DOI:
10.1039/C9RA07382E
(Paper)
RSC Adv., 2019,
9, 35429-35442
Untargeted metabolomics using liquid chromatography coupled with mass spectrometry for rapid discovery of metabolite biomarkers to reveal therapeutic effects of Psoralea corylifolia seeds against osteoporosis†
Received
13th September 2019
, Accepted 23rd October 2019
First published on 1st November 2019
Abstract
Liquid chromatography coupled with mass spectrometry has been used as metabolomics profiling tool to discover and identify the metabolites in metabolic diseases. Osteoporosis (OP) syndrome is a chronic metabolic disease characterized by bone mass reduction and changes in bone microstructure. Psoralea corylifolia Linn. seeds (PCS) have a therapeutic effect on osteoporosis, but their action mechanism and therapeutic target are still unclear. This study aims to explore the metabolic changes of the urine profile in glucocorticoid-induced OP model rats and the therapeutic effect of PCS. High-throughput metabolomics based on the liquid chromatography coupled with mass spectrometry quadrupole time-of-flight mass spectrometry and multivariate data analysis were used to analyze the urine metabolites. The results showed that has an obvious separation between model and control groups. OPLS-DA was used to further analyze and discover substances that contributed to the separation. 42 potential biomarkers and 12 related metabolic pathways were identified in combination with network databases. After the intervention of PCS, 24 biomarkers were significantly regulated, mainly with glycone, serine and threonine metabolism, glutathione metabolism and purine metabolism and other metabolic pathways are related and discovered. This study has proved that PCS has therapeutic effect against OP by regulating that metabolic pathways disturbed in the OP. It provided a basis for the research and future development of new drugs for OP treatment.
1. Introduction
With the gradual improvement of people's living standards and the continuous extension of life expectancy, the research on endocrine and metabolic diseases has become an important medical research direction in this century.1 Osteoporosis (OP) is a systemic and systemic chronic bone metabolic disease feature by decreased bone mass, increased bone fragility and bone microstructural damage, and fracture risk in patients with osteoporosis increase.2–4 OP can occur in different genders and at any age, but it is mostly found in postmenopausal women and elderly men.5 According to epidemiological statistics, the incidence of primary osteoporosis in the world is increasing year by year, which has already had a serious impact on the quality of life and physical health of the elderly, and has brought enormous economic pressure on families and society,6,7 It has become a public health problem that seriously jeopardizes the health of the elderly. The main pathological basis of the disease is the decline in osteoblast capacity and the ability of osteoclasts.8 Therefore, the development of corresponding inhibition and enhancement drugs for these two cases is of direct significance for the prevention and treatment of OP.9,10 However, its etiology and pathogenesis are still not completely clear, and the treatment and prevention measures are still not perfect. In recent years, many researchers have made remarkable progress in studying the treatment of OP with herbal medicine from the level of molecular biology. More and more basic researches have confirmed that traditional Chinese medicine has obvious effect in treating OP.11
Psoralea corylifolia Linn., seen in “Kai bao Materia Medica”, the modern research has proved that Psoralea corylifolia Linn. seeds (PCS) contains many chemical components, such as coumarins, flavonoids, monoterpene phenols and other components.12,13 Modern pharmacological studies show that it has a variety of pharmacological activities, such as anti-cancer activity, improvement of immune system function, estrogen-like effect, promotion of bone regeneration and reconstruction, antibacterial activity and treatment of psoriasis.14 Coumarin is the main active substance in anti-osteoporosis treatment. Coumarin compounds can be divided into 2 categories: (1) furocoumarin compounds,15 including psoralen, isopsoralen, 8-methoxypsoralen, psoralenglucoside, isopsoralenglucoside, etc.,16 of which psoralen and isopsoralen have been studied extensively and deeply; (2) pseudo-estriol compounds, including psoralen, isopsoralen, dihydroxyisopsoralen, neopsoralen, etc.17 PCS has a long history of cure of osteoporosis and is reflected in many clinical prescriptions.18 Pharmacology proves that PCS has estrogenic effect, and scholars have isolated PCS, an active ingredient with obvious estrogenic effect, from PCS.19 In recent years, the treatment of osteoporosis by PCS has become a hot spot in the basic research field of orthopaedics.20,21
Metabolomics is the key technology and acting method in system biology research,22 It has the characteristics of integration, dynamics, synthesis and analysis in methodology.23,24 Metabolic fingerprints containing metabolic product information are obtained through a series of data acquisition methods, and the information is mined and extracted by the method of multivariate data analysis to analyze all metabolic responses of endogenous metabolites in organisms to changes in time after being stimulated by interference such as pathological, physiological changes or gene variations, thus providing us with relevant information on drug action mechanism and action sites, further exploring the internal metabolic circulation pathway and signal pathway of diseases, and providing possibility for exploring the pathogenesis and mechanism of diseases.25–27 Ultra performance liquid chromatography-quadrupole-time-of-flight mass spectrometry (UPLC-Q-TOF/MS) has become a commonly used technique in metabolomics due to its high resolution, high resolution, high sensitivity, etc. However, the MSE mass spectrum acquisition function uses high and low collision energies to scan alternately in parallel so as to obtain precursor ion information (MS) at low collision energies or accurate mass fragments with complete scanning at high collision energies.28 The precursor ion and neutral loss information (MSE) can provide information similar to conventional MS2 (MS/MS) in one analysis.29 It provides structural information needed to identify unknown biomarkers under the background of non-target analysis, and provides a powerful technical means for rapid identification of trace components.30,31 In recent years, UPLC-Q-TOF/MS technology has been widely applied to the analysis of complex components in Chinese medicine,32–34 the analysis of components in medicine and health products,35 the analysis of complex components in vitro36 and metabolites in vivo such as drug metabolism and disease metabolism,37 it has become an indispensable means in the fields of food and drug safety and drug analysis.38 Understanding the characteristic patterns of metabolism can also help people to know more about the pathological process of diseases and broaden their understanding of diseases.
Based on the high-throughput metabolomics research platform, this study intends to establish a rat osteoporosis model by glucocorticoid stimulation. By comparing the metabolic spectrum changes between model rats and control rats, we will look for urine biomarkers related to glucocorticoid-induced osteoporosis. After treatment with PCS, we will explore the potential urine metabolic targets and mechanisms of PCS for preventing glucocorticoid-induced osteoporosis.
2. Experiments
2.1. Drugs and reagents
Prednisolone (purity 98.81%) was offered of Wuhan shunda fine chemical co., ltd (Wuhan, China). Acetonitrile, methanol is provided by German Merck Company (chromatographic grade, Merck, Germany). Leucine enkephalin was obtained from Sigma-Aldrich (St. Louis, MO, USA).
2.2. Animals
Animal experiments were performed in accordance with the animal experiment guidelines of Taizhou University Hospital, China. Thirty SPF healthy male SD rats (weighing 200–250 g) were provided by the Laboratory Animal Center. All rats were raised in a standard environment (temperature: 18–25 °C, humidity: 45–60%, light/dark cycles alternate every 12 hours). During the whole experiment, all animals were allowed to eat freely and take water.
2.3. Drug preparation
PCS (Fig. S1†) was purchased from Harbin branch of Beijing Tong Ren Tang and identified as the dried fruit of Psoralea corylifolia Linn. The sample (number 2018-361) has been deposited in Herbarium of Taizhou Central Hospital, Taizhou University Hospital, China. Drying the medicinal materials, pulverizing, taking appropriate amount of PCS medicinal powder, refluxing and extracting with 12 times of 75% ethanol for 3 times, filtering, mixing filtrates, recovering to dryness under reduced pressure, adding 0.5% CMC-Na to prepare 1.5 g mL−1 gastric lavage agent (calculated according to the amount of PCS medicinal material), and storing at 4 °C for later use. The main components of PCS were further analyzed and quantitatively determined by ultra-performance liquid chromatography and the HPLC chromatogram of the standard mixture and samples is shown in Fig. S2.†
2.4. Preparation of osteoporosis rat model
All rats were acclimated to the environment for one week, and then divided into three groups according to the principle of weight balance, which were the normal control group, the model group and the treatment group. Except the normal control group, each group of rats was intramuscularly injected with prednisolone (15 mg kg−1) twice a week for 12 weeks. Animals were free to eat and drink water during the experiment. At the same time of model replication, the treatment group was given 75% ethanol extract of PCS by gavage every day, and the dosage was 10 g kg−1 according to the crude drug. The experimental procedures were approved by the Animal Care and Ethics Committee at Taizhou University Hospital and all experiments were performed in accordance to the declaration of Helsinki.
2.5. Collection and preparation of urine samples
Collection. After 12 weeks of modeling, all rats were placed in rat metabolic cages, and the urine of each group of rats was collected 24 hours after the last administration, fasting, free drinking, centrifuged at 4 °C, 13
000 rpm for 15 min, supernatant removed, and stored in a refrigerator at −80 °C for later use.
Sample pretreatment. Urine sample is melted on ice, 200 μL urine is taken, 600 μL acetonitrile is added, vortex is carried out for 30 s, centrifugation is carried out at 4 °C for 10 min at 13
000 rpm, supernatant is filtered by 0.22 μm microporous filter membrane, filtrate is taken for UPLC-MS/MS system analysis.
2.6. Instrumental analysis conditions
The UPLC-MS/MS analysis was carried out using a Waters ACQUITY™ ultra performance liquid chromatography system (Waters Corp., Milford, USA) coupled with a Waters Synapt™ Q-TOF Mass system (Waters Corporation). Column: ACQUITY UPLC TM BEH C18 (2.1 mm × 100 mm, 1.7 μm, Waters, USA); mobile phase: phase A is aqueous solution containing 0.1% formic acid, phase B is acetonitrile containing 0.1% formic acid; gradient elution of A and B, 0–2 min, 2% B; 2–8 min, 2−30% B; 8–10 min, 30–98% B; 12–13 min, 98% B; 13–13.1 min, 98–2% B; 13.1–14 min, 2% B. Flow rate: 0.4 mL min−1; column temperature: 40 °C; sample room temperature: 4 °C; injection volume: 5 μL.
Mass spectrometry conditions: Waters Synapt™ Q-TOF Mass system using electrospray ion source (ESI); detection mode: simultaneous detection of positive and negative ions; scan mode: metabolite spectrum analysis adopts Full Scan mode with scan range of 100–1000 amu; metabolites were identified by secondary mass spectrometry (MS/MS scan). Ion source temperature: 120 °C; capillary voltage: 3.2 kV (ESI+), 3.0 kV (ESI−); taper hole voltage: 25 V (ESI+), 35 V (ESI−); desolvent gas and cone gas: nitrogen; desolvent gas temperature: 400 °C; desolvent gas flow rate: 450 L h−1; taper hole gas flow rate: 30 L h−1; collision gas: argon; collision energy: 5–25 eV.
2.7. Multivariate data processing
The established urine sample analysis method was used to carry out full scanning, and metabolic profile maps containing three-dimensional information of each group of samples were obtained. The data were processed by Waters Corporation Masslynx V4.1 (Waters Corporation, USA) workstation for peak matching, peak alignment normalization, etc., and then imported into EZinfo 3.0.3 (Waters Corporation, in USA) software, principal component analysis (PCA) is performed to generate score plot and 3D-PCA score plot to obtain clustering information between sample groups, and loading plot is used to find potential biomarkers. The further away from the origin, the greater the contribution of ions to changes in the metabolic profile trajectory. To further explore which differential metabolites play a essential role in metabolic profile changes, paired partial least squares discriminant analysis (OPLS-DA) was performed on metabolic profile data between blank group and model group to obtain VIP plot, S-plot. The further away from the center, the greater the contribution of ions to the grouping. Using Student's paired test for t-test analysis, p < 0.05 believed that there was significant difference. Finally, VIP > 1 and p < 0.05 were selected as screening conditions as potential biomarker sets. Through searching HMDB and KEGG databases for possible structural formulas based on the accurate mass-to-nucleus ratio of metabolites, searching and comparing statistically significant metabolites to determine differential metabolites, and then analyzing metabolic pathways and biological significance of biomarkers through such large databases as KEGG, MetPA, etc.
2.8. Confirmation of UPLC-MS/MS analysis method
All urine samples to be tested are taken with equal quality and mixed evenly to prepare mixed samples. Take appropriate amount of mixed samples and prepare quality control (QC) samples according to urine sample pretreatment method. Select 6 ions in ESI+, calculate the retention time of each extracted ion and RSD value of peak area to examine the precision of the instrument, repeatability of the method, and RE value of peak area to examine the room temperature stability, stability after pretreatment and freeze–thaw cycle stability. In the actual sample determination process, QC samples are analyzed once every 10 samples to monitor the stability of the system. The RSD and RE values of the test results are both less than 15%, indicating that the established analysis method meets the analysis requirements of biological samples.
2.9. Statistical methods
Statistical analysis of each group of urine samples was performed using SPSS v19.0 software package. Results are expressed as mean standard deviation, p < 0.05 indicating statistically significant differences.
3. Results
3.1. Changes in metabolite spectrum
Before starting to analyze urine samples, the analysis method was verified according to the above methodology investigation method. The results show that the RSD and RE values of precision, method repeatability, sample stability and system stability are all less than 15%, indicating that the established analysis method meets the analysis requirements of biological samples. All rat urine samples were prepared according to the above sample pretreatment method, and full scanning was carried out under the system stable UPLC-Q-TOF-MS system. The original data of all samples were collected and analyzed by MassLynx V4.1 software (Waters, USA). BPI chromatogram with three-dimensional information of urine sample is obtained. Then all urine ESI-MS metabolic profile data are imported into Progenesis QI software. Each chromatographic peak is subjected to peak matching and peak extraction. After standardization, ions are normalized. Then the ion information is transferred into Ezinfo3.0.3 software for multivariate data analysis and PCA is performed on all ions. The PCA score charts (Fig. 1A and B) and 3D-plot (Fig. 2A and B) are obtained, in the charts, we can clearly see that the clusters among the groups are obvious and far away from each other, and the groups also have different spatial positions in the three-dimensional space. The model group is significantly separated from the control group, which indicates that the urine metabolism level of the model group rats has changed significantly after the modeling is completed. The treatment group rats are far away from the model rats and call back to the control rats, which indicate that the glucocorticoid osteoporosis rat model is successfully replicated at the urine metabolism level, and a certain effect appears after being treated with PCS.
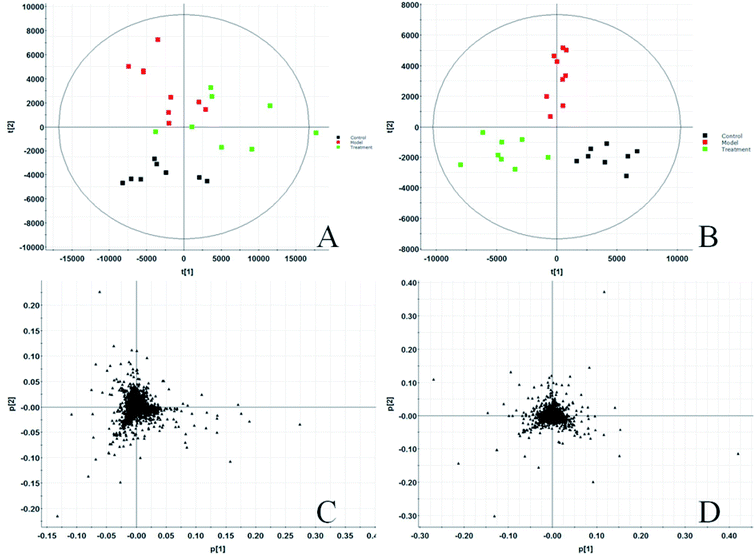 |
| Fig. 1 Score plot and loading plot of urine profile of control group, model group and treatment group scanned by PCA analysis. (A) Score plot in positive ion mode. (B) Score plot in negative ion mode. (C) Loading plot in positive ion mode. (D) Loading plot in negative ion mode. | |
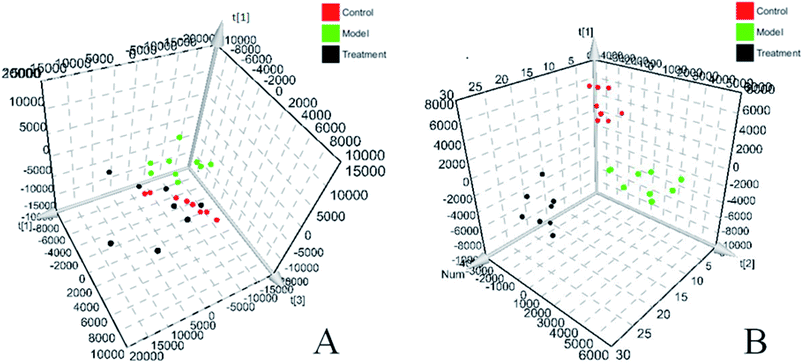 |
| Fig. 2 3D-score plot of urine profile of control group, model group and treatment group scanned by PCA analysis. (A) Positive ion mode. (B) Negative ion mode. | |
3.2. Screening and identification of potential biomarkers
In the PCA score chart, we can find that the clustering separation between groups is obvious, and further obtain the S-plot score chart (Fig. 3C and D), the farther from the origin in the chart, the greater the contribution rate of ions to the clustering between groups. To further exploit the urine metabolites play an important role in the metabolic cluster separation between the two groups. OPLS-DA analysis is carried out on the urine metabolic profile, score plot (Fig. 4A and B) and 3D-plot (Fig. 4C and D) were obtained, and indicates that the expression of metabolites in glucocorticoid-induced osteoporosis model rats is significantly different from that in the control group, and VIP-plot (Fig. 4A and B) and S-plot (Fig. 3C and D). It reflects the contribution rate of different ions to the separation between groups, and the contribution rate of ion pairs farther from the origin is larger. At the same time, combined with independent sample T-TEST of mathematical statistics, ions with p < 0.05 (p < 0.05 is considered to have statistical difference) were screened out, and finally selected VIP > 1 and p < 0.05 ions as potential metabolic markers. Combined with the retention time of each ion, accurate treatment and secondary fragment information of MS/MS obtained by UPLC-Q-TOF-MS/MS system, accurate molecular weight and element composition are obtained within a reasonable error range. The structure analysis of potential biomarkers is carried out by referring to literature and network databases such as HMDB and KEGG to determine the most likely chemical name and structure. Finally, we identified and characterized 42 potential biomarkers, 24 in ESI+ and 18 in ESI−, seen Table S1.† Among them, the content of 20 metabolites in model rats increased, and 22 decreased.
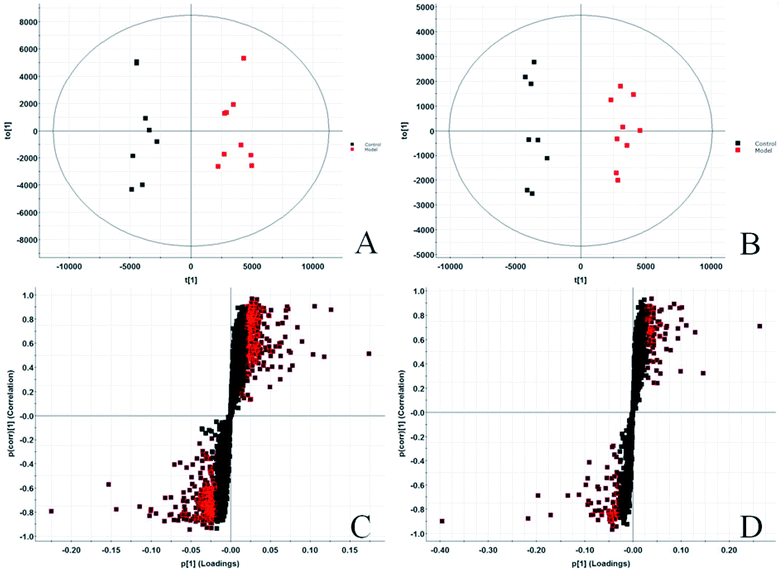 |
| Fig. 3 Metabolomics profiling of osteoporosis with the control group and model group. (A) Score plot of urine profile of control group and model group scanned by OPLS-DA analysis in positive ion mode. (B) Score plot of urine profile of control group and model group scanned by OPLS-DA analysis in negative ion mode. (C) S-plot of urine profile of control group and model group scanned by OPLS-DA analysis in positive ion mode. (D) S-plot of urine profile of control group and model group scanned by OPLS-DA analysis in negative ion mode. | |
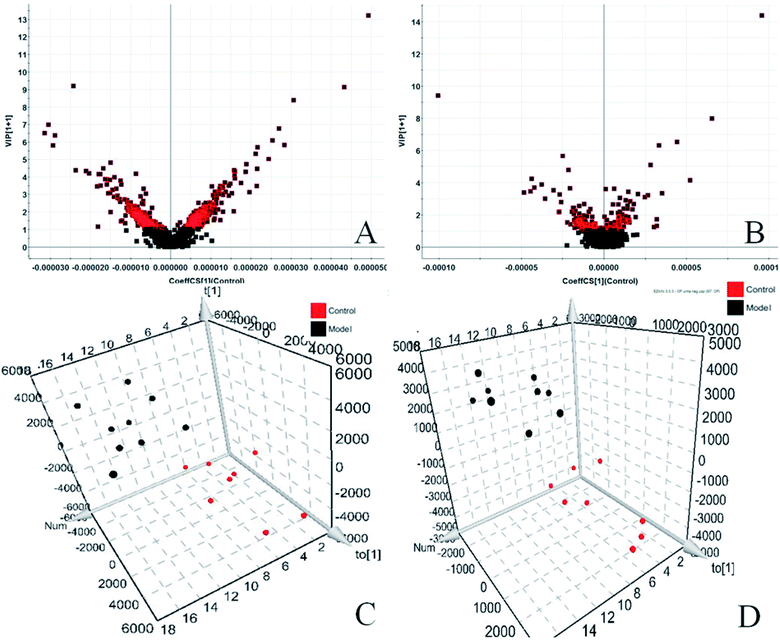 |
| Fig. 4 VIP-plot and 3D-plot of urine profile of control group and model group scanned by OPLS-DA analysis. (A) VIP-plot in positive ion mode. (B) VIP-plot in negative ion mode. (C) 3D-plot in positive ion mode. (D) 3D-plot in negative ion mode. | |
3.3. Biological pathway and function analysis
According to the 42 urine metabolic biomarkers identified, the enrichment analysis of relevant metabolic pathways was carried out using network databases such as MetaboAnalyst and KEGG, etc. The relevant metabolic pathways affected in urine of glucocorticoid-induced osteoporosis model rats were excavated. A total of 12 related metabolic pathways (Fig. 5) were characterized, including glycine, serine and threonine metabolism; cyanoamino acid metabolism; methane metabolism; nitrogen metabolism; nicotinate and nicotinamide metabolism; glutathione metabolism; porphyrin and chlorophyll metabolism; amino sugar and nucleotide sugar metabolism; tryptophan metabolism; arginine and proline metabolism; primary bile acid biosynthesis; purine metabolism. These results show that these endogenous metabolites produce strong disturbances in the whole metabolic pathway and are closely related to glucocorticoid osteoporosis. The most relevant to glucocorticoid-induced osteoporosis is amino acid metabolism. In addition, according to the path database in KEGG, the potential relationships focused on the main unordered paths are reconstructed in Fig. 6. Disordered biomarkers in these pathways can reflect the relationship between different biomarkers and pathways.
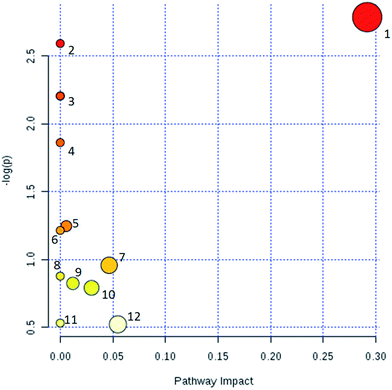 |
| Fig. 5 Metabolic pathways associated with potential biomarkers was generated using MetaboAnalyst in rat serum. (1) Glycine, serine and threonine metabolism (2) cyanoamino acid metabolism (3) methane metabolism (4) nitrogen metabolism (5) nicotinate and nicotinamide metabolism (6) Glutathione metabolism (7) porphyrin and chlorophyll metabolism (8) amino sugar and nucleotide sugar metabolism (9) tryptophan metabolism (10) arginine and proline metabolism (11) primary bile acid biosynthesis (12) purine metabolism. | |
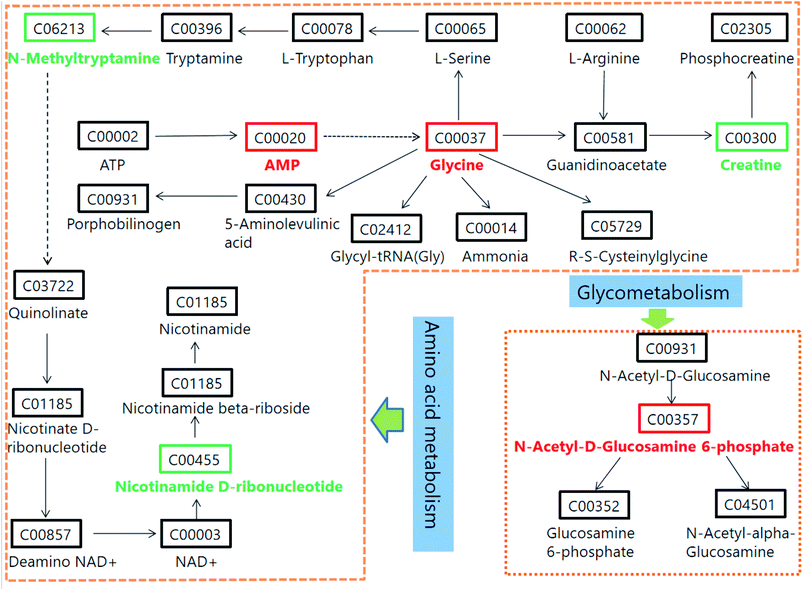 |
| Fig. 6 Framework diagram of potential biomarkers and related metabolic pathways. In the path shown in the frame, the KEGG identification of each biomarker is displayed. A red box around the KEGG marker indicates that the marker is up-regulated in the model group, a green box indicates that the marker is down-regulated, and a black box indicates that the biomarker is not found in our experiment. | |
3.4. PCS affects metabolism
To verification the therapeutic effect of PCS on glucocorticoid osteoporosis model rats and the influence of urine metabolism level, PCA analysis was carried out on urine metabolism of three groups of rats, and PCA score charts (Fig. 2A and B) and 3D-plot (Fig. 3A and B) were obtained, the results show that the rats in the treatment group are far away from the model group and callback to the control group, which shows that PCS has certain callback effect on urine metabolism profile of model rats, making it approach to healthy rats, and also shows that PCS has certain intervention effect on glucocorticoid-induced osteoporosis. Further analysis of the effect of PCS on the content of potential biomarkers in glucocorticoid-induced osteoporosis model rats shows that after treatment, PCS can affect the content metabolism of biomarkers in model rats and make them develop towards the direction of blank group. The content change thermogram (Fig. 7) of all identified potential biomarkers in rats in each group shows that most of them have obvious changes in the content of some biomarkers, and the trend of callback is shown after treatment. In the content change bar chart (Fig. 8), it can be found more intuitively that among the 42 potential biomarkers identified, the administration group can recall 24, among which 7 have significant difference (p < 0.05) and 11 have extremely significant difference (p < 0.01) compared with the model group, revealing the change of the relative content of potential biomarkers between the two groups. This indicates that the biomarkers in urine that may be related to the pathogenesis of osteoporosis tend to be normal under the action of PCS, and the metabolic disorders related to the body are adjusted. Through metabolic pathway analysis, it is found that the biomarkers of callback are mainly associated with metabolic pathways such as glycine, serine and threonine metabolism; glutathione metabolism and purify metabolism, etc. It is suggested that PCS may interfere with the occurrence and development of glucocorticoid-induced osteoporosis by affecting the above metabolic pathways made it tends to normal levels.
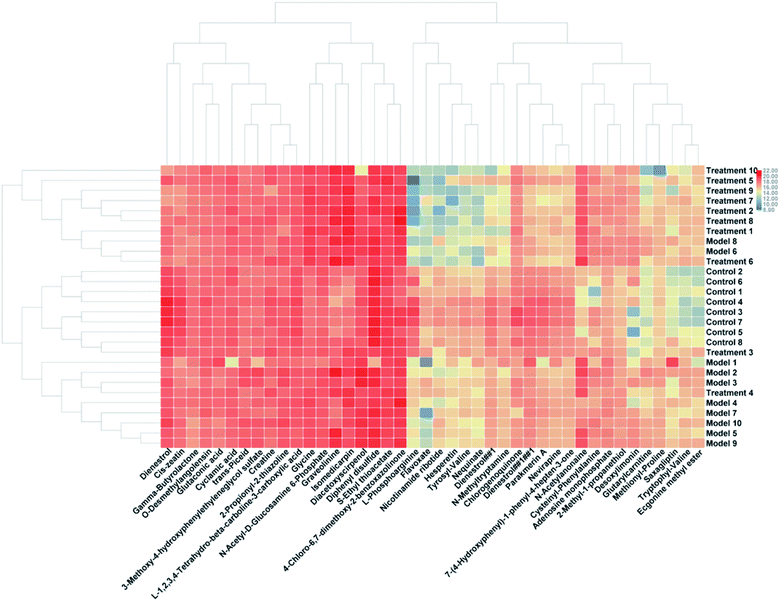 |
| Fig. 7 Heat map analysis of 42 differential metabolites in control group, model group and treatment group rats. The degree of change in the mark with different colors, each row represents a single sample, and each column represents a metabolite. | |
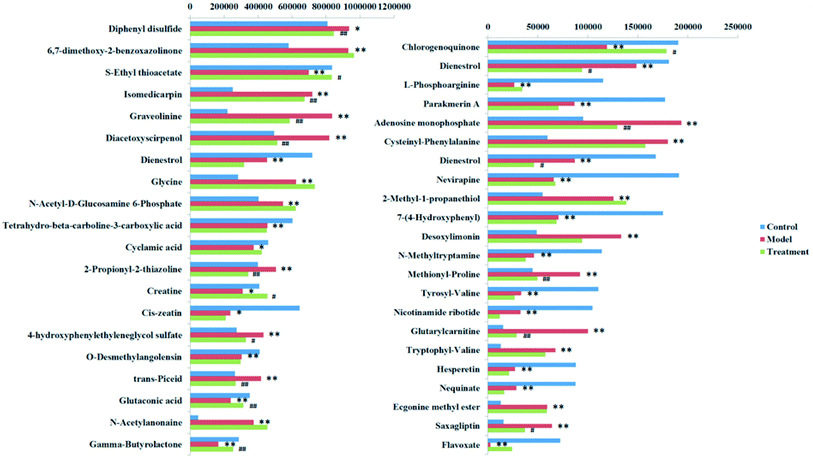 |
| Fig. 8 Changes in urine relative content of potential biomarkers after intragastric administration PCS solution. (Compare the control group to the model group, *p < 0.05, **p < 0.01; comparison between model group and treatment group, #p < 0.05, ##p < 0.01). | |
4. Discussion
OP is a worldwide problem that puzzles the elderly and seriously affects the health and normal life of the elderly. In recent years, the aging of the world's population is increasing and the incidence of OP is increasing, bringing great troubles to society and individuals.39 Finding effective biomarkers for early diagnosis of OP and developing effective therapeutic drugs with definite curative effect, safety and stability are the urgent problems for researchers at present. As the pathogenesis of OP is still unclear and no effective early diagnosis basis has been determined, therapeutic drugs with definite curative effect and high cure rate have not been developed.40 However, medical and pharmaceutical researchers have never stopped their exploration. A variety of advanced scientific research methods and strategies are applied to the study of diseases. With the continuous improvement of UPLC-MS detection and analysis instruments, high-throughput metabolomics technology has been more and more widely used in the diagnosis and treatment of OP diseases.41 Glucocorticoid-induced osteoporosis model is mainly used to study secondary osteoporosis. Its mechanism is to directly inhibit osteoblast activity, activate osteoclasts and reduce bone formation with super-physiological dose of glucocorticoid. At the same time, it can directly stimulate osteoclasts by reducing estrogen secretion, inhibit intestinal absorption of calcium, increase renal excretion of calcium and phosphorus, stimulate hyperparathyroidism, etc., further increase bone absorption, reduce bone formation, and eventually lead to bone metabolism in a negative balance state where bone absorption is greater than bone formation, resulting in bone loss, thus inducing osteoporosis.42,43 Some scholars believe that autophagy of bone cells may also be a mechanism. Usually, medium-effect prednisolone and methylprednisolone are selected, and occasionally high-effect dexamethasone is used. Obvious bone loss occurred at the earliest 10 days and 48 weeks after use. A large number of studies show that obvious changes have been found in endogenous metabolites of OP model rats. Whether in blood metabolism or urine metabolism or tissue, changes in the expression of endogenous metabolites may be the cause of OP occurrence or the result of OP formation. The connection is not clear. In this study, prednisolone was injected intramuscularly to duplicate the osteoporosis rat model to study the intervention effect and therapeutic mechanism of PCS on the osteoporosis rat model. However, the pathogenesis and course development of glucocorticoid-induced osteoporosis and primary osteoporosis are not consistent, so the model is not entirely suitable for the study of trying to evaluate the inhibitory effect of drugs on bone absorption.
Metabolomics has been widely used in the field of medicine since it was put forward.44 The abnormal physiological states of many diseases are related to endogenous small molecule metabolic disorders. Studying the changes of endogenous metabolites in different states of objects from the system level is helpful to analyze the mechanism of disease metabolic network. Identifying the key metabolites and related metabolic pathways is helpful to find the potential metabolic pathways, further search for abnormal physiological states and explore the pathogenesis mechanism, and finally dig out effective disease diagnosis basis and treatment methods.45,46 Previous studies have shown that PCS is a traditional Chinese herbal medicine, has a certain regulatory effect on OP, but its metabolomic mechanism of anti-OP is still unclear and its target is still unclear. It needs to be continuously explored and discovered. In order to explore glucocorticoid-induced changes in urine metabolic spectrum of OP and further elucidate its pathogenesis, the potential mechanism of PCS for prevention of OP and changes in endogenous biomarkers in urine were studied. The advantages of UPLC mass spectrometry such as high sensitivity and high resolution are utilized to obtain comprehensive and abundant molecular information. In this study, the glucocorticoid OP rat model was replicated by intramuscular injection of prednisolone, and the metabolites in rat urine were analyzed by high-throughput metabolomics platform. The advantages of UPLC-MS with high sensitivity and high resolution were utilized to obtain comprehensive and abundant metabolic molecular information. The metabolic spectrum changes of endogenous small molecule metabolites among different groups of rats are revealed, and then potential biomarkers are screened and identified, and the affected metabolic pathways are characterized by metabolic pathways. Some studies have shown that amino acid metabolism in patients with osteoporosis is abnormal.47 In this study, the most relevant to osteoporosis in rats is the disorder of amino acid metabolism. Human and animal proteins introduced by food or proteins that make up the body's cells and proteins synthesized in the cells must be hydrolyzed with the participation of the enzyme before being metabolized. The ammonia produced during catabolism can be excreted in the form of ammonia, urea or uric acid in different animals. Certain amino acids can be converted to other nitrogen-containing substances such as purines, pyrimidines, porphyrins, certain hormones, pigments, alkaloids, etc. by special metabolic pathways. Certain amino acids in the body can also be transformed into each other during metabolism.48 In this study, glycine, adenosine-monophosphate was elevated, while creatine, N-acetyl-D-glucosamine 6-phosphate and nicotinamide D-ribonucleotide were significantly reduced, and these biomarkers were involved in amino acids. Metabolism indicates that osteoporosis in rats is closely related to the disorder of amino acid metabolism in the body. In addition, this experiment also found the N-methyltryptamine biomarker in the tryptophan metabolism pathway, indicating that there is also a disorder of tryptophan metabolism in the uncle of osteoporosis, which may be related to the long-term supply of glucocorticoids. After being treated with PCS, the therapeutic effect of PCS on glucocorticoid osteoporosis model rats and the influence of PCS on potential biomarkers and metabolic pathways at urine metabolic level are further characterized. Finally, explore the therapeutic target and mechanism of PCS on osteoporosis. The results showed that the model group was significantly separated from the control group after the model replication, a total of 42 different metabolites were identified, of which 4 had significant difference (p < 0.05) and 38 had extremely significant difference (p < 0.01). 12 related metabolic pathways were mainly involved, of which 3 were the most relevant. After treatment with PCS, 24 metabolic markers can be obviously recalled. Eleven of them have extremely significant difference (p < 0.01), revealing that PCS may achieve the effect of treating glucocorticoid-induced osteoporosis by adjusting the metabolic level of these biomarkers.
Metabolomics method has demonstrated potential in some fields such as toxicology,49–55 nutrition,56–61 genetic manipulation,62–67 cancer,68–72 diabetes,73–81 environment,82–89 disease diagnosis90 and drug discovery and research.91–98 Liquid chromatography coupled with mass spectrometry has been used as powerful tools to discover and identify the metabolites in metabolic diseases. High-throughput metabolomics based on the liquid chromatography coupled with mass spectrometry quadrupole time-of-flight mass spectrometry and multivariate data analysis has been used to analyze the urine metabolites. This study showed that 42 potential biomarkers and 12 related metabolic pathways were discovered, 24 biomarkers were significantly regulated and related with glycone, serine and threonine metabolism, etc. It has proved that PCS has therapeutic effect against OP by regulating that metabolic pathways disturbed in the OP, and provided a basis for the research and future development of new drugs for OP treatment.
5. Conclusion
In this study, the rat model of glucocorticoid OP was reproduced by intramuscular injection of prednisolone. The metabolites in rat urine were analyzed by UPLC-Q-TOF-MS/MS and multivariate data analysis methods. UPLC-MS has the advantages of high sensitivity and high resolution to obtain comprehensive and abundant metabolic molecular information. From the level of rat urine metabolomics, 42 potential biomarkers related to glucocorticoid-induced OP and 12 related metabolic pathways related to these biomarkers are revealed, revealing that the occurrence and development of OP may be closely related to metabolic disorders of these metabolites. After treatment with PCS, 24 potential biomarkers can be significantly recalled, of which 7 have significant differences and 11 have extremely significant differences. These regulated biomarkers are mainly related to 3 metabolic pathways, which indicate that PCS can develop toward a healthy direction by affecting the level of these metabolites in glucocorticoid-induced OP model rats, laying a foundation for further finding therapeutic targets of PCS to treat OP, and providing basis for clinical diagnosis of OP.
Conflicts of interest
There are no conflicts to declare.
References
- J. A. Kanis, M. L. Rd, C. Christiansen, C. C. Johnston and N. Khaltaev, The diagnosis of osteoporosis, J. Bone Miner. Res., 2010, 9(8), 1137–1141 CrossRef.
- J. Compston, Glucocorticoid-induced osteoporosis: an update, Endocrine, 2018, 61(11), 1–10 Search PubMed.
- N. Bucay, I. Sarosi, C. R. Dunstan, S. Morony, J. Tarpley and C. Capparelli, Osteoprotegerin-deficient mice develop early onset osteoporosis and arterial calcification, Genes Dev., 1998, 12(9), 1260 CrossRef CAS.
- I. Akkawi and H. Zmerly, Osteoporosis: current concepts, Joints, 2018, 6(2), 122–127 CrossRef.
- J. E. M. Sale, A. Marwah, F. Naeem, W. Yu and L. Meadows, Evidence of patient beliefs, values, and preferences is not provided in osteoporosis clinical practice guidelines, Osteoporosis Int., 2019, 1–13 Search PubMed.
- M. L. Rd, E. A. Chrischilles, C. Cooper, A. W. Lane and B. L. Riggs, Perspective. how many women have osteoporosis?, J. Bone Miner. Res., 2010, 7(9), 1005–1010 Search PubMed.
- R. Burge, B. Dawson-Hughes, D. H. Solomon, J. B. Wong, A. King and A. Tosteson, Incidence and economic burden of osteoporosis-related fractures in the united states, 2005–2025, J. Bone Miner. Res., 2007, 22(3), 465–475 CrossRef PubMed.
- E. Canalis, G. Mazziotti, A. Giustina and J. P. Bilezikian, Glucocorticoid-induced osteoporosis: pathophysiology and therapy, Osteoporosis Int., 2007, 18(10), 1319–1328 CrossRef CAS.
- R. S. Weinstein, R. L. Jilka, A. M. Parfitt and S. C. Manolagas, Inhibition of osteoblastogenesis and promotion of apoptosis of osteoblasts and osteocytes by glucocorticoids. potential mechanisms of their deleterious effects on bone, J. Clin. Invest., 1998, 102(2), 274–282 CrossRef CAS.
- P. Garnero and P. D. Delmas, Osteoporosis, Endocrinol. Metab. Clin. North Am., 1997, 26(4), 913–936 CrossRef CAS.
- I. R. Reid, Denosumab for glucocorticoid-induced osteoporosis, Nat. Rev. Endocrinol., 2018, 14(7), 383–384 CrossRef CAS.
- P. S. Khushboo, V. M. Jadhav, V. J. Kadam and N. S. Sathe, Psoralea corylifolia linn. – “kushtanashini”, Pharmacogn. Rev., 2010, 4(7), 69–76 CrossRef CAS.
- P. L. Wang, Z. H. Yao, F. X. Zhang, X. Y. Shen, Y. Dai and L. Qin, Identification of metabolites of psoraleae fructus in rats by ultra performance liquid chromatography coupled with quadrupole time-of-flight tandem mass spectrometry analysis, J. Pharm. Biomed. Anal., 2015, 112, 23–35 CrossRef CAS.
- X. Zhang, W. Zhao, Y. Wang, J. Lu and X. Chen, The chemical constituents and bioactivities of psoralea corylifolia linn.: a review, Am. J. Chin. Med., 2016, 44(01), 35–60 CrossRef CAS.
- Y. Chen, H. D. Wang, X. Xia, H. F. Kung, Y. Pan and L. D. Kong, Behavioral and biochemical studies of total furocoumarins from seeds of psoralea corylifolia in the chronic mild stress model of depression in mice, Phytomedicine, 2007, 14(7), 523–529 CrossRef CAS.
- Y. Chen, Y. T. Cheung, L. D. Kong, T. B. Ng, C. Qiao and S. F. Mo, Transcriptional regulation of corticotrophin releasing factor gene by furocoumarins isolated from seeds of psoralea corylifolia, Life Sci., 2008, 82(21), 1117–1121 CrossRef CAS PubMed.
- F. Alam, G. N. Khan and A. Mhhb, Psoralea corylifolia l: ethnobotanical, biological, and chemical aspects: a review, Phytother. Res., 2017, 32(4), 597–615 CrossRef.
- G. A. Kassahun, T. D. Wijerathne, J. H. Kim, M. J. Kim, C. S. Seo and H. K. Shin, Psoralea corylifolia extract induces
vasodilation in rat arteries through both endothelium-dependent and -independent mechanisms involving inhibition of trpc3 channel activity and elaboration of prostaglandin, Pharm. Biol., 2017, 55(1), 2136 CrossRef.
- Z. B. Weng, Q. Q. Gao, F. Wang, G. H. Zhao, F. Z. Yin and B. C. Cai, Positive skeletal effect of two ingredients of psoralea corylifolia l. on estrogen deficiency-induced osteoporosis and the possible mechanisms of action, Mol. Cell. Endocrinol., 2015, 417(C), 103–113 CrossRef CAS.
- Y. X. Cai, Effects of psoralea corylifolia l. on bone metabolism in ovariectomized osteoporotic rats, J. Clin. Rehabil. Tissue Eng. Res., 2009, 13(2), 268–271 CAS.
- W. D. Li, C. P. Yan, Y. Wu, Z. B. Weng, F. Z. Yin and G. M. Yang, Osteoblasts proliferation and differentiation stimulating activities of the main components of fructus Psoraleae corylifoliae, Phytomedicine, 2014, 21(4), 400–405 CrossRef CAS.
- O. Fiehn, Metabolomics – the link between genotypes and phenotypes, Plant Mol. Biol., 2002, 48(1–2), 155–171 CrossRef CAS.
- D. Q. Chen, G. Cao, H. Chen, C. P. Argyopoulos, H. Yu, W. Su, L. Chen, D. C. Samuels, S. Zhuang, G. P. Bayliss, S. Zhao, X. Y. Yu, N. D. Vaziri, M. Wang, D. Liu, J. R. Mao, S. X. Ma, J. Zhao, Y. Zhang, Y. Q. Shang, H. Kang, F. Ye, X. H. Cheng, X. R. Li, L. Zhang, M. X. Meng, Y. Guo and Y. Y. Zhao, Identification of serum metabolites associating with chronic kidney disease progression and anti-fibrotic effect of 5-methoxytryptophan, Nat. Commun., 2019, 10(1), 1476 CrossRef.
- M. Wang, L. Chen, D. Liu, H. Chen, D. D. Tang and Y. Y. Zhao, Metabolomics highlights pharmacological bioactivity and biochemical mechanism of traditional Chinese medicine, Chem.-Biol. Interact., 2017, 273, 133–141 CrossRef CAS.
- D. Q. Chen, G. Cao, H. Chen, D. Liu, W. Su, X. Y. Yu, N. D. Vaziri, X. H. Liu, X. Bai, L. Zhang and Y. Y. Zhao, Gene and protein expressions and metabolomics exhibit activated redox signaling and wnt/β-catenin pathway are associated with metabolite dysfunction in patients with chronic kidney disease, Redox Biol., 2017, 12, 505–521 CrossRef CAS.
- Y. Shao and W. Le, Recent advances and perspectives of metabolomics-based investigations in parkinson's disease, Mol. Neurodegener., 2019, 14(1), 3 CrossRef.
- H. Chen, G. Cao, D. Q. Chen, M. Wang, N. D. Vaziri, Z. H. Zhang, J. R. Mao, X. Bai and Y. Y. Zhao, Metabolomics insights into activated redox signaling and lipid metabolism dysfunction in chronic kidney disease progression, Redox Biol., 2016, 10, 168–178 CrossRef CAS.
- N. E. Madala, P. A. Steenkamp, L. A. Piater and I. A. Dubery, Collision energy alteration during mass spectrometric acquisition is essential to ensure unbiased metabolomic analysis, Anal. Bioanal. Chem., 2012, 404, 367–372 CrossRef CAS.
- R. S. Plumb, K. A. Johnson, P. Rainville, B. W. Smith, I. D. Wilson and J. M. Castroperez, et al., Uplc/ms(e); a new approach for generating molecular fragment information for biomarker structure elucidation, Rapid Commun. Mass Spectrom., 2006, 20(13), 1989–1994 CrossRef CAS.
- Y. Y. Zhao, et al., Renal metabolic profiling of early renal injury and renoprotective effects of Poria cocos epidermis using UPLC Q-TOF/HSMS/MSE, J. Pharm. Biomed. Anal., 2013, 202–209 Search PubMed.
- Z. H. Zhang, N. D. Vaziri, F. Wei, X. L. Cheng, X. Bai and Y. Y. Zhao, An integrated lipidomics and metabolomics reveal nephroprotective effect and biochemical mechanism of Rheum officinale in chronic renal failure – eScholarship, Sci. Rep., 2016, 6(3), 22151 CrossRef CAS.
- H. Lin, H. Zhu, J. Tan, C. Wang, Q. Dong and F. Wu, et al., Comprehensive investigation on metabolites of wild-simulated american ginseng root based on ultra-high-performance liquid chromatography-quadrupole time-of-flight mass spectrometry, J. Agric. Food Chem., 2019, 67(20), 5801–5819 CAS.
- D. Zhang, L. Qu, Z. Wang and J. Zhang, Identification of the chemical components and metabolites of tripterygium glycoside tablets in mice by hplc-q/tof ms, J. Chromatogr. B: Anal. Technol. Biomed. Life Sci., 2019, 1125, 121721 CrossRef CAS.
- M. A. Farag, A. Porzel, E. A. Mahrous, M. M. El-Massry and L. A. Wessjohann, Integrated comparative metabolite profiling via ms and nmr techniques for senna drug quality control analysis, Anal. Bioanal. Chem., 2015, 407(7), 1937–1949 CrossRef CAS.
- Y. Li, H. Zhan, Y. Fan, J. Zhang, G. Cao and J. Yu, et al., Determination of dp-vpa and its active metabolite, vpa, in human plasma, urine, and feces by uplc-ms/ms: a clinical pharmacokinetics and excretion study, Drug Test. Anal., 2019, 11(7), 1035 CrossRef CAS.
- X. Zeng, W. Su, Y. Zheng, H. Liu, P. Li and W. Zhang, et al., Uflc-q-tof-ms/ms-based screening and identification of flavonoids and derived metabolites in human urine after oral administration of exocarpium citri grandis extract, Molecules, 2018, 23(4), 895 CrossRef.
- J. Jiang, L. Feng, E. Sun, H. Li, L. Cui and X. Jia, Metabolic profiling of isomeric aglycones central-icaritin (c-it) and icaritin (it) in osteoporotic rats by uplc-qtof-ms, Drug Test. Anal., 2015, 7(4), 309–319 CrossRef CAS.
- B. Yang, A. Zhang and H. Sun, et al., Metabolomic study of insomnia and intervention effects of suanzaoren decoction using ultra-performance liquid-chromatography/electrospray-ionization synapt high-definition mass spectrometry, J. Pharm. Biomed. Anal., 2012, 58, 113–124 CrossRef CAS.
- M. Vidal, R. J. Thibodaux, L. F. V. Neira and O. D. Messina, Osteoporosis: a clinical and pharmacological update, Clin. Rheumatol., 2019, 38(2), 385–395 CrossRef.
- X. Sun, J. Wei, J. Lyu, T. Bian, Z. Liu and J. Huang, Bone-targeting drug delivery system of biomineral-binding liposomes loaded with icariin enhances the treatment for osteoporosis, J. Nanobiotechnol., 2019a, 17(1), 10 Search PubMed.
- J. Wang, et al., Discovery of potential biomarkers for osteoporosis using LC-MS/MS metabolomic methods, Osteoporosis Int., 2019, 1–9 Search PubMed.
- B. Wallace, K. G. Saag, J. R. Curtis and A. K. Waljee, Just the frax: management of glucocorticoid-induced osteoporosis, Gastroenterology, 2018, 154(3), 748–750 CrossRef.
- I. C. Benlidayi, Denosumab in the treatment of glucocorticoid-induced osteoporosis, Rheumatol. Int., 2018, 38(11), 1–10 Search PubMed.
- H. Sun, X. Li and A. Zhang, et al., Exploring potential biomarkers of coronary heart disease treated by Jing Zhi Guan Xin Pian using high-throughput metabolomics, RSC Adv., 2019, 9(20), 11420–11432 RSC.
- J. Cheng, W. Lan, G. Zheng and X. Gao, Metabolomics: a high-throughput platform for metabolite profile exploration, Methods Mol. Biol., 2018, 1754, 265 CrossRef CAS PubMed.
- X. Li, H. Luo, T. Huang, L. Xu, X. Shi and K. Hu, Statistically correlating nmr spectra and lc-ms data to facilitate the identification of individual metabolites in metabolomics mixtures, Anal. Bioanal. Chem., 2019, 1–9 Search PubMed.
- Q. Zhao, H. Shen, K. J. Su, J. G. Zhang, Q. Tian and L. J. Zhao, et al., Metabolomic profiles associated with bone mineral density in us caucasian women, Nutr. Metab., 2018, 15(1), 57 CrossRef.
- B. J. M. And and P. J. Lea, Amino acid metabolism, Annu. Rev. Plant Physiol., 1977, 28(1), 299–329 CrossRef.
- Y. F. Li, S. Qiu and L. J. Gao, et al., Metabolomic estimation of the diagnosis of hepatocellular carcinoma based on ultrahigh performance liquid chromatography coupled with time-of-flight mass spectrometry, RSC Adv., 2018, 8(17), 9375–9382 RSC.
- H. Wang, G. Yan and A. Zhang, et al., Rapid discovery and global characterization of chemical constituents and rats metabolites of Phellodendri amurensis cortex by ultra-performance liquid chromatography-electrospray ionization/quadrupole-time-of-flight mass spectrometry coupled with pattern recognition approach, Analyst, 2013, 138(11), 3303–3312 RSC.
- X. Wang, J. Li and A. H. Zhang, Urine metabolic phenotypes analysis of extrahepatic cholangiocarcinoma disease using ultra-high performance liquid chromatography-mass spectrometry, RSC Adv., 2016, 6(67), 63049–63057 RSC.
- A. H. Zhang, H. Sun and G. L. Yan, et al., Chinmedomics: A Powerful Approach Integrating Metabolomics with Serum Pharmacochemistry to Evaluate the Efficacy of Traditional Chinese Medicine, Engineering, 2019, 5, 60–68 CrossRef CAS.
- H. Sun, H. L. Zhang and A. H. Zhang, et al., Network pharmacology combined with functional metabolomics discover bile acid metabolism as a promising target for mirabilite against colorectal cancer, RSC Adv., 2018, 8, 30061–30070 RSC.
- A. Zhang, H. Sun and G. Yan, et al., Metabolomics study of type 2 diabetes using ultra-performance LC-ESI/quadrupole-TOF high-definition MS coupled with pattern recognition methods, J. Physiol. Biochem., 2014, 70(1), 117–128 CrossRef CAS.
- H. Sun, A. Zhang and L. Yang, et al., High-throughput chinmedomics strategy for discovering the quality-markers and potential targets for Yinchenhao decoction, Phytomedicine, 2019, 54, 328–338 CrossRef CAS.
- A. Zhang, H. Wang and H. Sun, et al., Metabolomics strategy reveals therapeutical assessment of limonin on nonbacterial prostatitis, Food Funct., 2015, 6(11), 3540–3549 RSC.
- Y. Zhang, P. Liu and Y. Li, et al., Exploration of metabolite signatures using high-throughput mass spectrometry coupled with multivariate data analysis, RSC Adv., 2017, 7, 6780–6787 RSC.
- A. Zhang, H. Sun and H. Xu, et al., Cell metabolomics, OMICS, 2013, 17(10), 495–501 CrossRef CAS.
- H. L. Zhang, A. H. Zhang and X. H. Zhou, et al., High-throughput lipidomics reveal mirabilite regulating lipid metabolism as anticancer therapeutics, RSC Adv., 2018, 8(62), 35600–35610 RSC.
- H. Sun, B. Ni and A. Zhang, et al., Metabolomics study on Fuzi and its processed products using ultra-performance liquid-chromatography/electrospray-ionization synapt high-definition mass spectrometry coupled with pattern recognition analysis, Analyst, 2012, 137(1), 170–185 RSC.
- X. Wang, A. Zhang and X. Zhou, et al., An integrated chinmedomics strategy for discovery of effective constituents from traditional herbal medicine, Sci. Rep., 2016, 6, 18997 CrossRef CAS.
- A. Zhang, H. Sun and Y. Han, et al., Urinary metabolic biomarker and pathway study of hepatitis B virus infected patients based on UPLC-MS system, PLoS One, 2013, 8(5), e64381 CrossRef CAS.
- H. Xiong, A. H. Zhang and Q. Q. Zhao, et al., Discovery and screening quality-marker ingredients of Panax quinquefolius using chinmedomics approach, Phytomedicine, 2019, 152928 CrossRef.
- A. Zhang, H. Sun and P. Wang, et al., Salivary proteomics in biomedical research, Clin. Chim. Acta, 2013, 415, 261–265 CrossRef CAS.
- H. Cao, A. Zhang and H. Zhang, et al., The application of metabolomics in traditional Chinese medicine opens up a dialogue between Chinese and Western medicine, Phytother. Res., 2015, 29(2), 159–166 CrossRef.
- A. Zhang, H. Sun and X. Wang, Potentiating therapeutic effects by enhancing synergism based on active constituents from traditional medicine, Phytother. Res., 2014, 28(4), 526–533 CrossRef.
- X. Wang, A. Zhang and H. Sun, Power of metabolomics in diagnosis and biomarker discovery of hepatocellular carcinoma, Hepatology, 2013, 57(5), 2072–2077 CrossRef CAS.
- Y. Zhao, H. Lv and S. Qiu, et al., Plasma metabolic profiling and novel metabolite biomarkers for diagnosing prostate cancer, RSC Adv., 2017, 7(48), 30060–30069 RSC.
- A. Zhang, H. Sun and G. Yan, et al., Mass spectrometry-based metabolomics: applications to biomarker and metabolic pathway research, Biomed. Chromatogr., 2016, 30(1), 7–12 CrossRef.
- H. Fang, A. H. Zhang and H. Sun, et al., High-throughput metabolomics screen coupled with multivariate statistical analysis identifies therapeutic targets in alcoholic liver disease rats using liquid chromatography-mass spectrometry, J. Chromatogr. B: Anal. Technol. Biomed. Life Sci., 2019, 1109, 112–120 CrossRef CAS.
- A. Zhang, H. Sun and G. Yan, et al., Metabolomics in diagnosis and biomarker discovery of colorectal cancer, Cancer Lett., 2014, 345(1), 17–20 CrossRef CAS.
- H. Sun, A. Zhang and X. Wang, Potential role of metabolomic approaches for Chinese medicine syndromes and herbal medicine, Phytother. Res., 2012, 26(10), 1466–1471 CAS.
- A. Zhang, H. Sun and X. Wang, Emerging role and recent applications of metabolomics biomarkers in obesity disease research, RSC Adv., 2017, 7(25), 14966–14973 RSC.
- A. Zhang, H. Sun and Y. Han, et al., Exploratory urinary metabolic biomarkers and pathways using UPLC-Q-TOF-HDMS coupled with pattern recognition approach, Analyst, 2012, 137(18), 4200–4208 RSC.
- A. H. Zhang, J. B. Yu and H. Sun, et al., Identifying quality-markers from Shengmai San protects against transgenic mouse model of Alzheimer's disease using chinmedomics approach, Phytomedicine, 2018,(18), 30104–301011 Search PubMed.
- X. Wang, A. Zhang and Y. Han, et al., Urine metabolomics analysis for biomarker discovery and detection of jaundice syndrome in patients with liver disease, Mol. Cell. Proteomics, 2012, 11(8), 370–380 CrossRef.
- A. Zhang, H. Sun and S. Qiu, et al., Metabolomics insights into pathophysiological mechanisms of nephrology, Int. Urol. Nephrol., 2014, 46(5), 1025–1030 CrossRef CAS.
- X. N. Li, A. Zhang and M. Wang, et al., Screening the active compounds of Phellodendri Amurensis cortex for treating prostate cancer by high-throughput chinmedomics, Sci. Rep., 2017, 7, 46234 CrossRef CAS.
- X. Liu, A. Zhang and H. Fang, et al., Serum metabolomics strategy for understanding the therapeutic effects of Yin-Chen-Hao-Tang against Yanghuang syndrome, RSC Adv., 2018, 8, 7403–7413 RSC.
- H. Sun, A. H. Zhang and Q. Song, et al., Functional metabolomics discover pentose and glucuronate interconversion pathways as promising targets for Yang Huang syndrome treatment with Yinchenhao Tang, RSC Adv., 2018, 8, 36831–36839 RSC.
- A. Zhang, H. Sun and P. Wang, et al., Modern analytical techniques in metabolomics analysis, Analyst, 2012, 137(2), 293–300 RSC.
- X. J. Wang, J. L. Ren and A. H. Zhang, et al., Novel applications of mass spectrometry-based metabolomics in herbal medicines and its active ingredients: Current evidence, Mass Spectrom. Rev., 2019, 38(4–5), 380–402 CrossRef.
- H. Chu, A. Zhang and Y. Han, et al., Metabolomics approach to explore the effects of Kai-Xin-San on Alzheimer's disease using UPLC/ESI-Q-TOF mass spectrometry, J. Chromatogr. B: Anal. Technol. Biomed. Life Sci., 2016, 1015, 50–61 CrossRef.
- A. Zhang, H. Sun and P. Wang, et al., Salivary proteomics in biomedical research, Clin. Chim. Acta, 2013, 415, 261–265 CrossRef CAS.
- H. Sun, A. H. Zhang and S. B. Liu, et al., Cell metabolomics identify regulatory pathways and targets of magnoline against prostate cancer, J. Chromatogr. B: Anal. Technol. Biomed. Life Sci., 2018, 1102–1103, 143–151 CrossRef CAS.
- A. Zhang, H. Sun and G. Yan, et al., Metabolomics in diagnosis and biomarker discovery of colorectal cancer, Cancer Lett., 2014, 345(1), 17–20 CrossRef CAS.
- A. Zhang, H. Sun and Y. Han, et al., Exploratory urinary metabolic biomarkers and pathways using UPLC-Q-TOF-HDMS coupled with pattern recognition approach, Analyst, 2012, 137(18), 4200–4208 RSC.
- X. Li, A. Zhang and H. Sun, et al., Metabolic characterization and pathway analysis of berberine protects against prostate cancer, Oncotarget, 2017, 8, 65022–65041 Search PubMed.
- H. Sun, A. Zhang and G. Yan, et al., Metabolomic analysis of key regulatory metabolites in hepatitis C virus–infected tree shrews, Mol. Cell. Proteomics, 2013, 12(3), 710–719 CrossRef CAS.
- A. Zhang, H. Sun and P. Wang, et al., Future perspectives of personalized medicine in traditional Chinese medicine: a systems biology approach, Complement. Ther. Med., 2012, 20(1–2), 93–99 CrossRef.
- H. Zhang, A. Zhang and J. Miao, et al., Targeting regulation of tryptophan metabolism for colorectal cancer therapy: a systematic review, RSC Adv., 2019, 9, 3072–3080 RSC.
- A. Zhang, H. Sun and G. Yan, et al., Metabolomics for biomarker discovery: moving to the clinic, BioMed Res. Int., 2015, 2015, 1–6 Search PubMed.
- H. Sun, H. Wang and A. Zhang, et al., Berberine ameliorates nonbacterial prostatitis via multi-target metabolic network regulation, OMICS, 2015, 19(3), 186–195 CrossRef CAS.
- L. Wang, H. Dong and A. H. Zhang, et al., Exploring the detoxification effects and mechanism of Caowu in prescription using liquid chromatography-high-resolution mass spectrometry-based metabolomics, Open Journal of Proteomics and Genomics, 2018, 3(1), 011–023 Search PubMed.
- H. Fang, A. Zhang and J. Yu, et al., Insight into the metabolic mechanism of scoparone on biomarkers for inhibiting Yanghuang syndrome, Sci. Rep., 2016, 6, 37519 CrossRef CAS PubMed.
- Y. Nan, X. Zhou and Q. Liu, et al., Serum metabolomics strategy for understanding pharmacological effects of ShenQi pill acting on kidney yang deficiency syndrome, J. Chromatogr. B: Anal. Technol. Biomed. Life Sci., 2016, 1026, 217–226 CrossRef CAS.
- J. Xie, A. H. Zhang and S. Qiu, Identification of the perturbed metabolic pathways associating with prostate cancer cells and anticancer affects of obacunone, J. Proteomics, 2019, 206, 103447 CrossRef CAS.
- Q. Liang, H. Liu and X. Li, et al., High-throughput metabolomics analysis discovers salivary biomarkers for predicting mild cognitive impairment and Alzheimer's disease, RSC Adv., 2016, 6, 75499–75504 RSC.
Footnote |
† Electronic supplementary information (ESI) available. See DOI: 10.1039/c9ra07382e |
|
This journal is © The Royal Society of Chemistry 2019 |