DOI:
10.1039/C9RA06791D
(Paper)
RSC Adv., 2019,
9, 31425-31434
Understanding the R882H mutation effects of DNA methyltransferase DNMT3A: a combination of molecular dynamics simulations and QM/MM calculations†
Received
27th August 2019
, Accepted 17th September 2019
First published on 3rd October 2019
Abstract
DNA (cytosine-5)-methyltransferase 3A (DNMT3A), a key enzyme for de novo epigenetic methylation in human beings, was reported to undergo an R882H mutation in approximately 25% of M4/M5 subtype acute myeloid leukemia (AML) patients. In this work, a combination of classical molecular dynamics (MD) simulations and QM/MM calculation methods was utilized to reveal the molecular mechanism behind the activity attenuation caused by R882H mutation. We found that R882H mutation induces a “folded” conformation in the methyl donor S-adenosylmethionine (SAM) through different types of hydrogen bond formation at the terminal carbonyl oxygen atom and the hydroxyl O3′ atom of the ribose ring on SAM, with Arg891 as a mediator. Energetically, both the pre-reaction state (PRS) and transition state (TS) were stabilized in the R882H mutant. However, the energy barrier of the rate-determining step from the PRS to the TS was calculated to be roughly 1.0 kcal mol−1 larger in the R882H mutant than the WT. Also, a dynamic transformation occurred along the helix where R882H was located, tending to manifest in a quasi-“Newton's cradle” manner from the mutational site to the active site residues of DNMT3A. Our computational results provided molecular insights into the pathogenic R882H mutation and advanced the understanding of its mechanism.
Introduction
As one of the most important hallmarks of epigenetics, DNA methylation plays a critical role in metabolic and developmental science.1–5 Aberrant DNA methylation in the human genome is associated with the pathogenesis of severe diseases, like cancer.6 The determination of DNA methylation status and cancer classification via deep sequencing have been utilized increasingly frequently recently.7–9 Methyltransferases are classified into de novo and maintenance types, with de novo methyltransferases responsible for initializing the DNA methylation pattern during embryo development and the maintenance methyltransferase DNMT1 taking hemimethylated DNA as the substrate to maintain the methylation pattern. De novo methyltransferases can be further divided into DNMT3A and DNMT3B.10 In 2010, somatic mutations of DNMT3A were observed in a quarter of M4/M5 subtype acute myeloid leukemia (AML) patients,11 among which the R882H mutation presented the highest frequency (60%),12 in particular for seniors.13,14 It was reported that the DNA methylome tended to be globally hypomethylated in AML patients and this was accompanied by altered gene expression.15 The glutathione (GSH) metabolism was found to be affected by the R882H mutation with promoted malignant cell proliferation,16 and Tet2 inactivation, which can induce lymphoid malignancies, was cooperative with the R882H mutation.17
Owing to its significance in medical science, the R882H mutation has been intensively studied biochemically. Yamashita et al. observed a catalytic ability loss of over 2.3-fold from the R882H mutation through in vitro biochemical experiments.18 Blocked protein tetramerization was suggested to be one of the reasons for the decline in activity.19 On the other hand, Holz-Schietinger et al. reported a catalytic ability loss of 2.5-fold, accompanied by a 5.3-fold decrease in the poly-dIdC (DNA substrate) binding affinity.20 The DNA binding pattern was consistent with AML R882H pathogenesis,21 and a 40% reduction in DNMT3A catalytic ability was related to the flanking sequence preference.22 However, the mechanistic details relating to how the R882H mutation induces internal changes in the DNMT3A protein remain unknown.
Full-length DNMT3A consists of several domains: a flexible N-terminal domain; a proline-tryptophan-tryptophan-proline (PWWP) domain;23 an ATRX-like cysteine-rich domain; and the catalytic domain at the C-terminus.24,25 The active site is highly conserved in both eukaryotic and prokaryotic DNA methyltransferases.23–26 It consists of a large α/β catalytic core motif and a relatively small target recognition domain (TRD). R882 is located at the edge of the penultimate α-helix relative to the C terminal, relatively far from the catalytic pocket but spatially adjacent to the phosphate backbone of the substrate DNA (Fig. 1A and B). De novo cytosine C5-methylation occurs as the DNA substrate binds to the protein and its targeted cytosine is flipped outward from the DNA helical structure and implanted into the DNMT3A catalytic pocket, where the methyl group of the cofactor S-adenosylmethionine (SAM) is transferred onto the C5 atom of the target cytosine. Aranda et al. provided a relatively complete reaction energy profile of Haemophilus haemolyticus (M.HhaI) DNA methyltransferase, which shares a conserved catalytic domain with human DNMT3A.27,28 According to previous studies, the methyl transfer step is turn-over-frequency-determining (TOF-determining).26,27,29 The cytosine activation includes the deprotonation of Cys710 and subsequent addition, and methyl transfer to the flipped cytosine (Fig. 1C). Finally, the proton on C5 and the thiolate at C6 are eliminated to finalize the DNA methylation.30,31 The transition state of the methyl transfer step and its precursor thiolate adduct have been determined as the rate-determining (TDTS, TOF-determining TS) step from the resting state (TDI, TOF-determining intermediate) of the methyltransferase (Fig. 1D).32
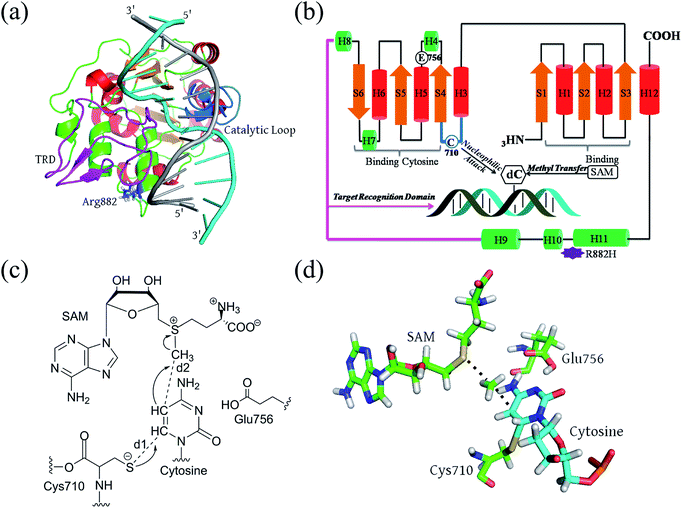 |
| Fig. 1 The structure and proposed reaction mechanism of human DNMT3A: (a) the 3D structure constructed via homology modeling; (b) the 2D topological structure with annotated secondary structures; (c) the TOF-determining step in the catalytic cycle; and (d) the TOF-determining transition structure of the methyl transfer step (TDTS). | |
In our recent papers, the pre-reaction states (PRSs), complexes of the substrate and the active site reactant, were successfully used to understand the substrate diversity of thioesterase.33,34 Here, the pre-reaction state (PRS) is also employed to understand the effects of R882H mutation on DNMT3A methylation. In practice, the pre-reaction state is defined as the product of the nucleophilic attack of Cys710 on cytosine. We have used a combination of classical molecular dynamics simulations (MD) and quantum mechanics/molecular mechanics (QM/MM) methods to investigate the structural and mechanical variations caused by R882H mutation on the monomer DNMT3A complex. First, the trajectory-based dynamic structural features of WT and R882H-mutated models were compared. Second, the reaction barrier of the methyl-transfer step was computed via a 2-layer ONIOM method to explore the structural and energetics variations. Finally, we provided a detailed explanation of the distal effects brought about by this mutation.
Materials and methods
Model construction
The crystal structure of the DNMT3A catalytic domain was downloaded from the RCSB Protein Data Bank (PDB ID: 2QRV),35 and the missing target recognition domain (TRD) was completed using Rosetta 3.5 software.36 500 homology models were generated, and the most representative one was selected as the apo-DNMT3A model (Fig. S1†). The methyl-donating cofactor SAM and the substrate DNA of the holo-model were extracted from the M.HhaI methyltransferase crystal structure (PDB ID: 2HR1). Both apo- and holo-models were subjected to the PROCHECK program37 to validate the geometric quality. In the case of the apo-structure, the residue percentages for the core, allowed, generally-allowed, and disallowed regions were 91.5%, 8.5%, 0.0%, and 0.0%, respectively. For the holo-structure, the corresponding percentages were 89.9%, 8.9%, 1.2%, and 0.0%, respectively (Fig. S2†). Discovery Studio 3.5 was used to mutate R882 to H882 in both the apo- and holo-models, named WT-apo, Mut-apo, WT-holo, and Mut-holo in the following discussion. The protonation state was determined using the PROPKA program on the PDB2PQR web server at pH 7.0.38 In particular, the δ-nitrogen of H882 is protonated in the mutant. A recently reported crystal structure of the DNMT3A-cofactor-substrate protein complex was also downloaded from RCSB Protein Data Bank (PDB ID: 5yx2) for structural verification. The D chain was extracted from the tetramer structure to serve as a holo-model. The protonation states of the crystal holo-models were the same as the homology models. The crystal models are named WT-holo and HIS-holo.
Molecular dynamics simulations
Two types of simulations were performed: multiple classical molecular dynamics simulations (cMD) on the homology models and accelerated molecular dynamics simulations (aMD) on the crystal models to confirm the conformational space. The AMBER12 GPU version39 with the PMEMD engine was employed for both classical and accelerated molecular dynamics simulations, using the ff03 force field.40 The force field parameters of the cofactor SAM were generated with the Antechamber module in AMBER. The charge distribution was calculated via the simple AM1-BCC method, which gave dynamic structures similar to those in the literature.41 Standard LEAP treatment was applied to neutralize the net charges, after which the system was placed in a TIP3P water environment with a thickness of 10.0 Å.42 The particle mesh Ewald (PME) method was exploited to model long-range electrostatic interactions with periodic boundary conditions.43 Initially, 1000-step steepest descent minimization was carried out, followed by 9000 steps of conjugate gradient minimization. Afterward, gradual heating from 0 to 300 K with the restraint of 5 kcal mol−1 Å2 of the protein, SAM, and DNA solutes was performed. These systems were then equilibrated at 300 K in an NPT ensemble for 500 ps with a step time of 2 fs. The SHAKE algorithm44 was used to constrain the hydrogen bonds during the whole procedure. The continuous 50 ns production of classical molecular dynamics (cMD) simulations was carried out in an NPT ensemble, with constant pressure set to 1 bar. The same simulation was repeated using ten independent replicates of each apo- and holo-homology model. 100 ns accelerated molecular dynamics simulations were performed on the crystal holo-models in the NPT ensemble as well. Structural analyses, such as root-mean-square deviation (RMSD), root-mean-square fluctuation (RMSF) and cluster analysis, were conducted with the CPPTRAJ tool, implemented in AMBER12. The acceptor–donor distance cutoff was set to 3.0 Å and the acceptor–hydrogen–donor angle cutoff was set to 135° to define a hydrogen bond. The hydrogen-bonding occupancy was measured via the frequency of appearance in the trajectories.
Quantum mechanics/molecular mechanics (QM/MM) calculations
QM/MM calculations were performed with a two-layered ONIOM method,45,46 using the Gaussian 09 program.47 A geometrical snapshot with the lowest RMSD value compared with the average structure of all 10 cMD trajectories (250
000 frames) was extracted for the QM/MM calculations, to ensure that the geometry selected was the most representative for the protein–ligand complex. The QM region included the catalytically essential residues Cys710 and Glu756, the target cytosine fragment, and the cofactor SAM. The QM region possessed 105 atoms in total, bearing a negative charge. The whole WT and R882H DNMT3A systems contained charges of −15 and −16, respectively, before neutralization with sodium cations. The transition state (TS) was determined via a two-dimensional potential energy scan with the B3LYP density functional and the 6-31G(d) basis set, while the MM region was treated with the AMBER parm99 force field implemented in Gaussian 09 software. Two reaction coordinates were used: the distance between the sulfur atom of SAM and the carbon atom of the transferred methyl group; and distance between the carbon atom of the methyl group and the C5 atom of the target cytosine. The energy barrier was estimated based on the single point optimization energy difference between the PRS and the transition state (TS) structures. For energy validation, the DFT calculations were repeated with the M06-2X and ωB98x-D functionals and three different basis sets, 6-31G(d), 6-311G(d), and 6-311+G(d). The modest estimations obtained using B3LYP/6-31G(d) are presented in the paper, while the M06-2x and ωB98x-D results are listed in Table S4.†
Molecular mechanics/generalized born surface area method
The MM/GBSA method48 was applied during the last 10 ns of each trajectory at intervals of 10 ps to calculate the binding free energies between the cofactor SAM and the enzyme. The entropy was also estimated via the normal mode analysis (NMA) method.49 Results from each trajectory were calculated individually and analyzed statistically.
Dynamical pathway analysis
Protein 3D structures were transformed into 2D undirected networks to explore the contact interactions between residues.50 In this work, amino acid residues were set as nodes, and the contact interactions between residues were denoted as edges if the distance between the mass centers of the two residues was less than 3.5 Å during the simulation time. The optimal (shortest) pathway and suboptimal pathways between the mutational site and the residues affected by R882H mutation were all calculated with the WISP Python package.51 One hundred pathways for each independent cMD trajectory were collected and the most probable one was determined based on the node degeneracy of all calculated pathways.
Statistical analysis
Two sample t-testing was performed to test the statistical significance of the calculated results, such as distances, angles, dihedral angles, RMSF values, etc.
Results and discussion
Structural variances upon R882H mutation
According to the root-mean-square deviation (RMSD), all 40 cMD trajectories (ten independent simulations for each homology system: WT-apo; Mut-apo; WT-holo; and Mut-holo) reached equilibrium after the first 10 ns of simulation (Fig. S3†). The structural integrity of the protein remained, as no significant variations in amino acid fluctuations were captured between wild type (WT) and R882H-mutated DNMT3A (Fig. S4†). The average structures from 10 replicate trajectories for each holo-model were used to compare the structural differences between WT and R882H-mutated DNMT3A (Fig. 2A). Besides the most flexible target recognition domain (TRD) region, large structural variations also manifested in two helices: the 2nd helix (H2, consisting of Glu667–His677) and the 11th helix (H11, where Arg882 located). In the R882H mutant, the H2 helix deviated from wild type DNMT3A by about 2.0–5.1 Å, with the maximum at Asp668, and the helix H11 deviated by 0.9–3.2 Å, with the maximum at the mutational site Arg882. These two helices were connected with loop regions that directly bind to the cofactor SAM, implying that the binding pattern of SAM could be changed upon R882H mutation.
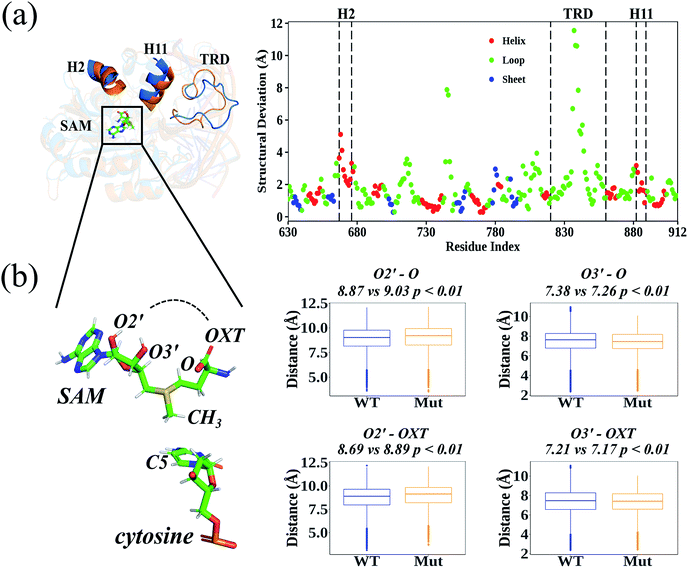 |
| Fig. 2 The structural variations caused by R882H mutation: (a) the structural deviations between the average structures of WT and R882H-mutated homology holo-models; and (b) a structural representation of the distances between hydroxyl O2′/O3′ atoms and the terminal O (carbonyl oxygen)/OXT (carboxyl oxygen) atoms, and the corresponding statistical t-test results. The unit of distance is the angstrom. | |
In order to analyze the conformational variations of SAM, all the dihedrals were analyzed in both the homology and crystal DNMT3A holo-models (Fig. S5 and S6†). Adenosine was quite stable at the active site, as the dihedrals upon it were nearly identical in WT and R882H-mutated DNMT3A. However, the glycosidic bond between adenosine and the ribose ring rotated, subsequently causing variations in the dihedrals on the ribose ring and in the amino acid portion. These variations caused the distances between the hydroxyl O2′ atom of the ribose ring and the amino acid terminal oxygen atoms to rise and the distances between the hydroxyl O3′ atom and the terminal oxygen atoms to fall; as a result, the linear portion of SAM cyclized and exhibited a ‘folded’ conformation (Fig. 2B). This geometrical variance appeared more obviously in the crystal model, where all these distances tended to shrink, and SAM took on the structure of a “folded” molecule in the R882H mutant (Fig. S7†). Both the homology and crystal models showed that the distance between the hydroxyl O3′ atom on the ribose ring and the terminal carbonyl oxygen atom (termed as O) significantly decreased in the mutant. The relationship between the critical conformational change of SAM and the DNMT3A methylation ability is discussed in detail below.
Hydrogen-bonding interactions of the cofactor SAM
Next, the binding patterns between DNMT3A and SAM were carefully examined to explore the mechanisms behind the geometrical disturbance of SAM through the MMGBSA method (Table S1†). Consistent with previous experimental results,20 the binding free energy was smaller by approximately 0.8 kcal mol−1 in the R882H mutant compared to WT DNMT3A, implying weaker interactions between DNMT3A and the cofactor SAM in the R882H mutant. There are explanations accounting for the decreased binding affinity between DNMT3A and the cofactor SAM. It has previously been validated that wild type DNMT3A forms a heterotetramer with DNMT3L through an ‘FF’ interface.19 DNMT3L was reported to function as a stimulator of DNMT3A methylation activity through stabilizing the interaction between DNMT3A and the cofactor SAM.52 It has also been reported that R882H mutation could induce DNMT3A to terminate interactions with DNMT3L and become a homodimer, giving rise to a decreased binding affinity between DNMT3A and the cofactor SAM, which has been validated via independent experiments.20 To further explore the binding details, free energy decomposition based on residue level was performed (Table S2†). Phe640, Thr645, Glu664 and Val665, Arg891–Trp893, and Asp686 played dominant roles in DNMT3A and SAM binding, in agreement with a previous report.25 In comparison with the wild type protein, the contribution of Arg891–Trp893 to the binding free energy became larger in the R882H mutant while the contribution of Thr645 became smaller, confirming the molecular interaction changes around the cofactor SAM.
Hydrogen-bonding interactions between DNMT3A and SAM were thoroughly explored using all cMD and aMD trajectories (Fig. 3 and Table S3†). The occupancy of the hydrogen bond between the oxygen atom of the peptide bond of Phe640 and the nitrogen atom of the SAM adenosyl moiety was decreased upon R882H mutation. The hydrogen-bonding occupancy between the oxygen atom of the Thr645 sidechain and the carboxyl oxygen atom (OXT) of the amino acid terminus of SAM was also decreased. Hydrogen bonds between the oxygen atoms of the Asp686 sidechain and the nitrogen atom of the SAM adenosyl moiety were relatively stable when comparing the two systems. As for the Arg891–Trp893 region, the frequencies of hydrogen bonding between these residues and the oxygen atoms (both O and OXT) of SAM were increased. We paid particular attention to Arg891, whose guanidyl group exhibited dynamic interactions with SAM. A pattern could be generalized where WT DNMT3A had an advantage over the R882H mutant in terms of the hydrogen bonding proportion between the Arg891 guanidyl group and the hydroxyl on the SAM ribose ring (i.e., O2′ and O3′), whereas WT bore a lower frequency of hydrogen bonds between the Arg891 guanidyl group and the O and OXT atoms on SAM in comparison with the R882H mutant. It appeared that the hydrogen bonds that Arg891 initially formed with hydroxyl oxygen atoms were ‘replaced’ with bonds with oxygen atoms on the SAM terminus. It was speculated that Arg891 served to pull the amino acid terminus towards the ribose ring of SAM, in accordance with the favorable ‘folded’ conformation of SAM in the R882H mutant.
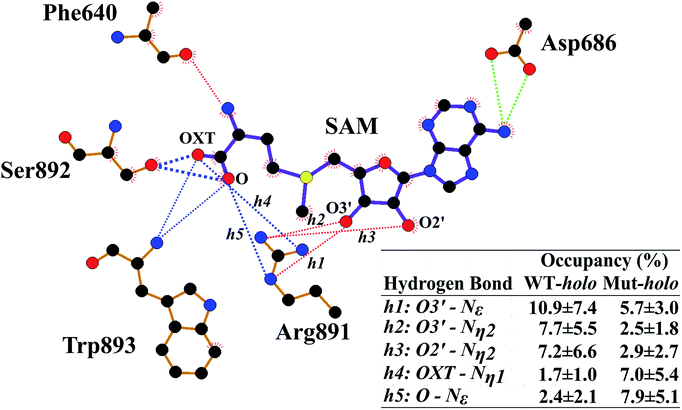 |
| Fig. 3 The hydrogen-bonding network distinctions around SAM upon R882H mutation. The light green dotted lines show hydrogen bonds that are conserved in both WT and R882H-mutated DNMT3A, the red dotted lines represent those that decline in the R882H mutant, and the blue dotted lines represent those that strengthen in the R882H mutant. The line weights accord with the hydrogen-bonding occupancy. | |
Reaction barrier of the rate-determining step
The energy profile of the rate-determining methyl transfer step was calculated using QM/MM methods. With the least-RMSD structure, the energy barriers of the methyl migration step were computed to be 14.9 kcal mol−1 and 15.6 kcal mol−1 for WT and R882H DNMT3A, respectively, similar to the values reported in the literature.27,31 The catalytic energy barrier increased slightly, by 0.7 kcal mol−1, in the R882H mutant, which is consistent with the 4-fold reduction in the catalytic ability of monomer DNMT3A from experimental observations.18–20 To validate the robustness of our results, QM/MM calculations with a combination of the M06-2X functional and various basis sets were further carried out (Table S4†). Overall, our calculations suggested a 1 kcal mol−1 reaction barrier increase in the case of R882H mutation. The distortion/interaction model developed by Bickelhaupt and Houk53 was introduced in our analysis to describe the energy variations in the methyl transfer step. The molecular mechanics (MM) and quantum mechanics (QM) relative energies of the two-layers in the ONIOM calculations were considered as the ‘protein distortion’ and ‘core interaction’ energies, conceptually mimicking the real distortion and interaction energies in the activation strain model that is widely used in organic and inorganic chemistry.53 Interestingly, R882H mutation lowered the ‘distortion’ energies of the protein layer (MM region) by 5.0 and 14.7 kcal mol−1 in the pre-reaction state (PRS) and transition state (TS), respectively, using a non-active structure (before covalent bond formation between Cys710 and cytosine) as an energy reference. The ‘core interaction’ energies that correspond to the energies in the QM region varied between the PRS and TS – the PRS complex was stabilized through R882H mutation by 4.9 kcal mol−1, while the TS complex was destabilized by 5.5 kcal mol−1. Taken together, and compared to WT, we could see a 9.2 (14.7–5.5) kcal mol−1 energy reduction for the TS in the R882H mutant, and a 9.9 (5.0 + 4.9) kcal mol−1 energy decrease for the PRS. Given that the energy barrier is defined as the difference between the TS and PRS, R882 mutation endowed the methyl transfer reaction with an overall 0.7 kcal mol−1 increase in energy (Fig. 4 and Table S5†).
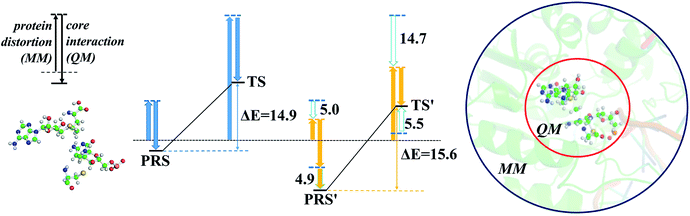 |
| Fig. 4 The ‘protein distortion’ and ‘core interaction’ energies of the MM and QM layers from ONIOM calculations for the methyl transfer step (the energy units are kcal mol; WT DNMT3A is colored blue; R882H-mutated DNMT3A is colored orange). | |
Geometric superpositions of the PRS structures revealed distinctive S–CH3–C5–C6 dihedral values in WT and R882H-mutated DNMT3A of around 107° and 63°, respectively, suggesting the conformational change of SAM (Fig. 5). Specifically, in WT DNMT3A, Phe640 and Thr645 could form hydrogen bonds with SAM, while in the R882H mutant, Arg891 undertook a dominant role in hydrogen bonding with the amino acid moiety of SAM; this is consistent with the hydrogen-bonding patterns discovered during MD simulations. The hydrogen-bonding network variations led to the formation of an intramolecular hydrogen bond between O3′ on the ribose ring and the terminal O atom, which was observed in both the PRS and TS of the R882H mutant. The formation of the hydrogen bond again corresponded to a decreased distance between these two atoms, which was captured in ground state simulations, verifying the advantageous ‘folded’ conformation of SAM. Furthermore, the fewer hydrogen interactions with SAM observed in the TS of the R882H mutant accorded with the increased ‘core interaction’ energy (destabilization in the QM region) found in the reaction barrier profile (Fig. 5).
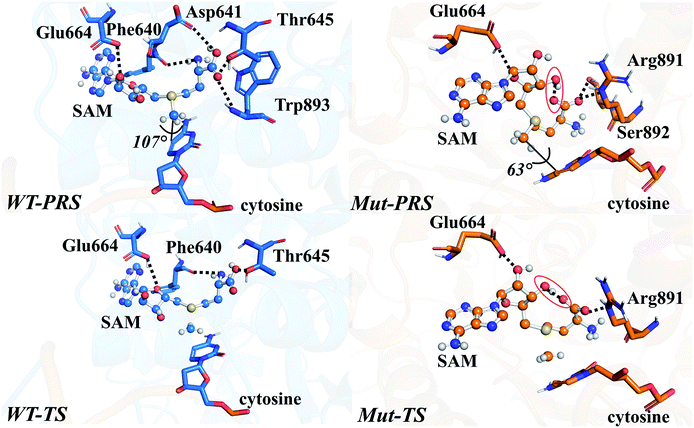 |
| Fig. 5 The structural representations and hydrogen-bonding patterns in the QM regions of WT-PRS, Mut-PRS, WT-TS, and Mut-TS systems, with WT DNMT3A colored blue and R882H-mutated DNMT3A colored orange; hydrogen bonds are shown as black dashed lines; the hydrogen bonds formed within SAM are highlighted in red circles; SAM is shown as a ball-and-stick model, while other residues are shown as sticks. | |
Previously, Lau et al. carried out a near attack conformation (NAC) study on M.HhaI DNA methyltransferase,54 generating the geometrical parameters of SAM, including the glycosidic torsion angle χ (O4′–C1′–N1–C2), the angle formed by cytosine C4 and C5, and CH3 (C4–C5–CH3), and the angle formed by cytosine C6 and C5, and CH3 (C6–C5–CH3). These parameters were applied in our study to check the geometrical availability of cytosine and SAM for performing DNA C5-methylation. From this investigation, the population of NAC conformers within the geometrical criteria decreased upon R882H mutation, indicating the transformation of both SAM and cytosine into less reactive analogues (Table S6†). Besides, using dihedral S–CH3–C5–C6 in the PRS as a reference, the populations of PRS-like conformations in the cMD trajectories were 3.2% and 0.3% for the WT and the R882H mutant, respectively, demonstrating a 9.8-fold decrease upon mutation. For the crystal models, the PRS-like populations were 4.2% and 1.1%, exhibiting a 3.8-fold decrease. Both geometrical parameters and conformation classification showed that WT DNMT3A was more reactive, validating the effects of mutation on the geometrical characteristics of both the PRS and TS complexes. Interestingly, entropy compensation seemed to be consistent with the enthalpy calculations in the current case. The pre-reactive population was inversely associated with the reaction barrier: a higher population of the pre-reaction state was accompanied by a lower reaction barrier. This indicated that the relative positions of the substrate and cofactor at the catalytic active site could have nontrivial impact on the enzyme activity.
Distal effects of R882H mutation
The internal transduction was described via dynamical pathways based on amino acid contact analysis. For each cMD trajectory, 100 suboptimal possible pathways from the source (Arg882 or His882) to the target residues (Phe640, Thr645, Arg891, and Ser892) were calculated from the residue-contact network, and the one with the largest degeneracy of residues from all 1000 suboptimal pathways was designated as the most probable pathway. The pathway length distribution showed that shorter pathways were taken in the R882H mutant to transfer dynamic contact information from the mutational site to Arg891, which could account for the favorability for folded SAM in the holo-models (Fig. S8†). Accordingly, an analysis of the most probable pathway showed that site 882 could establish communications with Arg891 and Ser892 along the H11 helix, as shown in Fig. 6.
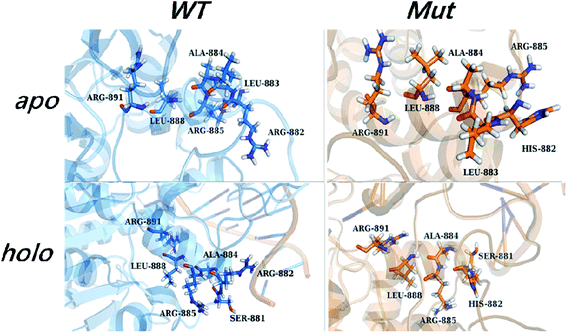 |
| Fig. 6 The most probable pathway from site 882 to Arg891 in the apo- and holo-models of the wild type and mutant, from amino acid contact analysis. | |
Owing to the rigidity of the helical structure,55 the distal effects are communicated in the fashion of quasi-“Newton's cradle” mechanism (Fig. 7). Steric repulsion was generated at the R882H site and transmitted through the stationary residues on H11, finally reaching the cofactor SAM at the catalytic site. Both homology and crystal models showed that the steric interactions between the sidechains of Arg882/His882 and Leu883 varied after mutation, with the sidechains of Leu883 and Arg887 being pushed towards the catalytic site. The shorter distance caused stronger hydrophobic interactions between the Leu883 and Arg887 sidechains and induced larger repulsive forces between the sidechains of Arg887 and Arg891 (Table S7†). Ultimately, the conformational changes of Arg891 and other residues at the active site gave rise to the more difficult fulfillment of the methyl transfer reaction.
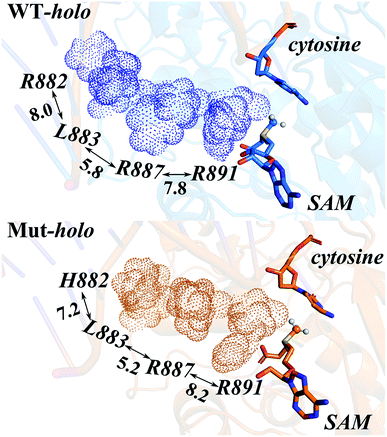 |
| Fig. 7 The quasi-“Newton's cradle” mechanism of dynamic information transduction from Arg882/His882 to Arg891 in WT DNMT3A and the R882H mutant. WT DNMT3A is colored blue and the R882H mutant is colored orange. | |
Conclusions
In this paper, the dynamic and mechanistic consequences of DNMT3A ‘hotspot’ R882H mutation in AML were investigated. Conformational analysis revealed that the cofactor SAM exhibited a preference for a ‘folded’ conformation in the mutated protein in both homology and crystal models. Hydrogen-bonding networks were used to further interpret the conformational alterations of SAM: Arg891 changed its interaction network pattern through forming more hydrogen bonds with the amino acid portion of SAM and reducing its interactions with the ribose ring. The conformational changes of SAM further induced an increase in the reaction barrier of the rate-determining step through forming less reactive geometries. Even though the protein was exposed to less structural distortion of the energy profile in both pre-reaction state (PRS) and transition state (TS) complexes following mutation, the overall reaction barrier was still estimated to rise by about 1 kcal mol−1. The distal transmission from the mutational site 882 to the active site was rationalized through internal dynamic information cascades occurring via a quasi-“Newton's cradle” mechanism on the helix where R882 is located. Therefore, the mechanistic consequences related to R882H mutation on monomer DNMT3A could be primarily attributed to the conformational changes of the cofactor SAM and the abnormal inter-residual communications induced by the aberrant pre-organization of DNMT3A.
Author contributions
YLZ and TS conceived and designed the investigation, LLX and TS performed the energetic calculations and analyses, and LLX, TS, KNH, and YLZ wrote up the paper.
Conflicts of interest
The authors declare no competing financial interests.
Acknowledgements
This work is supported by grants from the National Key R&D Program of China (2018YFA0901200), National Basic Research Program of China “973” (2013CB966802 and 2012CB721005), and National Science Foundation of China (31970041, 31770070, and 21377085), and by the SJTU-HPC computing facility.
References
- L. D. Moore, T. Le and G. Fan, Neuropsychopharmacology, 2013, 38, 23–38 CrossRef CAS.
- A. Bird, Genes Dev., 2002, 16, 6–21 CrossRef CAS.
- Z. D. Smith and A. Meissner, Nat. Rev. Genet., 2013, 14, 204–220 CrossRef CAS.
- D. M. Messerschmidt, B. B. Knowles and D. Solter, Genes Dev., 2014, 28, 812–828 CrossRef CAS.
- F. Chiacchiera, A. Piunti and D. Pasini, Cell. Mol. Life Sci., 2013, 70, 1495–1508 CrossRef CAS.
- I. P. Pogribny and F. A. Beland, Cell. Mol. Life Sci., 2009, 66, 2249–2261 CrossRef CAS PubMed.
- R. Kandimalla, A. A. van Tilborg and E. C. Zwarthoff, Nat. Rev. Urol., 2013, 10, 327–335 CrossRef CAS.
- M. S. Kim, J. Lee and D. Sidransky, Cancer Metastasis Rev., 2010, 29, 181–206 CrossRef CAS.
- M. H. Tao and J. L. Freudenheim, Epigenetics, 2010, 5, 491–498 CrossRef CAS.
- T. Chen and E. Li, Curr. Top. Microbiol. Immunol., 2006, 301, 179–201 CAS.
- T. J. Ley, L. Ding, M. J. Walter, M. D. McLellan, T. Lamprecht, D. E. Larson, C. Kandoth, J. E. Payton, J. Baty and J. Welch, N. Engl. J. Med., 2010, 363, 2424–2433 CrossRef CAS.
- X.-J. Yan, J. Xu, Z.-H. Gu, C.-M. Pan, G. Lu, Y. Shen, J.-Y. Shi, Y.-M. Zhu, L. Tang and X.-W. Zhang, Nat. Genet., 2011, 43, 309–315 CrossRef CAS.
- E. Papaemmanuil, M. Gerstung, L. Bullinger, V. I. Gaidzik, P. Paschka, N. D. Roberts, N. E. Potter, M. Heuser, F. Thol, N. Bolli, G. Gundem, P. Van Loo, I. Martincorena, P. Ganly, L. Mudie, S. McLaren, S. O'Meara, K. Raine, D. R. Jones, J. W. Teague, A. P. Butler, M. F. Greaves, A. Ganser, K. Döhner, R. F. Schlenk, H. Döhner and P. J. Campbell, N. Engl. J. Med., 2016, 374, 2209–2221 CrossRef CAS.
- T. Schoofs, W. Berdel and C. Müller-Tidow, Leukemia, 2014, 28, 1–14 CrossRef CAS.
- J. Xu, Y.-Y. Wang, Y.-J. Dai, W. Zhang, W.-N. Zhang, S.-M. Xiong, Z.-H. Gu, K.-K. Wang, R. Zeng and Z. Chen, Proc. Natl. Acad. Sci. U. S. A., 2014, 111, 2620–2625 CrossRef CAS.
- L. Yang, Y. N. Liu, N. Zhang, X. Y. Ding, W. Zhang, K. F. Shen, L. Huang, J. F. Zhou, S. Cui, Z. M. Zhu, Z. Hu and M. Xiao, Oncotarget, 2017, 8, 30395–30409 Search PubMed.
- L. Scourzic, L. Couronne, M. T. Pedersen, V. Della Valle, M. Diop, E. Mylonas, J. Calvo, E. Mouly, C. K. Lopez, N. Martin, M. Fontenay, A. Bender, S. Guibert, P. Dubreuil, P. Dessen, N. Droin, F. Pflumio, M. Weber, P. Gaulard, K. Helin, T. Mercher and O. A. Bernard, Leukemia, 2016, 30, 1388–1398 CrossRef CAS.
- Y. Yamashita, J. Yuan, I. Suetake, H. Suzuki, Y. Ishikawa, Y. Choi, T. Ueno, M. Soda, T. Hamada and H. Haruta, Oncogene, 2010, 29, 3723–3731 CrossRef CAS.
- D. A. Russler-Germain, D. H. Spencer, M. A. Young, T. L. Lamprecht, C. A. Miller, R. Fulton, M. R. Meyer, P. Erdmann-Gilmore, R. R. Townsend and R. K. Wilson, Cancer Cell, 2014, 25, 442–454 CrossRef CAS.
- C. Holz-Schietinger, D. M. Matje and N. O. Reich, J. Biol. Chem., 2012, 287, 30941–30951 CrossRef CAS PubMed.
- Z.-M. Zhang, R. Lu, P. Wang, Y. Yu, D. Chen, L. Gao, S. Liu, D. Ji, S. B. Rothbart, Y. Wang, G. G. Wang and J. Song, Nature, 2018, 554, 387–391 CrossRef CAS.
- M. Emperle, A. Rajavelu, S. Kunert, P. B. Arimondo, R. Reinhardt, R. Z. Jurkowska and A. Jeltsch, Nucleic Acids Res., 2018, 46, 3130–3139 CrossRef CAS.
- O. V. Lukashevich, N. A. Cherepanova, R. Z. Jurkovska, A. Jeltsch and E. S. Gromova, BMC Biochem., 2016, 17, 1–10 CrossRef PubMed.
- X. D. Cheng and R. M. Blumenthal, Structure, 2008, 16, 341–350 CrossRef CAS.
- H. Gowher, P. Loutchanwoot, O. Vorobjeva, V. Handa, R. Z. Jurkowska, T. P. Jurkowski and A. Jeltsch, J. Mol. Biol., 2006, 357, 928–941 CrossRef CAS.
- E. G. Malygin and S. Hattman, Crit. Rev. Biochem. Mol. Biol., 2012, 47, 97–193 CrossRef CAS.
- J. Aranda, K. Zinovjev, K. Swiderek, M. Roca and I. Tunon, ACS Catal., 2016, 6, 3262–3276 CrossRef CAS.
- F. Chédin, Prog. Mol. Biol. Transl. Sci., 2011, 101, 255–285 Search PubMed.
- D. M. Matje, D. F. Coughlin, B. A. Connolly, F. W. Dahlquist and N. O. Reich, Biochemistry, 2011, 50, 1465–1473 CrossRef CAS.
- X. Zhang and T. C. Bruice, Proc. Natl. Acad. Sci. U. S. A., 2006, 103, 6148–6153 CrossRef CAS.
- J. Yang, L. Lior-Hoffmann, S. Wang, Y. Zhang and S. Broyde, Biochemistry, 2013, 52, 2828–2838 CrossRef CAS.
- S. Kozuch and S. Shaik, Acc. Chem. Res., 2011, 44, 101–110 CrossRef CAS.
- X.-P. Chen, T. Shi, X.-L. Wang, J. Wang, Q. Chen, L. Bai and Y.-L. Zhao, ACS Catal., 2016, 6, 4369–4378 CrossRef CAS.
- T. Shi, L. Liu, W. Tao, S. Luo, S. Fan, X.-L. Wang, L. Bai and Y.-L. Zhao, ACS Catal., 2018, 8, 4323–4332 CrossRef CAS.
- D. Jia, R. Z. Jurkowska, X. Zhang, A. Jeltsch and X. Cheng, Nature, 2007, 449, 248–251 CrossRef CAS.
- R. Das and D. Baker, Annu. Rev. Biochem., 2008, 77, 363–382 CrossRef CAS.
- R. A. Laskowski, M. W. MacArthur, D. S. Moss and J. M. Thornton, J. Appl. Crystallogr., 1993, 26, 283–291 CrossRef CAS.
- D. C. Bas, D. M. Rogers and J. H. Jensen, Proteins, 2008, 73, 765–783 CrossRef CAS.
-
D. A. Case, T. A. Darden, T. E. Cheatham III, C. L. Simmerling, J. Wang, R. E. Duke, R. Luo, R. C. Walker, W. Zhang, K. M. Merz, B. Roberts, S. Hayik, A. Roitberg, G. Seabra, J. Swails, A. W. Götz, I. Kolossváry, K. F. Wong, F. Paesani, J. Vanicek, R. M. Wolf;J. Liu, X. Wu, S. R. Brozell, T. Steinbrecher, H. Gohlke, Q. Cai, X. Ye, J. Wang, M.-J. Hsieh, G. Cui, D. R. Roe, D. H. Mathews, M. G. Seetin, R. Salomon-Ferrer, C. Sagui, V. Babin, T. Luchko, S. Gusarov, A. Kovalenko and P. A. Kollman, AMBER 12, University of California, San Francisco, CA, 2012 Search PubMed.
- J. Wang, W. Wang, P. A. Kollman and D. A. Case, J. Mol. Graphics Modell., 2006, 25, 247–260 CrossRef CAS.
-
(a) A. Jakalian, B. L. Bush, D. B. Jack and C. I. Bayly, J. Comput. Chem., 2000, 21, 132–146 CrossRef CAS;
(b) D. A. Saez and E. Vohringer-Martinez, J. Comput.-Aided Mol. Des., 2015, 29, 951–961 CrossRef CAS.
- W. L. Jorgensen, J. Chandrasekhar, J. D. Madura, R. W. Impey and M. L. Klein, J. Chem. Phys., 1983, 79, 926–935 CrossRef CAS.
- T. Darden, D. York and L. Pedersen, J. Chem. Phys., 1993, 98, 10089–10092 CrossRef CAS.
- J. P. Ryckaert, G. Ciccotti and H. J. C. Berendsen, J. Comput. Phys., 1977, 23, 327–341 CrossRef CAS.
- T. Vreven, M. Frisch, K. Kudin, H. Schlegel and K. Morokuma, Mol. Phys., 2006, 104, 701–714 CrossRef CAS.
- T. Vreven, K. S. Byun, I. Komáromi, S. Dapprich, J. A. Montgomery Jr, K. Morokuma and M. J. Frisch, J. Chem. Theory Comput., 2006, 2, 815–826 CrossRef CAS.
-
M. J. Frisch, G. W. Trucks, H. B. Schlegel, G. E. Scuseria, M. A. Robb, J. R. Cheeseman, G. Scalmani, V. Barone, B. Mennucci, G. A. Petersson, H. Nakatsuji, M. Caricato, X. Li, H. P. Hratchian, A. F. Izmaylov, J. Bloino, G. Zheng, J. L. Sonnenberg, M. Hada, M. Ehara, K. Toyota, R. Fukuda, J. Hasegawa, M. Ishida, T. Nakajima, Y. Honda, O. Kitao, H. Nakai, T. Vreven, J. A. Montgomery Jr, J. E. Peralta, F. Ogliaro, M. Bearpark, J. J. Heyd, E. Brothers, K. N. Kudin, V. N. Staroverov, R. Kobayashi, J. Normand, K. Raghavachari, A. Rendell, J. C. Burant, S. S. Iyengar, J. Tomasi, M. Cossi, N. Rega, N. J. Millam, M. Klene, J. E. Knox, J. B. Cross, V. Bakken, C. Adamo, J. Jaramillo, R. Gomperts, R. E. Stratmann, O. Yazyev, A. J. Austin, R. Cammi, C. Pomelli, J. W. Ochterski, R. L. Martin, K. Morokuma, V. G. Zakrzewski, G. A. Voth, P. Salvador, J. J. Dannenberg, S. Dapprich, A. D. Daniels, O. Farkas, J. B. Foresman, J. V. Ortiz, J. Cioslowski and D. J. Fox, Gaussian 09, Gaussian, Inc., Wallingford, CT, 2013 Search PubMed.
- J. M. J. Swanson, R. H. Henchman and J. A. McCammon, Biophys. J., 2004, 86, 67–74 CrossRef CAS.
- D. A. Case, Curr. Opin. Struct. Biol., 1994, 4, 285–290 CrossRef CAS.
- V. A. Feher, J. D. Durrant, A. T. Van Wart and R. E. Amaro, Curr. Opin. Struct. Biol., 2014, 25, 98–103 CrossRef CAS.
- A. T. Van Wart, J. Durrant, L. Votapka and R. E. Amaro, J. Chem. Theory Comput., 2014, 10, 511–517 CrossRef CAS.
- M. S. Kareta, Z. M. Botello, J. J. Ennis, C. Chou and F. Chédin, J. Biol. Chem., 2006, 281, 25893–25902 CrossRef CAS.
- F. M. Bickelhaupt and K. N. Houk, Angew. Chem., Int. Ed., 2017, 56, 10070–10086 CrossRef CAS.
- E. Y. Lau and T. C. Bruice, J. Mol. Biol., 1999, 293, 9–18 CrossRef CAS.
- Y. D. Wu and Y. L. Zhao, J. Am. Chem. Soc., 2001, 123, 5313–5319 CrossRef CAS PubMed.
Footnote |
† Electronic supplementary information (ESI) available. See DOI: 10.1039/c9ra06791d |
|
This journal is © The Royal Society of Chemistry 2019 |
Click here to see how this site uses Cookies. View our privacy policy here.