DOI:
10.1039/C9RA08096A
(Paper)
RSC Adv., 2019,
9, 41107-41119
Metabolomics profiling of methamphetamine addicted human serum and three rat brain areas
Received
6th October 2019
, Accepted 29th November 2019
First published on 12th December 2019
Abstract
Methamphetamine (METH) has already been a serious problem all over the world. The identification of related biomarkers and pathways is helpful to evaluate the degree of METH addiction, develop appropriate treatment during abstinence, and explore the mechanism. Here, it is the first time to perform metabolomics profiling of METH addicted human serum and three regions of METH-induced conditioned place preference (CPP) rat brain by using UHPLC-MS/MS and matrix-assisted laser desorption/ionization-mass spectrometry imaging (MALDI-MSI), respectively. Untargeted metabolomics analysis demonstrated clear differences between METH abusers and the healthy control by finding 35 distinct expressed metabolites in serum, including 5 TCA intermediates, 17 amino acids and 13 other biomolecules, 15 of which were newly identified following METH exposure. By using MALDI-MSI, the relative quantification and distribution of 14 metabolites were investigated in the nucleus accumbens (NAc), dorsal hippocampus (dHPC) and ventral hippocampus (vHPC) of CPP rat brain. Taken together, METH addiction could influence energy metabolism, amino acids metabolism, and phospholipids metabolism. A multi-parameter model consisting of these related metabolites can be established as a METH addiction biomarker in the future. The mapping of phospholipids provided new insights into the mechanism of METH addiction. Notably, the trend of metabolite changes in NAc and dHPC was almost the same, while it was opposite between dHPC and vHPC. It seems that NAc and dHPC were the two regions more susceptible to METH administration in the brain. And dHPC and vHPC play different roles in METH addiction proved by metabolites mapping.
1. Introduction
METH is an amphetamine-type central nervous system (CNS) stimulant with known neurotoxic and neurocognitive effects.1 The abuse of METH can lead to cognitive deficits and psychiatric disorders, such as anxiety, paranoia and hallucinations2,3 by causing adverse changes in brain structure and function, including damaging dopaminergic neurons in the midbrain and serotonin neurons in the CNS.4 This stimulant can be easily synthesized from inexpensive and readily obtainable chemical precursors.2 During these years, METH abuse has gone up among East/South-East/South-West Asia, Oceania, North America, and parts of Europe, with estimates of 35 million regular users globally.2 Due to the illegal production, distribution, sale and possession,5 METH abuse has become a public health and safety problem around the world. However, research about METH addiction related biomarkers and the mechanism is far from enough.
Approximately 43% of METH is excreted in urine unchanged and 4–7% is discharged as amphetamine.2 High urine acidity is associated with higher METH levels,2 so METH abusers are usually confirmed via urine test. But the half-life of METH elimination is 8–13 h,6 which makes the time window of METH abuse detection by using urine is only 48 h.7 Biomarkers of METH abuse/addiction are required to get objective, real assessment of the degree of METH addiction and to evaluate the treatment during abstinence.
Biomarkers are proposed to offer a number of important benefits, ranging from early diagnosis to withdrawal treatment choice. With the development in informatics and analytical technologies, the omics-based analysis of biological samples, such as blood, urine or saliva, have the potential to map dysregulated systems and uncharacteristic pathways involved in disease pathogenesis.8 By comparing the expression of miRNAs in the serum exosomes of METH-dependent and ketamine-dependent rats, METH upregulated the expression of 276 miRNAs and downregulated 25 miRNAs.9 Shi et al. identified five METH addiction related proteins by evaluating the serum protein expression changes of METH addicted patients via 2-DE-based proteomics.10 Li et al. investigated the depression and alterations in the hypothalamic–pituitary–adrenal and hypothalamic–pituitary–thyroid axis function in METH abusers after abstinence, finding serum levels of adrenocorticotropic hormone and thyroxine increased, but cortisol, triiodothyronine, and thyroid-stimulating hormone decreased.11 Several experimental models have been used to study the metabolic disturbances under a variety of METH treatment.12 It has been reported that METH exposure can induce perturbation of neurotransmitters,13 as well as influencing energy metabolism, in animals.14 But due to the lack of models reflecting personal genetic variances and environmental factors, METH addiction mechanisms remain unclear. Furthermore, with the modification of post-transcription and post-translation, genomics, transcriptomics and proteomics are more suitable for predicting what will happen in the organism, while metabolomics analysis can be applied to detect subtle alterations of metabolites with higher sensitivity and specificity than traditional diagnostic approaches. Therefore, metabolomics has unlocked new possibilities to explore the mechanisms and develop potential biomarkers underlying kinds of physiological conditions and aberrant processes, including METH addiction.8
The common methodologies of metabolomics analysis are untargeted and targeted mass spectrometry-based metabolomics.8,15 It can be used to detect and quantify a large variety of chemical entities, such as peptides, lipids, and drugs.15 However, the sample homogenization is required, so tissue-specific information on compounds of interest is sacrificed.15 MALDI-MSI is able to reveal the location of various biochemicals or selected metabolites within a tissue sample.8,15 Combining both advantages of mass spectrometry and cytomics, MALDI-MSI has been widely used to identify differently expressed molecules including peptides, lipids in the tissue of patients and healthy control to develop new biomarkers, locate targeted biochemicals or drugs.16,17 Rising researches have been reported about the successful performance of MALDI-MSI ranging from cardiovascular,18 neurologic,19 joint disorders,20 renal diseases,21 to the field of reproductive medicine22 and various tumour entities.23–25
In this study, in order to identify METH addiction related biomolecules and pathway, to explore the mechanism of METH addiction, metabolomics profiling of METH addicted human serum and rat brain were performed. 35 differentially expressed metabolites were identified in the serum, which included energy metabolism related molecules, amino acids neurotransmitters. They were involved in several metabolic pathways, such as citrate cycle, alanine, aspartate and glutamate metabolism, and were related to many diseases. By using MALDI-MSI, METH addiction related metabolites, mainly energy metabolism related molecules, amino acids, antioxidant, and phospholipids, were found and mapped in NAc, dHPC and vHPC. A multi-parameter model including some of these metabolites can be established for METH addiction diagnosis and treatment. The mapping of phospholipids provided new insight into the mechanism of METH addiction. NAc and dHPC are more susceptible to METH administration. Furthermore, dHPC and vHPC play different roles in METH addiction proved by metabolites mapping.
2. Materials and methods
2.1. Untargeted metabolomic analysis of human serum
2.1.1. Serum sample collection. Male Han Chinese chronic METH abusers (mean age ± SD: 25.9 ± 4.0) were recruited from Shenzhen Detoxification & Rehabilitation Center in this study. They were all active METH users at study entry with urine toxicology screen. Exclusion criteria included acutely psychosis and infectious disease. Finally, the serum of 30 patients have been collected for this study. The controls were age equivalent healthy people (mean age ± SD: 21 ± 4.5) with no history of METH or any other addictive drug usage, and consecutively recruited at Peking University Third Hospital. This study was approved by the Human Subjects Division at Peking University Health Science Center (Licence number: IRB00001052-16078). Informed consent was obtained from all individual participants included.Fasting peripheral blood was collected by venepuncture between 7 am and 9 am in the morning and centrifuged within 30 min in a serum separator tube at 4 °C. Serum was collected and stored at −80 °C.
2.1.2. Metabolite extraction from serum. The metabolites were extracted from human serum following the description of Yuan et al.26 100 μL of homogeneous serum was mixed with 4 volume pre-chilled methanol containing internal standard (Gln-C13, 2 μg mL−1). After 2 hours incubation at −80 °C, the mixture was centrifuged at 12
000 × g at 4 °C for 10 min and same volume supernatant was collected to a fresh tube. The samples were dried by using speedvac. 80% (v/v) methanol with internal standard (Trp-D5, 2 μg mL−1) was added to reconstitute each dried sample. Incubated the samples for 15 min at 4 °C, and centrifuged them again. Finally, 20 μL of supernatant were taken from each tube for UHPLC-MS/MS analysis.
2.1.3. UHPLC-MS/MS methods for metabolites. Ultimate 3000 UHPLC (Dionex) coupled with Q Exactive (Thermo, CA) was used for the untargeted metabolite screening. Atlantis HILIC Silica column (2.1 × 100 mm, 3 μm, waters) and BEH amide columns (2.1 × 100 mm, 1.7 μm, waters) were used for separation at 35 °C in positive and negative mode, respectively. The mobile phase A and B for positive and negative modes were prepared according to the method of Tian et al.27 In positive mode, mobile phase A/B were 95%/50% acetonitrile with 10 mmol L−1 ammonium formate and 0.1% formic acid, while in negative mode, mobile phase A and B were 95% and 50% acetonitrile with 10 mmol L−1 ammonium formate. The pH of A and B was 9.0 adjusted with ammonium hydroxide in negative mode. All mobile phases were freshly prepared to avoid bacterial contamination. The mass spectrometer parameters were the same as described by Tang et al.28 and summarized as following: spray voltage, positive (3.5 kV)/negative (2.5 kV); capillary temperature, positive (275 °C)/negative (320 °C); sheath gas flow rate (arb), 35; aux gas flow rate (arb), 8; mass range (m/z), positive (70–1050)/negative (80–1200); full MS resolution, 70
000; MS/MS resolution, 17
500; top N, 10; NCE, 15/30/45; duty cycle, 1.2 s.A quality control (QC) sample was generated by mixing equal volume of the final supernatant mentioned in metabolite extraction section from every sample. QC was injected at the beginning of the sample queue and after every 15 samples for system condition assessment. The samples were analysed in random order.
2.1.4. Data processing and statistical analysis for metabolomic analysis. Only the features existing in more than 80% samples were kept for further analysis. TraceFinder (Thermo) was used for data dependent MS/MS acquisition with 10 ppm and 15 ppm mass tolerance for precursor and fragment, respectively. And 0.25 min retention time shift was allowed for quantitation. Normalization was performed by transferring the relative ion intensity to the sum of the peak area of a sample. SIMCA-P 13.0 software package (Umetrics, Umeå, Sweden) was used for Principal components analysis (PCA) and partial least-squares discriminant analysis (PLS-DA) after the normalization and Pareto scale. The data of the five QC injections were applied to data correction and involved in the PCA to check system stability. A 200-times permutation test was performed to validate the model against overfitting.METH addiction related biomolecules were chosen according to variable importance in the project (VIP) values and p-values in PLS-DA analysis. The features with VIP > 1 and p < 0.05 were used for pathway analysis by using MetaboAnalyst 4.0 following the instruction described by Xia et al.29 and Chong et al.30 The pathway analysis algorithms were set as below: hypergeometric test for over representation analysis; relative-betweenness centrality for pathway topology analysis. And Homo sapiens (KEGG) was selected for pathway library.
2.2. MALDI-MSI of METH induced CPP rat brain
2.2.1. Animals. 30 male healthy Sprague-Dawley rats (280 ± 20 g) were purchased from Beijing Vital River Laboratory Animal Technology Co., Ltd (License number: SCXK(JING) 2012-0001). They were kept in a room under 22–24 °C, 50–60% relative humidity and a 12 h light/dark cycle with free access to sufficient chow and water. All animal experiments were approved by the Ethics Committee for Laboratory Animal Care and Use of Peking University Health Science Center and conducted according to the National Institutes of Health Guide for the Care and Use of Laboratory Animals.
2.2.2. Establishment of the CPP model. The CPP model of this study was established based on slight modification of previous report.10 It can be briefly described as following. After a 5 days adaption phase in the environment-controlled room mentioned above, the animals were put into the CPP apparatus from the transition-chamber (T-chamber) and allowed for a 900 s exploration for 3 days as habituation phase. The third day of the habituation phase was used for pre-conditioning test to determine their preferred chamber of each rat according to the chamber (A/B) which they spent more time to stay in. Then, 20 rats were selected, which spent less than 550 s in their preferred chambers. They were randomly divided into Saline group (SA) and METH group (METH), n = 10 for either group. During conditioning test of the next 6 days, they were kept in the nonpreferred chamber for 30 min after receiving METH (1 mg kg−1, i.p.) or saline (i.p.) on day 1, 3, and 5. On day 2, 4, and 6, they were placed in preferred chamber after saline injection. On day 15, a post-condition test was performed as the procedure of the habituation phase to get the CPP score (CPP score = time (in nonpreferred chamber) − time (in preferred chamber)) to confirm the establishment of CPP model. The time of every animal spent in the chambers were recorded via an automatic monitoring system during pre and post conditioning tests. According to CPP score, 12 rats meeting CPP model requirement were chosen for statistical analysis and further experiment (n = 6 for METH group; n = 6 for SA group). Within 2 hours after the post-condition test, the brains of the selected animals were collected under full anaesthesia as soon as possible, rapidly frozen in liquid nitrogen and stored at −80 °C.
2.2.3. Mass spectrometry imaging. The brain was fixed on the cutting stage by ddH2O and sectioned to 10 μm thickness coronary slices using a Leica CM1950 Cryostat (Leica Microsystems GmbH, Wetzlar, Germany). The slices containing NAc, dHPC and vHPC, together or respectively, were selected according to the description of Paxinos and Watson31 and placed onto indium tin oxide (ITO) coated glass slides. The conjunct slices were also collected for hematoxylin–eosin (H&E) staining. The slides were dried via vacuum desiccator for 30 min. Then they were used for MALDI-MSI after matrix application as described before.32 1,5-diaminonaphthalene (1,5-DAN hydrochloride) in 50% ethanol/water was sprayed on the ITO coated slides with tissue sections using an automatic matrix sprayer (ImagePrep, Bruker Daltonics) under regular process.32 An autoflex speed MALDI-MSI (Bruker Daltonics, Billerica, MA) was applied to perform the mass spectrometry imaging of molecules between 60 and 1000 Da using reflector, negative ion mode at 1000 Hz and 400 consecutive laser shots. The laser spot was set as medium. Random walk mode was employed with 50 shots at every 50 μm diameter raster spot.The peaks were identified by comparison of mass spectrometry with the database established by Liu et al.32 SCiLS Lab 2018b was used to normalize the raw data against total ion count.32 The imaging results of interested regions were manually defined and visualized by flexImaging (Bruker Daltonics). The average signal intensity of the SA control group was set as 100% and the ratio of the average signal intensity between METH and SA control group was used for relative quantitation. Unpaired t test in GraphPad Prism 6.01 was used for statistical analysis.
3. Results
3.1. Metabolomic analysis of serum specimens
3.1.1. Serum metabolomic profiling of METH abusers. Here, the serum specimens of 30 METH abusers and 30 healthy control were subjected to untargeted metabolomics analysis by UHPLC-MS/MS. 228 and 350 features were identified from electrospray ionization positive (ESI+) and negative (ESI−) mode, respectively. PCA (Fig. 1A) including QC has been performed to validate the system stability, which was demonstrated by the tightly clustered QC dots in PCA scores plot. Moreover, clear metabolic difference between the METH group (M) and control group (C) was suggested by the obvious distinction via PCA (Fig. 1A) and PLS-DA analysis (Fig. 1B). The R2Y (cum) and Q2 (cum) values of this PLS-DA model were 0.964 and 0.953, which showed this model had good fitness and predictability. To further validate the model and guard against model overfitting, a 200-times permutation test was performed. The result showed that all the Q2 values were lower than the R2 values in the left of the permutation test (Fig. 1C) and the intercept for the Q2 (0, −0.0988) to the y-axis is below zero, indicating the validity of the current mode.33 METH administration caused distinct metabolic deviations in human serum.
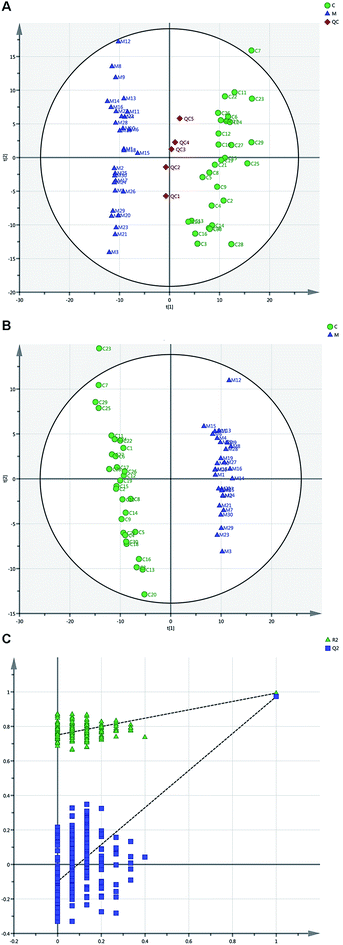 |
| Fig. 1 Metabolomic profiling of serum between METH abusers (M) and health subjects (C). (A) PCA scores plot based on data of 30 METH abusers, 30 health subjects and 5 QC dots demonstrated the stability and repeatability of untargeted metabolomics analysis by UHPLC-MS (R2X (cum) = 0.178, R2Y (cum) = 0.964, Q2 (cum) = 0.143). (B) PLS-DA of METH and health groups showed clear difference between these two groups (R2X (cum) = 0.18, R2Y (cum) = 0.964, Q2 (cum) = 0.953). (C) A 200-times permutation test for the corresponding model. The Y-axis intercepts were: R2 (0, 0.751), Q2 (0, −0.0988). | |
3.1.2. Identification of METH addiction related metabolites. METH addiction related metabolites were selected according to the statistically significance with VIP scores > 1 in the PLS-DA model and p < 0.05 in the Wilcoxon Mann–Whitney test. As a result, 35 potential marker metabolites have been identified, including 5 TCA intermediates, 17 amino acids and 13 other biomolecules. The levels of lactate, glutamine, ornithine, niacinamide, histidine, creatinine, acetylcholine, asparagine, glutamate, malic acid, 5-aminolevulinic acid, taurine, 1-methylnicotinamide, citrulline, guanidine acetic acid were significantly increased in the serum of METH addicts compared with that of the control group, while less choline, succinate semialdehyde, threonine, glycine, serine, isoleucine, proline, xanthine, creatine, alanine, sulfate, methionine, uric acid, citrate, pyruvic acid, fumarate, N-acetyl-L-aspartate, ketoleucine, valine, succinate were detected in METH abusers' serum. The details of these 35 metabolites were summarized in Table 1.
Table 1 METH addiction related metabolites in METH abuser seruma
No. |
Mass |
Name |
m/z (delta (ppm)) |
HMDB ID |
PubChem ID |
KEGG ID |
VIP |
p-Value |
Change trend |
↑, upregulated in the serum of METH abusers; ↓, downregulated in the serum of METH abusers. |
1 |
104.107 |
Choline |
−0.140438615 |
HMDB0000097 |
305 |
C00114 |
2.06161 |
1.68 × 10−17 |
↓ |
2 |
101.024 |
Succinate semialdehyde |
2.570022727 |
HMDB0001259 |
1112 |
C00232 |
1.9904 |
2.0925 × 10−15 |
↓ |
3 |
118.05044 |
Threonine |
2.519590758 |
HMDB0000167 |
6288 |
C00188 |
1.96783 |
8.0762 × 10−15 |
↓ |
4 |
89.0239 |
Lactate |
3.541025606 |
HMDB0000190 |
107689 |
C00186 |
1.876 |
1.7958 × 10−12 |
↑ |
5 |
76.03983 |
Glycine |
−5.629295231 |
HMDB0000123 |
750 |
C00037 |
1.86682 |
1.3022 × 10−8 |
↓ |
6 |
104.03479 |
Serine |
0.453296615 |
HMDB0000187 |
5951 |
C00065 |
1.80436 |
4.1363 × 10−11 |
↓ |
7 |
132.10243 |
Isoleucine |
−4.708096154 |
HMDB0000172 |
6306 |
C00407 |
1.77176 |
0.012889 |
↓ |
8 |
147.077 |
Glutamine |
−4.470840154 |
HMDB0000641 |
5961 |
C00064 |
1.6827 |
0.00022639 |
↑ |
9 |
131.083 |
Ornithine |
−2.458734697 |
HMDB0000214 |
6262 |
C00077 |
1.66003 |
6.8538 × 10−9 |
↑ |
10 |
123.056 |
Niacinamide |
−4.665154923 |
HMDB0001406 |
936 |
C00153 |
1.65134 |
9.216 × 10−9 |
↑ |
11 |
154.062 |
Histidine |
1.347303333 |
HMDB0000177 |
6274 |
C00135 |
1.61968 |
2.0229 × 10−8 |
↑ |
12 |
116.07113 |
Proline |
2.050077424 |
HMDB0000162 |
145742 |
C00148 |
1.60381 |
2.8708 × 10−8 |
↓ |
13 |
112.051 |
Creatinine |
2.848437424 |
HMDB0000562 |
588 |
C00791 |
1.60007 |
3.6136 × 10−8 |
↑ |
14 |
146.118 |
Acetylcholine |
−0.774038462 |
HMDB0000895 |
187 |
C01996 |
1.58033 |
5.1677 × 10−8 |
↑ |
15 |
133.061 |
Asparagine |
−4.4289 |
HMDB0000168 |
6267 |
C00152 |
1.47652 |
0.0064085 |
↑ |
16 |
148.06096 |
Glutamate |
−4.337684462 |
HMDB0000148 |
33032 |
C00025 |
1.43134 |
0.000006964 |
↑ |
17 |
151.026 |
Xanthine |
1.280707727 |
HMDB0000292 |
1188 |
C00385 |
1.33306 |
0.000016751 |
↓ |
18 |
130.062 |
Creatine |
−5.594166769 |
HMDB0000064 |
586 |
C00300 |
1.2895 |
0.000033869 |
↓ |
19 |
133.014 |
Malic acid |
1.647021212 |
HMDB0000744 |
525 |
C00711 |
1.26188 |
0.000053201 |
↑ |
20 |
130.05 |
5-Aminolevulinic acid |
1.681785758 |
HMDB0001149 |
137 |
C00430 |
1.23992 |
0.000095042 |
↑ |
21 |
126.022 |
Taurine |
−4.388877692 |
HMDB0000251 |
1123 |
C00245 |
1.23244 |
0.000095896 |
↑ |
22 |
137.071 |
1-Methylnicotinamide |
−0.536136923 |
HMDB0000699 |
457 |
C02918 |
1.22002 |
0.00011278 |
↑ |
23 |
145.061 |
Alanine |
3.950847879 |
HMDB0000161 |
5950 |
C00041 |
1.19032 |
0.00022639 |
↓ |
24 |
96.9601 |
Sulfate |
−2.252080303 |
HMDB0001448 |
1117 |
C00059 |
1.16743 |
0.00029476 |
↓ |
25 |
176.103 |
Citrulline |
−3.005762462 |
HMDB0000904 |
9750 |
C00327 |
1.16532 |
0.00086161 |
↑ |
26 |
148.04325 |
Methionine |
−4.013574769 |
HMDB0000696 |
6137 |
C00073 |
1.1651 |
0.0003691 |
↓ |
27 |
167.021 |
Uric acid |
1.227497424 |
HMDB0000289 |
1175 |
C00366 |
1.15444 |
0.00030944 |
↓ |
28 |
191.01921 |
Citrate |
1.014883788 |
HMDB0000094 |
311 |
C00158 |
1.12963 |
0.0006675 |
↓ |
29 |
87.0088 |
Pyruvic acid |
−2.242745455 |
HMDB0000243 |
1060 |
C00022 |
1.1218 |
0.00052042 |
↓ |
30 |
115.00316 |
Fumarate |
1.752625303 |
HMDB0000134 |
444972 |
C00122 |
1.11808 |
0.00057395 |
↓ |
31 |
174.04 |
N-Acetyl-L-aspartate |
1.060201061 |
HMDB0000812 |
65065 |
C01042 |
1.11576 |
0.00063535 |
↓ |
32 |
129.05517 |
Ketoleucine |
2.232136818 |
HMDB0000695 |
70 |
C00233 |
1.11576 |
0.00063535 |
↓ |
33 |
118.08678 |
Valine |
−4.941874769 |
HMDB0000883 |
6287 |
C00183 |
1.10723 |
0.00056765 |
↓ |
34 |
118.062 |
Guanidineacetic acid |
−3.743986769 |
HMDB0000128 |
763 |
C00581 |
1.06017 |
0.0011203 |
↑ |
35 |
117.01881 |
Succinate |
2.459106364 |
HMDB0000254 |
1110 |
C00042 |
1.04523 |
0.0021343 |
↓ |
3.1.3. Metabolic pathway analysis of METH addiction related metabolites. Prior to metabolic pathway analysis of these 35 biomolecules shown above, the compound name standardization of them were performed by using the three following databases: The Human Metabolome Database (HMDB, http://www.hmdb.ca/), The PubChem Project (https://pubchem.ncbi.nlm.nih.gov/), Kyoto Encyclopedia of Genes and Genomes (KEGG, http://www.genome.jp/kegg/). They were all found in these three databases as shown by Table 1. According to the metabolic pathway impact analysis (Fig. 2A), the network included amino acid metabolisms (alanine, aspartate and glutamate metabolism, glycine, serine and threonine metabolism, taurine and hypotaurine metabolism, arginine and proline metabolism, glutamine and glutamate metabolism, valine, leucine and isoleucine biosynthesis, cysteine and methionine metabolism), citrate cycle (TCA cycle), butanoate metabolism, aminoacyl-tRNA biosynthesis, glycolysis, nicotinate and nicotinamide metabolism, purine metabolism and nitrogen metabolism. The enrichment analysis by using pathway-associated metabolite sets proved the consistence of this result (Fig. 2B), such as, urea cycle, ammonia recycling, alanine metabolism, and glucose–alanine cycle.
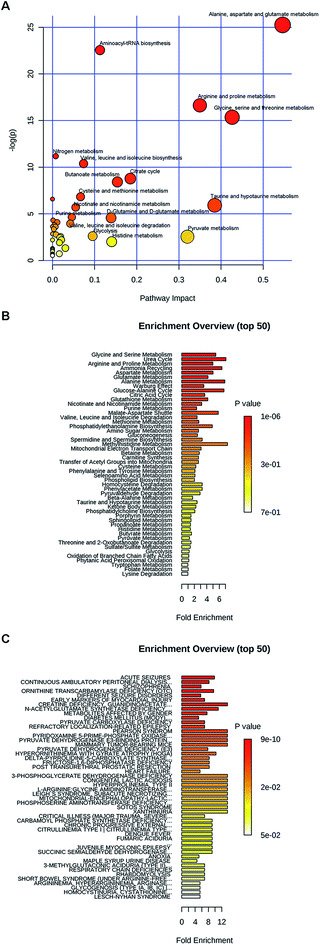 |
| Fig. 2 The impact of METH on serum metabolites of human. (A) The pathway impact of METH on serum metabolites of METH addicts. (B) Enrichment analysis of pathway-associated metabolite sets in METH addicts' serum. (C) Enrichment analysis of disease-associated metabolite sets (blood) in METH addict's serum. | |
Moreover, these metabolites were found to be involved in different diseases (Fig. 2C). For example, glutamate, glutamine, threonine, alanine, asparagine, citrulline, valine, methionine, isoleucine and histidine were all related to seizures. Histidine, threonine, valine, citrulline, glutamate, glutamine, ornithine, serine and taurine were involved into continuous ambulatory peritoneal dialysis. Citrulline, glycine, alanine, asparagine, glutamate, glutamine, isoleucine, serine, threonine, N-acetyl-L-aspartate and taurine were schizophrenia related metabolites. Alanine, choline, isoleucine, proline, serine, threonine and xanthine were early markers of myocardial injury.
3.2. MALDI-MSI of metabolites in METH-induced CPP rat brain
3.2.1. Evaluation of CPP model. There were different clues between A and B chambers. The animals spent same time in these two chambers during pre-conditioning test according to statistical analysis (A chamber: 260.58 ± 47.06, n = 12; B chamber: 290.33 ± 66.05, n = 12) (Fig. 3A), proving that they had no spatial preference before the conditioning test. METH injection in this study did not affect the animals moving ability, which was demonstrated by Fig. 3B. The shuttle frequency of the animals in METH group was the same as that of the SA control group (METH: 72 ± 20, n = 6; SA control: 64 ± 13, n = 6). And the animals in METH group shuttled as frequently as the SA control group. As shown by Fig. 3C, there was no difference in CPP scores between METH and SA control group in pre-conditioning test (METH: −79.60 ± 28.80, n = 6; SA control: −63.40 ± 59.22, n = 6), while the CPP score of the METH group was significantly higher than that of the SA control group after 6 days conditioning test (METH: 278.40 ± 91.59, n = 6; SA control: −103.40 ± 65.11, n = 6) (Fig. 3C). The animals of the METH group spent more time in nonpreferred chamber in post-conditioning test. Rat METH addiction model was established and METH enhanced drug-seeking memory of the METH group.
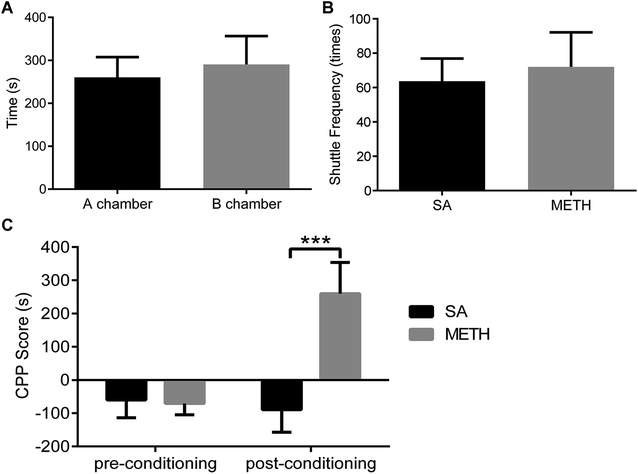 |
| Fig. 3 Evaluation of METH induced CPP rat model. (A) The animals spent same time in A and B chambers during pre-conditioning test (A chamber: 260.58 ± 47.06, n = 12; B chamber: 290.33 ± 66.05, n = 12). (B) METH group and the control group had the same shuttle frequency in post-conditioning test (METH: 72 ± 20, n = 6; SA control: 64 ± 13, n = 6). (C) There was no difference between these two groups in CPP score during pre-conditioning test (METH: −79.60 ± 28.80, n = 6; SA control: −63.40 ± 59.22, n = 6), while the CPP score of METH group increased significantly in post-conditioning test (METH: 278.40 ± 91.59, n = 6; SA control: −103.40 ± 65.11, n = 6). SA: control group; METH: METH group. | |
3.2.2. MALDI-MSI of CPP rat brain sections. 1,5-DAN hydrochloride based MALDI-MSI was applied to investigate the metabolite changes in METH induced CPP rat brain coronary sections. MALDI-MSI focused on three parts of the brain, NAc (Fig. 4), dHPC (Fig. 5) and vHPC (Fig. 6), which are three important regions of the rewarding pathway. 14 differently expressed metabolites were detected and mapped between METH and SA control groups, including energy metabolism related molecules, amino acid neurotransmitters, antioxidant, and phospholipids.
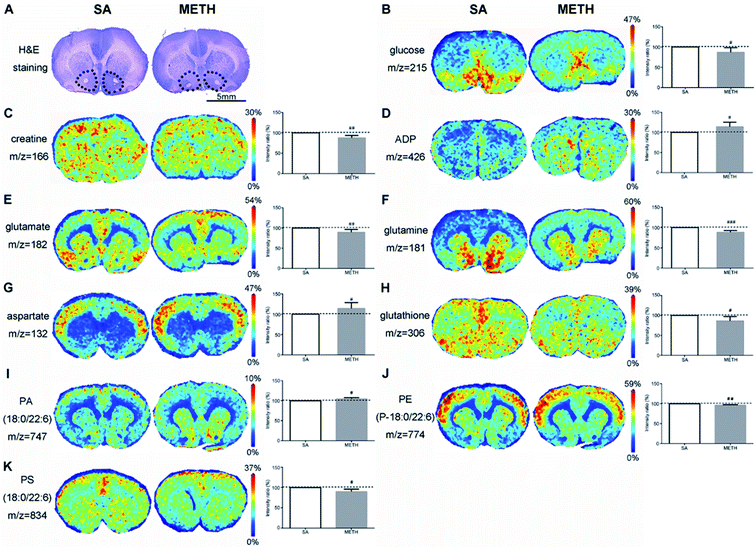 |
| Fig. 4 MALDI-MSI of metabolites changes in NAc of METH induced CPP rat brain (METH) and the SA control group (SA). (A) H&E staining of rat brain tissue adjacent to the one used for MALDI-MSI. NAc area was highlighted in black dots. Less glucose (B), creatine (C), glutamate (E), glutamine (F), glutathione (H), PE(P-18:0/22:6) (J), and PS(18:0/22:6) (K) were detected in NAc of the METH group than that of the SA control group. More ADP (D), aspartate (G) and PA(18:0/22:6) (I) were found in NAc of the METH group. The average signal intensity of the control group was set as 100% and the ratio of the average signal intensity between METH and control group was used for relative quantitation. n = 6; mean with SD; #p < 0.05; ##p < 0.01; ###p < 0.001. | |
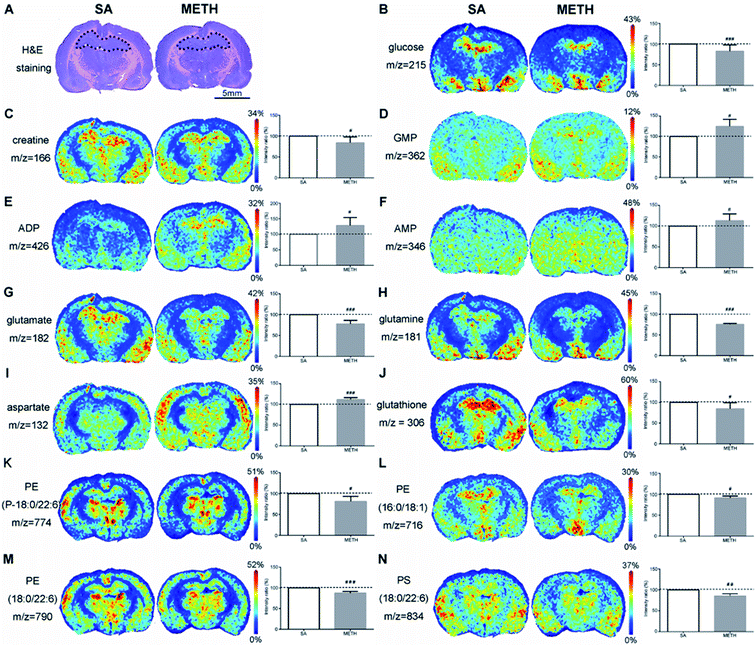 |
| Fig. 5 MALDI-MSI of metabolites changes in dHPC of METH induced CPP rat brain (METH) and the SA control group (SA). (A) H&E staining of rat brain tissue adjacent to the one used for MALDI-MSI. dHPC area was highlighted in black dots. Less glucose (B), creatine (C), glutamate (G), glutamine (H), glutathione (J), PE(P-18:0/22:6) (K), PE(16:0/18:1) (L), PE(18:0/22:6) (M), and PS(18:0/22:6) (N) were detected in dHPC of the METH group than that of the SA control group. More GMP (D), ADP (E), AMP (F), and aspartate (I) were found in dHPC of the METH group. The average signal intensity of the control group was set as 100% and the ratio of the average signal intensity between METH and control group was used for relative quantitation. n = 6; mean with SD; #p < 0.05; ##p < 0.01; ###p < 0.001. | |
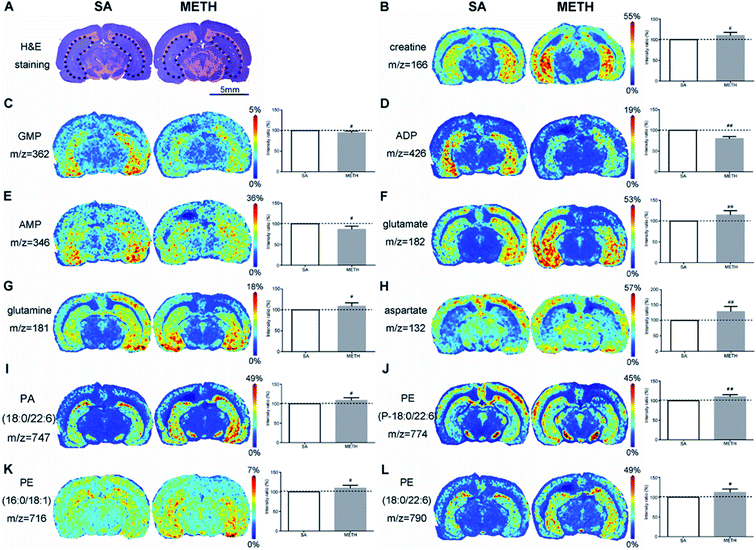 |
| Fig. 6 MALDI-MSI of metabolites changes in vHPC of METH induced CPP rat brain (METH) and the SA control group (SA). (A) H&E staining of rat brain tissue adjacent to the one used for MALDI-MSI. vHPC area was highlighted in black dots. More creatine (B), glutamate (F), glutamine (G), aspartate (H), PA(18:0/22:6) (I), PE(P-18:0/22:6) (J), PE(16:0/18:1) (K), and PE(18:0/22:6) (L) were found in vHPC of the METH group. Less GMP (C), ADP (D), and AMP (E) were detected in vHPC of the METH group than that of the SA control group. The average signal intensity of the control group was set as 100% and the ratio of the average signal intensity between METH and control group was used for relative quantitation. n = 6; mean with SD; #p < 0.05; ##p < 0.01. | |
In NAc found by H&E staining (Fig. 4A), there were clear decreased glucose and creatine in METH group (Fig. 4B and C), while the concentration of ADP was significantly increased (Fig. 4D). The concentration of three amino acids were also different between these two groups. Less glutamate and glutamine were detected in METH group, while the signal of aspartate in METH was stronger (Fig. 4E–G). METH caused lower expression of glutathione in NAc (Fig. 4H). In addition, phosphatidic acid (PA), phosphatidylethanolamine (PE), and phosphatidylserine (PS) were mapped in NAc. PA(18:0/22:6) were higher expressed in NAc in METH induced CPP rat brain (Fig. 4I), while PE(P-18:0/22:6) and PS(18:0/22:6) were both decreased in NAc after METH addiction (Fig. 4J and K).
By using H&E staining, dHPC (Fig. 5A) and vHPC (Fig. 6A) were found. In dHPC, glucose, creatine, were clearly decreased in METH group (Fig. 5B and C). But there were more GMP, ADP, and AMP e in dHPC after receiving METH (Fig. 5D–F). However, the expression pattern of creatine, GMP, ADP, AMP were different in vHPC. The METH group had more creatine but less GMP, ADP and AMP in vHPC (Fig. 6B–E). When it comes to the changes of amino acids, glutamate and glutamine were decreased but aspartate increased in dHPC (Fig. 5G–I). But stronger signals of glutamate, glutamine and aspartate were got in vHPC (Fig. 6F–H). A lower concentration of antioxidant glutathione was only found in dHPC (Fig. 5J). Moreover, decreased PE(P-18:0/22:6), PE(16:0/18:1), PE(18:0/22:6) and PS(18:0/22:6) were observed in dHPC of the METH group (Fig. 5K–N), while PA(18:0/22:6) (Fig. 6I) and those three PEs were all increased in vHPC (Fig. 6J–L).
4. Discussion
Rising number of studies have been reported about the relationship between brain alternations and cognitive function in METH abusers.1,3,34 However, knowledge about METH dependence/addiction metabolomics is still rare, especially about human. Via mass spectrometry-based metabolomics experiments on rats treated with METH at 10 mg kg−1 h−1 for 4 h, only increased glucose and decreased 3-hydroxybutyrate were detected in the samples after 24 h of METH treatment. Their levels recovered to normal after 96 h.14 Kei Zaitsu et al. performed metabolic profiling of blood plasma in METH induced CPP rat model and found upregulated n-propylamine and downregulated lauric acid.35 Distinct perturbations of 35 metabolites were observed in the serum of rats after the injection with METH at escalating doses of mg kg−1 for 5 consecutive days, whereas the withdrawal of METH restored most of these biomolecules metabolic patterns to baseline.13 In this study, metabolomic analysis of METH addicts' serum was performed initially.
To our knowledge, this is the first report about metabolomics profiling of human serum samples of METH addiction with 35 differently expressed metabolites identified, including 5 TCA intermediates, 17 amino acids and 13 other biomolecules. 20 of these 35 metabolites have been reported in rat serum after METH administration.13 Decreased threonine, glycine, serine, isoleucine, proline, alanine, methionine, citrate, ketoleucine, valine, pyruvic acid, fumarate, succinate, and increased glutamate were observed in the serum of both human METH abusers here and rat in previous reports. But the changes of lactate, ornithine, creatinine, asparagine, taurine, citrulline were different between human and rat, and the remaining 15 metabolites including choline, succinate semialdehyde, glutamine, niacinamide, histidine, acetylcholine, xanthine, creatine, malic acid, 5-aminolevulinic acid, 1-methylnicotinamide, sulfate, uric acid, N-acetyl-L-aspartate, guanidineacetic acid have never been reported in animal models, which may be caused by the species variation. The dose regiment or sampling scheme used in animal metabolomics research could not match well with our human samples got from patients at abstinence stage either. The identification of these 15 metabolites enriched the knowledge about human METH dependence/addiction, whereas further study is required to validate this finding. The discussion below will be primarily focused on the molecules reported before and here.
By using metabolic pathway analysis, the differently expressed metabolites were found to have responses to a range of metabolisms. The result was consistent with that obtained in other animal experiments. For example, alanine, aspartate and glutamate metabolism, arginine and proline metabolism, glutamine and glutamate metabolism, citrate cycle were the primary metabolic pathways altered following METH exposure in human and rat.12 The enrichment analysis of disease-associated metabolite sets demonstrated that glutamate, glutamine, threonine, alanine, asparagine, citrulline, and isoleucine were all related to both epilepsy and schizophrenia. And threonine, alanine, and isoleucine were also early markers of myocardial injury. On the one hand, it proved that METH abuse may induce nerve disease, psychiatric disorder, or cardiovascular disease. On the other hand, we had to admit that these metabolites were not specific to METH addiction. Although the subjects were carefully selected in order to eliminate the influence of other factors and make sure these two groups were mainly distinguished by METH administration, the sample size of this study was small and the potential markers could be affected by many things. A validation set containing a larger sample size is required to assess their sensitivity and stability in the future. However, our identified metabolites at the abstinence stage should be candidates for the diagnosis of METH addiction. Xanthine is in the opioids abuse diagnosis package.36 Glutamate and aspartate in the plasma of Alzheimer's disease patient are associated with hippocampal volume and memory, as well as amyloidosis.37 Recent drug exposure can be detected by trace of METH, but it is still urgently needed to develop additional peripheral biomarkers to diagnose the severity of drug addiction, to monitor therapeutic efficacy, and to predict treatment response.
Due to the limitation of material and ethical regulation for human, it is almost impossible to simultaneously detect and characterize of the spatial distribution and relative abundance of various metabolites in the human brain. MALDI-MSI was used to investigate the alteration and spatial distribution of characteristic molecules in the brain of METH induced CPP rat models. And comparative analysis of metabolites changes among different brain areas has been performed. The results achieved from animal model could also be helpful to explore the mechanism of METH addiction. The metabolites influenced by METH administration in CPP rat brain included energy metabolism related molecules, amino acid neurotransmitters, antioxidant, and phospholipids. Some of them were also listed in the human metabolomics profiling result.
Energy metabolism related molecules are important group of metabolites affected by METH. In rat model, the sera levels of citrate, pyruvic acid, fumarate, and succinate were decreased after the first intraperitoneal injection of METH, but few of them were restored to normal levels after 5 continuous days METH administration.13 The decreases of these four TCA intermediates were found here in the serum of chronic METH abusers. Their dynamic changes in human require systematic study in a larger population comprised of people in different status of METH dependence/addiction. It has been reported that the nutritional supplement creatine monohydrate can be used to reduce symptoms of depression and increase brain phosphocreatine in healthy volunteers.38 The lower level of creatine was detected in NAc and dHPC, as well as glucose. There is a large amount of creatine distributed in the brain, which can be transformed with creatine phosphate and crucial to maintain ATP metabolism stability. But an increase of creatine was found in vHPC. The mechanism of creatine changes in different areas induced by METH requires deeper analysis.
The activation of neurons stimulated by METH require a large consumption of ATP. Meanwhile, evidence indicated that energetic metabolism in mitochondria was deregulated following exposure to METH.39 After 1 h of 2 mg kg−1 METH injection, ATP was significantly decreased in rat brain with increases of ADP, adenosine and lactate.40 More ADP, GMP and AMP in NAc and dHPC mean more energy consumed in these two regions after METH administration. The ADP, GMP and AMP in vHPC may be used to generate ATP to supply energy for other regions of the brain, such as NAc and dHPC, which led to the decrease of these three metabolites in vHPC. The different changes of energy metabolism related molecules in NAc, dHPC and vHPC suggested that NAc and dHPC should be more susceptible to METH treatment. This hypothesis requires further research.
Earlier studies demonstrated that METH administration could perturb amino acid metabolism.13 By using metabolomic analysis of rat serum13 and neuroblastoma cells,41 amino acids were the most notable metabolites affected by METH, including glutamate, aspartate, methionine, phenylalanine and serine. Summarizing the results of METH associated metabolomics profiling, 13 amino acids were decreased in the serum of rat model except glutamate,12 but glutamine, ornithine, histidine, asparagine, glutamate, taurine, and citrulline were increased in the serum of human METH abusers. As the most ubiquitous neurotransmitter, glutamate mediates the major excitatory processes of learning, memory, and retention of learned cues and events.42 The release of glutamate from neurons is a key neurotoxic mechanism of METH, because increased glutamate could cause oxidative stress by inducing the build-up of reactive oxygen species.42 The higher level of glutamate, glutamine in the abuser serum suggested an increased nervous system activity. Furthermore, glycine and alanine are inhibitory neurotransmitters in the brain. Although their changes were not observed in rat brain, their decrease in the serum may be an evidence that METH reduced the inhibition of nervous system activity. Here, the increased glutamate and glutamine, as well as decreased glycine and alanine were observed in the serum of METH abusers, which was consistent with reported study and provided new evidence that altering glutamate, glycine, and alanine metabolism should be one of the mechanisms of METH disturbing the nervous system activity.
The studies about amino acids in the brain after METH treatment were mainly about glutamate and glutamine. Their changes were complicated in different brain regions. Repeated administration of METH increased the extracellular concentration of glutamate in the anteromedial striatum of rat.43 In medial prefrontal cortex and NAc of rat, glutamate level was reduced after approximately two weeks following repeated stimulant administration.44 During early abstinence, there was lower overall glutamate and glutamine concentrations in frontal gray and white matter of human brain.45,46 But there was an inverse trend after 5 months to correlate the glutamate and glutamine concentrations in frontal gray matter with the duration of abstinence.45 White et al. found acute use of METH increased the level of glutamate and glutamine significantly in the dorsal anterior cingulate cortex of female.42 By using magnetic resonance spectroscopic imaging, it has been found that in right inferior frontal cortex and right insula, glutamate + glutamine was lower in METH users than in controls and correlated negatively with depressive symptoms.47 Here, with the application of MALDI-MSI, there were less glutamate or glutamine detected in NAc and dHPC in METH group, while increased glutamate and glutamine were observed in vHPC after METH administration. The inconsistence of glutamate and glutamine expression in different regions reflect METH work on multiple points of the glutamatergic metabolic pathway, such as synthesis, catabolism, transport and release. Here, another excitatory neurotransmitter aspartate was identified. The increase of aspartate was consistently observed in three brain regions of METH addiction rat. Together with reported evidence13,41 and the results obtained in this study, the expressions of glutamate, glutamine and aspartate seem to be more easily to be affected by METH exposure, which have the potential to be METH peripheral biomarkers.
It has been confirmed that METH could induce mitochondrial impairment and neuroinflammation. NAc and HPC are the first two regions affected by METH.48 Antioxidants play important role to eliminate oxidative stress and inflammation. Glutathione could get rid of reactive oxygen species directly to protect brain tissues from damage.49 Here, decreased glutathione was found in NAc and dHPC, which can be explained by easing the METH induced oxidative stress. This is corresponding to changes of energy metabolism related molecules in NAc and dHPC mentioned above, which can be new evidence that the impairment caused by METH has region specificity or different brain areas have diverse ability against METH. And antioxidants were suggested to have therapeutic potential for METH associated neurotoxicity.
Phospholipids are basic structural components of the neurons and glial cells, the target of addictive substances.50 PA, as a kind of cytoskeletal component, plays an important role in the regulation of intracellular signalling and cellular functions, such as dendritic spine formation and maturation.51 The upregulation of PA(18:0/22:6) in detected in vHPC in this study suggest that PA should be involved in the cytoskeletal reorganization and METH induced CPP memory consolidation. PE-binding protein (PEBP) was up-regulated in rat hippocampus after chronic morphine administration, indicating that the protein is functional in addiction.52 However, no direct evidence has been reported to certify the relationship between PE and METH addiction. Here, the decreased PEs found in NAc and dHPC may be caused by PEBP and prove that these two regions are more susceptible to METH administration than vHPC. PS is another critical component of healthy nerve cell membrane and supports human cognitive functions.53 The decrease of PS(18:0/22:6) in NAc and dHPC should be caused by their consumption to consolidate memory. The mapping of phospholipids provided new insight into the mechanism of METH addiction.
By comparative analysis of the metabolites changes, it was shown that the changes of many metabolistes, including glucose, creatine, ADP, glutamate, glutamine, aspartate, glutathione, PE(P-18:0/22:6), PS(18:0/22:6), were the same in both NAc and dHPC. But the changes of creatine, ADP, glutamate, glutamine, aspartate, and PE(P-18:0/22:6) were different between NAc/dHPC and vHPC. Especially, the changes of metabolites detected in both dHPC and vHPC were almost opposite except aspartate. The MALDI-MSI results provided new evidence that these three brain subregions should play different roles in METH addiction.
5. Conclusions
In conclusion, this study not only confirmed previous finding but also enriched the knowledge about METH addiction metabolomics. Firstly, 35 METH addiction related metabolites have been identified via untargeted metabolomics analysis in human serum, which included 20 previously reported molecules and 15 reported in the current study. Larger scale of validation set is required to check the specificity and sensitivity of these metabolites used for METH addiction degree assessment. If possible, it is recommended to establish a METH abuser serum sample library for systematic study. And further research should be focused on the metabolic pathway predicted here to study the mechanism of METH addiction. Moreover, with the application of MALDI-MSI, it is possible to analyse metabolite changes in drug dependence/addiction related substructure of the brain. Here, energy metabolism related molecules, neurotransmitter amino acids, antioxidant and phospholipids were mapped in several regions of METH induced CPP rat brain. A multi-parameter model consist of these related metabolites can be established as METH addiction biomarker in the future. The changes of phospholipids pointed a new direction that attention should also be given to phospholipids metabolic pathway to clarify their roles in METH addiction. Finally, according to comparative analysis, NAc and dHPC are likely to be more susceptible to METH administration, and dHPC and vHPC seem to play different roles in METH addiction.
Author contributions
Conceived the study: M. L., J. X. and X. P.; designed the study: M. L., J. X. and X. P.; performed the study: M. L., J. X.; analysed data: M. L., J. X.; sample collection: X. L., Z. D., Z. L.; writing – original draft preparation: M. L.; writing – review and editing: M. L., J. X., Y. Z., Y. S., X. P.; supervision: X. P.; funding acquisition: X. P., M. L.
Conflicts of interest
Authors declare that they do not have any conflicts of interests.
Acknowledgements
Funding: This research was funded by the National Natural Science Foundation of China, grant numbers 81571354, 81801364, and China Postdoctoral Science Foundation, grant numbers 2018M631277. General: We also thank support from Yupei Jiao and Lina Xu from the Metabolomics Facility at the Technology Center for Protein Sciences of Tsinghua University and Dr Zhiyong Du from School of Pharmaceutical Sciences of Peking University.
References
- R. Yasaei and A. Saadabadi, in StatPearls, StatPearls Publishing LLC, Treasure Island (FL), 2019 Search PubMed.
- T. J. Abbruscato and P. C. Trippier, ACS Chem. Neurosci., 2018, 9, 2373–2378 CrossRef CAS PubMed.
- H. Mizoguchi and K. Yamada, Neurochem. Int., 2019, 124, 106–113 CrossRef CAS.
- S. Yu, L. Zhu, Q. Shen, X. Bai and X. Di, Behav. Neurol., 2015, 2015, 103969 Search PubMed.
- J. R. Richards, A. Waheed and E. G. Laurin, in StatPearls, StatPearls Publishing LLC, Treasure Island (FL), 2019 Search PubMed.
- U. Busto, R. Bendayan and E. M. Sellers, Clin. Pharmacokinet., 1989, 16, 1–26 CrossRef CAS.
- W. Shen, H. Liu, X. Xie, H. Liu and W. Zhou, Adv. Exp. Med. Biol., 2017, 1010, 169–202 CrossRef PubMed.
- C. H. Johnson, J. Ivanisevic and G. Siuzdak, Nat. Rev. Mol. Cell Biol., 2016, 17, 451–459 CrossRef CAS PubMed.
- H. Li, C. Li, Y. Zhou, C. Luo, J. Ou, J. Li and Z. Mo, Exp. Ther. Med., 2018, 15, 3369–3375 Search PubMed.
- W. L. Shi, X. Zhao, Z. M. Liu, M. Zhang, B. Y. Zhou and X. P. Pu, Neurosci. Lett., 2012, 525, 23–28 CrossRef CAS.
- S. X. Li, S. Y. Yan, Y. P. Bao, Z. Lian, Z. Qu, Y. P. Wu and Z. M. Liu, Hum. Psychopharmacol., 2013, 28, 477–483 CrossRef CAS PubMed.
- M. Kim, W. J. Jang, R. Shakya, B. Choi, C. H. Jeong and S. Lee, Metabolites, 2019, 9, 195 CrossRef CAS PubMed.
- T. Zheng, L. Liu, J. Shi, X. Yu, W. Xiao, R. Sun, Y. Zhou, J. Aa and G. Wang, Mol. BioSyst., 2014, 10, 1968–1977 RSC.
- N. Shima, I. Miyawaki, K. Bando, H. Horie, K. Zaitsu, M. Katagi, T. Bamba, H. Tsuchihashi and E. Fukusaki, Toxicology, 2011, 287, 29–37 CrossRef CAS PubMed.
- T. C. Luehr, E. M. Koide, X. Wang, J. Han, C. H. Borchers and C. C. Helbing, Gen. Comp. Endocrinol., 2018, 265, 237–245 CrossRef CAS PubMed.
- J. Kriegsmann, M. Kriegsmann and R. Casadonte, Int. J. Oncol., 2015, 46, 893–906 CrossRef CAS PubMed.
- M. L. Reyzer and R. M. Caprioli, Curr. Opin. Chem. Biol., 2007, 11, 29–35 CrossRef CAS PubMed.
- A. Thomas, S. Lenglet, P. Chaurand, J. Deglon, P. Mangin, F. Mach, S. Steffens, J. L. Wolfender and C. Staub, Thromb. Haemostasis, 2011, 106, 20–33 CrossRef CAS PubMed.
- R. A. McClure, C. W. Chumbley, M. L. Reyzer, K. Wilson, R. M. Caprioli, J. C. Gore and W. Pham, NeuroImage Clin., 2013, 2, 620–629 CrossRef PubMed.
- M. Kriegsmann, R. Casadonte, T. Randau, S. Gravius, P. Pennekamp, A. Strauss, J. Oldenburg, K. Wieczorek, S. O. Deininger, M. Otto and J. Kriegsmann, Haemophilia, 2014, 20, 446–453 CrossRef CAS PubMed.
- V. Mainini, F. Pagni, F. Ferrario, F. Pieruzzi, M. Grasso, A. Stella, G. Cattoretti and F. Magni, Histopathology, 2014, 64, 901–906 CrossRef PubMed.
- M. Lagarrigue, M. Becker, R. Lavigne, S. O. Deininger, A. Walch, F. Aubry, D. Suckau and C. Pineau, Mol. Cell. Proteomics, 2011, 10, M110.005991 CrossRef PubMed.
- E. E. Jones, T. W. Powers, B. A. Neely, L. H. Cazares, D. A. Troyer, A. S. Parker and R. R. Drake, Proteomics, 2014, 14, 924–935 CrossRef CAS PubMed.
- M. A. Rodrigo, O. Zitka, S. Krizkova, A. Moulick, V. Adam and R. Kizek, J. Pharm. Biomed. Anal., 2014, 95, 245–255 CrossRef PubMed.
- S. Steurer, A. S. Seddiqi, J. M. Singer, A. S. Bahar, C. Eichelberg, M. Rink, R. Dahlem, H. Huland, G. Sauter, R. Simon, S. Minner, E. Burandt, P. R. Stahl, T. Schlomm, M. Wurlitzer and H. Schluter, Anticancer Res., 2014, 34, 2255–2261 Search PubMed.
- M. Yuan, S. B. Breitkopf, X. Yang and J. M. Asara, Nat. Protoc., 2012, 7, 872–881 CrossRef CAS PubMed.
- Y. Tian, Z. Wang, X. Liu, J. Duan, G. Feng, Y. Yin, J. Gu, Z. Chen, S. Gao, H. Bai, R. Wan, J. Jiang, J. Liu, C. Zhang, D. Wang, J. Han, X. Zhang, L. Cai, J. He and J. Wang, Clin. Cancer Res., 2018, 24, 2100–2109 CrossRef CAS PubMed.
- H. Tang, X. Wang, L. Xu, X. Ran, X. Li, L. Chen, X. Zhao, H. Deng and X. Liu, Talanta, 2016, 156–157, 163–171 CrossRef CAS PubMed.
- J. Xia and D. S. Wishart, Curr. Protoc. Bioinf., 2016, 55, 14 10 11–14 10 91 Search PubMed.
- J. Chong, O. Soufan, C. Li, I. Caraus, S. Li, G. Bourque, D. S. Wishart and J. Xia, Nucleic Acids Res., 2018, 46, W486–W494 CrossRef CAS PubMed.
- P. George and W. Charles, The rat brain in stereotaxic coordinates, Academic Press, 6th edn, 2007 Search PubMed.
- H. Liu, R. Chen, J. Wang, S. Chen, C. Xiong, J. Wang, J. Hou, Q. He, N. Zhang, Z. Nie and L. Mao, Anal. Chem., 2014, 86, 10114–10121 CrossRef CAS PubMed.
- T. Chen, P. He, Y. Tan and D. Xu, Biochem. Biophys. Res. Commun., 2017, 485, 119–125 CrossRef CAS PubMed.
- S. Sabrini, G. Y. Wang, J. C. Lin, J. K. Ian and L. E. Curley, Drug Alcohol Depend., 2019, 194, 75–87 CrossRef CAS PubMed.
- K. Zaitsu, I. Miyawaki, K. Bando, H. Horie, N. Shima, M. Katagi, M. Tatsuno, T. Bamba, T. Sato, A. Ishii, H. Tsuchihashi, K. Suzuki and E. Fukusaki, Anal. Bioanal. Chem., 2014, 406, 1339–1354 CrossRef CAS PubMed.
- A. A. Patkar, S. Rozen, P. Mannelli, W. Matson, C. U. Pae, K. R. Krishnan and R. Kaddurah-Daouk, Psychopharmacology, 2009, 206, 479–489 CrossRef CAS PubMed.
- M. Kim, S. Snowden, T. Suvitaival, A. Ali, D. J. Merkler, T. Ahmad, S. Westwood, A. Baird, P. Proitsi, A. Nevado-Holgado, A. Hye, I. Bos, S. Vos, R. Vandenberghe, C. Teunissen, M. Ten Kate, P. Scheltens, S. Gabel, K. Meersmans, O. Blin, J. Richardson, E. De Roeck, K. Sleegers, R. Bordet, L. Rami, P. Kettunen, M. Tsolaki, F. Verhey, I. Sala, A. Lleo, G. Peyratout, M. Tainta, P. Johannsen, Y. Freund-Levi, L. Frolich, V. Dobricic, S. Engelborghs, G. B. Frisoni, J. L. Molinuevo, A. Wallin, J. Popp, P. Martinez-Lage, L. Bertram, F. Barkhof, N. Ashton, K. Blennow, H. Zetterberg, J. Streffer, P. J. Visser, S. Lovestone and C. Legido-Quigley, Alzheimer's Dementia, 2019, 15, 817–827 CrossRef PubMed.
- T. L. Hellem, Y. H. Sung, X. F. Shi, M. A. Pett, G. Latendresse, J. Morgan, R. S. Huber, D. Kuykendall, K. J. Lundberg and P. F. Renshaw, J. Dual Diagnosis, 2015, 11, 189–202 CrossRef PubMed.
- R. Moratalla, A. Khairnar, N. Simola, N. Granado, J. R. Garcia-Montes, P. F. Porceddu, Y. Tizabi, G. Costa and M. Morelli, Prog. Neurobiol., 2017, 155, 149–170 CrossRef CAS PubMed.
- T. Shiba, M. Yamato, W. Kudo, T. Watanabe, H. Utsumi and K. Yamada, NeuroImage, 2011, 57, 866–872 CrossRef CAS PubMed.
- G. L. Maker, T. Green, I. Mullaney and R. D. Trengove, Metabolites, 2018, 8, 38 CrossRef.
- T. L. White, M. A. Monnig, E. G. Walsh, A. Z. Nitenson, A. D. Harris, R. A. Cohen, E. C. Porges, A. J. Woods, D. G. Lamb, C. A. Boyd and S. Fekir, Neuropsychopharmacology, 2018, 43, 1498–1509 CrossRef CAS PubMed.
- J. F. Nash and B. K. Yamamoto, Brain Res., 1992, 581, 237–243 CrossRef CAS PubMed.
- A. A. Herrold, R. M. Voigt and T. C. Napier, Eur. Neuropsychopharmacol., 2013, 23, 691–696 CrossRef CAS PubMed.
- T. Ernst and L. Chang, J. Neuroimmune Pharmacol., 2008, 3, 165–172 CrossRef PubMed.
- J. O'Neill, M. C. Tobias, M. Hudkins and E. D. London, Int. J. Neuropsychopharmacol., 2014, 18, pyu059 CrossRef PubMed.
- J. Tang, J. O'Neill, J. R. Alger, Z. Shen, M. C. Johnson and E. D. London, Int. J. Neuropsychopharmacol., 2019, 22, 1–9 CrossRef CAS PubMed.
- E. J. Shin, D. K. Dang, T. V. Tran, H.
Q. Tran, J. H. Jeong, S. Y. Nah, C. G. Jang, K. Yamada, T. Nabeshima and H. C. Kim, Arch. Pharmacal Res., 2017, 40, 403–428 CrossRef CAS PubMed.
- M. T. Islam, Neurol. Res., 2017, 39, 73–82 CrossRef CAS PubMed.
- A. Bodzon-Kulakowska, A. Antolak, A. Drabik, M. Marszalek-Grabska, J. Kotlinska and P. Suder, Biochim. Biophys. Acta, Mol. Cell Biol. Lipids, 2017, 1862, 686–691 CrossRef CAS PubMed.
- M. R. Ammar, N. Kassas, M. F. Bader and N. Vitale, Biochimie, 2014, 107, 51–57 CrossRef CAS PubMed.
- C. Atmanene, A. Laux, E. Glattard, A. Muller, F. Schoentgen, M. H. Metz-Boutigue, D. Aunis, A. Van Dorsselaer, G. B. Stefano, S. Sanglier-Cianferani and Y. Goumon, Med. Sci. Monit., 2009, 15, Br178–Br187 CAS.
- M. J. Glade and K. Smith, Nutrition, 2015, 31, 781–786 CrossRef CAS.
Footnote |
† Both of the authors are listed as co-first authors and contributed equally to this work. |
|
This journal is © The Royal Society of Chemistry 2019 |
Click here to see how this site uses Cookies. View our privacy policy here.