DOI:
10.1039/C8SC04320E
(Edge Article)
Chem. Sci., 2019,
10, 4246-4258
New insights into spectral histopathology: infrared-based scoring of tumour aggressiveness of squamous cell lung carcinomas
Received
28th September 2018
, Accepted 1st March 2019
First published on 5th March 2019
Abstract
Spectral histopathology, based on infrared interrogation of tissue sections, proved a promising tool for helping pathologists in characterizing histological structures in a quantitative and automatic manner. In cancer diagnosis, the use of chemometric methods permits establishing numerical models able to detect cancer cells and to characterize their tissular environment. In this study, we focused on exploiting multivariate infrared data to score the tumour aggressiveness in preneoplastic lesions and squamous cell lung carcinomas. These lesions present a wide range of aggressive phenotypes; it is also possible to encounter cases with various degrees of aggressiveness within the same lesion. Implementing an infrared-based approach for a more precise histological determination of the tumour aggressiveness should arouse interest among pathologists with direct benefits for patient care. In this study, the methodology was developed from a set of samples including all degrees of tumour aggressiveness and by constructing a chain of data processing steps for an automated analysis of tissues currently manipulated in routine histopathology.
Introduction
Lung Carcinoma (LC), including trachea and bronchus carcinomas, is the most commonly diagnosed cancer with 1.82 million of new cases worldwide (12.9% of all diagnosed cancer cases) and is also the deadliest. Indeed, LC represents near a fifth (19.4%) of cancer deaths with 1.69 million of deaths (number estimated in 2012).1 Consequently, it means that near 93% of LC diagnosed people succumb to this disease. The research community against cancer reports that LC is an aggressive and heterogeneous disease divided into two main types: small and non-small squamous cell carcinomas. Non-small cell lung cancer (NSCLC) represents around 80% of diagnosed LC.2,3 In addition, squamous cell lung carcinoma (SCC), which is strongly associated with smoking, accounts for 35% of NSCLC. They develop from large and medium sized bronchi through a process of squamous metaplasia. Control and prevention of known carcinogens (such as tobacco, asbestos or radon gas) have permitted the reduction of new cases of LC. For example, within the European Community, a reduction of LC new cases close to 20% was noticed between years 1988 and 2008.4–7
Nevertheless, the survival rate remains low despite advances in surgery, radiotherapy, chemotherapy and especially in new targeted therapies such as checkpoint inhibitors (anti-CTLA4 and anti-PD1/PDL1).8 This is principally due to the late diagnosis of advanced lesions. Actually, checkpoint inhibitor status is studied in view to adapt targeted therapies but there is no more adaptive therapeutic strategy. The identification of new prognostic and predictive tools is therefore a necessity for better management of patients.
Clinical staging and identification of the precise histological type are fundamental to establishing an appropriate therapeutic strategy. The examination of the tissue morphology after Haematoxylin Eosin (HE) staining is the current gold standard to determine the lesion extension, which is one of the main prognostic indicators.9,10 Despite the pathologist expertise, there exists a wide range of opinion regarding the choice of the treatment and the expected impact of this treatment all the more that pathologist conclusions can be intrinsically subjective leading to consensus default.11–14 The participation of physicians to clinical boards appears also not reliable in regards to survival of LC-advanced stage patients.15 When staging, subtyping or any prognosis indices are not clearly displayed, pathologists can have recourse to extensive analyses such as immunohistochemical labelling.16–18 These approaches represent interesting complementary tools for the routine diagnosis of LC by identifying different histological types and accessing certain prognostic criteria. This analysis, realized on resection tissues or cell biopsies, aims at categorizing patients in order to define appropriate personalized treatment, as well as identifying tumours with high risk of recurrence and fatal outcomes. The principal immunohistochemistry panel for the diagnosis of NSCLC includes the following markers: CK7, CK20, TTF-1, p40, Napsin A, chromogranin, synaptophysin, and CD56. Moreover, the complexity and diversity of NSCLC genetic mutations and rearrangements open the way to targeted therapy.19 In another way, the immunologic environment and its potential stimulation represents a new approach for the prognosis and the treatment of NSCLC. Nevertheless, besides TNM staging, there are few histologic markers of aggressiveness of tumoral lesions. Particularly, preneoplastic lesions are difficult to evaluate in terms of aggressiveness. So, to answer to this question, researchers and pathologists try new, specific and reliable immunomarkers. These experimental procedures are usually destructive, expensive, and time-consuming and give poorly relevant results.20 Furthermore, the development of various specific/target markers multiplies the required number of tissue sections to determine the most appropriate therapeutic strategy.21,22
In order to improve objectivity, the potential of new analytical techniques was investigated. For example, optical coherence tomography, imprint cytology and ultra-sonography are non-invasive and fast imaging techniques that could be of interest to improve prognosis. Images or videos are collected very quickly but molecular information obtained is rather poor.23–26 In contrary, spectral techniques such as reflectance/fluorescence, elastic light scattering, and Raman or Fourier-Transform-infrared (FT-IR) spectroscopy present the potential to provide molecular information specific of the sample status, in a label-free manner. In addition, these optical methods lead to objective and reproducible data. The extracted molecular information allows identifying cellular biochemical components, to obtain the cell morphology and tissue architecture, in various tissular specimens including lung cancers.27–32
It was demonstrated that FT-IR spectroscopy coupled with microimaging mapping and statistical processing of spectral data was usefully employed in many characterization studies of biological tissues.33–39 These demonstrations led to defining the concept of spectral histopathology (SHP).40–42 In addition, improvements in instrumental devices and developments in advanced chemometrics for exploiting multivariate IR spectra permit us to consider the deployment of SHP in pathology departments.42–44
The aim of the present work is to develop an IR micro-imaging procedure to score in an automatic and reproducible manner the aggressiveness phenotype of progressive bronchial lesions from precancerous dysplastic lesions and in situ carcinoma to invasive SCC of the lung. This objective requires in a first step to recover precisely the tissue architecture for highlighting the epithelial cellular component which is the structure of interest in the present biomedical issue. Secondly, to construct a spectral scale of aggressiveness, we used as a reference the histopathological characterization of these epithelial cells actually performed by the collaborating pathologists. The biopsy samples, embedded in paraffin, were first mathematically dewaxed by means of Extended Multiplicative Signal Correction (EMSC).45,46 Then all IR images were processed together in order to build an ordered spectral data bank. This bank of spectral images was split into two sets: a calibration set for constructing the supervised models and a test set (also called the external validation set) for the evaluation of the model performances on independent images. Discriminative classification and quantitative models were developed on the basis of Partial Least Squares algorithms.47
Results
In order to implement the aggressiveness spectral scale, several processing steps must be carried out sequentially (Table 1). First, the processing performed separately for each spectral image includes a pre-treatment for numerical dewaxing and an unsupervised clustering to highlight the tissue structures of interest. Secondly, the treatment performed on the whole set of spectral images corresponds to the formatting of an ordered matrix of normalized data originating from all the spectral images, and the construction of PLS-DA and PLS models to automatically select and score the pixels of interest on the basis of their infrared signature.
Unsupervised recovering tissue structures on the basis of their spectral signatures
Thirty four paraffin-embedded biopsies were analysed using IR micro-imaging; the spectral images recorded were first mathematically pre-treated by smoothing and numerical dewaxing by means of a first EMSC. More precisely, this first EMSC allowed us to neutralize the paraffin spectral variability and to carry out a quality test for removing outlier spectra. Thus, pixels with only paraffin or CaF2 signals were eliminated from the data. The corresponding pixels were displayed in white on the spectral images presented thereafter.
Next, the ASK algorithm was employed on each image separately from the others, to highlight the tissue structures in a totally unsupervised way by determining automatically the optimal number of clusters. As shown in Fig. 2, the ASK algorithm permitted forming color-coded images. The mean number of sub-clusters obtained for each image is equal to 16. Since the spectral images were processed separately from each other, there is no correspondence of colours between the different images. By comparing these clustered spectral images with the conventional histology of adjacent sections, the majority of spectral sub-clusters can be labelled. Particular attention was paid to the assignment of the epithelium structure due to the importance of the associated IR spectra for the subsequent construction of the aggressiveness scale.
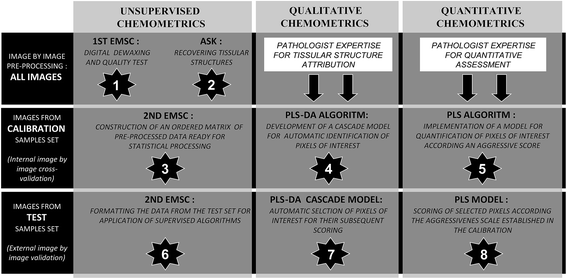 |
| Fig. 1 Schematic processing organigram used to process infrared multivariate data. The chemometric chain follows 8 steps applied to different sets of images. The 3 black lines concern the considered sample set (all, calibration or external validation samples). EMSC (Extended Multiplicative Signal Correction), ASK (Automatic Serial K-means), PLS-DA (Partial Least Squares Discriminant Analysis) and PLS (Partial Least Squares) algorithms were employed sequentially as described. | |
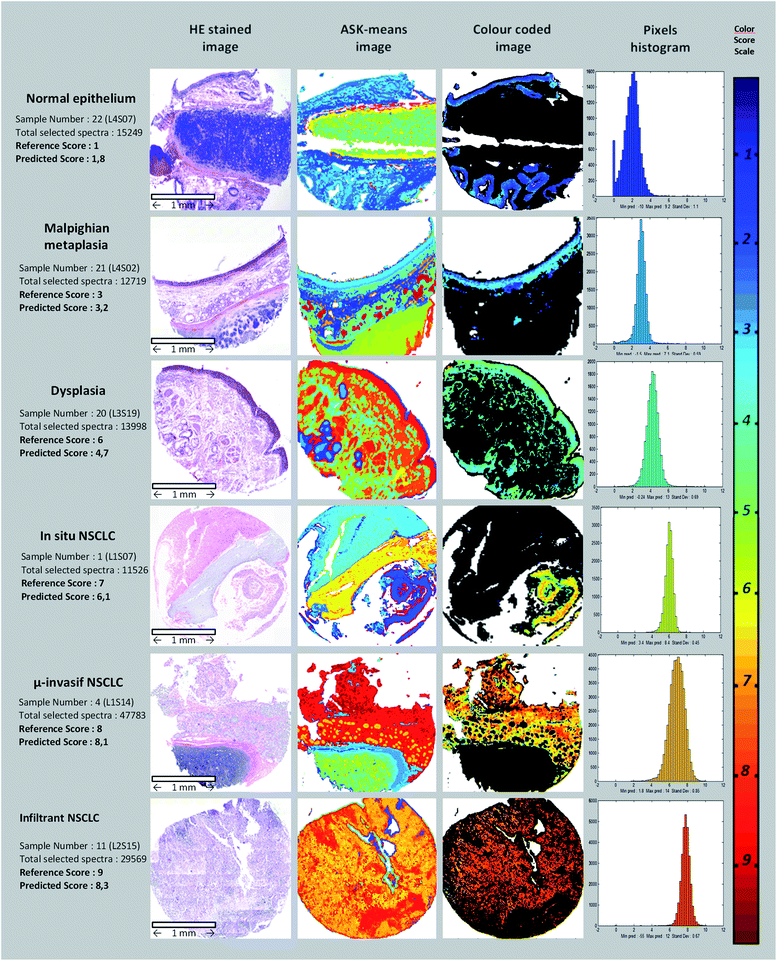 |
| Fig. 2 Results of unsupervised and supervised models constructed from the calibration. Representative calibration samples of NSCLC corresponding to the 6 phenotypes included in the analysis are presented. For each sample, three images are depicted: HE staining image revealing the tissue morphology, ASK image recovering the histological structures on the basis of their infrared signature and colour-coded image indicating the pixels of interest (associated with epithelial cells) scored according to the aggressiveness scale. From this last image, a graph is extracted for quantifying the numbers of pixels as a function of the aggressiveness scale. The last column of this figure shows the colour scale adopted to highlight the PLS pixel scoring. | |
Tumoral aggressiveness calibration
The construction of an aggressiveness spectral scale requires a sharp selection of the samples that must be representative of the different states and phenotypes encountered for the pathology of interest. In this study on bronchial carcinomas, 34 bronchial biopsies were selected by the collaborating pathologists and characterized using histopathological examinations. Table 2 lists 26 out of the 34 samples chosen to constitute the calibration data set. On the basis of the histological characterization, an empirical aggressiveness score was associated to each of these calibration samples. A score of 1 was given to the samples corresponding to normal epithelial tissues and a score of 9 was attributed to invasive tissues. Within this range of values, a progressive ascending score was given to the other samples according to their aggressiveness phenotype as indicated in Table 1.
Table 1 Elaboration of the aggressiveness scale on the basis of the histopathological characterisation of the lesions
Lesion type |
Phenotypic characteristics |
Aggressiveness score based on histopathology criteria |
Hypertrophy |
An increase in cell size and/or functional activity in response to a stimulus: score of 1 to 3 |
1 to 3 |
Hyperplasia |
An increase of cell numbers, via an increased mitotic activity in response to a stimulus |
2 to 4 |
Proliferative lesion |
An increase in cell growth and lication that is not dependent on an external stimulus |
3 to 5 |
Metaplasia |
A reversible process in which one mature cell type is replaced by another mature cell type (adaptive response to a stimulus) |
4 to 6 |
Dysplasia |
Reversible, irregular, atypical, proliferative cellular changes in response to irritation or inflammation |
5 to 7 |
Severe dysplastic lesion |
A lack of differentiation of tissue cells; a tumor with fewer differentiated cells is more malignant |
6 to 8 |
In situ carcinoma |
A local SCC tumor |
7 to 8 |
Invasive and micro-invasive carcinoma |
An irregular, atypical, proliferative SCC tumor |
8 to 9 |
The raw data workspace of this study corresponds to the total number of spectra multiplied by the number of points by spectrum which is related to the spectral range. Thus, for 34 images with a mean of 75 kspectra by image and 812 points by spectrum, the data workspace was initially constituted by more than 3 billion data points (for a total tissue surface of 1,48 cm2, 3.09 × 109 values were recorded). The calibration matrix corresponds to the workspace of only calibration images, after wavenumber range reduction to 800–1800 cm−1, EMSC quality test (elimination of outliers corresponding mainly to non-tissular pixels), and ASK epithelial structure selection (exclusion of non-epithelial structures). In this step, the calibration matrix, structured at the image level, contains 0.13 billion data points (corresponding to 506 kspectra with nearly 20 kspectra by samples). Finally, aggressiveness scores were linked to the calibration images to form the matrix of reference.
Fig. 3a presents the mean spectrum of the calibration matrix (dotted line) together with the min/max variability. Vibrations assigned to paraffin (around 1470 cm−1) still appeared but with almost no variability thanks to the twin EMSC pre-processing. Amide I and amide II signals, specific of protein content, were clearly visible. Fig. 3b shows the spectral distribution of the calibration data matrix by plotting the PCA scores on the first three components. Each point on this graph corresponded to one spectrum with the colour linked to the aggressiveness score determined by the pathologists (according to the colour order of the rainbow, right side of Fig. 2). Thus, each spectral image formed a monochromic cloud of points, and the repartition of the set of data tended to follow the order of their aggressiveness score. This observation reflects the fact that the vibrational data are likely to contain informative inputs about the aggressiveness phenotype of the NSCLC tissues.
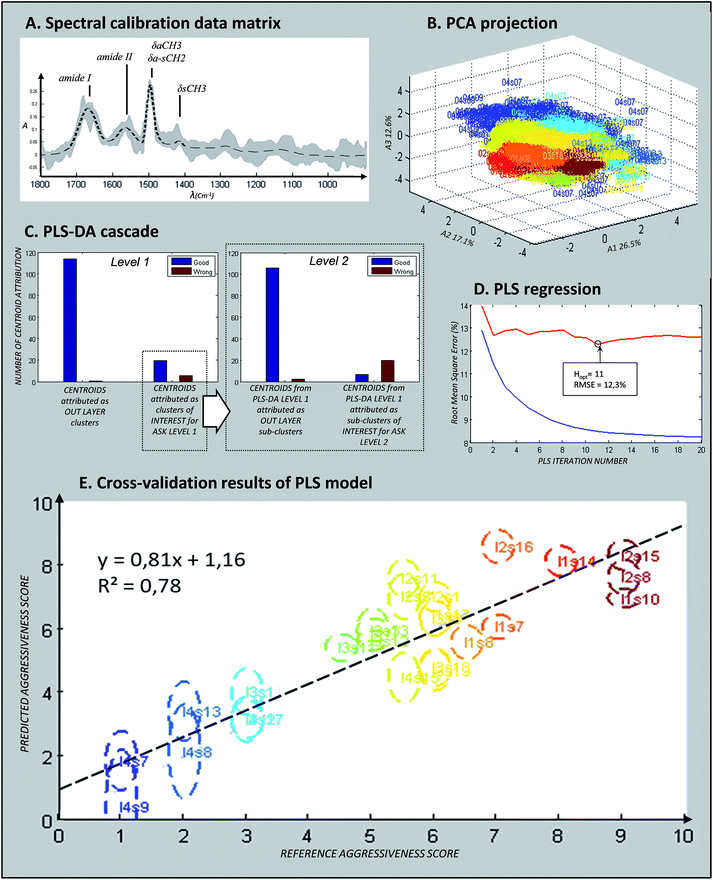 |
| Fig. 3 Results of the various chemometric steps required in calibration. (a) Representation of the mean (dotted line) and of min/max variability (grey zone) of the data matrix of calibration obtained after EMSC pre-processing. (b) Principal Component Analysis (PCA) projection of the calibration data matrix on the first 3 components. The percentage of variance for each PC is also indicated. Spectra are coloured according to the reference aggressiveness scale, based on histology expertise. The colours follow the rainbow order with blue associated with normal tissues (score of 1) and red-brown for NSCLC invasive tissues (score of 9). (c) Cross-validation results of the Partial Least Squares Discriminant Analysis (PLS-DA) cascade model. Inputs and outputs correspond to K-means clusters and sub-clusters highlighted by ASK. The histograms refer to confusion matrixes obtained at each of the two levels of the model cascade. Clusters and sub-clusters of interest correspond to clusters containing epithelial cells, whatever the aggressiveness phenotype appraised. Only clusters identified as of interest by the level 1 PLS-DA model were used to develop the level 2 model. (d) Root Mean Square Error (RMSE) of the aggressiveness scoring model (based on the PLS algorithm). The blue and red curves correspond to the RMSE of calibration and internal validation (image by image cross validation) as a function of H respectively, with H the iteration number (or also the number of computed dimensions of the PLS vectorial space). (e) Predicted aggressiveness score based on the IR model as a function of the reference aggressiveness score based on histology for each image of the calibration set. The prediction was realized at the pixel level. For each image, the prediction was visualised by an ellipse, with the ellipse centre corresponding to the mean predicted score and the ellipse vertical axis corresponding to pixel score standard deviation. | |
Automatic recognition of epithelial tissue structures
The PLS-DA cascade model was constructed by using ASK cluster centroids of the calibration samples. Similar to the ASK, PLS-DA models were constructed in a two-level way to identify the pixels associated with the epithelial structures (normal or malignant). First, centroids from ASK level 1 were used to build the first discriminative PLS-DA model considering 2 classes: one class (entitled the “epithelium” class) for clusters associated with the epithelium tissue structures and another class (“non-epithelium” class) for the rest of the clusters. Secondly, sub-centroids from ASK level 2 were used to build the second discriminative PLS-DA model. More precisely, only the sub-centroids from centroids attributed to the “epithelium” class at level 1 were used. This way of proceeding is schematically indicated in Fig. 3c. By using an image-by-image cross-validation, the performances of the PLS-DA cascade models can be evaluated by means of the level 1 and level 2 confusion matrixes viewable in the form of histograms in Fig. 3c. The blue and red bars represent the number of good and wrong attributions, respectively, for each class and level. For the first level, the results were encouraging and high percentage of good prediction could be reached in external validation. The second level optimization was not as good as for the first level optimization because few sub centroids of interest were predicted as non-epithelial structures. Despite this default of identification, the cascade PLS-DA models present the advantage of selecting a reduced number of pixels to be scored, avoiding considering pixels of non-interest. For further evaluation of the PLS-DA cascade models for automatic identification of pixels associated with epithelial cells, an independent (external) set of samples was used. These results are presented in Fig. 4, dedicated to an external validation of our models. The black pixels on the pictures of the third column corresponded to pixels not attributed to epithelial cells; the adjacent HE stained sections were also presented for visual comparison (second column). The comparison of these images highlighted that the tissue structure of interest was on the whole correctly identified by means of PLS-DA cascade models. Concerning sample #28 (second line of Fig. 4), the algorithm failed to recover a piece of epithelium tissue (left-up corner); however this tissue appeared structurally complex since it is composed of normal epithelium with invasive tumoral cells, making tedious the histological assignment of spectral clusters. For the other samples (#27, #29 and #30), the PLS-DA attribution was successful.
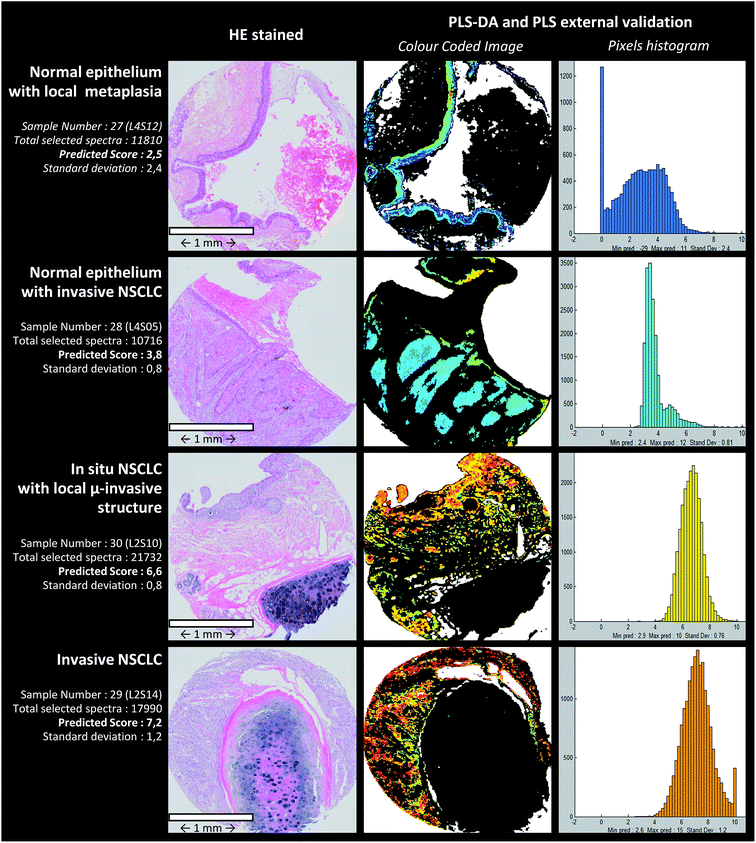 |
| Fig. 4 Prediction of the aggressiveness for independent samples of the test set. Analysis of 4 representative samples containing cells of different aggressiveness phenotypes. For each sample, the HE-stained image, PLS color-coded image and the associated histogram are obtained. | |
Aggressiveness scoring
After the assignment and scoring of each calibration sample on the basis of their histological features (third and fourth columns of Table 2), the PLS scoring model was calibrated by using leave-one-image-out cross-validation (internal validation). The blue and red curves correspond to the relative Root Mean Square Error (RMSE) of calibration and internal validation respectively (Fig. 3d). Hopt, the optimal number of dimensions of the vectorial space was determined to be 11 from the evolution of RMSE as a function of H, preventing under and over-fitting. Fig. 3e permits assessing the performances of the optimized model for the set of the 26 calibration spectral images. Indeed, the graph presents the correlation between the reference aggressiveness scores and the predicted scores based on the IR signatures of the epithelial cells. For each image, the ellipse reflects the intra-image variability with the vertical axis linked to the standard deviation. The value of the correlation coefficient (R2) was computed to be 0.78. A regression can be found between the infrared measurements and the aggressiveness phenotype (R2 > rα, with rα=0.01 = 0.5368 for n − 2 = 20 as degrees of freedom48). The fourth column of Table 2 details the results of PLS scoring for each of these samples. A global relative error of 12.3% was reached for the calibration set with a mean standard deviation close to 0.8, and this value has to be compared to the extent of the aggressiveness scale ranging from 1 to 9. These observations highlight the heterogeneity of scoring within the spectral images and a positive global bias reflecting a slight over scoring. Fig. 2 (last two columns) depicts examples of calibration images reconstructed by scoring the pixels selected by the PLS-DA model corresponding to epithelial cells. Various tissues from normal to invasive types are presented.
Table 2 Description of the calibration sample set and results of the internal leave-one-image-out-cross validationb
Experimental references |
References used for model calibration (pathologist empirical attribution) |
Result of internal validation (leave one image out cross validation) |
Sample image number |
Lame & spot numbers |
Pathologist expertise attribution |
Reference aggressiveness |
Predicted aggressiveness (mean of pixel-scores/images) |
Relative RMSEa for a 0 to 10 scale (%) |
Standard deviation (at the pixel level) |
Bias (at the image level) |
RMSE: Root Mean Square Error.
List of all used calibration samples with the lame number, spot position (allowing us to present sample images in Fig. 3e) and pathologist expertise. Reference aggressiveness is presented next to the predicted values of PLS internal cross validation. The 3 last columns show the prediction error, standard deviation and bias for each sample and the last row shows the mean of these 3 statistical values reached with internal cross validation.
|
1 |
L1S07 |
In situ SCC |
7.0 |
6.1 |
10.1 |
0.45 |
−0.93 |
2 |
L1S08 |
Dysplasia (medium/serious) |
6.5 |
5.6 |
10.6 |
0.72 |
−0.92 |
3 |
L1S10 |
Infiltrating SCC |
9.0 |
7.0 |
20.6 |
0.37 |
−2.04 |
4 |
L1S14 |
Micro-invasive SCC |
8.0 |
8.11 |
4.8 |
0.85 |
0.13 |
5 |
L1S17 |
Malpighian metaplasia + slight dysplasia |
6.0 |
6.5 |
8.1 |
0.51 |
0.45 |
6 |
L2S01 |
Malpighian metaplasia + slight dysplasia |
6.0 |
7.0 |
11.1 |
0.64 |
1.01 |
7 |
L2S03 |
Hyperplasia of basal cell + muco-secretion |
5.0 |
6.0 |
11.8 |
0.95 |
0.98 |
8 |
L2S05 |
Malpighian metaplasia |
5.5 |
7.0 |
16.7 |
0.87 |
1.52 |
9 |
L2S08 |
Infiltrating SCC |
9.0 |
7.6 |
14.7 |
0.52 |
−1.37 |
10 |
L2S11 |
Malpighian metaplasia |
5.5 |
7.6 |
21.6 |
0.63 |
2.09 |
11 |
L2S15 |
Infiltrating SCC |
9.0 |
8.3 |
8.3 |
0.67 |
−0.69 |
12 |
L2S16 |
In situ SCC |
7.0 |
8.6 |
16.6 |
0.55 |
1.58 |
13 |
L3S08 |
Malpighian metaplasia + slight dysplasia |
6.0 |
6.3 |
6.3 |
0.67 |
0.34 |
14 |
L3S11 |
Hyperplasia of basal cell |
4.5 |
5.4 |
10.1 |
0.48 |
0.91 |
15 |
L3S12 |
Hyperplasia of basal cell + mucosecretion |
5.0 |
5.6 |
7.1 |
0.58 |
0.64 |
16 |
L3S13 |
Hyperplasia of basal cell + mucosecretion |
5.0 |
6.0 |
10.3 |
0.51 |
0.96 |
17 |
L3S14 |
Normal epithelium + malpighian metaplasia |
3.0 |
4.0 |
12.6 |
0.85 |
1.03 |
18 |
L3S17 |
Normal epithelium + malpighian metaplasia |
3.0 |
3.2 |
7.3 |
0.91 |
0.17 |
19 |
L3S18 |
Malpighian metaplasia + slight dysplasia |
6.0 |
4.8 |
13.4 |
0.95 |
−1.20 |
20 |
L3S19 |
Malpighian metaplasia + slight dysplasia |
6.0 |
4.7 |
14.5 |
0.69 |
−1.31 |
21 |
L4S02 |
Normal epithelium + malpighian metaplasia |
3.0 |
3.2 |
6.2 |
0.59 |
0.17 |
22 |
L4S07 |
Normal epithelium |
1.0 |
1.8 |
12.8 |
1.10 |
0.80 |
23 |
L4S08 |
Normal epithelium + mucosecretion |
2.0 |
2.2 |
14.8 |
1.37 |
0.16 |
24 |
L4S09 |
Normal epithelium |
1.0 |
0.4 |
19.0 |
1.70 |
−0.59 |
25 |
L4S13 |
Normal epithelium + mucosecretion |
2.0 |
3.4 |
17.0 |
1.05 |
1.42 |
26 |
L4S15 |
Maplighian metaplasia |
5.5 |
4.5 |
13.0 |
0.90 |
−1.03 |
|
Mean of RMSE |
Mean of StDev |
Mean of bias |
12.3 |
0.78 |
0.16 |
External validation of the approach
To further assess the performance of our approach, the prediction model was tested on independent samples. The PLS scoring results obtained on 8 images from samples of different phenotypes are presented in Table 3. Prediction scores are overall in agreement with the phenotypes defined on the basis of histological features. In addition, a marked standard deviation at the pixel level prediction can be observed. Fig. 4 shows predicted images computed by PLS-DA and PLS models for 4 representative independent samples. From such images, histograms quantifying the number of pixels as a function of the aggressiveness score were computed. This representation permits providing objective criteria for characterizing the heterogeneity of the tissue samples at the cellular level.
Table 3 Results of the external validation on independent samplesa
Sample image number |
Pathologist expertise attribution (external validation images were prior chosen for their tissue heterogeneity) |
Predicted aggressiveness (mean of pixel scores for one image) |
Standard deviation (at the pixel level) |
For the first four samples (#27 to #30), the results of the IR scoring correspond to the PLS color-coded images shown in Fig. 4.
|
27 |
Normal epithelium + malpighian metaplasia |
2.5 |
2.40 |
28 |
Normal epithelium + invasive SCC |
3.8 |
0.81 |
29 |
In situ + micro-invasive SCC |
6.6 |
0.76 |
30 |
Invasive SCC |
7.2 |
1.20 |
31 |
Normal epithelium |
2.7 |
0.74 |
32 |
Normal epithelium + malpighian metaplasia |
3.5 |
0.86 |
33 |
In situ SCC |
6.4 |
1.30 |
34 |
Invasive SCC |
7.5 |
0.51 |
Discussion
The ASK algorithm allowed recovering via an unsupervised way the main structures of bronchial tissue samples at the micrometric pixel level, as shown in Fig. 2. Nevertheless, a slight confusion between bronchial epithelium and mucus cell gland can be observed as for sample #20 corresponding to a dysplastic tissue (Fig. 2). Increasing manually the number of pixels could solve this default but make difficult the assignment of the spectral clusters to specific histological structures. Except for this dysplastic sample, results of the ASK classification appeared very satisfactory, and with the advantage of determining automatically the optimal number of clusters.
After this unsupervised processing, a supervised model was implemented for an automatic detection of pixels of interest that will be subsequently scored according to an aggressiveness scale. PLS-DA was also applied in 2 steps, following the clustering steps of ASK and using ASK centroids as the objects to process during PLS-DA. This original method to construct a predictive model appeared more efficient than using a single step as currently done. This type of cascade PLS-DA predictive model was ever employed on vibrational data. In a study aiming at demonstrating the potential of infrared spectroscopy to classify filamentous fungi, the concept of cascade models was used to achieve a classification mimicking the phylogenic tree.49 In fact, for each phylogenic branching, one PLS-DA model was optimized and by this way, a cascade of interlocked PLS-DA models was elaborated.
The infrared-based aggressiveness scale was developed to describe the multi-step evolution of epithelial cells from normal until invasive squamous cell carcinoma. By considering various intermediary states, a scale with 9 distinctive levels was chosen in order to dispose enough calibration samples for each level and to differentiate the subtle differences between successive aggressiveness phenotypes. After preliminary experiments, intermediate levels were also defined as shown in Table 2 for certain samples; for example an aggressiveness score of 5.5 was given to sample #26 corresponding to squamous cell metaplasia. Similarly, a score equal to 6.5 was defined for sample #2 associated with a mixed phenotype of moderate and severe dysplasia. The achieved aggressiveness scale appeared appropriate as demonstrated by the results obtained on calibration and external independent sample sets although the number of images attributed to each level of the aggressiveness scale was relatively limited in this study. But, we made sure in our selection that there is a balanced number of samples between the different phenotypes, particularly for the normal and invasive tissues which correspond to the extreme phenotypes. PLS results presented in Table 2 and Fig. 3e for the calibration samples highlighted a correlation (R2 = 0.78) between the infrared signatures and the aggressiveness phenotypes of preneoplastic and squamous cell carcinoma tissues. Based on the Beer–Lambert law verified by infrared absorption, a hypothesis of linearity was followed for constructing the PLS model. However, it could be also possible to test other algorithms based on logarithmic or quadratic models to link the predicted spectral aggressiveness score to the phenotype.50
An interesting point of our approach is the highlighting of a marked cellular heterogeneity even within a single tissue spot. This tumoral heterogeneity, based on the vibrational features, can be quantified at the pixel level. Thus, the standard deviations computed from 0.37 to 1.70 for calibration images reflect the dispersion of pixel scores. In addition, for the independent samples of the external validation set, a higher standard deviation was observed (Table 3). Such a tumoral heterogeneity is also efficiently revealed and visualized by the reconstructed PLS color-coded images and associated histograms displayed in Fig. 4. For certain spots such as sample #28, we can even see that the histogram presents a double distribution of the pixels reflecting two distinctive tissue types within the same sample. Most of the samples of the external validation set were selected because they present, within the same section, structures with various aggressiveness phenotypes. The benefit of revealing and quantifying the tumoral heterogeneity makes spectral histopathology a complementary tool of conventional histology, for more precise characterisation of pathological tissues. In addition, the PLS-based approach employed to construct the predictive model can also provide the vibrational markers of the aggressiveness scale. Indeed, from the PLS regression vectors it is possible to identify infrared features involved in the scale.51 Since the infrared signals are of multivariate nature, several vibrations assigned to different chemical groups (e.g. 963 cm−1 PO4− symmetric stretching of phosphorylated proteins, 1015 and 1117 cm−1 assigned to C–O stretching vibrations in carbohydrates, amide I band specific of the protein content around 1650 cm−1, 1740 cm−1 attributed to C
O stretching in lipid compounds (triglycerides or phospholipids)…) can be highlighted as contributing to the spectral diagnosis.34,37,39 The implementation of this IR methodological approach relies strongly on the involvement of the pathologists that ensure the gold standard criteria. Indeed, not only for the construction of the predictive models but also for their validation on selected human tissues currently manipulated in clinics, pathologists had a key role in this innovative development.
Conclusions
In this study focussed on squamous cell preneoplastic and cancerous samples, we demonstrated the possibility to implement an automatic method for scoring the aggressiveness degree of tumoral lesions. This methodological development relies on an original chemometric chain permitting us to exploit the biochemical information contained in vibrational spectra. It provides quantitative indicators revealing inter- and intra-tumoral heterogeneities based on the infrared signatures of the tissues, opening the way to more precise histopathology. Our approach can be easily adapted to other malignancies. In this pilot study, the processing of multivariate data was developed in close collaboration with pathologists in order to ensure its validation on relevant samples sets. For potential use in routine clinics in the future, the approach will have to be assessed on larger datasets originating from multicentre cohorts. The possibility to analyse directly paraffin-embedded tissues will facilitate such large scale studies.52 It will also benefit technological advances, particularly with the emergence of new infrared sources based on quantum cascade lasers for making the acquisition time compatible with medical constraints.44
Materials and methods
Tumour tissue samples
The paraffin-embedded tumour pieces were obtained from samples of the Tumour Bank of the Reims University Hospital Biological Resource Collection No. DC-2008-374 declared at the Ministry of Health, according to the French Law, for the utilisation of tissue samples for research. Surgically resected tumours were collected after obtaining informed consent from patients with SSC (provided document). The samples were analysed in the form of 4 Tissue Micro-Arrays (TMA) containing a total of 34 tissue samples. Three consecutive slices were cut from these TMA cytoblocks, and the first and the third slices (5 μm of thickness) were stained with HE and the second slice (10 μm of thickness) was mapped by IR micro imaging. This way of preparation permits us to ensure that the middle slice dedicated to IR measurements contains tissue structures of interest.
Histopathological examination
Histopathological evaluation was performed independently by two dedicated pathologists on the HE sections. For each sample, main sites and tissue structures were identified including the alveolar space, mucus, bronchial epithelium (containing the squamous cells), Reissessen muscle, conjunctive tissue, serous and mucus-secreting cell glands, capillary network, under epithelium and finally cartilage. The aggressiveness phenotype was determined from the histological features of the epithelial structures. Thus, a reference scale covering all the aggressiveness levels was elaborated beginning from normal epithelium until invasive carcinoma as described in Table 1. The histological characterisation of the samples distributed in the calibration and external validation sets can be found in Tables 2 and 3, respectively.
IR instrumentation
The IR acquisitions were performed with a tandem device combining a Spotlight™ 400 microscope and a FTIR Frontier™ spectrometer (Perkin Elmer®, Courtaboeuf, France). The detector is composed of a 16 pixel matrix. Each pixel can be considered as an individual IR detector, with a spectrum associated with it. The pixel size on the sample was 6.25 × 6.25 μm2 and the size of tissue sections was approximately 2–3 mm2 which corresponds to 50
000–80
000 spectra/images. Spectra were collected in the wavenumber range from 750 to 4000 cm−1, with a spectral resolution of 4 cm−1 and 8 accumulations/measurements. For each sample, a paraffin image was collected on an area located at the periphery of the tissue with the same parameters. The backgrounds were recorded on a clean area of CaF2 substrates with 32 accumulations/measurements.
Data analysis
Fig. 1 presents a simplified scheme of the 8 steps followed to establish a structured data-bank and to construct qualitative and quantitative models. Pre-processing and unsupervised discrimination steps were principally based on EMSC (Extended Multiplicative Signal Correction) and ASK (Automatic Serial K-means) algorithms respectively. Subsequently, supervised algorithms based on PLS (Partial Least Squares) were employed. Predictive models were constructed from calibration samples annotated by the collaborative pathologist, and tested on independent samples in an external validation way.
Pre-processing of raw spectral data
Tissue and pure paraffin spectral images were firstly smoothed using the Savitzky–Golay algorithm with the following parameters: a window size of 6 points (discrete wavenumbers) and a polynomial degree of 1 for estimating the curve.53 Then, each tissue infrared image was processed by EMSC independently from the other images (Fig. 1, step 1). This correction was first proposed by Martens and Stark in 1991. In addition to baseline correction and normalisation, a major advantage of this correction is that spectral interferences, mainly due to the paraffin embedding in our case, can be integrated into the correction.45,46 EMSC also permits eliminating outlier spectra by applying a quality test.
Automatic Serial K-means (ASK) for spectral recognition of tissue structures
Unsupervised identification of tissue structures was realized by using the K-means algorithm that distributes all spectra of an image in a given number (K) of clusters (Fig. 1, step 2) on the basis of the spectral similarity between the data. Each cluster is then characterized by a specific infrared signature that corresponds to the mean spectrum (also called the centroid) of the pixels composing the cluster. The used algorithm, developed in our team, presents the advantage of determining automatically the optimal number of clusters, by using a validity index named PBM (Pakhira–Bandyopadhyay–Maulik) and 2 consecutive steps of clustering.54,55 The first K-means permits the partition of the pixels of an image into clusters, and then for each of these clusters a new K-means is performed to split the corresponding pixels into sub-clusters. The final number of clusters is equal to the number of sub-clusters. The ASK algorithm provides color-coded images where each sub-cluster is represented by a specific colour and is associated with a particular spectral signature (i.e. its sub-centroid).
Second EMSC to build a spectral data bank from various spectral images
To construct an ordered data matrix, a second EMSC was carried out in order to neutralize paraffin and baseline inter-image variability as also necessary in a previous study on cellular samples.51 The advantages of this approach were that one can correct the major part of parasitic interference variability due to the sample preparation, paraffin embedding or recording conditions. The interference matrix that is an input of the EMSC algorithm was constructed from the set of paraffin spectral images, collected at the periphery of the tissues (Fig. 1, step 3).
Partial Least Squares Discriminant Analysis (PLS-DA) for automatic selection of tissue structures of interest
PLS-DA is a supervised discriminative method developed from the PLS algorithm described below. This classification method is widely used for vibrational data classification.56 In this study, this processing allowed us to build predictive models able to identify the pixels corresponding to the histological structure of interest containing squamous epithelial cells. PLS-DA was also applied in 2 steps, following the clustering steps of ASK and using centroids as the objects to process during PLS-DA (Fig. 1, step 4).
From the first step of ASK applied to calibration images, two classes of cluster centroids were constructed based on the comparison of K-means images with the conventional histology of an adjacent section. The centroids associated with the epithelial structures formed the class of interest and the centroids associated with the other structures formed the class of non-interest which will have to be excluded in the further processing of the data. A PLS-DA model was then constructed on these centroids to discriminate between the two classes. At the end of this step, only the clusters identified as belonging to epithelial structures are retained. In order to refine the detection of epithelial structure pixels, the same principle was applied to the sub-centroids estimated on these epithelial clusters during the second step of ASK.
Partial Least Squares regression (PLS) for aggressiveness scoring at the pixel level
The PLS algorithm is based on a multivariate regression principle. It allows us to maximize the covariance between 2 matrices by means of multidimensional and orthonormal regression vectors. The established vectorial space allows linking infrared spectra to quantitative values, corresponding in our case to the empirical aggressiveness score established from the histological criteria (Fig. 1, step 5).47,57
External validation and optimization
Samples that present simultaneously several degrees of aggressiveness were excluded from the calibration set and reserved for the external validation set. Indeed, such heterogeneous samples images are not recommended for the calibration step due to the difficulty in assigning them a precise reference value. In contrast, they are particularly suitable to assess the performance of the discriminative PLS-DA and scoring PLS models (Fig. 1, steps 6, 7 and 8). The rest of tissues constituted the calibration set for the construction of the supervised models. For their optimization, a cross validation at the image level (image by image leave one out) was employed.58
All the computing steps were processed using Matlab R2013a (32 bit) (Mathwork, USA), EMSC, PLS and PLS-DA algorithms originated from the “saisir” toolbox developed by Bertrand and Cordella, INRA, France.59
Conflicts of interest
There are no conflicts to declare.
Acknowledgements
The authors thank gratefully the ITMO Cancer and ITMO Technologies pour la Santé coordinated by AVIESAN (National Alliance for Life Sciences & Health), for the financial support within the framework of the Cancer Plan. The authors thank warmly Dr Mohammed Essendoubi and Mr Nicolas Goffin for their help in the preparation of the manuscript, and the Lions Clubs of Soissons and Crépy-en-Valois for their support in our research.
References
- J. Ferlay, I. Soerjomataram, R. Dikshit, S. Eser, C. Mathers, M. Rebelo, D. M. Parkin, D. Forman and F. Bray, Int. J. Cancer, 2015, 136, E359–E386 CrossRef CAS PubMed.
- L. Ding, G. Getz, D. A. Wheeler, E. R. Mardis, M. D. McLellan, K. Cibulskis, C. Sougnez, H. Greulich, D. M. Muzny, M. B. Morgan, L. Fulton, R. S. Fulton, Q. Zhang, M. C. Wendl, M. S. Lawrence, D. E. Larson, K. Chen, D. J. Dooling, A. Sabo, A. C. Hawes, H. Shen, S. N. Jhangiani, L. R. Lewis, O. Hall, Y. Zhu, T. Mathew, Y. Ren, J. Yao, S. E. Scherer, K. Clerc, G. A. Metcalf, B. Ng, A. Milosavljevic, M. L. Gonzalez-Garay, J. R. Osborne, R. Meyer, X. Shi, Y. Tang, D. C. Koboldt, L. Lin, R. Abbott, T. L. Miner, C. Pohl, G. Fewell, C. Haipek, H. Schmidt, B. H. Dunford-Shore, A. Kraja, S. D. Crosby, C. S. Sawyer, T. Vickery, S. Sander, J. Robinson, W. Winckler, J. Baldwin, L. R. Chirieac, A. Dutt, T. Fennell, M. Hanna, B. E. Johnson, R. C. Onofrio, R. K. Thomas, G. Tonon, B. A. Weir, X. Zhao, L. Ziaugra, M. C. Zody, T. Giordano, M. B. Orringer, J. A. Roth, M. R. Spitz, I. I. Wistuba, B. Ozenberger, P. J. Good, A. C. Chang, D. G. Beer, M. A. Watson, M. Ladanyi, S. Broderick, A. Yoshizawa, W. D. Travis, W. Pao, M. A. Province, G. M. Weinstock, H. E. Varmus, S. B. Gabriel, E. S. Lander, R. A. Gibbs, M. Meyerson and R. K. Wilson, Nature, 2008, 455, 1069–1075 CrossRef CAS PubMed.
- H. R. Sanders and M. Albitar, Cancer Genet. Cytogenet., 2010, 203, 7–15 CrossRef CAS PubMed.
- H. K. Biesalski, B. Bueno de Mesquita, A. Chesson, F. Chytil, R. Grimble, R. J. Hermus, J. Kohrle, R. Lotan, K. Norpoth, U. Pastorino and D. Thurnham, Ca-Cancer J. Clin., 1998, 48, 167–176 CrossRef CAS.
-
L. Horn, W. Pao and D. H. Johnson, Neoplasms of the Lung, in Harrison's Principles of Internal Medicine, McGraw-Hill Medical Education, New York, 2012, pp. 737–753 Search PubMed.
- M. Malvezzi, P. Bertuccio, T. Rosso, M. Rota, F. Levi, C. La Vecchia and E. Negri, Ann. Oncol., 2015, 26, 779–786 CrossRef CAS PubMed.
- K. M. O'Reilly, A. M. McLaughlin, W. S. Beckett and P. J. Sime, Am. Fam. Physician, 2007, 75, 683–688 Search PubMed.
- A. Jemal, R. Siegel, J. Xu and E. Ward, Ca-Cancer J. Clin., 2010, 60, 277–300 CrossRef PubMed.
- I. Macia, J. Moya, I. Escobar, R. Ramos, C. Masuet, C. Gamez, R. Llatjos and I. Martinez-Ballarin, Eur. J. Cardio-Thorac. Surg., 2010, 37, 540–545 CrossRef PubMed.
- G. A. Silvestri, A. V. Gonzalez, M. A. Jantz, M. L. Margolis, M. K. Gould, L. T. Tanoue, L. J. Harris and F. C. Detterbeck, Chest, 2013, 143, e211S–e250S CrossRef PubMed.
- A. López-Encuentra, R. García-Luján, J. J. Rivas, J. Rodríguez-Rodríguez, J. Torres-Lanza and G. Varela-Simo, Ann. Thorac. Surg., 2005, 79, 974–979 CrossRef PubMed.
- J. D'Cunha, J. E. Herndon, D. L. Herzan, G. A. Patterson, L. J. Kohman, D. H. Harpole, K. H. Kernstine, J. A. Kern, M. R. Green, M. A. Maddaus and R. A. Kratzke, Lung Cancer, 2005, 48, 241–246 CrossRef PubMed.
- M. Ebrahimi, M. Auger, S. Jung and R. S. Fraser, Cancer Cytopathol., 2016, 124, 737–743 CrossRef PubMed.
- E. A. Perez, Chest, 1998, 114, 593–604 CrossRef CAS.
- K. L. Kehl, M. B. Landrum, K. L. Kahn, S. W. Gray, A. B. Chen and N. L. Keating, J. Oncol. Pract., 2015, 11, e267–e278 CrossRef PubMed.
- V. L. Capelozzi, J. Bras. Pneumol., 2009, 35, 375–382 CrossRef PubMed.
- O. Rena, R. Boldorini, E. Papalia, D. Turello, F. Massera, F. Davoli, A. Roncon, G. Baietto and C. Casadio, Ann. Thorac. Surg., 2014, 97, 987–992 CrossRef PubMed.
- A. Warth, T. Muley, E. Herpel, M. Meister, F. J. Herth, P. Schirmacher, W. Weichert, H. Hoffmann and P. A. Schnabel, Histopathology, 2012, 61, 1017–1025 CrossRef PubMed.
- D. Morgensztern, M. J. Campo, S. E. Dahlberg, R. C. Doebele, E. Garon, D. E. Gerber, S. B. Goldberg, P. S. Hammerman, R. S. Heist, T. Hensing, L. Horn, S. S. Ramalingam, C. M. Rudin, R. Salgia, L. V. Sequist, A. T. Shaw, G. R. Simon, N. Somaiah, D. R. Spigel, J. Wrangle, D. Johnson, R. S. Herbst, P. Bunn and R. Govindan, J. Thorac. Oncol., 2015, 10, S1–S63 CrossRef CAS PubMed.
- M. von Laffert, A. Warth, R. Penzel, P. Schirmacher, K. M. Kerr, G. Elmberger, H. U. Schildhaus, R. Buttner, F. Lopez-Rios, S. Reu, T. Kirchner, P. Pauwels, K. Specht, E. Drecoll, H. Hofler, D. Aust, G. Baretton, L. Bubendorf, S. Stallmann, A. Fisseler-Eckhoff, A. Soltermann, V. Tischler, H. Moch, F. Penault-Llorca, H. Hager, F. Schaper, D. Lenze, M. Hummel and M. Dietel, J. Thorac. Oncol., 2014, 9, 1685–1692 CrossRef CAS PubMed.
- H. J. An, Y. J. Lee, S. A. Hong, J. O. Kim, K. Y. Lee, Y. K. Kim, J. K. Park and J. H. Kang, Pathol., Res. Pract., 2016, 212, 357–364 CrossRef CAS.
- M. Mino-Kenudson, L. R. Chirieac, K. Law, J. L. Hornick, N. Lindeman, E. J. Mark, D. W. Cohen, B. E. Johnson, P. A. Janne, A. J. Iafrate and S. J. Rodig, Clin. Cancer Res., 2010, 16, 1561–1571 CrossRef CAS.
- K. Adams, P. L. Shah, L. Edmonds and E. Lim, Thorax, 2009, 64, 757–762 CrossRef CAS.
- L. P. Hariri, M. Mino-Kenudson, M. Lanuti, A. J. Miller, E. J. Mark and M. J. Suter, Ann. Am. Thorac. Soc., 2015, 12, 193–201 CrossRef PubMed.
- T. P. Kotadia, J. H. Jasani and P. N. Vekaria, Int. J. Biomed. Adv. Res., 2013, 4, 579–584 CrossRef.
- S. W. Um, H. K. Kim, S. H. Jung, J. Han, K. J. Lee, H. Y. Park, Y. S. Choi, Y. M. Shim, M. J. Ahn, K. Park, Y. C. Ahn, J. Y. Choi, K. S. Lee, G. Y. Suh, M. P. Chung, O. J. Kwon, J. Kim and H. Kim, J. Thorac. Oncol., 2015, 10, 331–337 CrossRef PubMed.
- C. Kallaway, L. M. Almond, H. Barr, J. Wood, J. Hutchings, C. Kendall and N. Stone, Photodiagn. Photodyn. Ther., 2013, 10, 207–219 CrossRef CAS PubMed.
- K. Kong, C. J. Rowlands, S. Varma, W. Perkins, I. H. Leach, A. A. Koloydenko, H. C. Williams and I. Notingher, Proc. Natl. Acad. Sci. U. S. A., 2013, 110, 15189–15194 CrossRef CAS PubMed.
- J. W. Spliethoff, D. J. Evers, H. M. Klomp, J. W. van Sandick, M. W. Wouters, R. Nachabe, G. W. Lucassen, B. H. Hendriks, J. Wesseling and T. J. Ruers, Lung Cancer, 2013, 80, 165–171 CrossRef PubMed.
- H. Zeng, A. McWilliams and S. Lam, Photodiagn. Photodyn. Ther., 2004, 1, 111–122 CrossRef CAS PubMed.
- E. Kaznowska, J. Depciuch, K. Łach, M. Kołodziej, A. Koziorowska, J. Vongsvivut, I. Zawlik, M. Cholewa and J. Cebulski, Talanta, 2018, 186, 337–345 CrossRef CAS PubMed.
- E. Kaznowska, K. Łach, J. Depciuch, R. Chaber, A. Koziorowska, S. Slobodian, K. Kiper, A. Chlebus and J. Cebulski, Infrared Phys. Technol., 2018, 89, 282–290 CrossRef CAS.
- M. Verdonck, A. Denayer, B. Delvaux, S. Garaud, R. De Wind, C. Desmedt, C. Sotiriou, K. Willard-Gallo and E. Goormaghtigh, Analyst, 2016, 141, 606–619 RSC.
- N. Wald, N. Bordry, P. G. Foukas, D. E. Speiser and E. Goormaghtigh, Biochim. Biophys. Acta, 2016, 1862, 202–212 CrossRef CAS PubMed.
- E. Ly, O. Piot, A. Durlach, P. Bernard and M. Manfait, Analyst, 2009, 134, 1208–1214 RSC.
- J. D. Pallua, C. Pezzei, B. Zelger, G. Schaefer, L. K. Bittner, V. A. Huck-Pezzei, S. A. Schoenbichler, H. Hahn, A. Kloss-Brandstaetter, F. Kloss, G. K. Bonn and C. W. Huck, Analyst, 2012, 137, 3965–3974 RSC.
- E. Ly, N. Cardot-Leccia, J. P. Ortonne, M. Benchetrit, J. F. Michiels, M. Manfait and O. Piot, Br. J. Dermatol., 2010, 162, 1316–1323 CrossRef CAS PubMed.
- J. Nallala, O. Piot, M. D. Diebold, C. Gobinet, O. Bouché, M. Manfait and G. D. Sockalingum, Cytometry, Part A, 2013, 83, 294–300 CrossRef PubMed.
- K. Augustyniak, K. Chrabaszcz, A. Jasztal, M. Smeda, G. Quintas, J. Kuligowski, K. M. Marzec and K. Malek, J. Biophotonics, 2018, e201800345 CrossRef PubMed.
- A. Akalin, X. Mu, M. A. Kon, A. Ergin, S. H. Remiszewski, C. M. Thompson, D. J. Raz, M. Diem, B. Bird and M. Miljkovic, Lab. Invest., 2015, 95, 406–421 CrossRef PubMed.
- B. Bird, M. S. Miljkovic, S. Remiszewski, A. Akalin, M. Kon and M. Diem, Lab. Invest., 2012, 92, 1358–1373 CrossRef PubMed.
- X. Mu, M. Kon, A. Ergin, S. Remiszewski, A. Akalin, C. M. Thompson and M. Diem, Analyst, 2015, 140, 2449–2464 RSC.
- M. Khanmohammadi and A. B. Garmarudi, Trends Anal. Chem., 2011, 30, 864–874 CrossRef CAS.
- S. Mittal, K. Yeh, L. S. Leslie, S. Kenkel, A. Kajdacsy-Balla and R. Bhargava, Proc. Natl. Acad. Sci. U. S. A., 2018, 115, E5651–E5660 CrossRef CAS PubMed.
- A. Kohler, U. Bocker, J. Warringer, A. Blomberg, S. W. Omholt, E. Stark and H. Martens, Appl. Spectrosc., 2009, 63, 296–305 CrossRef CAS PubMed.
- R. Wolthuis, A. Travo, C. Nicolet, A. Neuville, M. P. Gaub, D. Guenot, E. Ly, M. Manfait, P. Jeannesson and O. Piot, Anal. Chem., 2008, 80, 8461–8469 CrossRef CAS PubMed.
- W. Land, F. William, J. W. Park, R. Mathur, N. Hotchkiss, J. J. Heine, S. Eschrich, X. Qiao and T. Yeatman, Procedia Computer Science, 2011, 6, 273–278 CrossRef.
-
R. A. Fisher and F. Yates, in Statistical Tables for Biological, Agricultural and Medical Research, Oliver & Boyd, Edinburgh and London, 6th edn, 1963, p. 72 Search PubMed.
- V. Gaydou, A. Lecellier, D. Toubas, J. Mounier, L. Castrec, G. Barbier, W. Ablain, M. Manfaitab and G. D. Sockalingum, Anal. Methods, 2015, 7, 766–778 RSC.
- J. R. Beattie, A. M. Pawlak, M. E. Boulton, J. Zhang, V. M. Monnier, J. J. McGarvey and A. W. Stitt, FASEB J., 2010, 24, 4816–4824 CrossRef CAS PubMed.
- V. Gaydou, M. Polette, C. Gobinet, C. Kileztky, J. F. Angiboust, M. Manfait, P. Birembaut and O. Piot, Anal. Chem., 2016, 88, 8459–8467 CrossRef CAS PubMed.
- J. Depciuch, E. Kaznowska, K. Szmuc, I. Zawlik, M. Cholewa, P. Heraud and J. Cebulski, Infrared Phys. Technol., 2016, 76, 217–226 CrossRef CAS.
- P. Lasch, Chemom. Intell. Lab. Syst., 2012, 117, 100–114 CrossRef CAS.
- T. N. Nguyen, P. Jeannesson, A. Groh, D. Guenot and C. Gobinet, Analyst, 2015, 140, 2439–2448 RSC.
- T. N. Nguyen, P. Jeannesson, A. Groh, O. Piot, D. Guenot and C. Gobinet, J. Biophotonics, 2016, 9, 521–532 CrossRef PubMed.
- O. Preisner, J. A. Lopes and J. C. Menezes, Chemom. Intell. Lab. Syst., 2008, 94, 33–42 CrossRef CAS.
- P. Bastien, P. Bastiena, V. E. Vinzi and M. Tenenhaus, Comput. Stat. Data Anal., 2005, 48, 17–46 CrossRef.
- S. Arlot and A. Celisse, Stat. Surv., 2010, 4, 40–79 CrossRef.
- C. Cordella and D. Bertrand, Trends Anal. Chem., 2014, 54, 75–82 CrossRef CAS.
|
This journal is © The Royal Society of Chemistry 2019 |
Click here to see how this site uses Cookies. View our privacy policy here.