Simulation of H2/CH4 mixture permeation through MOF membranes using non-equilibrium molecular dynamics†
Received
22nd October 2018
, Accepted 19th December 2018
First published on 15th January 2019
Abstract
Grand canonical Monte Carlo (GCMC) simulations are widely used with equilibrium molecular dynamics (EMD) to predict gas adsorption and diffusion in single-crystals of metal–organic frameworks (MOFs). Adsorption and diffusion data obtained from these simulations are then combined to predict gas permeabilities and selectivities of MOF membranes. This GCMC + EMD approach is highly useful to screen a large number of MOFs for a target membrane-based gas separation process. External field non-equilibrium molecular dynamics (NEMD) simulations, on the other hand, can directly compute gas permeation by providing an accurate representation of MOF membranes but they are computationally demanding and require long simulation times. In this work, we performed NEMD simulations to investigate H2/CH4 separation performances of MOF membranes. Both single-component and binary mixture permeabilities of H2 and CH4 were computed using the NEMD approach and results were compared with the predictions of the GCMC + EMD approach and experimental measurements reported in the literature. Our results showed that there is a good agreement between NEMD simulations and experiments for the permeability and selectivity of MOF membranes. NEMD simulations provided the direct observation of the mass transfer resistances on the pore mouth of MOF membranes, which is neglected in the GCMC + EMD approach. Our results suggested that once the very large numbers of MOF materials were screened using the GCMC + EMD approach, more detailed NEMD calculations can be performed for the best membrane candidates to unlock the actual gas transport mechanism before the experimental fabrication of MOF membranes.
Introduction
Hydrogen (H2) has been accepted as an alternative energy carrier for fuel cell electric devices and as a storage medium in stationary fuel cell systems. H2 is commonly produced either by the reformation of hydrocarbons or by the decomposition of methane (CH4) and it should be purified to be used in industrial applications. Separation of H2 from natural gas, which is mainly composed of CH4, through a conventional physisorption process is challenging due to the very similar physical and chemical properties of H2 and CH4. Membrane-based gas separation technology offers a great advantage for separation of H2 from H2/CH4 mixtures because of the differences in the kinetic diameters of H2 and CH4 molecules. Membrane-based H2/CH4 separation is accepted as an industry-practiced technology for H2 purification and it has several advantages such as low energy consumption, facile and adaptable operation, and cost effectiveness. However, there are challenges in tuning the pore structure of membranes. Metal–organic frameworks (MOFs) are different from other porous membrane materials due to the ability to tune their pore sizes and shapes in addition to their inherent mechanical and thermal stabilities.1,2 However, finding the optimal MOF membrane for a target gas separation is a tedious task because thousands of MOFs have been already synthesized to date and it is not practical to fabricate membranes from every synthesized MOF and test their separation performances using purely experimental ways. High-throughput computational studies, which screen thousands of MOFs to identify the best materials for a desired separation process, play an important role in directing the experimental efforts, time and resources to the most promising materials for the development of high-performance MOF membranes.
Molecular simulations have been extensively used in high-throughput screening studies to examine gas adsorption and gas diffusion in MOFs.3 Adsorption properties of gases in MOFs are calculated using grand canonical Monte Carlo (GCMC) simulations, and diffusivities of gases are estimated from the mean square displacement of the gas molecules in MOFs determined from the equilibrium molecular dynamics (EMD) simulations. Adsorption data obtained from the GCMC simulations and diffusion data obtained from the EMD simulations are then combined to predict gas permeabilities through MOF membranes. In this method, which will be referred to as GCMC + EMD throughout this manuscript, both simulations are performed using the unit cell representation of single MOF crystals without a membrane representation. It is assumed that a MOF membrane would have the same gas adsorption and diffusion properties as those of the single-crystals of the MOF. Non-equilibrium MD (NEMD) simulations, on the other hand, directly compute gas permeabilities by the proper representation of an experimental membrane system in which gas permeation results from the successive adsorption, diffusion and desorption processes. NEMD simulations compute the desired properties such as thermal conductivity, mass transport, components of the stress tensor, etc. by using external forces or thermostats.4,5 A range of gradients such as temperature, concentration, and pressure may be used for both linear and nonlinear transport responses. Since the equivalence of such a homogeneous external forcing function that drives the gas diffusion has not been formally demonstrated, NEMD simulations are not widely used in predicting the gas transport of membranes.6 In EMD simulations, mass transfer resistance through the nanopore mount is not taken into account. However, it is well-known that this resistance is the rate-limiting step in the mass transfer of real porous systems. Mass transfer resistance on the nanopore surface can lead to concentration polarization which yields a decrease in the permeability and selectivity of the membrane material. Pore blockage may also occur in the pore mount of materials because of this resistance.7 NEMD simulations account for the mass transfer resistance and the resistance in the entrance of the pore mouth can be shown with the density distribution of the adsorbed gases on the surface of the membrane along the x-axis. For example, Newsome and Sholl8 investigated the mass transfer resistance and its importance using the NEMD simulations for gas transport through zeolite membranes. They concluded that the density of CH4 in the zeolite–gas interface is almost half of its density inside the zeolite membrane resulting in a considerable mass resistance at the entrance of the zeolite membrane. Another difference between the EMD and NEMD simulations is that the former calculates the self-diffusion coefficients of gases to determine the transport properties, which is correct at only infinitely low gas uptakes.9 Transport diffusivities should be computed to describe diffusion in the presence of a concentration gradient10 and NEMD simulations access the actual gas permeation through nanoporous membranes using transport diffusivities.4,6,9
NEMD simulations have been recently used to examine water transport across various nanoporous materials such as zeolites,11 carbon nanotubes,12 graphene13 and graphene oxide nanosheets,14 and aquaporin.15 In contrast to water simulations, a small variety of materials, such as polymers of intrinsic microporosity,5 zeolites,10 inorganic mesoporous silica,16 and MOFs,17–20 were investigated using different NEMD simulation approaches such as an external field-NEMD (EF-NEMD), dual control volume grand canonical molecular dynamics (DCV-GCMD), and impermeable moving wall approach in gas simulation studies. Snurr's group10 estimated the transport properties of binary mixtures of short n-alkanes in faujasite type zeolite by using both the EMD and NEMD simulations. They reported that error bars in the Onsager coefficient, which is analogous to the diffusivities of tagged gas molecules, are smaller in the NEMD simulations than the ones in the EMD simulations.10 Frentrup et al.5 used the NEMD simulations to examine the permeation of He and CO2 across a polymeric membrane and a good agreement between experiments and simulations was reported although there were large uncertainties in the experimental permeability data.5 Transport diffusivities of H2 in four different MOFs were estimated using the EF-NEMD simulations to study MOF adsorbents for H2 purification.18 Jiang et al.19 investigated the glucose adsorption properties of three MOFs using the NEMD simulations. The effects of the presence of impurities in the aqueous solution on the glucose adsorption were discussed by analyzing the mean square displacement and the density profile of glucose.19 Ozcan et al.20 proposed a modified EF-NEMD approach named as concentration gradient driven molecular dynamics and tested it on a ZIF-8 membrane by computing methane, ethane and ethylene permeation. Wilmer et al.17 reported CH4 transport in a widely studied MOF, Cu-BTC, using the NEMD simulations and suggested that the gas density leading to mass transport resistance in the MOF–gas interface is higher than the bulk gas density. As can be seen from this literature review, a study comparing the GCMC + EMD and NEMD methods for predicting the gas permeation performances of MOF membranes is still missing.
Motivated by this, we aimed to compare the gas permeation results of these two methods with the experimental permeation measurements of the MOF membranes to reveal the advantages and limitations of both simulation methods. In this work, we performed the NEMD simulations to provide a detailed understanding of the complex nature of transport and separation of H2/CH4 mixtures through MOF membranes. We focused on three well-known MOFs (MOF-5, Cu-BTC and ZIF-8) since they have been fabricated and tested as gas separating membranes, which makes it possible to compare the results of NEMD simulations with the experimental data. We also studied a MOF (MEFMEQ) that was previously identified as the top membrane candidate for H2/CH4 separation in a high-throughput computational screening study21 which used the GCMC + EMD simulations. Both single-component and binary mixture permeabilities of H2 and CH4 predicted from GCMC + EMD and NEMD simulations were compared in detail for each MOF membrane.
Methods and simulation details
GCMC + EMD simulations
Considering the very large number of MOFs, experimental fabrication and testing of a MOF membrane is significantly time-consuming and requires substantial resources and efforts. We recently discussed that although several thousands of MOFs have been synthesized, only a small number of MOF structures has been experimentally tested as membranes.22 High-throughput computational screening using the combination of GCMC and EMD simulation methods provides an opportunity to define the best performing MOF membranes. In these screening studies, GCMC simulations are used to compute gas adsorption data in MOFs and EMD simulations are used to calculate the self-diffusion coefficients of adsorbed gases in the MOFs. In other words, the results of GCMC simulations, adsorbed gas loadings, are used as the input of EMD simulations. Adsorption and diffusion data are then combined to predict the gas permeability through a single MOF crystal. The huge potential of using GCMC + EMD simulations in screening a large number of materials and identifying the promising MOFs for a desired gas separation has been provided in several studies.21,23–25 More details of this method can be found in our previous studies.21,26
NEMD simulations
NEMD is a simulation technique ideally suited to represent an experimental membrane system in which an external driving force, such as a chemical potential or pressure gradient, is applied to a membrane. A schematic representation of the initial design of a MOF membrane system used in our simulations is shown in Fig. 1. The external driving force is applied to gas molecules that fall into the defined region or slab in the z-direction. Each system is composed of four layers, namely graphene, gas, MOF and vacuum layers. Since we aimed to have a concentration gradient between the entrance and exit of the MOF membrane, a gas bath with a volume of ∼40 × 40 × 80 Å3 was placed at one side and a vacuum layer with 200 Å length in the x-axis was located on the other side as illustrated in Fig. 1. The purpose of aligning the graphene sheet parallel to the MOF in the initial equilibration step was to create a physical barrier to prevent the escape of the gas molecules towards the vacuum region due to the periodicity of the unit cell. In the production step, the interaction between graphene and gas molecules was turned-off to eliminate the physical barrier effect of the graphene layer.
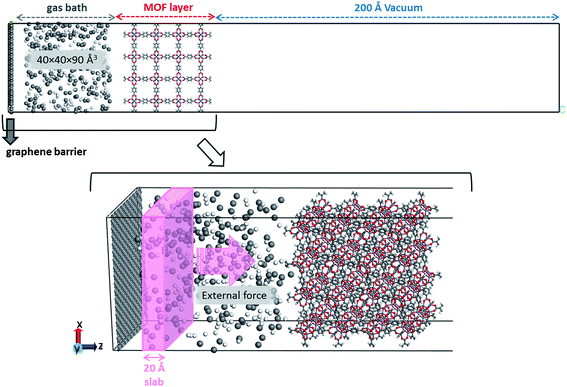 |
| Fig. 1 Representation of the simulation system. Each system consists of four regions: a graphene barrier, well-equilibrated gas bath, MOF layer and vacuum region. H2 and CH4 are shown as spheres by the van der Waals (vdW) representation in a gas bath with white and grey colors, respectively. Hydrogen, carbon, oxygen and zinc atoms in the MOF structure (MOF-5) and in the graphene sheet are shown by the ball-and-stick representation with white, grey, red and dark blue colors, respectively. | |
All molecular dynamics simulations were carried out using the Large-scale Atomic/Molecular Massively Parallel Simulator (LAMMPS),27 and analyses and molecular visualization were performed using Visual Molecular Dynamics (VMD)28 packages. The initial configurations of each system were generated using the Packmol software.29 The gas bath consisted of equimolar H2/CH4 mixtures, having 200 H2 and 200 CH4 molecules. Both gases were modeled by single-site spherical LJ 12–6 potentials.30,31 MOF atoms which were fixed during the simulations were modeled using the Universal Force Field (UFF).32 A cut-off distance of 18 Å was adopted for non-bonded interactions. In order to have approximately 40–50 Å thick MOF membranes, we extended the primitive length of MOF, which is periodic in x- and y-axes, by two- or three-fold. Although this membrane thickness is lower than the actual thickness observed in experiments, simulation studies focusing on porous membranes generally use this range of thickness in order to save computational time.33,34
Initially, adsorption simulations were performed using EMD and the equilibrated system from the adsorption simulations was used in the NEMD simulations. For adsorption simulations, a fixed graphene barrier was used similar to the system configuration shown in Fig. 1. EMD simulations were used to compute gas adsorption properties and results were compared with those obtained from the GCMC simulations. EMD simulations were performed using the Nosé–Hoover thermostat and barostat35,36 with a coupling time constant of 500 fs. The simulation time step was set to 1 fs. Each system was relaxed with zero-temperature minimizations for 1 ns while a well-equilibrated gas bath was in contact with the MOF structure. Then, the temperature of each system was increased to 298 K using a canonical ensemble (NVT) run within 500 ps. Finally, the systems were equilibrated without an external force using an NVT run at 298 K for 4 ns with configurations saved at every 2000 fs time interval and only the final 2 ns was used for analysis.
For the NEMD simulation, the method of external force field was employed to introduce a pressure gradient between phases, namely the permeate and retentate. A constant force was applied in the x-direction to each moving gas molecule in the defined slab as illustrated in Fig. 1. This leads to a pressure difference across the MOF layer and gas fluxes were computed using the gas density distribution at each side of the membrane. Simulations involving pressure differences were performed under NVT using a Nosé–Hoover thermostat35,36 for the MOF membrane whose atoms were tethered to their initial positions with a spring constant of 5 kcal (mol−1 Å−2). On the other hand, gas molecules were left without a thermostat using the microcanonical ensemble (NVE) in order to release the additional energy, which was gained by the external force by interacting with the MOF membrane. We note that simulations may be sensitive to the applied thermostat but the Nosé–Hoover thermostat has been very widely used both in EMD and NEMD studies in the literature.5,11,14,20 The reason for using different ensembles was to eliminate the increase in the kinetic energy of gas molecules resulting from the applied external force and if one wants to keep the system in the steady-state, the generated heat must be removed. Gas flux (J) is simply calculated by counting the net number of gas molecules passing across the MOF layer during 40 ns simulation time (predetermined based on equilibration and production time) using eqn (1):
| 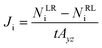 | (1) |
here, for an i type gas molecule,
NLRi and
NRLi are the number of molecules crossing the MOF surface area of
Ayz from left to right and
vice versa, respectively and
t is the total simulation time. The permeability (
Ki) of each gas species was calculated with the help of flux according to
eqn (2),
| 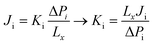 | (2) |
where Δ
Pi =
xiΔ
P is the partial pressure drop for gas type i along the MOF layer, with
xi being the mole fraction, and
Lx is the MOF thickness along the
x-direction. Selectivity is defined as the ratio of the permeability of gases as follows:
|  | (3) |
Results and discussion
MOF-5
MOF-5 (also known as isoreticular MOF (IRMOF-1)) has been the most extensively studied MOF since it is the prototype of MOFs with great potential in gas storage and separation. It has a cubic cage structure with an LCD (largest cavity diameter) of 14.9 Å and PLD (pore limiting diameter) of 7.8 Å in three dimensions as calculated by using the Zeo++ algorithm.37 Three different experimental studies exist on the H2/CH4 separation performance of MOF-5 membranes in the literature38–40 in which H2 and CH4 permeabilities were measured in the range of 1.4 × 104 to 1.6 × 105 and 3.5 × 104 to 1.0 × 105 barrer, respectively. There were differences in the membrane preparation methods in these studies which yield different membrane thicknesses. Liu et al.38 and Yoo et al.39 fabricated thick MOF-5 membranes (∼25, 40 and 85 μm) by the in situ method whereas Zhao et al.40 prepared a thinner membrane (∼14 μm) by the secondary growth method. Available experimental data for all MOF membranes are given in Fig. S1.† Although most gas separation membranes operate at feed pressures between 1 and 10 bar depending on the target gas separation, we conducted the NEMD simulations at pressure differences higher than 10 bar since this allows gaining higher fluxes within the limited simulation time. Transport properties we reported change linearly with the applied external force as shown in Fig. 2(a). A set of field strengths, ranging from 0.03 to 0.15 kcal (mol−1 Å−1), was applied. Using the relationship between the external force and the desired pressure difference, flux data were computed as shown in Fig. 2(a). In order to get gas fluxes at lower pressure differences, one can easily extrapolate the data to lower pressures and define the corresponding external force as shown in the inset graph of Fig. 2(a). Force–flux analysis shown in Fig. 2(a) was only performed for the binary gas mixture permeating through the MOF-5 membrane and was not repeated for the other MOF membranes to save computational time by assuming a linear response. We note that the feed and permeate pressures change between 3 and 4.5, and 2 and 3.5 bar, respectively with a pressure difference of 1 bar at the NEMD simulations whereas both the feed pressure and pressure difference were set to 1 bar in GCMC + EMD simulations since the permeate pressure was assumed as a vacuum.
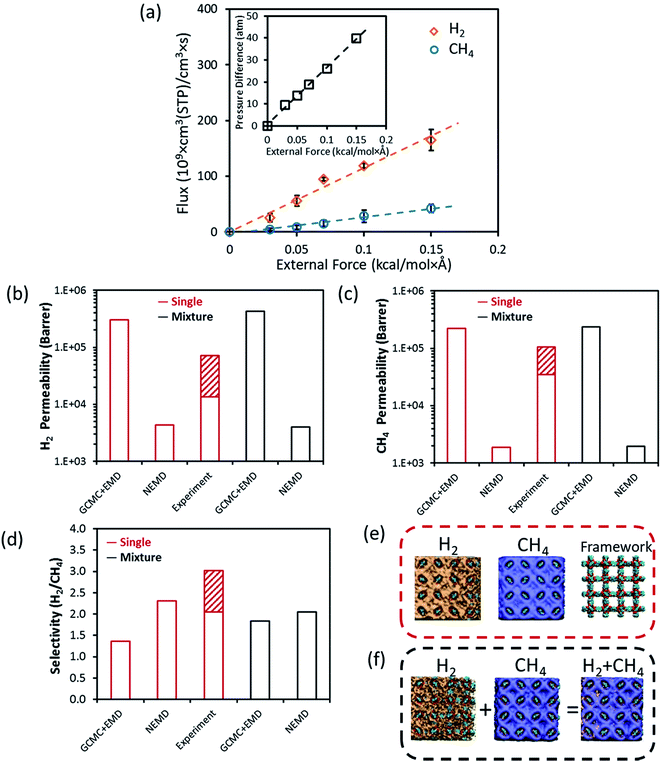 |
| Fig. 2 H2 and CH4 transport data through the MOF-5 membrane. (a) Gas fluxes as a function of external force. The pressure difference as a function of external force is given in the inset figure. Comparison of (b) H2 permeability, (c) CH4 permeability, (d) H2/CH4 selectivity calculated from the GCMC + EMD simulations, NEMD simulations and those reported in experimental studies.38–40 Density occupancy distribution of H2 (orange regions) and CH4 (blue regions) in (e) single-component and (f) binary mixture cases obtained from the NEMD simulations. Hydrogen, carbon, oxygen and zinc atoms in the MOF structure (MOF-5) are shown by the vdW representation with white, cyan, red and dark blue colors, respectively. | |
Fig. 2(b)–(d) compare the transport properties of the MOF-5 membrane, permeability and H2/CH4 selectivity, obtained from the GCMC + EMD simulations with the ones obtained from the NEMD simulations both for single-component gases and binary mixture at 1 bar, 298 K. Experimental data are also shown and the pattern-filled column represents the variations in the experimental measurements at 1–2 bar, 298 K. The minimum and maximum permeability and selectivity values correspond to the bottom and top borders of the pattern-filled column. While equilibrium simulations (GCMC + EMD) overestimate the experimentally reported single-component H2 and CH4 permeability data, NEMD simulations underestimate them. Overpredictions of equilibrium simulations were expected since these simulations consider only the self-diffusion coefficient, which is generally higher than the transport diffusivity.4,5 The self-diffusion calculations are correct at the infinite dilution conditions and they only account for the transport of tagged gas molecules instead of considering the transport of the bulk gas phase. Therefore, calculating gas permeability using the self-diffusion coefficients generally overestimates the actual gas permeabilities. NEMD simulations predict lower H2 and CH4 permeabilities for both single-component and binary mixture calculations compared to the GCMC + EMD simulations because they consider the surface resistance effects existing on the feed side of the MOF membrane. This resistance leads to the blockage of the pore mouth and decreases the gas flux. At that point, it is important to note that molecular simulations deal with the ideal membrane structure without defects and/or cracks. Molecular simulations do not consider the non-selective inter-crystalline and distorted regions in the intra-crystalline parts of the MOF membrane which generally result in the enhancement of the gas diffusion coefficients and increase in the gas permeabilities. Knudsen type transport of small gas molecules like H2 through a MOF-5 membrane was suggested in the literature38,39 due to the relatively large LCD (14.9 Å) of MOF-5 compared to the small kinetic diameter of H2 (∼2.5 Å). Knudsen diffusion combined with the non-selective inter-crystalline grain boundaries and defects present in the intra-crystalline regions of the MOF structure may lead to high experimental permeabilities compared to the predictions of the NEMD simulations which only account for the surface diffusion. We so far discussed the differences between the permeability predictions of two different approaches, GCMC + EMD and NEMD, in terms of gas diffusivities because gas adsorption data calculated from the GCMC and EMD simulations performed in this work were found to be close to each other as shown in Fig. S2(a), (e) and (i).† This result indicates that the influence of adsorption on the membrane transport mechanism is less pronounced compared to the diffusion.
The difference between the single-component and mixture permeabilities predicted from the NEMD simulations is not very large as can be seen from Fig. 2(b) and (c). H2 permeability slightly decreases in the binary NEMD simulation compared to the single-component one whereas CH4 permeability slightly increases. This can be attributed to the multi-component mixture effects: the bulky structure of CH4 molecule limits the diffusion of H2 by blocking the pore mouth and standing as a barrier for H2 diffusion. The presence of the fast diffusing H2 molecules increases the diffusion of CH4 molecules. As a result, there is a small reduction in the mixture selectivity compared to the ideal selectivity computed from the single-component gas permeabilities. This is also due to the competition between the gas molecules for the same preferential adsorption sites of MOF-5. Fig. 2(e) and (f) show the density of gas occupancy in the framework obtained from single-component and mixture simulations. The hindrance of transport of H2 molecule in the binary mixture is observable when the first snapshots of Fig. 2(e) and (f) are compared. It is worth noting that Fig. 2(e) and (f) can be considered as the concentration gradient maps and they are only observable from the NEMD simulations. Therefore, NEMD simulations are highly appropriate to represent the experimental membrane setup, especially dead-end cell module design where the feed stream is on the top of the membrane and the vacuum is at the bottom, leading to a pressure hence a concentration gradient.
Cu-BTC
Cu-BTC (also known as HKUST-1) has been extensively investigated in membrane-based gas separation applications due to its microporous structure and robustness. It contains intersecting three dimensional channels with an LCD of 13.2 Å surrounded by tetrahedral side pockets of 6.67 Å (PLD) in diameter, which are calculated using the Zeo++ algorithm.37 Several different fabrication methods such as in situ solvothermal,41,42 secondary growth,43,44 counter-diffusion,45 step-by-step growth seeding,46 and rapid thermal deposition47 were used in the synthesis of Cu-BTC membranes, and various types of support materials such as α-alumina,46,47 polymers,42,44 and others41,48 were used in the literature. This obviously led to the different membrane thicknesses as well as various membrane qualities in terms of continuity, compactness and quantity of defects. Therefore, single-component H2 and CH4 permeabilities of Cu-BTC membranes were reported to be in a very wide range, between 7.8 × 102 to 2.1 × 105 and 98–7.5 × 104 barrer, respectively, whereas H2/CH4 selectivities were reported to change from 1.4 to 8.4 in the literature.41–52 Additionally, six different binary mixture permeability measurements were reported in the range of 6.6 × 102 to 2.9 × 104 and 1.3 × 102 to 9.4 × 103 barrer for H2 and CH4, respectively for Cu-BTC membranes.46,48–52 Most of the fabricated Cu-BTC membranes were reported to display Knudsen diffusion with ideal H2/CH4 selectivity around 2.83.43,46,47,49,50 There were some exceptions where the ideal selectivities were reported to be between 4 and 8.45,48,51 The low selectivities were attributed to the interactions between the MOF and porous support and non-selective inter-crystalline diffusion through the grain boundaries in the literature.43 If the diffusion follows the nonselective intra-crystalline region through the grain boundaries instead of following the selective inter-crystalline pathway, high permeabilities and low selectivities are observed. Single-component and mixture permeabilities calculated from the GCMC + EMD simulations exceed the highest experimental permeability limit where the Knudsen diffusion was present. As shown in Fig. 3(a) and (b), H2 and CH4 permeabilities calculated from the NEMD simulations are close to the lower limit of the experimental data where Knudsen diffusion was absent and diffusion through the grain boundaries and cracks was minimized. Both ideal and mixture selectivities obtained from the NEMD simulations were within the range of experimental data and higher than the Knudsen selectivity. However, both ideal and mixture selectivities predicted from the GCMC + EMD simulations were lower than unity indicating that Cu-BTC is not an appropriate membrane to efficiently separate H2 from CH4 in contrast to the findings of experimental studies. This result suggests that permeability and selectivity predictions of the NEMD simulations are much closer to the experiments than the GCMC + EMD simulations for Cu-BTC membranes.
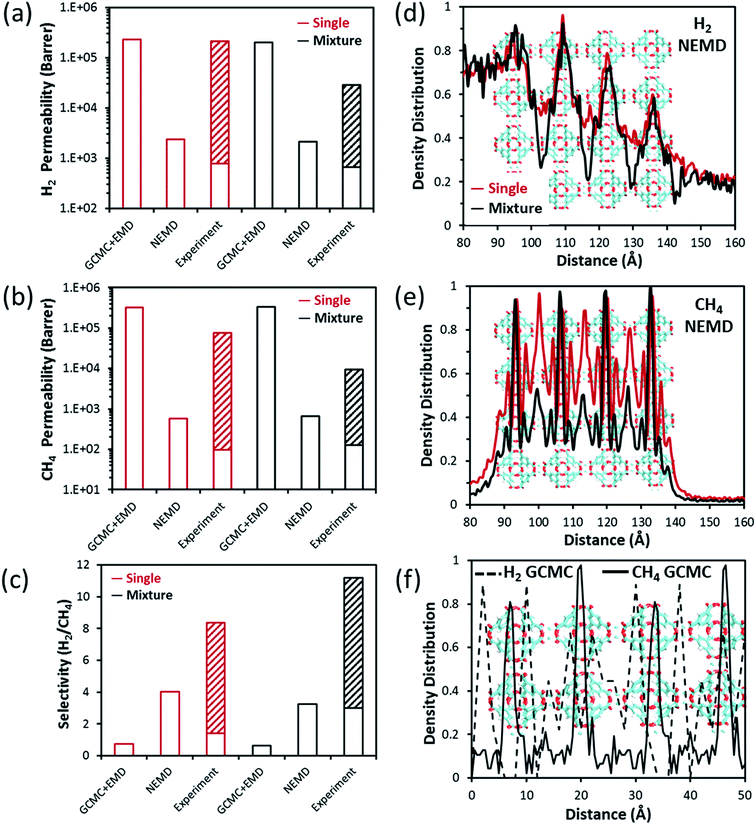 |
| Fig. 3 H2 and CH4 transport data through the Cu-BTC membrane. Comparison of (a) H2 permeability, (b) CH4 permeability, (c) H2/CH4 selectivity calculated from the GCMC + EMD simulations, NEMD simulations and those reported in experimental studies.41–52 Relative density distribution of (d) H2 and (e) CH4 in single-component gas (shown with red) and binary gas mixture (shown with black) calculations obtained from the NEMD simulation. (f) Relative density distribution of H2 (dashed black line) and CH4 (full black line) in a binary mixture predicted from the GCMC simulations. | |
Mixture selectivity was predicted to be slightly lower than the ideal selectivity by the NEMD simulations. This is probably due to the hindering of the H2 diffusion by the slow-diffusing CH4 molecules leading to a decrease in the transport rate of H2. Additionally, strongly adsorbed CH4 molecules inhibit H2 adsorption in the mixture resulting in a reduction in H2 permeability. Therefore, mixture selectivity predicted from the NEMD simulation is at the lower limit of the experimental selectivity and higher than the Knudsen diffusion selectivity. We also examined the competitive adsorption between H2 and CH4 in Cu-BTC. Adsorption selectivity of Cu-BTC is the highest among all MOFs investigated in this work as shown in Fig. S2(j).† In order to compare the gas adsorption preference of Cu-BTC, the density distribution profile of H2 and CH4 both under single-component and mixture conditions was obtained from the NEMD simulations and is shown in Fig. 3(d) and (e). When the single-component feed conditions are compared, it is obvious that H2 and CH4 share the same preferential adsorption sites in the MOF. There are more CH4 peaks compared to H2 in the density distribution diagrams indicating that CH4 is more strongly preferred than H2 in the framework. Fig. 3(d) shows that H2 preference of the MOF is not significantly affected by the presence of CH4 in the mixture case as can be seen from the small alteration in the peak intensities compared to the single-component case. However, CH4 preference of the MOF is dramatically influenced as shown in Fig. 3(e). A comparison of Fig. 3(e) and (f) shows that there is consistency between GCMC and NEMD for the most preferential adsorption sites of CH4 in the binary gas mixture. However, the population of CH4 in other regions is smaller in the GCMC simulations (Fig. 3(f)) compared to the NEMD simulations (Fig. 3(e)). All these results suggest that NEMD simulations clearly show the competition between gas species in the mixture compared to the GCMC + EMD simulations for the Cu-BTC membrane. We note that this type of comparison was not possible for MOF-5 since binary mixture permeability and selectivity data were not available in the literature for the MOF-5 membrane, to the best of our knowledge.
ZIF-8
ZIF-8 is a widely studied membrane due to its exceptionally high thermal and chemical stability. ZIF-8 has a sodalite topology, formed by bridging 2-methylimidazolate anions and zinc cations. It has narrow pore openings (∼3.4 Å) which can separate H2 from larger molecules. A special feature of ZIF-8 is that according to the inelastic neutron scattering analysis, it is inherently flexible.53 The above-mentioned challenges in the membrane formation for MOF-5 and Cu-BTC such as the presence of non-selective inter-crystalline and distorted regions in the intra-crystalline parts of MOFs are also valid for ZIF-8. Besides flat sheet membrane designs, tubular ZIF-8 membranes are proposed in order to fabricate membranes with low-defect quality but other challenges such as reproducibility and scalability were observed.54
Fig. 4(a)–(c) compare the transport properties of ZIF-8 calculated by the NEMD and GCMC + EMD simulations together with 20 different experimental data we collected from the literature.47,54–64 H2 and CH4 permeabilities of single-component (mixture) gas feeds through ZIF-8 vary in the range of 2.7 × 103 to 4.4 × 104 (3.2 × 103 to 8.4 × 103) barrer and 3.1 × 102 to 4.5 × 103 (3.3 × 102 to 7.8 × 102) barrer, respectively. The wide range in the single-component gas permeability results in varying ideal selectivities of ZIF-8 membranes from 4 to 14 whereas mixture selectivity only changes from ∼10 to 15. GCMC + EMD simulations predict higher H2 and CH4 permeabilities and lower H2/CH4 selectivity compared to the experimental measurements. The high CH4 permeability computed from the GCMC + EMD simulation led to reverse membrane selectivity for CH4 over H2. On the other hand, permeability and selectivity predictions of the NEMD simulations are at the lower and upper limits of the experimental measurements, respectively. Results of the NEMD simulations perfectly agree with the experimental data of 5 ZIF-8 membranes which were reported to have an improved microstructure quality.54,55,57,58,60 Bux et al.57 fabricated an oriented ZIF-8 membrane with a better intergrowth of the grains and reported higher selectivity (15) compared to the previously synthesized non-oriented one (11).55 Lower leak transport resulted in an increase in the separation performance of the membrane. Tao et al.59 reported that there were inter-crystalline defects but not large defects in the membrane resulting in an almost fixed ideal selectivity of 10.3 at 1–3 bars.
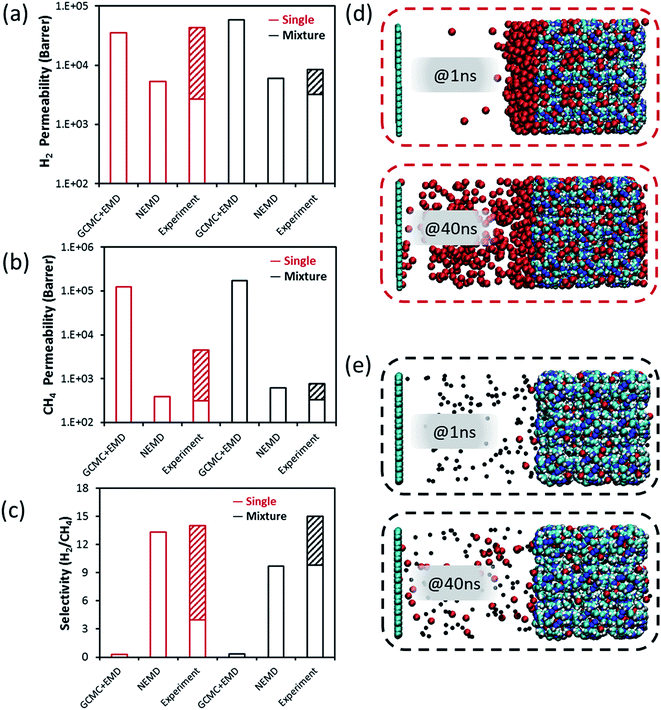 |
| Fig. 4 H2 and CH4 transport data through the ZIF-8 membrane. Comparison of (a) H2 permeability, (b) CH4 permeability, (c) H2/CH4 selectivity calculated from the GCMC + MD simulations, NEMD simulations and those reported in experimental studies.47,54–64 Snapshots of the feed side of the MOF membrane for (d) single-component CH4 permeation, (e) H2 and CH4 mixture permeation in the NEMD simulation at the 1st and 40th ns. Hydrogen, carbon, and zinc atoms of ZIF-8 in (d and e) are shown by the vdW representation with white, cyan, and dark blue colors, respectively. For clarification, H2 and CH4 molecules are represented with black and red colors, respectively in (d and e). | |
Both ideal and mixture H2/CH4 selectivities of ZIF-8 were reported to be higher than those of MOF-5 and Cu-BTC membranes in the experimental studies due to the narrower pore size of ZIF-8. We computed the PLD and LCD of ZIF-8 as 3.95 and 11.49 Å, respectively. The PLD of ZIF-8 is only slightly larger than the kinetic diameter of CH4 and it was reported that ZIF-8 has a flexible linker enabling the CH4 permeation.53 Therefore, we tethered the framework atoms rather than making the ZIF-8 fully flexible in the molecular simulations to save significant computational time. When we tethered the atoms of ZIF-8 around a specific value with the aim of introducing a slight flexibility to the ZIF-8 membrane, transport of gas molecules becomes faster. The severe differences in the single-component CH4 permeability calculated with and without tethering are illustrated by the movies provided in the ESI.† Although tethering was applied, the concentration polarization was obvious in the single-component CH4 transport as shown in Fig. 4(d). The CH4 layer in the feed side was so thick at the end of 1 ns due to the instantaneous external force applied in the beginning of the NEMD simulation and the thickness of the CH4 layer reduced at the end of the total simulation time, 40 ns. However, concentration polarization did not disappear with time in agreement with the literature where it was shown that concentration polarization on the feed side of the membrane can be observed in the highly permeable membranes that have pores narrower than the kinetic diameter of the gas molecules.60 On the other hand, the concentration polarization was not observed at the end of the simulation time for the binary gas mixture because of the accelerating effect of H2 (see Fig. 4(e)). As a result, NEMD simulations predicted both the single-component and mixture permeation of H2 and CH4 through the ZIF-8 membrane in very good agreement with the experiments.
MEFMEQ
We so far used the NEMD simulations to model MOF membranes for which experimental H2 and CH4 permeability data were available to compare and discuss the applicability of this method. We now turn to a MOF membrane which has not been experimentally fabricated and tested yet. MEFMEQ was identified as a promising MOF membrane in our previous high-throughput computational study21 which utilized GCMC + EMD simulations to screen large number of MOFs for membrane-based H2/CH4 separation. MEFMEQ has three dimensional interconnected pores with an asymmetry in each direction. It has an LCD of 5.24 Å and PLD of 4.26 Å, which were calculated using the Zeo++ algorithm. H2 permeability and H2/CH4 selectivity of MEFMEQ were computed as 8.1 × 103 barrer and 52.6, respectively for an equimolar H2/CH4 mixture using the GCMC + EMD simulations. This high membrane selectivity was attributed to the high diffusion selectivity for H2 over CH4 (527) surpassing the low adsorption selectivity for CH4 over H2 (10).21Fig. 5(a)–(c) represent the transport properties of MEFMEQ calculated using the NEMD simulations considering each surface of the unit cell in contact with the gas feed (Fig. S3†). Gas transport at each direction was calculated and the lowest permeability was observed in the y-direction (xz-surface) due to the pore size limitation. The averaged H2 and CH4 permeabilities over each direction in the single-component gas feeds (equimolar binary mixture) were computed as 4.8 × 103 (3 × 103) and 1.5 × 102 (2.2 × 102) barrer, respectively. Similar to our findings for MOF-5, Cu-BTC and ZIF-8 membranes, NEMD simulations estimated lower H2 and CH4 permeabilities through the MEFMEQ membrane for both single-component and mixture gas conditions compared to the GCMC + EMD simulations. For other three MOFs, gas adsorption values predicted from the GCMC and EMD simulations were found to be similar as shown in Fig. S2(a–c) and (e–g).† However, CH4 and H2 uptakes predicted from the EMD simulations were found to be significantly higher than those predicted from the GCMC simulations for MEFMEQ as shown in Fig. S2(d), (h) and (l).† Radial distribution functions (RDFs) can be used to identify these adsorption differences. Based on the location and intensity of the peaks in the RDF, preferential adsorption sites in the framework for an adsorbate can be defined. The RDFs of H2–Cu and H2–S obtained from EMD and NEMD simulations for single-component and binary mixture conditions are given in Fig. 5(d) to reveal the intensity difference in the density of H2 molecules. Due to the asymmetry of MEFMEQ, instead of the axial density distribution, radial density distributions of H2 and CH4 around four different possible preferential sites (sulphur, oxygen, nitrogen and copper atoms) were examined and results are given in Fig. S4 and S5.† The reason for using the data obtained from the EMD simulation to perform RDF analysis is that adsorbed gases in the GCMC simulation enable surface diffusion through adsorption to the most preferential sites in the framework. On the other hand, EMD simulation performed in this work represents the system of the NEMD simulation without an external force and the flux obtained from the NEMD simulation implicitly includes the adsorption data obtained from the EMD. The lower intensity observed in the EMD simulations at the most preferential adsorption sites of H2 is the evidence of the low population of H2 around those sites. Due to the high population and strong confinement of both gas molecules in NEMD simulations, gas diffusion coefficients and hence permeabilities were found to be lower in NEMD compared to the GCMC + EMD simulations. Finally, we compared the H2 permeability and H2/CH4 selectivity of MEFMEQ obtained from three different calculations: GCMC + EMD with 1 ns simulation time, GCMC + EMD with 200 ns simulation time and NEMD simulations in Fig. 5(e). Self-diffusion coefficients of gases were calculated within 1 ns in our previous work since the GCMC + EMD method was used to efficiently screen thousands of materials.21 We increased this time from 1 ns to 200 ns in this work using the same initial concentrations defined from the GCMC simulations to capture the long time diffusive behavior of gases. As a result, CH4 permeability, H2 permeability and H2/CH4 selectivity of MEFMEQ were changed from 155 to 440 barrer, from 8.1 × 103 to 9.9 × 103 barrer and from 52.6 to 22.6. Although the NEMD simulations predicted lower gas permeabilities and selectivities than the GCMC + EMD simulations, these permeability and selectivity data were still high enough to locate MEFMEQ above the upper bound as shown in Fig. 5(e). This result suggests that GCMC + EMD simulations can be used to quickly screen the MOFs in order to identify the promising membrane candidates that are above the upper bound and more realistic and computationally demanding NEMD simulations can be then performed only for the promising membrane candidates.
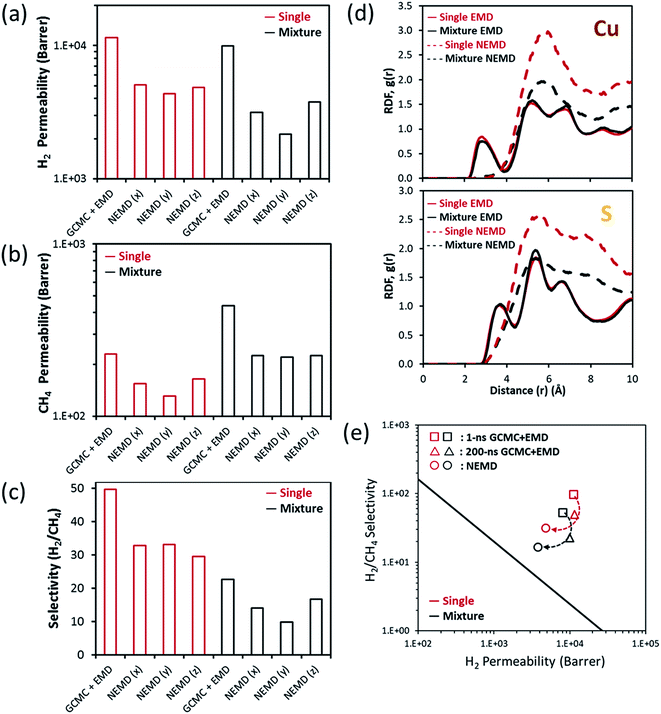 |
| Fig. 5 H2 and CH4 transport data through the MEFMEQ membrane. Comparison of (a) H2 and (b) CH4 permeability, (c) H2/CH4 selectivity calculated from the NEMD simulations at each direction and GCMC + MD simulations. (d) Radial density distribution of H2 around specific atoms (Cu and S) selected in the MOF in the single-component and binary mixture calculations obtained from the NEMD and EMD simulations. (e) Performance of MEFMEQ together with Robeson's upper bound. | |
Separation performances of MOF membranes
Finally, we showed the simulation results, both from the GCMC + EMD and NEMD, together with the available experimental data for the four MOF membranes that we examined in this work in Fig. 6. GCMC + EMD simulations suggested that MOF-5 is CH4 selective over H2 in contrast to the experimental data whereas NEMD simulations showed that this membrane is H2 selective. While H2/CH4 selectivity calculated from the NEMD simulations agreed well with experiments, H2 permeability was underestimated compared to the experiments. The high experimental gas permeabilities of MOF-5 were attributed to the presence of defects and non-selective inter-crystalline regions in the fabricated membranes reported in the literature.38 If well-packed, perfect MOF membranes can be fabricated, measurements on these membranes will be probably much closer to the permeability predictions of the NEMD simulations where the ideal MOF structure is simulated as a membrane. For the Cu-BTC membrane, the GCMC + EMD method slightly overpredicted the permeability and underpredicted the selectivity. Both the selectivity and permeability predictions of the NEMD simulations were found to be within the experimental range. However, it is important to note that the experimental permeability data are very wide in the literature due to the differences in the fabrication of Cu-BTC membranes. The experimental separation performance of ZIF-8 varies in a narrow region compared to MOF-5 and Cu-BTC membranes. There was an excellent agreement between the experiments and NEMD simulations both for the permeability and selectivity of the ZIF-8 membrane. Finally, GCMC + EMD and NEMD simulations showed that MEFMEQ is a promising membrane surpassing Robeson's upper bound for H2/CH4 separation although NEMD simulations could not reach the high permeability and selectivity values predicted by the GCMC + EMD simulations.
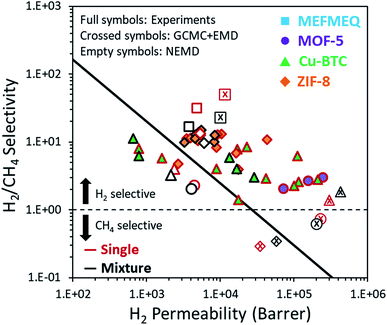 |
| Fig. 6 H2/CH4 separation performances of MOF membranes studied in this work. Full, crossed and empty symbols correspond to the properties measured experimentally, predicted from GCMC + EMD simulations and NEMD simulations, respectively. Red and black borders in the symbols correspond to the single-component and mixture feed gas, respectively. The black solid line represents Robeson's upper bound for H2/CH4 separation performance of the polymeric membranes and the dashed line shows the gas preference of the membrane. | |
We finally note that we compared the results of NEMD simulations with the predictions of GCMC + EMD approach that we used in our previous work. MOFs were not treated as flexible in our previous EMD study and in order to be consistent within the comparison, we did not treat MOF structures as completely flexible in the NEMD simulations of this work but introduced a slight flexibility by tethering MOF atoms as discussed above. In some recent studies, flexible force fields were used in EMD simulations and results for diffusion of gas molecules in MOFs were found to be in good agreement with the experimental data.65–69 Flexible EMD simulations are specifically useful to examine gas diffusion in narrow-pored MOFs but they are very computationally demanding since they require development of flexible force fields from detailed quantum chemistry calculations for the accurate description of intra-molecular interactions. We finally note that the NEMD approach accounts for the interfacial barrier near the pore mouth of the membrane which is evidently neglected in the EMD approach regardless of the framework representation (either fixed or flexible) and hence it can be accepted as more realistic than the other approaches to mimic a membrane-based gas separation.
Conclusions
We used NEMD simulations to examine single-component and binary mixture transport of H2 and CH4 through four different MOF membranes. Similar to experimental measurements, steady-state gas fluxes were computed during the NEMD simulations and used to calculate gas permeabilities and selectivities of MOF membranes. There is a reasonable agreement between the gas permeabilities estimated by the NEMD simulations and measured by the experiments although measurements have wide variations even for the same type of MOF membrane. Permeability predictions of the GCMC + EMD simulations were found to be at the upper limit of the experimental data or much higher than that whereas permeability predictions of the NEMD simulations were generally within the experimentally measured values. Moreover, using the NEMD approach allowed us to directly observe the mass transfer resistance on the pore mouth of the MOF membrane, which causes concentration polarization. Finally, it is important to note that NEMD simulations require significantly longer simulation times than GCMC + EMD simulations. In high-throughput screening studies, simulation time for one MOF structure is in the orders of hours for the GCMC + EMD approach, whereas approximately several days are required for the NEMD simulations even with the same computational resources. Additionally, multiple NEMD simulations using different external fields should be applied in order to ensure the linear relationship between the pressure drop and the applied external force. Unit cell representation of a single-MOF-crystal obtained from the crystallographic information file is directly used in the GCMC + EMD simulations whereas accurate representation of the MOF membrane system, including the barrier and gas bath, is required in the NEMD simulations as shown in Fig. 1. Overall, it can be concluded that GCMC + EMD simulations which offer a quick way of predicting gas permeability and selectivity of a single MOF crystal can be used to make an initial estimate about the membrane-based gas separation performances of MOFs and more detailed NEMD simulations can be then performed for the most promising MOF membranes to unlock their actual separation performances before directing experimental efforts to membrane fabrication. Finally, it is important to note that fabrication of a membrane from new materials such as MOFs is a challenging process. Several issues that hinder the achievement of actual separation performances of MOFs such as the adhesion of the MOF membrane to the support, poor crystal intergrowth, large crystal size leading to non-continuous membranes and poor reproducibility have been reported in the literature.70 Therefore, performing molecular simulations such as NEMD prior to conducting extensive experiments will be highly useful to direct the efforts, resources and time to the best membrane candidates.
Conflicts of interest
There are no conflicts to declare.
Acknowledgements
S. K. acknowledges ERC-2017-Starting Grant. This research has received funding from the European Research Council (ERC) under the European Union's Horizon 2020 research and innovation programme (ERC-2017-Starting Grant, grant agreement no. 756489-COSMOS).
References
- H. Furukawa, K. E. Cordova, M. O'Keeffe and O. M. Yaghi, The Chemistry and Applications of Metal–Organic Frameworks, Science, 2013, 341(6149), 1230444 CrossRef PubMed.
- C. Wang, D. Liu and W. Lin, Metal–Organic Frameworks as a Tunable Platform for Designing Functional Molecular Materials, J. Am. Chem. Soc., 2013, 135(36), 13222–13234 CrossRef CAS PubMed.
-
D. Frenkel and B. Smit, Understanding Molecular Simulations: From Algorithms to Applications, Academic Press, 2002 Search PubMed.
- H. Frentrup, C. Avendaño, M. Horsch, A. Salih and E. A. Müller, Transport Diffusivities of Fluids in Nanopores by Non-Equilibrium Molecular Dynamics Simulation, Mol. Simul., 2012, 38(7), 540–553 CrossRef CAS.
- H. Frentrup, K. E. Hart, C. M. Colina and E. A. Müller,
In Silico Determination of Gas Permeabilities by Non-Equilibrium Molecular Dynamics: CO2 and He through PIM-1, Membranes, 2015, 5(1), 99–119 CrossRef CAS PubMed.
- G. Arya, H.-C. Chang and E. J. Maginn, A Critical Comparison of Equilibrium, Non-Equilibrium and Boundary-Driven Molecular Dynamics Techniques for Studying Transport in Microporous Materials, J. Chem. Phys., 2001, 115(17), 8112–8124 CrossRef CAS.
- M. Fasano, T. Humplik, A. Bevilacqua, M. Tsapatsis, E. Chiavazzo, E. N. Wang and P. Asinari, Interplay between Hydrophilicity and Surface Barriers on Water Transport in Zeolite Membranes, Nat. Commun., 2016, 7, 12762 CrossRef CAS PubMed.
- D. A. Newsome and D. S. Sholl, Predictive Assessment of Surface Resistances in Zeolite Membranes Using Atomically Detailed Models, J. Phys. Chem. B, 2005, 109(15), 7237–7244 CrossRef CAS PubMed.
- E. J. Maginn, A. T. Bell and D. N. Theodorou, Transport Diffusivity of Methane in Silicalite from Equilibrium and Nonequilibrium Simulations, J. Phys. Chem., 1993, 97(16), 4173–4181 CrossRef CAS.
- S. Chempath, R. Krishna and R. Q. Snurr, Nonequilibrium Molecular Dynamics Simulations of Diffusion of Binary Mixtures Containing Short n-Alkanes in Faujasite, J. Phys. Chem. B, 2004, 108(35), 13481–13491 CrossRef CAS.
- S. Turgman-Cohen, J. C. Araque, E. M. Hoek and F. A. Escobedo, Molecular Dynamics of Equilibrium and Pressure-Driven Transport Properties of Water through LTA-Type Zeolites, Langmuir, 2013, 29(40), 12389–12399 CrossRef CAS.
- K. Falk, F. Sedlmeier, L. Joly, R. R. Netz and L. Bocquet, Molecular Origin of Fast Water Transport in Carbon Nanotube Membranes: Superlubricity Versus Curvature Dependent Friction, Nano Lett., 2010, 10(10), 4067–4073 CrossRef CAS.
- D. Cohen-Tanugi and J. C. Grossman, Water Desalination across Nanoporous Graphene, Nano Lett., 2012, 12(7), 3602–3608 CrossRef CAS PubMed.
- L.-C. Lin and J. C. Grossman, Atomistic Understandings of Reduced Graphene Oxide as an Ultrathin-Film Nanoporous Membrane for Separations, Nat. Commun., 2015, 6, 8335 CrossRef CAS.
- F. Zhu, E. Tajkhorshid and K. Schulten, Theory and Simulation of Water Permeation in Aquaporin-1, Biophys. J., 2004, 86(1), 50–57 CrossRef CAS.
- J. J. Williams, N. A. Seaton and T. Duren, Influence of Surface Groups on the Diffusion of Gases in MCM-41: A Molecular Dynamics Study, J. Phys. Chem. C, 2011, 115(21), 10651–10660 CrossRef CAS.
- H. Babaei, A. J. McGaughey and C. E. Wilmer, Transient Mass and Thermal Transport During Methane Adsorption into the Metal–Organic Framework HKUST-1, ACS Appl. Mater. Interfaces, 2018, 10(3), 2400–2406 CrossRef CAS PubMed.
- A.-M. Banu, D. Friedrich, S. Brandani and T. Düren, A Multiscale Study of MOFs as Adsorbents in H2 PSA Purification, Ind. Eng. Chem. Res., 2013, 52(29), 9946–9957 CrossRef CAS.
- K. M. Gupta, K. Zhang and J. Jiang, Glucose Recovery from Aqueous Solutions by Adsorption in Metal–Organic Framework MIL-101: A Molecular Simulation Study, Sci. Rep., 2015, 5, 12821 CrossRef CAS PubMed.
- A. Ozcan, C. Perego, M. Salvalaglio, M. Parrinello and O. Yazaydin, Concentration Gradient Driven Molecular Dynamics: A New Method for Simulations of Membrane Permeation and Separation, Chem. Sci., 2017, 8(5), 3858–3865 RSC.
- C. Altintas, G. Avci, H. Daglar, E. Gulcay, I. Erucar and S. Keskin, Computer Simulations of 4240 MOF Membranes for H2/CH4 Separations: Insights into Structure–Performance Relations, J. Mater. Chem. A, 2018, 6(14), 5836–5847 RSC.
- E. Adatoz, A. K. Avci and S. Keskin, Opportunities and Challenges of MOF-Based Membranes in Gas Separations, Sep. Purif. Technol., 2015, 152, 207–237 CrossRef CAS.
- P. Z. Moghadam, T. Islamoglu, S. Goswami, J. Exley, M. Fantham, C. F. Kaminski, R. Q. Snurr, O. K. Farha and D. Fairen-Jimenez, Computer-Aided Discovery of a Metal–Organic Framework with Superior Oxygen Uptake, Nat. Commun., 2018, 9(1), 1378 CrossRef PubMed.
- Z. Qiao, C. Peng, J. Zhou and J. Jiang, High-Throughput Computational Screening of 137
953 Metal–Organic Frameworks for Membrane Separation of a CO2/N2/CH4 Mixture, J. Mater. Chem. A, 2016, 4(41), 15904–15912 RSC.
- T. Watanabe and D. S. Sholl, Accelerating Applications of Metal–Organic Frameworks for Gas Adsorption and Separation by Computational Screening of Materials, Langmuir, 2012, 28(40), 14114–14128 CrossRef CAS PubMed.
- H. Daglar and S. Keskin, Computational Screening of Metal–Organic Frameworks for Membrane-Based CO2/N2/H2O Separations: Best Materials for Flue Gas Separation, J. Phys. Chem. C, 2018, 122(30), 17347–17357 CrossRef CAS.
- S. Plimpton, Fast Parallel Algorithms for Short-Range Molecular Dynamics, J. Comput. Phys., 1995, 117(1), 1–19 CrossRef CAS.
- W. Humphrey, A. Dalke and K. Schulten, VMD: Visual Molecular Dynamics, J. Mol. Graphics, 1996, 14(1), 33–38 CrossRef CAS PubMed.
- L. Martínez, R. Andrade, E. G. Birgin and J. M. Martínez, Packmol: A Package for Building Initial Configurations for Molecular Dynamics Simulations, J. Comput. Chem., 2009, 30(13), 2157–2164 CrossRef PubMed.
- V. Buch, Path Integral Simulations of Mixed Para-D2 and Ortho-D2 Clusters: The Orientational Effects, J. Chem. Phys., 1994, 100(10), 7610–7629 CrossRef CAS.
- M. G. Martin and J. I. Siepmann, Transferable Potentials for Phase Equilibria. 1. United-Atom Description of n-Alkanes, J. Phys. Chem. B, 1998, 102(14), 2569–2577 CrossRef CAS.
- A. K. Rappé, C. J. Casewit, K. Colwell, W. A. Goddard III and W. Skiff, UFF, a Full Periodic Table Force Field for Molecular Mechanics and Molecular Dynamics Simulations, J. Am. Chem. Soc., 1992, 114(25), 10024–10035 CrossRef.
- K. N. Han, S. Bernardi, L. Wang and D. J. Searles, Water Diffusion in Zeolite Membranes: Molecular Dynamics Studies on Effects of Water Loading and Thermostat, J. Membr. Sci., 2015, 495, 322–333 CrossRef CAS.
-
T. Kovács, S. Papp and T. Kristóf, Membrane Separation Study for Methane-Hydrogen Gas Mixtures by Molecular Simulations, arXiv preprint arXiv:1706.07250, 2017.
- W. G. Hoover, Canonical Dynamics: Equilibrium Phase-Space Distributions, Phys. Rev. A: At., Mol., Opt. Phys., 1985, 31(3), 1695 CrossRef.
- S. Nosé, A Unified Formulation of the Constant Temperature Molecular Dynamics Methods, J. Chem. Phys., 1984, 81(1), 511–519 CrossRef.
- T. F. Willems, C. H. Rycroft, M. Kazi, J. C. Meza and M. Haranczyk, Algorithms and Tools for High-Throughput Geometry-Based Analysis of Crystalline Porous Materials, Microporous Mesoporous Mater., 2012, 149(1), 134–141 CrossRef CAS.
- Y. Liu, Z. Ng, E. A. Khan, H.-K. Jeong, C.-B. Ching and Z. Lai, Synthesis of Continuous MOF-5 Membranes on Porous α-Alumina Substrates, Microporous Mesoporous Mater., 2009, 118(1), 296–301 CrossRef CAS.
- Y. Yoo, Z. Lai and H.-K. Jeong, Fabrication of MOF-5 Membranes Using Microwave-Induced Rapid Seeding and Solvothermal Secondary Growth, Microporous Mesoporous Mater., 2009, 123(1–3), 100–106 CrossRef CAS.
- Z. Zhao, X. Ma, Z. Li and Y. Lin, Synthesis, Characterization and Gas Transport Properties of MOF-5 Membranes, J. Membr. Sci., 2011, 382(1–2), 82–90 CrossRef CAS.
- F. Cao, C. Zhang, Y. Xiao, H. Huang, W. Zhang, D. Liu, C. Zhong, Q. Yang, Z. Yang and X. Lu, Helium Recovery by a Cu-BTC Metal–Organic-Framework Membrane, Ind. Eng. Chem. Res., 2012, 51(34), 11274–11278 CrossRef CAS.
- D. Nagaraju, D. G. Bhagat, R. Banerjee and U. K. Kharul, In Situ Growth of Metal–Organic Frameworks on a Porous Ultrafiltration Membrane for Gas Separation, J. Mater. Chem. A, 2013, 1(31), 8828–8835 RSC.
- V. V. Guerrero, Y. Yoo, M. C. McCarthy and H.-K. Jeong, HKUST-1 Membranes on Porous Supports Using Secondary Growth, J. Mater. Chem., 2010, 20(19), 3938–3943 RSC.
- W. Li, Y. Zhang, C. Zhang, Q. Meng, Z. Xu, P. Su, Q. Li, C. Shen, Z. Fan and L. Qin, Transformation of Metal–Organic Frameworks for Molecular Sieving Membranes, Nat. Commun., 2016, 7, 11315 CrossRef CAS PubMed.
- N. Hara, M. Yoshimune, H. Negishi, K. Haraya, S. Hara and T. Yamaguchi, Metal–Organic Framework Membranes with Layered Structure Prepared within the Porous Support, RSC Adv., 2013, 3(34), 14233–14236 RSC.
- J. Nan, X. Dong, W. Wang, W. Jin and N. Xu, Step-by-Step Seeding Procedure for Preparing HKUST-1 Membrane on Porous α-Alumina Support, Langmuir, 2011, 27(8), 4309–4312 CrossRef CAS PubMed.
- M. N. Shah, M. A. Gonzalez, M. C. McCarthy and H.-K. Jeong, An Unconventional Rapid Synthesis of High Performance Metal–Organic Framework Membranes, Langmuir, 2013, 29(25), 7896–7902 CrossRef CAS PubMed.
- H. Guo, G. Zhu, I. J. Hewitt and S. Qiu, “Twin Copper Source” Growth of Metal–Organic Framework Membrane: Cu3(BTC)2 with High Permeability and Selectivity for Recycling H2, J. Am. Chem. Soc., 2009, 131(5), 1646–1647 CrossRef CAS PubMed.
- Y. Mao, W. Cao, J. Li, Y. Liu, Y. Ying, L. Sun and X. Peng, Enhanced Gas Separation through Well-Intergrown MOF Membranes: Seed Morphology and Crystal Growth Effects, J. Mater. Chem. A, 2013, 1(38), 11711–11716 RSC.
- Y. Mao, H. Huang, W. Cao, J. Li, L. Sun, X. Jin and X. Peng, Room Temperature Synthesis of Free-Standing HKUST-1 Membranes from Copper Hydroxide Nanostrands for Gas Separation, Chem. Commun., 2013, 49(50), 5666–5668 RSC.
- T. Ben, C. Lu, C. Pei, S. Xu and S. Qiu, Polymer-Supported and Free-Standing Metal–Organic Framework Membrane, Chem.–Eur. J., 2012, 18(33), 10250–10253 CrossRef CAS.
- S. Zhou, X. Zou, F. Sun, F. Zhang, S. Fan, H. Zhao, T. Schiestel and G. Zhu, Challenging Fabrication of Hollow Ceramic Fiber Supported Cu3(BTC)2 Membrane for Hydrogen Separation, J. Mater. Chem., 2012, 22(20), 10322–10328 RSC.
- S. A. Moggach, T. D. Bennett and A. K. Cheetham, The Effect of Pressure on ZIF-8: Increasing Pore Size with Pressure and the Formation of a High-Pressure Phase at 1.47 GPa, Angew. Chem., Int. Ed., 2009, 48(38), 7087–7089 CrossRef CAS PubMed.
- X. Zhang, Y. Liu, L. Kong, H. Liu, J. Qiu, W. Han, L.-T. Weng, K. L. Yeung and W. Zhu, A Simple and Scalable Method for Preparing Low-Defect ZIF-8 Tubular Membranes, J. Mater. Chem. A, 2013, 1(36), 10635–10638 RSC.
- H. Bux, F. Liang, Y. Li, J. Cravillon, M. Wiebcke and J. R. Caro, Zeolitic Imidazolate Framework Membrane with Molecular Sieving Properties by Microwave-Assisted Solvothermal Synthesis, J. Am. Chem. Soc., 2009, 131(44), 16000–16001 CrossRef CAS PubMed.
- Y. Liu, N. Wang, L. Diestel, F. Steinbach and J. Caro, MOF Membrane Synthesis in the Confined Space of a Vertically Aligned LDH Network, Chem. Commun., 2014, 50(32), 4225–4227 RSC.
- H. Bux, A. Feldhoff, J. Cravillon, M. Wiebcke, Y.-S. Li and J. Caro, Oriented Zeolitic Imidazolate Framework-8 Membrane with Sharp H2/C3H8 Molecular Sieve Separation, Chem. Mater., 2011, 23(8), 2262–2269 CrossRef CAS.
- D. Liu, X. Ma, H. Xi and Y. Lin, Gas Transport Properties and Propylene/Propane Separation Characteristics of ZIF-8 Membranes, J. Membr. Sci., 2014, 451, 85–93 CrossRef CAS.
- K. Tao, L. Cao, Y. Lin, C. Kong and L. Chen, A Hollow Ceramic Fiber Supported ZIF-8 Membrane with Enhanced Gas Separation Performance Prepared by Hot Dip-Coating Seeding, J. Mater. Chem. A, 2013, 1(42), 13046–13049 RSC.
- Y. Pan, B. Wang and Z. Lai, Synthesis of Ceramic Hollow Fiber Supported Zeolitic Imidazolate Framework-8 (ZIF-8) Membranes with High Hydrogen Permeability, J. Membr. Sci., 2012, 421, 292–298 CrossRef.
- G. Xu, J. Yao, K. Wang, L. He, P. A. Webley, C.-S. Chen and H. Wang, Preparation of ZIF-8 Membranes Supported on Ceramic Hollow Fibers from a Concentrated Synthesis Gel, J. Membr. Sci., 2011, 385, 187–193 CrossRef.
- M. C. McCarthy, V. Varela-Guerrero, G. V. Barnett and H.-K. Jeong, Synthesis of Zeolitic Imidazolate Framework Films and Membranes with Controlled Microstructures, Langmuir, 2010, 26(18), 14636–14641 CrossRef CAS PubMed.
- Y. Pan and Z. Lai, Sharp Separation of C2/C3 Hydrocarbon Mixtures by Zeolitic Imidazolate Framework-8 (ZIF-8) Membranes Synthesized in Aqueous Solutions, Chem. Commun., 2011, 47(37), 10275–10277 RSC.
- Y. Liu, N. Wang, J. H. Pan, F. Steinbach and J. R. Caro, In Situ Synthesis of MOF Membranes on ZnAl–Co3 LDH Buffer Layer-Modified Substrates, J. Am. Chem. Soc., 2014, 136(41), 14353–14356 CrossRef CAS PubMed.
- J. A. Greathouse, T. L. Kinnibrugh and M. D. Allendorf, Adsorption and Separation of Noble Gases by IRMOF-1: Grand Canonical Monte Carlo Simulations, Ind. Eng. Chem. Res., 2009, 48(7), 3425–3431 CrossRef CAS.
- L. Zhang, G. Wu and J. Jiang, Adsorption and Diffusion of CO2 and CH4 in Zeolitic Imidazolate Framework-8: Effect of Structural Flexibility, J. Phys. Chem. C, 2014, 118(17), 8788–8794 CrossRef CAS.
- S. Amirjalayer, M. Tafipolsky and R. Schmid, Molecular Dynamics Simulation of Benzene Diffusion in MOF-5: Importance of Lattice Dynamics, Angew. Chem., Int. Ed., 2007, 46(3), 463–466 CrossRef CAS PubMed.
- I. Erucar and S. Keskin, Computational Modeling of Bio-MOFs for CO2/CH4 Separations, Chem. Eng. Sci., 2015, 130, 120–128 CrossRef CAS.
- N. Ramsahye, J. Gao, H. Jobic, P. Llewellyn, Q. Yang, A. Wiersum, M. Koza, V. Guillerm, C. Serre, C. Zhong and G. Maurin, Adsorption and Diffusion of Light Hydrocarbons in UIO-66(Zr): A Combination of Experimental and Modeling Tools, J. Phys. Chem. C, 2014, 118(47), 27470–27482 CrossRef CAS.
- S. R. Venna and M. A. Carreon, Metal–Organic Framework Membranes for Carbon Dioxide Separation, Chem. Eng. Sci., 2015, 124, 3–19 CrossRef CAS.
Footnote |
† Electronic supplementary information (ESI) available: Experimental membrane data reported for MOF-5, Cu-BTC and ZIF-8. Comparison of CH4 and H2 uptakes and adsorption selectivities of MOFs computed by EMD and GCMC together with experimental data. Unit cell representation of MEFMEQ. RDFs between adsorbents (namely CH4 and H2) and four different atom types (namely Cu, N, O, and S) existing in MEFMEQ. See DOI: 10.1039/c8ta10167a |
|
This journal is © The Royal Society of Chemistry 2019 |
Click here to see how this site uses Cookies. View our privacy policy here.