. 3a). Unfortunately, the prohibitive cost and lack of availability of isotopically labelled BMAA standards limited their use in earlier studies. With more commercially available stable BMAA internal standards currently available, more researchers will be able to use this technique for quantitation. However, the use of internal standards does not always account for analyte loss during sample preparation. Although authors used a D3-BMAA internal standard in a comparison study of 3 sample matrices (cyanobacteria, animal, brain) using a HILIC method, they were unable to explain why D3-BMAA spiking of the cyanobacterial strain Leptolyngbya resulted in very low recoveries of 7–21% while D3-BMAA spiking with the zooplankton Daphnia magna resulted in very high recoveries of 141%.94 These authors also analyzed the same sample matrices after SPE cleanup and derivatization with AQC and achieved recoveries of less than 10% for all matrices. Another validated RPLC method with AQC derivatization demonstrated an adequate recovery (between 104.8–107.5%) of BMAA in a cyanobacterial matrix (spirulina) at 3 different analyte spike concentrations (0.122–0.183 ng mL−1).71 Curiously, while these spiking levels were close to the LOQ for the method (0.746 ng mL−1) and the highest spiking level was nearly at the LOD (0.187 ng mL−1), the spiking level for the cyanobacterial matrix in the HILIC method (40 ng mL−1) was much higher than the reported LOQ (5 ng mL−1).71,94,96 This indicates that while SPE cleanup steps may contribute to poor recovery, differences in sample preparation and instrumentation also contribute to the loss of target analyte and cannot be fully accounted for by use of an internal standard. Methods must minimize losses during sample preparation and determine that the recovery is in an acceptable range at different spiking levels, especially close to the LOQ, before they can be considered fit for purpose.
Additionally, while most studies thoroughly reported sample preparation procedures, many studies could have benefited from clearer and more thorough reporting of matrix effects and/or ruggedness trials for a given sample matrix. Only 24% of total studies reported results from testing of matrix effects and/or ruggedness trials with very few reporting full ruggedness trial results.97 A Youden Ruggedness Trial examines the effects of 7 factors in a single experiment and determines the major factors contributing to the variability in a method.72,98 Several studies compared specific sample preparation steps, including sample solvents and the aforementioned SPE cartridges, but future studies should attempt to perform a Youden Ruggedness Trial or determine matrix effects for all new methods developed and new sample matrices analyzed.72
4.4 Method instrumentation and validation
BMAA analytical methods require high selectivity and sensitivity due to the small, polar nature of BMAA and its similarity in size and shape to other naturally occurring molecules including protein amino acids. While traditional amino acid analysis techniques such as LC coupled to a fluorescence detector (FD) have been criticized due to their lack of specificity, comparison studies have demonstrated that FD and MS or MS/MS detectors provide similar results with the same samples.35,52,58,59,76 However, tandem mass spectrometry (MS/MS) is the most common and preferred detector as it provides the greatest selectivity due to the use of one or more selected precursors to product ion transitions. Nevertheless, MS is not without its faults and ionization efficiency and matrix effects may lead to inaccurate results.41 BMAA can form dimers and adducts with common metals such as copper and zinc; it is likely that less than 10% of BMAA can be detected in the native form (m/z 119) using electrospray ionization (ESI).39,99 Derivatization may prevent the spontaneous formation of adducts because the amine groups of the molecule are protected and the m/z of the ion is increased, which results in greater accuracy and selectivity.41 Some authors, however, argue that derivatization may overestimate the concentration of the target analyte especially with optical detectors such as FD due to a lack of derivatization specificity.54,60,87 While environmental sample matrices are complex with many undesired compounds that can become derivatized during sample preparation, careful and optimized procedures limit losses in derivatization efficiency and are measured by monitoring singly and doubly derivatized lysine or with the use of an internal standard.41 Furthermore, the type of derivative did not seem to have a large effect on the results of RPLC studies; methods with AQC, PCF and DC derivatization all reported a positive result in over 80% of studies (Fig. 6c). Very few RPLC studies (6%) used other derivatives such as FMOC, FLEC or no derivatives. While only 50% of these studies reported a positive result for BMAA, only two of these were published within the last 5 years making direct comparison difficult79,100 (Fig. 6c).
Although the use of several characteristic ion transitions in MS/MS improves method accuracy, the choice of ion transition used may provide different results.26 HILIC-MS/MS methods suffer from poor chromatographic separation between BMAA and its isomer BAMA using the m/z transition 119 > 102 which could lead to an inconsistent result.25,47 Many groups have reported incomplete separation of BMAA from its isomer BAMA and since analysis and quantification of BAMA has only started being performed since ca. 2012, earlier studies may have failed to obtain adequate selectivity of BMAA over its isomers.6,45–49,70 Additional ion separation steps, such as differential mobility spectrometry (DMS), which acts as an ion filter by separating ions in a matrix based on their mobility in high or low electrical field strength, may be required to adequately separate BMAA from BAMA.25,45 Discrepancies in results may also arise from different linear analysis ranges and limits of detection (LOD) between methods, especially as BMAA is generally present in low concentration in environmental samples and may be at or slightly below the LOD, producing a negative result. While both HILIC and RPLC methods generally reported a linear analysis range for standards in the ng mL−1 range, some earlier HILIC studies reported linear ranges from 0.01–1 μg mL−1 while the linear ranges for several RPLC studies were as low as 0.8–100 ng mL−1.7,54,71,94,101 HILIC methods generally report a higher LOD than RPLC methods. A comparison study of three common BMAA analytical methods including HILIC (TSKgel amide-80 column), RPLC with AQC derivatization and RPLC with PCF derivatization reported a LOD 150 times higher for HILIC compared to the RPLC methods26 and another comparison study reported a higher LOD for the HILIC method compared to RPLC.69 The higher sensitivity of derivatized methods is generally attributed to the increased molecular weight of BMAA facilitating identification of the precursor and product ions by MS/MS and higher robustness of RPLC columns which do not require careful re-equilibration regimes for sensitive analysis.69,94 We found that certain HILIC columns tend to have a short lifetime which can result in a loss of signal of up to 100% over a 6-week period, resulting in extremely low recoveries and inaccurate and imprecise results (unpublished data). This may implicate studies involving cyanobacteria, since cyanobacteria may contain low levels of BMAA that are unquantifiable by less sensitive methods.
The use of suitable orthogonal, non-chromatographic methods to analyze BMAA can also help to clarify some of the discrepancies in the literature. Researchers have reported positive results for BMAA in cyanobacteria and seafood tissues using CE56,102,103 (Fig. 6). The use of CE, however, may require coupling to MS to achieve a low enough LOD for low concentration BMAA samples.102,103 Most of the methods reported thus far use lengthy sample preparation procedures and expensive equipment that may require a skilled analyst. Environmental analysts would benefit from having a simpler and quicker method for routine BMAA analysis. Unfortunately, there have been several contradictory results between the BMAA ELISA kit, which is intended for this purpose. The BMAA ELISA utilizes a direct competitive assay format, where BMAA present in the sample competes with BMAA-horse radish peroxidase (HRP) analogues for binding sites on antibodies in solution which in turn bind to substrate-bound antibodies. Although several studies have successfully detected BMAA in water samples using an ELISA kit and reported adequate selectivity, repeatability and recovery, others have questioned the validity of the kit and reported that it is likely to give a false positive result.57,80,92,97,103,104 The BMAA ELISA guide provided by Abraxis indicates that the cross-reactivity for other amino acids ranges from 0–0.2% and reports that 2,4-DAB has very low cross reactivity at 0.01%. However, cross-reactivity with AEG and BAMA was not reported and the LOD is 4 ng mL−1, which is higher than those reported for some RPLC methods (0.001 ng mL−1).26 Additionally, none of the studies using an ELISA kit reported linearity values for the calibration curve, making comparisons between studies difficult. Nevertheless, the use of a method employing a biological element to selectively bind BMAA prior to detection may create a highly sensitive method capable of detecting BMAA in complex matrices and could be explored as a viable method for environmental BMAA analysis.
In general, one of the largest issues with BMAA results in the literature is a lack of thorough reporting of all performance characteristic data. Less than half of the studies (43%) reported full performance characteristic data or referenced a fully validated analytical method (Fig. 3b). More RPLC studies (47%) reported full performance characteristics than HILIC studies (39%) or other studies (24%). However, there were a great deal of studies for all method types that provided limited data that could indicate whether that method was fit for purpose for the specific sample matrix indicated. Future studies should seek to carefully validate the method and sample preparation steps for the intended application and use an internal standard to account for losses during preparation and analysis. Improvements in method validation and reporting for future studies will ensure that analytical results for BMAA analysis are accurate and valid for each sample matrix.
5 Limitations of the study
The data presented in this study could be limited by several factors. Only English language literature is included and methods published in other languages could contain valuable information that is not considered. Additionally, standard operating protocols of commercial analysis labs are frequently proprietary and unpublished. Such sources could not be included in our analysis. Finally, any method validation data that have not been made public could not be included in this work.
6 Conclusion and future prospects
Despite an exponentially growing interest in BMAA resulting in hundreds of publications since 2003, there remains a great deal of confusion and controversy in the literature. BMAA has been detected in global ecosystems by many methods, but analysis of certain sample matrices leads to a lack of consensus between results. To date, the only validated method accepted by the AOAC™ is a single laboratory validation (SLV) using UPLC-MS/MS with AQC derivatization for a cyanobacterial matrix.71 Further validation of this procedure between multiple laboratories can confirm that the method is reproducible and accurate, independent of the analyst. Other methods that report adequate selectivity, linearity, accuracy, precision and a reasonable LOD/LOQ in a specified matrix are also suitable for BMAA analysis. Certain methods may not be selective or sensitive enough for BMAA analysis in certain matrices and should be carefully validated before use. More studies should seek to determine where the discrepancies in analytical results between HILIC and RPLC methods lie, especially in cyanobacterial matrices where concentrations of BMAA may be very low. Additional method development using non-chromatographic techniques that utilize a BMAA-specific biological recognition element will help to confirm the accuracy of current methods and perhaps even allow for direct BMAA analysis in the field. By carefully analyzing and validating current published methods and protocols, researchers can discover where discrepancies in analytical results arise and thus improve ineffective methods. It is essential that all research groups can accurately quantify BMAA in a specific tissue type or matrix in order to ensure that health authorities and the public are provided with the correct information to minimize the risks of environmental BMAA exposure.
Funding
Funding support from the Natural Sciences and Engineering Council of Canada (NSERC) is gratefully acknowledged.
Conflicts of interest
The authors declare no competing interests.
References
- H. W. Paerl and T. G. Otten, Microb. Ecol., 2013, 65, 995–1010 CrossRef CAS PubMed.
- W. W. Carmichael and G. L. Boyer, Harmful Algae, 2016, 54, 194–212 CrossRef.
- M. Rutkowska, J. Piotka-Wasylka, T. Majchrzak, W. Wojnowski, H. Mazur-Marzec and J. Namiesnik, Trends Anal. Chem., 2019, 112, 112–122 CrossRef CAS.
- K. Manolidi, T. M. Triantis, T. Kaloudis and A. Hiskia, J. Hazard. Mater., 2019, 365, 346–365 CrossRef CAS PubMed.
- D. Réveillon, V. Séchet, P. Hess and Z. Amzil, Harmful Algae, 2016, 58, 45–50 CrossRef PubMed.
- D. Réveillon, E. Abadie, V. Séchet, E. Masseret, P. Hess and Z. Amzil, Mar. Environ. Res., 2015, 110, 8–18 CrossRef PubMed.
- S. Lage, A. Burian, U. Rasmussen, P. R. Costa, H. Annadotter, A. Godhe and S. Rydberg, Environ. Sci. Pollut. Res., 2016, 23, 338–350 CrossRef CAS PubMed.
- N. Torbick, B. Ziniti, E. Stommel, E. Linder, A. Andrew, T. Caller, J. Haney, W. Bradley, P. L. Henegan and X. Shi, Neurotoxic. Res., 2018, 33, 199–212 CrossRef PubMed.
- M. G. Whiting, Econ. Bot., 1963, 17, 270–302 CrossRef.
- A. Arnold, D. C. Edgren and V. S. Palladino, J. Nerv. Ment. Dis., 1953, 117, 135–139 CrossRef CAS PubMed.
-
G. L. Laqueur, O. Mickelsen, M. G. Whiting and L. T. Kurland, Carcinogenic properties of nuts from cycas circinalis L. indigenous to guam, 1963, vol. 31 Search PubMed.
- D. Reed, D. Labarthe, K. M. Chen and R. Stallones, Am. J. Epidemiol., 1987, 125, 92–100 CrossRef CAS PubMed.
- A. Vega and E. A. Bell, Phytochemistry, 1967, 6, 759–762 CrossRef CAS.
- A. Vega, E. a. Bell and P. B. Nunn, Phytochemistry, 1968, 7, 1885–1887 CrossRef CAS.
- P. S. Spencer, P. B. Nunn, J. Hugon, A. C. Ludolph, S. M. Ross, D. N. Roy and R. C. Robertson, Science, 1987, 237, 517–522 CrossRef CAS.
- M. Duncan, I. Kopin, R. Garruto, L. Lavine and S. Markey, Lancet, 1988, 332, 631–632 CrossRef.
- P. A. Cox and O. W. Sacks, Neurology, 2002, 58, 956–959 CrossRef PubMed.
- C. S. Monson, S. A. Banack and P. A. Cox, Conserv. Biol., 2003, 17, 678–686 CrossRef.
- S. J. Murch, P. A. Cox and S. A. Banack, Proc. Natl. Acad. Sci. U. S. A., 2004, 101, 12228–12231 CrossRef CAS.
- P. A. Cox, S. A. Banack and S. J. Murch, Proc. Natl. Acad. Sci. U. S. A., 2003, 100, 13380–13383 CrossRef CAS.
- S. A. Banack and P. A. Cox, Neurology, 2003, 61, 387–389 CrossRef CAS.
- P. A. Cox, S. A. Banack, S. J. Murch, U. Rasmussen, G. Tien, R. R. Bidigare, J. S. Metcalf, L. F. Morrison, G. A. Codd and B. Bergman, Proc. Natl. Acad. Sci. U. S. A., 2005, 102, 5074–5078 CrossRef CAS.
- S. A. Banack, S. J. Murch and P. A. Cox, J. Ethnopharmacol., 2006, 106, 97–104 CrossRef.
- L. Jiang, N. Kiselova, J. Rosén and L. L. Ilag, Sci. Rep., 2014, 4, 6931 CrossRef CAS.
- D. G. Beach, E. S. Kerrin, S. D. Giddings, M. A. Quilliam and P. McCarron, Sci. Rep., 2018, 8, 1–11 CrossRef CAS.
- T. C. Baker, F. J. M. Tymm and S. J. Murch, Neurotoxic. Res., 2018, 33, 43–54 CrossRef CAS.
- L. E. Brand, J. Pablo, A. Compton, N. Hammerschlag and D. C. Mash, Harmful Algae, 2010, 9, 620–635 CrossRef CAS.
- S. J. Murch, P. A. Cox, S. A. Banack, J. C. Steele and O. W. Sacks, Acta Neurol. Scand., 2004, 110, 267–269 CrossRef CAS.
- J. Pablo, S. A. Banack, P. A. Cox, T. E. Johnson, S. Papapetropoulos, W. G. Bradley, A. Buck and D. C. Mash, Acta Neurol. Scand., 2009, 120, 216–225 CrossRef CAS PubMed.
- S. D. Rao, S. A. Banack, P. A. Cox and J. H. Weiss, Exp. Neurol., 2006, 201, 244–252 CrossRef CAS PubMed.
- P. S. Spencer, P. B. Nunn, J. Hugon, A. C. Ludolph, S. M. Ross, D. N. Roy and R. C. Robertson, Science, 1987, 237, 517–522 CrossRef CAS PubMed.
- W. B. Glover, D. C. Mash and S. J. Murch, Amino Acids, 2014, 46, 2553–2559 CrossRef CAS.
- R. A. Dunlop, P. A. Cox, S. A. Banack and K. J. Rodgers, PLoS One, 2013, 8, 1–8 CrossRef.
- P. A. Cox, D. A. Davis, D. C. Mash, J. S. Metcalf and S. A. Banack, Proc. R. Soc. B, 2016, 283, 1–10 CrossRef.
- D. A. Davis, K. Mondo, E. Stern, A. K. Annor, S. J. Murch, T. M. Coyne, L. E. Brand, M. E. Niemeyer, S. Sharp, W. G. Bradley, P. A. Cox and D. C. Mash, PLoS One, 2019, 14, 1–18 Search PubMed.
- O. Karlsson, C. Berg, E. B. Brittebo and N. G. Lindquist, Pigm. Cell Melanoma Res., 2009, 22, 120–130 CrossRef CAS.
- M. Arif, S. F. Kazim, I. Grundke-Iqbal, R. M. Garruto and K. Iqbal, Proc. Natl. Acad. Sci. U. S. A., 2014, 111, 1144–1149 CrossRef CAS.
- A. Rauk, J. Phys. Chem. B, 2018, 122, 4472–4480 CrossRef CAS.
- W. B. Glover, C. M. Liberto, W. S. McNeil, S. A. Banack, P. R. Shipley and S. J. Murch, Anal. Chem., 2012, 84, 7946–7953 CrossRef CAS.
- P. Diaz-Parga, J. J. Goto and V. V. Krishnan, Neurotoxic. Res., 2018, 33, 76–86 CrossRef CAS.
- S. A. Banack and S. J. Murch, Neurotoxic. Res., 2018, 33, 184–191 CrossRef CAS.
- S. A. Banack, J. S. Metcalf, Z. Spáčil, T. G. Downing, S. Downing, A. Long, P. B. Nunn and P. A. Cox, Toxicon, 2011, 57, 730–738 CrossRef CAS.
- S. A. Banack, J. S. Metcalf, L. Jiang, D. Craighead, L. L. Ilag and P. A. Cox, PLoS One, 2012, 7, 1–4 CrossRef PubMed.
- S. L. Bishop, J. K. Kerkovius, F. Menard and S. J. Murch, Neurotoxic. Res., 2018, 33, 133–142 CrossRef CAS.
- D. G. Beach, E. S. Kerrin and M. A. Quilliam, Anal. Bioanal. Chem., 2015, 407, 8397–8409 CrossRef CAS.
- L. Jiang, B. Aigret, W. M. De Borggraeve, Z. Spacil and L. L. Ilag, Anal. Bioanal. Chem., 2012, 403, 1719–1730 CrossRef CAS.
- A. Li, Y. Hu, J. Song, S. Wang and L. Deng, Toxicon, 2018, 151, 129–136 CrossRef CAS.
- A. J. Foss, N. Chernoff and M. T. Aubel, Toxicon, 2018, 152, 150–159 CrossRef CAS.
- D. Réveillon, V. Séchet, P. Hess and Z. Amzil, Toxicon, 2016, 110, 35–46 CrossRef.
- G. E. Kisby, D. N. Roy and P. S. Spencer, J. Neurosci. Methods, 1988, 26, 45–54 CrossRef CAS.
- M. Esterhuizen-Londt, S. Downing and T. Downing, Water SA, 2011, 37, 133–139 CrossRef CAS.
- S. A. Banack, H. E. Johnson, R. Cheng and P. A. Cox, Mar. Drugs, 2007, 5, 180–196 CrossRef CAS.
- M. L. Salomonsson, A. Hansson and U. Bondesson, Anal. Methods, 2013, 5, 4865–4874 RSC.
- J. Rosén and K. E. Hellenäs, Analyst, 2008, 133, 1785–1789 RSC.
- S. Moura, M. de A. Ultramari, D. M. L. de Paula, M. Yonamine and E. Pinto, Toxicon, 2009, 53, 578–583 CrossRef CAS.
- M. S. Baptista, R. C. C. Cianca, V. R. Lopes, C. M. R. Almeida and V. M. Vasconcelos, Toxicon, 2011, 58, 410–414 CrossRef CAS.
- E. J. Faassen, W. Beekman and M. Lürling, PLoS One, 2013, 8, 1–8 CrossRef.
- H. E. Johnson, S. R. King, S. A. Banack, C. Webster, W. J. Callanaupa and P. A. Cox, J. Ethnopharmacol., 2008, 118, 159–165 CrossRef CAS.
- R. Cheng and S. A. Banack, Amyotrophic Lateral Scler., 2009, 10, 41–43 CrossRef CAS.
- E. J. Faassen, F. Gillissen and M. Lürling, PLoS One, 2012, 7, 1–8 CrossRef.
- M. W. Duncan, Adv. Neurol., 1991, 56, 301–310 CAS.
- M. Duncan, I. Kopin, J. S. Crowley, S. M. Jones and S. P. Markey, J. Anal. Toxicol., 1989, 13, 169–175 CrossRef CAS.
- G. E. Kisby, M. Ellison and P. S. Spencer, Neurology, 1992, 42, 1336–1340 CrossRef CAS.
- I. Khabazian, J. S. Bains, D. E. Williams, J. Cheung, J. M. B. Wilson, B. A. Pasqualotto, S. L. Pelech, R. J. Andersen, Y. T. Wang, L. Liu, A. Nagai, S. U. Kim, U. K. Craig and C. A. Shaw, J. Neurochem., 2002, 82, 516–528 CrossRef CAS PubMed.
- T. Kubo, N. Kato, K. Hosoya and K. Kaya, Toxicon, 2008, 51, 1264–1268 CrossRef CAS.
- Z. Spácil, J. Eriksson, S. Jonasson, U. Rasmussen, L. L. Ilag and B. Bergman, Analyst, 2010, 135, 127–132 RSC.
- B. R. Roney, L. Renhui, S. A. Banack, S. Murch, R. Honegger and P. A. Cox, Amyotrophic Lateral Scler., 2009, 10, 44–49 CrossRef CAS PubMed.
- M. Esterhuizen-Londt and T. Downing, Water SA, 2011, 37, 523–528 CrossRef CAS.
- B. Yan, Z. Liu, R. Huang, Y. Xu, D. Liu, T. F. Lin and F. Cui, Anal. Chem., 2017, 89, 10991–10998 CrossRef CAS PubMed.
- D. G. Beach, E. S. Kerrin, S. D. Giddings, M. A. Quilliam and P. McCarron, Sci. Rep., 2018, 8, 1–11 CrossRef CAS.
- W. B. Glover, T. C. Baker, S. J. Murch and P. N. Brown, J. AOAC Int., 2015, 98, 1559–1565 CrossRef CAS.
- AOAC, AOAC Off. Methods Anal., 2013, 1–32 Search PubMed.
- D. Moher, A. Liberati, J. Tetzlaff, D. G. Altman, D. Altman, G. Antes, D. Atkins, V. Barbour, N. Barrowman, J. A. Berlin, J. Clark, M. Clarke, D. Cook, R. D'Amico, J. J. Deeks, P. J. Devereaux, K. Dickersin, M. Egger, E. Ernst, P. C. Gøtzsche, J. Grimshaw, G. Guyatt, J. Higgins, J. P. A. Ioannidis, J. Kleijnen, T. Lang, N. Magrini, D. McNamee, L. Moja, C. Mulrow, M. Napoli, A. Oxman, B. Pham, D. Rennie, M. Sampson, K. F. Schulz, P. G. Shekelle, D. Tovey and P. Tugwell, J. Chin. Integr. Med., 2009, 7, 889–896 CrossRef.
- S. Jonasson, J. Eriksson, L. Berntzon, Z. Spácil, L. L. Ilag, L.-O. Ronnevi, U. Rasmussen and B. Bergman, Proc. Natl. Acad. Sci. U. S. A., 2010, 107, 9252–9257 CrossRef CAS PubMed.
- M. Esterhuizen and T. G. Ã. Downing, Ecotoxicol. Environ. Saf., 2008, 71, 309–313 CrossRef CAS PubMed.
- K. Mondo, W. B. Glover, S. J. Murch, G. Liu, Y. Cai, D. A. Davis and D. C. Mash, Food Chem. Toxicol., 2014, 70, 26–32 CrossRef CAS PubMed.
- T. J. Montine, K. Li, D. P. Perl and D. Galasko, Neurology, 2005, 65, 768–769 CrossRef CAS PubMed.
- L. Berntzon, L. O. Ronnevi, B. Bergman and J. Eriksson, Neuroscience, 2015, 292, 137–147 CrossRef CAS PubMed.
- D. Bhandari, B. A. Bowman, A. B. Patel, D. M. Chambers, V. R. De Jesús and B. C. Blount, J. Chromatogr. B: Anal. Technol. Biomed. Life Sci., 2018, 1083, 86–92 CrossRef CAS PubMed.
- L. Bláhová, J. Kohoutek, E. Kadlecová, L. Kozáková and L. Bláha, Toxicon, 2017, 133, 48–57 CrossRef PubMed.
- S. Downing, L. L. Scott, N. Zguna and T. G. Downing, Toxins, 2018, 10, 1–15 Search PubMed.
- S. A. Banack, T. G. Downing, Z. Spácil, E. L. Purdie, J. S. Metcalf, S. Downing, M. Esterhuizen, G. A. Codd and P. A. Cox, Toxicon, 2010, 56, 868–879 CrossRef CAS PubMed.
- D. Craighead, J. S. Metcalf, S. A. Banack, L. Amgalan, H. V. Reynolds and M. Batmunkh, Amyotrophic Lateral Scler., 2009, 10, 96–100 CrossRef CAS PubMed.
- A. Mlinarić, M. Horvat and V. Š. Smolčić, Biochem. Med., 2017, 27, 1–6 Search PubMed.
- J. P. Meneely, O. P. Chevallier, S. Graham, B. Greer, B. D. Green and C. T. Elliott, Sci. Rep., 2016, 6, 1–9 CrossRef PubMed.
- M. W. Duncan, J. Mass Spectrom., 2012, 47, 795–809 CrossRef CAS PubMed.
- E. J. Faassen, Toxins, 2014, 6, 1109–1138 CrossRef PubMed.
- D. S. S. Lim, B. E. Laval, G. Slater, D. Antoniades, A. L. Forrest, W. Pike, R. Pieters, M. Saffari, D. Reid, D. Schulze-Makuch, D. Andersen and C. P. Mckay, Fundam. Appl. Limnol., 2009, 173, 329–351 CrossRef CAS.
- S. Downing, M. van de Venter and T. G. Downing, Microb. Ecol., 2012, 63, 149–156 CrossRef CAS PubMed.
- M. Monteiro, M. Costa, C. Moreira, V. M. Vasconcelos and M. S. Baptista, J. Appl. Phycol., 2017, 29, 879–888 CrossRef CAS.
- H. S. Yin, B. C. Li, Y. L. Zhou, H. Y. Wang, M. H. Wang and S. Y. Ai, Biosens. Bioelectron., 2017, 96, 106–112 CrossRef CAS PubMed.
- E. Pip, K. Munford and L. Bowman, Environ. Pollut., 2016, 5, 110–118 CrossRef CAS.
- Y. Jiao, Q. Chen, X. Chen, X. Wang, X. Liao, L. Jiang, J. Wu and L. Yang, Sci. Total Environ., 2014, 468–469, 457–463 CrossRef CAS PubMed.
- E. J. Faassen, M. G. Antoniou, W. Beekman-Lukassen, L. Blahova, E. Chernova, C. Christophoridis, A. Combes, C. Edwards, J. Fastner, J. Harmsen, A. Hiskia, L. L. Ilag, T. Kaloudis, S. Lopicic, M. Lürling, H. Mazur-Marzec, J. Meriluoto, C. Porojan, Y. Viner-Mozzini and N. Zguna, Mar. Drugs, 2016, 14, 1–12 CrossRef PubMed.
- A. Li, H. Fan, F. Ma, P. McCarron, K. Thomas, X. Tang and M. A. Quilliam, Analyst, 2012, 137, 1210 RSC.
-
E. J. Faassen, in Handbook of Cyanobacterial Monitoring and Cyanotoxin Analysis, ed. J. Meriluoto, L. Spoof and G. A. Codd, John Wiley & Sons, West Sussex, 2017, pp. 439–446 Search PubMed.
- M. T. Clausi, V. Vita, M. Bruno, C. Franchino, G. Trifirò, M. P. Palumbo, F. Floridi and R. De Pace, Int. J. Environ. Anal. Chem., 2016, 96, 1290–1299 CrossRef CAS.
- W. J. Youden, Anal. Chem., 1948, 20, 1136–1140 CrossRef CAS.
- P. B. Nunn, P. O'Brien, L. D. Pettit and S. I. Pyburn, J. Inorg. Biochem., 1989, 37, 175–183 CrossRef CAS PubMed.
- J. Zurita, N. Zguna, R. Andrýs, A. Strzelczak, L. Jiang, G. Thorsen and L. L. Ilag, Anal. Methods, 2019, 11, 432–442 RSC.
- D. Réveillon, E. Abadie, V. Séchet, L. Brient, V. Savar, M. Bardouil, P. Hess and Z. Amzil, Mar. Drugs, 2014, 12, 5441–5467 CrossRef PubMed.
- E. S. Kerrin, R. L. White and M. A. Quilliam, Anal. Bioanal. Chem., 2017, 409, 1481–1491 CrossRef CAS PubMed.
- J. Beri, K. I. Kirkwood, D. C. Muddiman and M. S. Bereman, Anal. Bioanal. Chem., 2018, 410, 2597–2605 CrossRef CAS PubMed.
- H. Almuhtaram, Y. Cui, A. Zamyadi and R. Hofmann, Toxins, 2018, 10, 1–15 CrossRef PubMed.
- J. Rosén, E. Westerberg, S. Schmiedt and K. E. Hellenäs, Toxicon, 2016, 109, 45–50 CrossRef PubMed.
- J. P. Violi, S. M. Mitrovic, A. Colville, B. J. Main and K. J. Rodgers, Ecotoxicol. Environ. Saf., 2019, 172, 72–81 CrossRef CAS PubMed.
- M. Esterhuizen-Londt and S. Pflugmacher, Environ. Res., 2019, 169, 357–361 CrossRef CAS PubMed.
- B. Li, S. Yu, G. Li, X. Chen, M. Huang, X. Liao, H. Li, F. Hu and J. Wu, Chemosphere, 2019, 219, 997–1001 CrossRef CAS PubMed.
- J. S. Metcalf, S. Anne, J. T. Powell, F. J. M. Tymm, S. J. Murch, L. E. Brand and P. A. Cox, Water Policy, 2018, 20, 919–932 CrossRef.
- A. D. Jungblut, J. Wilbraham, S. A. Banack, J. S. Metcalf and G. A. Codd, Eur. J. Phycol., 2018, 53, 115–121 CrossRef CAS.
- A. D. Chatziefthimiou, E. J. Deitch, W. B. Glover, J. T. Powell, S. A. Banack, R. A. Richer, P. A. Cox and J. S. Metcalf, Neurotoxic. Res., 2018, 33, 143–152 CrossRef CAS PubMed.
- B. J. Main and K. J. Rodgers, Neurotoxic. Res., 2018, 33, 33–42 CrossRef CAS PubMed.
- L. L. Scott, S. Downing and T. Downing, Toxicon, 2018, 150, 261–266 CrossRef CAS PubMed.
- P. Rzymski, B. Poniedziałek, J. Mankiewicz-Boczek, E. J. Faassen, T. Jurczak, I. Gągała-Borowska, A. Ballot, M. Lürling and M. Kokociński, Algal Res., 2017, 24, 72–80 CrossRef.
- A. C. Braga, S. Lage, M. Pacheco, S. Rydberg and P. R. Costa, Mar. Environ. Res., 2017, 129, 147–155 CrossRef CAS PubMed.
- J. S. Metcalf, D. Lobner, S. A. Banack, G. A. Cox, P. B. Nunn, P. B. Wyatt and P. A. Cox, Amino Acids, 2017, 49, 1427–1439 CrossRef CAS PubMed.
- A. Roy-Lachapelle, M. Solliec, M. F. Bouchard and S. Sauvé, Toxins, 2017, 9, 1–18 CrossRef PubMed.
- J. Rosén, E. Westerberg, K. E. Hellenäs and M. L. Salomonsson, Toxicon, 2016, 121, 105–108 CrossRef PubMed.
- A. Li, J. Song, Y. Hu, L. Deng, L. Ding and M. Li, Mar. Drugs, 2016, 14, 1–12 CrossRef PubMed.
- N. Hammerschlag, D. Davis, K. Mondo, M. Seely, S. Murch, W. Brocglover, T. Divoll, D. Evers and D. C. Mash, Toxins, 2016, 8, 1–14 CrossRef PubMed.
- A. D. Chatziefthimiou, J. S. Metcalf, W. B. Glover, S. A. Banack, S. R. Dargham and R. A. Richer, Toxicon, 2016, 114, 75–84 CrossRef CAS PubMed.
- H. Fan, J. Qiu, L. Fan and A. Li, Environ. Sci. Pollut. Res., 2015, 22, 5943–5951 CrossRef CAS PubMed.
- S. A. Banack, T. Caller, P. Henegan, J. Haney, A. Murby, J. S. Metcalf, J. Powell, P. Alan and E. Stommel, Toxins, 2015, 7, 322–336 CrossRef CAS PubMed.
- R. Richer, S. A. Banack, J. S. Metcalf and P. A. Cox, J. Arid Environ., 2015, 112, 134–139 CrossRef.
- R. Andrýs, J. Zurita, N. Zguna, K. Verschueren, W. M. De Borggraeve and L. L. Ilag, Anal. Bioanal. Chem., 2015, 407, 3743–3750 CrossRef PubMed.
- M. L. Salomonsson, E. Fredriksson, A. Alfjorden, M. Hedeland and U. Bondesson, Toxicol. Rep., 2015, 2, 1473–1481 CrossRef CAS PubMed.
- A. Roy-Lachapelle, M. Solliec and S. Sauvé, Anal. Bioanal. Chem., 2015, 407, 5487–5501 CrossRef CAS PubMed.
- J. S. Metcalf, S. A. Banack, R. Richer and P. A. Cox, J. Arid Environ., 2015, 112, 140–144 CrossRef.
- P. McCarron, A. C. Logan, S. D. Giddings and M. A. Quilliam, Aquat. Biosyst., 2014, 10, 1–7 CrossRef.
- A. Combes, S. El Abdellaoui, J. Vial, E. Lagrange and V. Pichon, Anal. Bioanal. Chem., 2014, 406, 4627–4636 CrossRef CAS PubMed.
- M. A. Al-Sammak, K. D. Hoagland, D. Cassada and D. D. Snow, Toxins, 2014, 6, 488–508 CrossRef CAS PubMed.
- S. A. Banack, J. S. Metcalf, W. G. Bradley and P. A. Cox, Toxicon, 2014, 90, 167–173 CrossRef CAS PubMed.
- S. Lage, P. R. Costa, T. Moita, J. Eriksson, U. Rasmussen and S. J. Rydberg, Aquat. Toxicol., 2014, 152, 131–138 CrossRef CAS PubMed.
- L. L. Scott, S. Downing, R. R. Phelan and T. G. Downing, Toxicon, 2014, 87, 1–5 CrossRef CAS PubMed.
- S. Downing, V. Contardo-Jara, S. Pflugmacher and T. G. Downing, Ecotoxicol. Environ. Saf., 2014, 101, 51–58 CrossRef CAS PubMed.
- A. Combes, S. El Abdellaoui, C. Sarazin, J. Vial, A. Mejean, O. Ploux and V. Pichon, Anal. Chim. Acta, 2013, 771, 42–49 CrossRef CAS PubMed.
- M. A. Al-Sammak, K. D. Hoagland, D. D. Snow and D. Cassada, Toxicon, 2013, 76, 316–325 CrossRef CAS PubMed.
- E. Masseret, S. Banack, F. Boumédiène, E. Abadie, L. Brient, F. Pernet, R. Juntas-Morales, N. Pageot, J. Metcalf, P. Cox, W. Camu, G. Besson, V. Bonneterre, P. Couratier, M. Druet-Cabanac, E. Lagrange, B. Marin, A. Mejean and P. M. Preux, PLoS One, 2013, 8, 1–10 CrossRef PubMed.
- J. S. Metcalf, S. A. Banack, K. Kotut, L. Krienitz and G. A. Codd, Chemosphere, 2013, 90, 835–839 CrossRef CAS PubMed.
- L. Jiang, E. Johnston, K. M. Åberg, U. Nilsson and L. L. Ilag, Anal. Bioanal. Chem., 2013, 405, 1283–1292 CrossRef CAS PubMed.
- N. C. Field, J. S. Metcalf, T. A. Caller, S. A. Banack, P. A. Cox and E. W. Stommel, Toxicon, 2013, 70, 179–183 CrossRef CAS PubMed.
- K. Mondo, N. Hammerschlag, M. Basile, J. Pablo, S. A. Banack and D. C. Mash, Mar. Drugs, 2012, 10, 509–520 CrossRef CAS PubMed.
- R. C. Cervantes Cianca, M. S. Baptista, L. P. Da Silva, V. R. Lopes and V. M. Vasconcelos, Toxicon, 2012, 59, 379–384 CrossRef CAS PubMed.
- R. C. Cervantes Cianca, M. S. Baptista, V. R. Lopes and V. M. Vasconcelos, Amino Acids, 2012, 42, 2473–2479 CrossRef CAS PubMed.
- S. J. Christensen, T. K. Hemscheidt, H. Trapido-Rosenthal, E. A. Laws and R. R. Bidigare, Limnol. Oceanogr.: Methods, 2012, 10, 891–898 CrossRef CAS.
- K. de C. Mariotti, F. Barreto, G. C. Schmitt, I. Zancanaro, E. Dallegrave, R. B. Singer, M. B. Leal and R. P. Limberger, Braz. J. Pharm. Sci., 2011, 47, 623–628 CrossRef CAS.
- S. Downing, S. A. Banack, J. S. Metcalf, P. A. Cox and T. G. Downing, Toxicon, 2011, 58, 187–194 CrossRef CAS PubMed.
- T. E. Marler, L. R. Snyder and C. A. Shaw, Toxicon, 2010, 56, 563–568 CrossRef CAS PubMed.
- T. Krüger, B. Mönch, S. Oppenhäuser and B. Luckas, Toxicon, 2010, 55, 547–557 CrossRef PubMed.
- A. Li, Z. Tian, J. Li, R. Yu, S. A. Banack and Z. Wang, Toxicon, 2010, 55, 947–953 CrossRef CAS PubMed.
- L. R. Snyder, R. Cruz-Aguado, M. Sadilek, D. Galasko, C. A. Shaw and T. J. Montine, Neurology, 2009, 72, 1360–1361 CrossRef CAS PubMed.
- E. J. Faassen, F. Gillissen, H. A. J. Zweers and M. Lrling, Amyotrophic Lateral Scler., 2009, 10, 79–84 CrossRef CAS PubMed.
- P. A. Cox, R. Richer, J. S. Metcalf, S. A. Banack, G. A. Codd and W. G. Bradley, Amyotrophic Lateral Scler., 2009, 10, 109–117 CrossRef CAS PubMed.
- J. Eriksson, S. Jonasson, D. Papaefthimiou, U. Rasmussen and B. Bergman, Amino Acids, 2009, 36, 43–48 CrossRef CAS PubMed.
- T. A. Caller, J. W. Doolin, J. F. Haney, A. J. Murby, K. G. West, H. E. Farrar, A. Ball, B. T. Harris and E. W. Stommel, Amyotrophic Lateral Scler., 2009, 10, 101–108 CrossRef CAS PubMed.
- P. M. Scott, B. Niedzwiadek, D. F. K. Rawn and B. P.-Y. Lau, J. Food Prot., 2009, 72, 1769–1773 CrossRef CAS PubMed.
- M. M. Kushnir and J. Bergquist, Eur. J. Mass. Spectrom., 2009, 15, 439–443 CrossRef CAS PubMed.
- R. R. Bidigare, S. J. Christensen, S. B. Wilde and S. A. Banack, Amyotrophic Lateral Scler., 2009, 10, 71–73 CrossRef PubMed.
- J. S. Metcalf, S. A. Banack, J. Lindsay, L. F. Morrison, P. A. Cox and G. A. Codd, Environ. Microbiol., 2008, 10, 702–708 CrossRef CAS PubMed.
- S. J. Murch, P. A. Cox and S. A. Banack, Proc. Natl. Acad. Sci. U. S. A., 2004, 101, 12228–12231 CrossRef CAS PubMed.
- S. A. Banack and P. A. Cox, Bot. J. Linn. Soc., 2003, 143, 165–168 CrossRef.
- D. Wiltsie, A. Schnetzer, J. Green, M. Vander Borgh and E. Fensin, Toxins, 2018, 10, 1–23 CrossRef PubMed.
- E. S. Kerrin, R. L. White and M. A. Quilliam, Anal. Bioanal. Chem., 2017, 409, 1481–1491 CrossRef CAS PubMed.
Footnote |
† Electronic supplementary information (ESI) available. See DOI: 10.1039/c9an01252d |
|
This journal is © The Royal Society of Chemistry 2020 |
Click here to see how this site uses Cookies. View our privacy policy here.