DOI:
10.1039/C9RA06706J
(Paper)
RSC Adv., 2019,
9, 32034-32046
Computational fluid dynamics simulation as a tool for optimizing the hydrodynamic performance of membrane bioreactors
Received
26th August 2019
, Accepted 1st October 2019
First published on 9th October 2019
Abstract
The hydrodynamic properties and shear stresses experienced by a membrane bioreactor (MBR) are directly related to its rate of membrane fouling. In this study, computational fluid dynamic models have been combined with cold model PIV experimental studies to optimize the performance properties of MBRs. The effects of membrane module height, number of aeration tubes and membrane spacing on liquid phase flow rates, gas holdup and shear stresses at the membrane surface have been investigated. It has been found that optimal MBRs experience the greatest shear forces on their surfaces at a distance of 250 mm from the aeration tube, around the 7 aeration tubes used to introduce gas and at the 40 mm spacings between the membrane sheets. Use of an aeration intensity of between 0.02 and 0.47 m3 min−1 generated shear stresses that were 50–85% higher than the original MBR for the same aeration intensity, thus affording optimal membrane performance that minimizes membrane fouling.
1 Introduction
Membrane bioreactor (MBR) technology has been used for the membrane filtration of activated sludge for wastewater treatment to produce high effluent quality with a small environmental footprint.1–3 However, problems associated with membrane fouling constitute a major drawback for the use of MBRs in waste water treatment plants. Aerated membrane tanks can be used for fouling control, however use of these membrane systems involves high energy consumption.4–6 The structure of MBR directly affects their flow characteristics, with ‘good gas–liquid’ interactions at the surface of the membrane reducing the probability that membrane fouling will occur. For example, Liu et al.7 incorporated a baffle into an MBR, which improved the fluid circulating flow rates at the membrane surface and reduced membrane fouling rates. The compact design of MBR membranes means that they are difficult to access to accurately obtain velocity distribution values within the reactor in real-time, which can make design/optimization studies difficult. Therefore, computational fluid dynamic (CFD) calculations provide an opportunity to quantify flow field distribution values in MBRs that cannot be detected experimentally.8–11 Consequently, numerical methods have been developed that enable flow rates in aerated membrane filtration processes to be predicted accurately.12–15 Yang et al.16 used CFD model for the cost-effective optimization of MBR and the model further revealed that the high nitrogen removal efficiency (>90%) was achieved due to the high recirculation ratio driven by airlift force without destroying the oxygen deprivation and enrichment in the anoxic and oxic zone, respectively. Ndinisa et al.17 used CFD to simulate gas–liquid two-phase flow in immersed flat membrane MBRs to predict gas phase velocities, liquid phase velocities and membrane surface shear stress values during aeration. Brannock et al.18,19 used CFD to investigated the effects of aeration and membrane configuration such as size and position of inlets, baffles, or membrane orientation on the overall membrane performance. However, MBR hollow fiber membrane model contains large numbers of membrane filaments that adopt a fluid–solid coupling state during filtration that increases the difficulty of producing accurate calculations. Wang et al.20 used an alternative porous medium in their MBR calculation model to develop a method based on experimental determination of the resistance coefficient of the fluid flowing through the hollow fiber membrane. This porous medium model was coupled with a 3D multiphase model to improve the accuracy of the calculations used. Zamani et al.21 developed a fluid–solid coupling model based on fluid and structural mechanic governing equations, which predicted that enhanced vibrational shear forces are effective for reducing membrane fouling. Wang et al.22 developed a model coupled the Navier–Stokes and Darcy Brinkman equations to simulate a complete filtration run. Radaei et al.23 developed a 3D CFD model to the study shear stress induced by spherical cap bubbles in hollow fibre membrane modules.
After several decades of development, Particle Image Velocimetry (PIV) is now recognized as a mature technology for 2D flow field measurement. For example, PIV is widely used throughout the chemical industry for the study of flow field distributions in stirred tank reactors,24 at gas-evolving electrodes,25 in bubble columns26 and flat sheet MBR.27 For example, Liu et al.28 have used PIV to investigate the hydrodynamic characteristics of two-phase flow fields at gas-evolving electrodes, whilst Leslie et al.29 used PIV to determine the flow states in an aerated bench scale MBR.
This study has used CFD to improve the hydrodynamics of MBR through modification of their structures, including an examination of the effects of changing the height of the membrane, the number of aeration tubes and membrane spacings. The mathematical models developed have been validated through cold testing experiments using PIV technology. Finally, the hydrodynamic characteristics of standard and optimized MBR have been measured under different gas flow rate to evaluate their comparative efficiencies.
2 Simulation procedures
Gas–liquid two-phase or gas–liquid–solid three-phase flow modelling approaches have been used to optimise the performance of various types of sewage treatment and chemical reactors.30 MBRs are often modelled as a three-phase mixing system, with water used as the continuous phase and air and sludge treated as a dispersed phase. Sludge distribution is relatively uniform because its density is close to that of water, so they can be combined into a continuous phase and treated as a two-phase system.
Two different approaches have been developed to simulate multiphase flow, based on Lagrangian or Eulerian models.31 The former method employs liquid as the continuous phase and bubbles considered as the discrete phase, which enables motion trajectories to be tracked in each discrete phase. The latter method considers the two phases to be a continuous phase that coexist at the same spatial point, thus enabling processes to be modelled using their respective momentum, mass, and energy transfer equations. The aeration tube air flotation design used in MBR systems generates a large number of bubbles, with large computational resources required to model the motion of each bubble. Consequently, the focus of this study concentrated on modelling the macroscopic flow of the continuous phase, rather than the motion trajectories of individual bubbles. Therefore, a Eulerian model incorporating a standard k–epsilon analysis has been used to describe the turbulent flow of the fluid in the MBR. The complex fluid flow in the MBR means that not all factors could be calculated, and so the following simplifications were incorporated into the numerical model used:
(1) The material properties in the MBR remained unchanged throughout the analysis, with the liquid phase considered to be incompressible and conforming to Newtonian principles.
(2) No bubble coalescence or break-up was considered to occur, with bubble diameters remaining constant over the course of the simulation process.
(3) No mass exchange between the gas and liquid phases was considered to occur.
Base on these assumptions, the volume fractions of the gas and liquid phases in the Euler–Euler model used were set to φl and φg, respectively. Continuity equations were developed independently for both phases, without the need to include an additional mass-transfer term.32
|  | (1) |
Compared with turbulent viscous stress, the effect of molecular viscous stress on the two phases was considered to be negligible with the momentum equation written as follows.33
|  | (3) |
where
u is velocity (m s
−1),
p is pressure (Pa),
ρ is density (kg m
−3),
g is the gravity vector (m s
−2),
Fb is the additional volume force (N m
−3),
Tkturb is the turbulent stress tensor (N m
−3).
The stress tensor used was described as follows:
|  | (4) |
where
μeff,k is the effective viscosity, and
I denote the identity tensor. For the liquid phase, the effective viscosity is the sum of the dynamic viscosity
μL,k and the turbulent viscosity
μT,k,
In this case, the turbulence properties, including the turbulent kinetic energy k (m2 s−2) and the dissipation rate of turbulent kinetic energy ε (m s−3) were solved using a multiphase k–ε model.
Additional volume forces that act on the bubbles are drag forces, lift forces and virtual mass forces, however, the magnitude of the lift and virtual mass forces are relatively small in comparison to the drag forces. Díaz et al.34 have shown that inclusion of virtual mass forces does not significantly effect calculations, whilst Sokolichin and Eigenberger32 have shown that inclusion of lift forces is not relevant without clear experimental evidence of their direction and magnitude. Therefore, only the effect of drag forces on the BMR model was considered in this study, with the drag force defined as,
|  | (6) |
where
db is the bubble diameter (m),
CD is the drag coefficient which was defined mathematically using semi-empirical methods. Evaluation of the drag coefficient required consideration of the bubble Reynolds number (Re
b), whose value is based on the slip velocity of a single bubble in the fluid. Extensive results on drag coefficient values have been reported in the literature, with the drag coefficient reported by Schiller and Naumann used as the basis for our calculations:
|  | (7) |
These equations were solved using the COMSOL Multiphysics software package using a time-dependent mode, with solutions calculated using a backward differential (BDF) method with an order of 2. The initial step size and the maximum solution step size were both set to 10−4 s, with each step of the solution having an absolute tolerance level of 10−5. Flow field and gas content distribution levels were found to stabilize after a calculation time of 100 s, with a “timeavg” function then used to average global results in the last 10 s.
3 Simulation validation
It was necessary to validate the accuracy of the mathematical models before using them to analyse real systems, with the models developed in this report validated using flow field data generated using PIV techniques.
3.1 Grid independence
The quality of the simulation results was confirmed using grid independence tests to determine the effect of the grid size. An unstructured tetrahedral grid was used in the model, with the grid size being reduced from 250 mm to 60 mm and the corresponding grid numbers increased from 3.6 × 103 to 1.8 × 104.
As shown in Fig. 1, the MBR numerical model reveals that different grid sizes produce large differences in fluid flow rates, with velocity distribution points tending to be stable for grid sizes between 20 and 25 mm. As a result, an unstructured tetrahedral grid size of 25 mm was selected for all subsequent simulations, which generated acceptable accuracy levels for manageable computational times.
 |
| Fig. 1 Effect of grid independence on simulation results for different mesh sizes. | |
3.2 PIV experiments
Numerical accuracy levels were verified using a cold model of MBR with aeration and liquid level heights of 9 L min−1 and 50 cm and 3 L min−1 and 46 cm, 15 L min−1, respectively. Liquid phase velocity distributions were compared for a range of x = 0–98 mm, y = 150 mm and z = 375 mm. The equipment and the corresponding model used for simulation experiments are shown in Fig. 2, with fluid motions measured using PIV techniques. The PIV measurement was conducted using a double pulsed Nd:YAG laser of 532 nm wavelength, and maximum energy of 100 mJ per pulse (Continuum Inc., USA). The laser beam was passed through an optical arrangement consisting of cylindrical and spherical lenses, which generated a laser sheet with a thickness of 1 mm. The images were acquired by a single frame-straddling digital CCD camera (PowerView Plus, TSI Inc., USA) at a resolution of 2048 × 2048 pixels, which was positioned perpendicular to the plane of the light sheet in the glass tank. Frame and laser sequencing were controlled by a Laser pulse 610035 synchronizer (TSI Inc., USA). For all images processing in TSI Insight 4G software, the interrogation area (IA) was 64 × 64 pixels, with 50% overlap. A range filter was implemented to the raw data to remove obvious spurious vectors. The flow characteristics of gas–liquid two-phase flow in this MBR study were instantaneous, with error curves determined for 500 PIV images (three times averaged) for each set of experimental conditions.
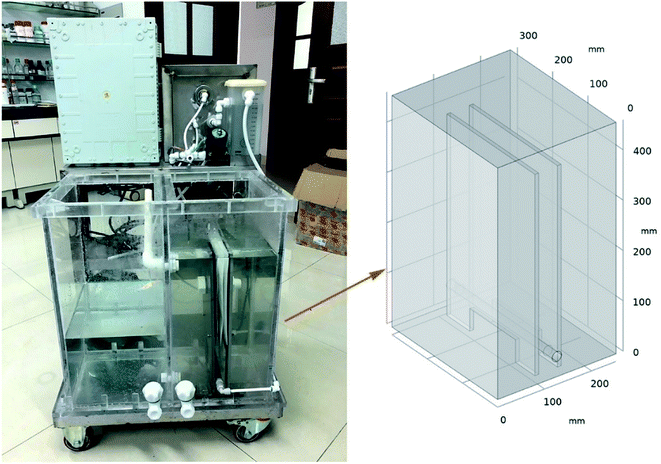 |
| Fig. 2 Schematic of the experimental setup that was modeled in this study. | |
The results of this modelling study are shown in Fig. 3, with the flow rates generated from experimental and calculated showing large gaps at two sides of the membrane wall (boundary condition set at u = 0 m s−1). The velocity distribution of the wall boundary could not be measured experimentally, however modelling predictions agreed well with previously determined experimental values, thus indicating that the numerical model has good reliability over the flow range tested.
 |
| Fig. 3 Comparison of time-averaged velocities for different lines (generated using PIV techniques) with the mathematical model for aeration and liquid level heights of (a) 9 L min−1, 50 cm, (b) 3 L min−1, 46 cm, and (c) 15 L min−1, 46 cm. | |
4 Results and discussion
4.1 Experimental apparatus
MBRs are usually prepared from the combination of membranes sheets which simplifies their industrial production, with this paper employing CFD studies to model fluid dynamics of this type of MBR fitted with a series of aeration tubes in a membrane tank filled with aqueous media (see Fig. 4). The geometrical dimensions of the computed model were established according to the structure of the MBRs that were available, whose detailed structural parameters are reported in Table 1.
 |
| Fig. 4 Schematic diagram of MBR and the streamline and velocity (color legend) of the liquid phase in the MBR. | |
Table 1 Structural parameters of the membrane bioreactor
Definition |
Parameters |
Number of membranes |
8 |
Number of aeration tubes |
7 |
Dimensions of MBR |
750 mm × 750 mm × 1890 mm |
Dimensions of membrane |
600 mm × 30 mm × 1015 mm |
Distance of membrane |
80 mm |
Distance of aeration tube |
80 mm |
Distance from membrane to bottom of tank |
450 mm |
Distance from membrane to liquid surface |
375 mm |
4.2 Hydrodynamic characteristics of the MBR
The densities and viscosities of the bubbles and water in the system were set at 1.22 kg m−3 and 1.75 × 10−5 kg m−1 s−1, and 998.06 kg m−3 and 1.00 × 10−3 kg m−1 s−1, respectively. The initial conditions used to generate the MBR model were: aeration volume of 1.7 m3 min−1, bubble size of 1 mm, membrane module height of 450 mm, 7 aeration tubes, with membrane spacings of 5 mm. The overall state of the liquid flowing throughout the MBR is shown in Fig. 4.
Fig. 5(a) shows the distribution of the mean liquid phase velocities and gas volumes fraction in the center section (y = 400) of the MBR reactor, which reveals a maximum flow rate of 0.74 m s−1 in the centers of the channels between the membranes. The fluid flows from the channel to the liquid surface from the left and right sides of the membrane plate, forming two left and right cycles at their cross-section. Similar velocity profiles are obtained by the experiments of Liu et al.35Fig. 5(b) shows a cloud plot of the average gas holdup distribution at the same position in the MBR reactor, with bubbles accumulating at the lower edge of the aeration tube with a gas content of up to 17%. The gas phase (with a gas content of around 5%) is evenly distributed between the membrane plates, with Fig. 5(c) and (d) showing the average velocity and average gas fraction distribution in the side section (x = 400) of the MBR. In addition to the aeration tubes, the gas holdup is mainly concentrated between the membrane plates, with gas holdup rates lower and unevenly distributed in other places. The distribution of gas content on both sides of the module is relatively low, which is not ideal for washing precipitated pollutants away from the surface of the membrane to prevent its blocking.
 |
| Fig. 5 Distribution of mean liquid phase velocities and gas volume fractions for y = 400 mm and x = 400 mm. (a) liquid phase velocities for y = 400 mm; (b) gas volume fractions for y = 400 mm; (c) liquid phase velocities for x = 400 mm; (d) gas volume fractions for x = 400 mm. | |
4.3 Effect of membrane module height on performance
Bubbles fare generated from the aeration tube and float up to the liquid surface, where they gradually disperse to form a feather-like shapes, with the membrane module structure having a significant effect on gas–liquid mixing efficiency and fluid circulation dynamics.36 Therefore, the height of the arrangement of the membrane modules is an important factor determining gas holdup and fluid flow, which led us to investigate the effect of varying the height of the membrane from 250 mm to 650 mm, for an aeration volume of 1.7 m3 min−1. The average velocity distributions determined for different membrane heights at the y = 400 mm plane are shown in Fig. 6, which shows that the liquid phase passes through the channel between the membrane plates, before flowing down from the outside of the membrane plate. The average flow rates after stabilization were found to be 0.299, 0.293, 0.287, 0.260 and 0.238 m s−1, respectively. As the distance increased, the overall liquid phase flow rates decreased, with the highest flow velocities between the membranes showing a downward trend of 0.795, 0.827, 0.739, 0.728 and 0.691 m s−1, respectively. As the distance from the membrane module to the aeration tube increases, more fluid flows from the periphery of the membrane plate, ultimately causing a decrease in flow rate between the membrane plates.
 |
| Fig. 6 Distribution of mean liquid phase velocities and gas volume fractions at y = 400 mm planes for different membrane module heights: (a) 250 mm; (b) 350 mm; (c) 450 mm; (d) 550 mm; (e) 650 mm. | |
Fig. 6 shows the average gas holdup distribution for different membrane heights at the y = 400 mm plane, with gas distribution in the membrane channels in the five structures found to be relatively uniform. However, the gas volume fraction gradually increased with an increase in the height of the membrane, meaning that more bubbles rise directly from the periphery of the membrane to the liquid surface. Fig. 7 shows the average liquid phase velocity distribution and the average gas-capacity distribution cloud map for the x–y plane at z = 1000 mm, with fluid flowing mainly through the central passage of the diaphragm, with a low velocity in the peripheral passage of the diaphragm. These modelling studies also revealed that the flow velocity distributions between each channel were uneven and that there was a velocity gradient in each channel, with the liquid flow rate between membrane plates decreasing as the distance between them increased. The gas phase was essentially the same for all five structural types which were evenly distributed between the membrane sheets.
 |
| Fig. 7 Distribution of mean liquid phase velocities and gas volume fractions at z = 1000 mm with different membrane module heights of: (a) 250 mm; (b) 350 mm; (c) 450 mm; (d) 550 mm; (e) 650 mm. | |
Fig. 8 shows the average, maximum and minimum values of shear stresses on the membrane surface, with average shear stresses after stabilization equal to 0.150, 0.141, 0.133, 0.128 and 0.119 Pa, respectively. Average shear stress values of the membrane assemblies were found to gradually decrease for an increasing distance from their aeration tube. This indicates that shorter distance between the membrane assembly and the aeration tube are favored in order to maximize erosion effects that minimize membrane fouling. Liu et al.15 found that the shear stress grew not significantly with the distance extended from 150 mm to 300 mm.
 |
| Fig. 8 Shear stress on membrane surfaces for different membrane module heights. | |
4.4 Effect of the number of aeration tubes on membrane performance
Results on the effect of the height of the membrane modules revealed that the flow field passes primarily through the most central membrane channel of the membrane module. This means that the number and placement position of aeration tubes in the MBR will have a significant effect on fluid flow. Liu et al.15 observed that the water velocity contour distributed in parallel arrangements (pipes under the membranes and between adjacent membranes) was much more even than that of perpendicular arrangement. Therefore, the model with the performance of MBRs containing aeration tube numbers of 1, 3 and 7 investigated for a gas flow rate of 1.7 m3 min−1.
Distributions of mean liquid phase velocities and gas volume fractions for different numbers of aeration tubes on the y = 400 mm plane are shown in Fig. 9. The average flow rates after stabilization were 0.155, 0.162 and 0.287 m s−1, respectively, which indicates that the overall flow rate of the liquid phase for multiple aeration tubes is increased. The highest flow velocities between the membranes showed a downward trend of 0.617, 0.451 and 0.738 m s−1, respectively. A reactor fitted with a single aeration tube results in gas being concentrated in the channel directly above the aeration tube, whilst use of three aeration tubes results in a more uniform flow velocity distribution between the channels. The average gas holdup of the three models investigated was 0.35%, 1.25%, and 2.66%, respectively, with the gas content in the MBR gradually increasing and becoming more uniformly distributed as the number of aeration tubes increased.
 |
| Fig. 9 Distribution of mean liquid phase velocities and gas volume fraction for different numbers of aerating tubes: (a) 1; (b) 3; (c) 7. | |
Fig. 10 shows the velocity profile at the MBR centerline (y = 375 mm and z = 1000 mm), with 7 aeration tubes affording a higher velocity in almost every channel, three aeration tubes giving the most uniform flow velocity distribution; and one aeration tube resulting in the greatest differences in flow velocities between channels.
 |
| Fig. 10 Mean liquid phase velocity distributions at y = 375 mm and z = 1000 mm for different numbers of aerating tubes. | |
The distribution of mean liquid phase velocities and gas volume fractions for different numbers of aerating tubes at z = 1000 mm is shown in Fig. 11, with their liquid phases flowing mainly from the center of the membrane plate, with low flow velocities in the peripheral passage of the membrane module. The flow velocity was higher in the middle of the channel, while a boundary layer with much lower velocity existed near the membrane surface. The non-uniformity of gas–liquid flow in channels between two membranes was also found by Zhang et al.11 Fluid flowed through the central member channel of the MBR fitted with only one aeration tube, thus indicating that flow velocity distribution between the channels was non-uniform and that significant velocity gradients were present. However, the gas content in the MBR fitted with seven aeration tubes was much higher are more uniformly distributed, indicating a more optimal design.
 |
| Fig. 11 Distribution of mean liquid phase velocities and gas volume fractions at z = 1000 mm for different numbers of aerator tubes: (a) 1; (b) 3; (c) 7. | |
Fig. 12 shows the average, maximum, and minimum shear stress values at the membrane surface for aeration tube numbers of 1, 3, and 7, respectively, with average values after stabilization increasing from 0.062 through 0.072 to 0.133 Pa, respectively.
 |
| Fig. 12 Shear stress at membrane surfaces for different numbers of aerating tubes. | |
4.5 Effect of membrane spacing
Membrane spacing is an important factor affecting fluid flow between membrane sheets, and so the effect of increasing membrane distances from 30 to 80 cm on the flow velocity distribution investigated. The distribution of mean liquid phase velocities and gas volume fractions for different membrane spacings at the y = 400 mm plane are shown in Fig. 13. In all configurations, the liquid phase passes through the middle of the membrane, before reaching the liquid level and flowing downwards. The average flow rates after stabilization were found to be 0.262, 0.276, 0.287, 0.257, 0.288 and 0.311 m s−1, respectively. The flow rates range is simular to the model in the study of Wu et al.10 As the distance from the membrane module to the aeration tube increased, then the overall flow rate of the liquid phase decreases, with gas distribution levels found to be uniform for all five models, resulting in gas content levels of 3.42%, 2.94%, 2.66%, 2.47%, 2.18%, and 1.91%, respectively. Increasing the spacing between the membranes resulted in gas holdup between the membranes gradually decreasing. When the membrane spacing was 30 cm, then the gas accumulates under the membrane because it cannot pass smoothly through the membrane channels. This results in the membrane channel being easily blocked, thus preventing further fluid flow through the channel.
 |
| Fig. 13 Distribution of mean liquid phase velocities and gas volume fractions for different membrane separation distances: (a) 30 cm, (b) 40 cm, (c) 50 cm, (d) 60 cm, (e) 70 cm, (f) 80 cm. | |
Fig. 14 shows the average shear stress, maximum shear stress and minimum shear stress of the membrane surface for different membrane spacings, with the average shear stress after stabilization found to be 0.038, 0.181, 0.133, 0.026, 0.085, and 0.087 Pa, respectively. The average shear stress on the membrane surface reached a maximum when the membrane spacing was set at 40 mm.
 |
| Fig. 14 Shear stresses of membrane surfaces for different separation distances. | |
4.6 Hydrodynamic characteristics of an optimized MBR
Increasing gas flow rates is the simplest way to increase aeration intensity levels, however increasing gas flow rates also result in increased energy consumption. A previous study on MBR structures revealed that a distance of 250 mm between the membrane and aeration tube was optimal, with our study showing that an aeration tube number of 7 and membrane spacing of 40 mm was optimal for inducing the highest shear stress. The effect of gas flow rates from 0.2, 0.7, 1.7, 2.7, 3.7 to 4.7 m3 min−1 on fluid flow in MBR were then investigated, with Fig. 15 showing shear stresses at membrane surfaces before and after optimization of gas flow rates. The shear stress of the membrane surface increased for increasing gas flow rates, with the shear stress at an optimized membrane surface being increased from 0.004 to 0.138 Pa for an aeration volume of 0.57 m3 min−1. Average flow velocities decreased rapidly for larger rates of aeration, indicating that the gas content in the membrane plate channel rises sharply, resulting in access of the liquid phase to the membrane surface being hindered.
 |
| Fig. 15 Comparison of the shear forces experienced by membrane systems for different gas flow rates. | |
When the gas flow rate was 1.7 m3 min−1, the membrane surface shear stress levels of the optimized membrane structure was increased from 0.133 to 0.209 Pa, which corresponds to an overall increase of 57%. When the aeration volume was increased to 4.7 m3 min−1, then the shear stress of the optimized structure was increased from 0.187 to 0.343 Pa, which corresponds to an 83% increase. In the case of Liu et al.,15 the shear stress increase 82% with the aeration intensity increase 150%. When the aeration intensity was from 0.02 to 4.7 m3 min−1, the optimized cell which can save 57–69% of energy consumption than of original cell. These results show that the shear force on the surface of membrane can be significantly improved by optimizing the membrane structure and aeration flow rates.
5 Conclusions
It is difficult to study structurally complicated MBR structures using real-time measurements and so this study has combined CFD numerical calculations and MBR cold model experimental models to carry out optimization studies on MBRs. These studies have revealed the following results:
(1) A two-phase gas–liquid flow model can be used to effectively simulate MBR performance, with cold model experiments that employ PIV techniques used to generate experimental data to validate the results produced by the computational model. Our two-phase simulation studies showed good agreement with experimental results, thus establishing the reliability of the numerical model for MBR optimization studies.
(2) The validated CFD simulation model was used to simulate the effects of varying membrane module height, number of aeration tubes and membrane spacing on liquid phase flow rates, gas holdups and shear stresses of the performance of the membrane surfaces within MBRs.
(3) An optimal design was identified based on an MBR containing 40 mm spacings between membranes and the use of 7 aerating tubes to introduce gas into the system. This design resulted in the largest shearing force being generated at the bottom of the membrane module at a distance of 250 mm from the aeration tube. Use of an aeration intensity of 0.02–0.47 m3 min−1 in an optimal MBR resulted in shear stress levels that were 50–85% greater than those generated in a standard MBR system, meaning that the optimal MBR is much less likely to become blocked under these operating conditions.
Conflicts of interest
There are no conflicts to declare.
Acknowledgements
We acknowledge the financial support provided by the National Natural Science Foundation of China (Grant 21206038).
References
- T. Melin, B. Jefferson, D. Bixio, C. Thoeye, W. De Wilde, J. De Koning, J. van der Graaf and T. Wintgens, Desalination, 2006, 187, 271–282 CrossRef CAS.
- M. Aslam, A. Charfi, G. Lesage, M. Heran and J. Kim, Chem. Eng. J., 2017, 307, 897–913 CrossRef CAS.
- A. Drews, J. Membr. Sci., 2010, 363, 1–28 CrossRef CAS.
- F. Meng, S.-R. Chae, A. Drews, M. Kraume, H. S. Shin and F. Yang, Water Res., 2009, 43, 1489–1512 CrossRef CAS.
- K. Kimura, N. Yamato, H. Yamamura and Y. Watanabe, Environ. Sci. Technol., 2005, 39, 6293–6299 CrossRef CAS.
- P. Le-Clech, V. Chen and T. A. G. Fane, J. Membr. Sci., 2006, 284, 17–53 CrossRef CAS.
- R. Liu, X. Huang, C. Wang, L. Chen and Y. Qian, Process Biochem., 2000, 36, 249–254 CrossRef CAS.
- Z. Trad, C. Vial, J. P. Fontaine and C. Larroche, Chem. Eng. J., 2015, 282, 77–90 CrossRef CAS.
- C.-L. Liu, Q.-W. Zhao, Z. Sun, G.-M. Lu and J.-G. Yu, Arabian J. Sci. Eng., 2018, 43, 5965–5976 CrossRef CAS.
- Q. Wu, X. Yan, K. Xiao, J. Guan, T. Li, P. Liang and X. Huang, Bioresour. Technol., 2018, 249, 402–409 CrossRef CAS.
- Z. Qing, X. Rongle, Z. Xiang and F. Yaobo, Water Sci. Technol., 2014, 69, 1846–1852 CrossRef CAS PubMed.
- W. Naessens, T. Maere and I. Nopens, Bioresour. Technol., 2012, 122, 95–106 CrossRef CAS.
- W. Naessens, T. Maere, N. Ratkovich, S. Vedantam and I. Nopens, Bioresour. Technol., 2012, 122, 107–118 CrossRef CAS.
- M. Yang, D. Yu, M. Liu, L. Zheng, X. Zheng, Y. Wei, F. Wang and Y. Fan, Bioresour. Technol., 2017, 227, 102–111 CrossRef CAS.
- M. Liu, M. Yang, M. Chen, D. Yu, J. Zheng, J. Chang, X. Wang, C. Ji and Y. Wei, Bioresour. Technol., 2018, 269, 300–308 CrossRef CAS.
- M. Yang, Y. Wei, X. Zheng, F. Wang, X. Yuan, J. Liu, N. Luo, R. Xu, D. Yu and Y. Fan, Bioresour. Technol., 2016, 219, 566–575 CrossRef CAS PubMed.
- N. V. Ndinisa, A. G. Fane, D. E. Wiley and D. F. Fletcher, Sep. Sci. Technol., 2006, 41, 1411–1445 CrossRef CAS.
- M. Brannock, Y. Wang and G. Leslie, Water Res., 2010, 44, 3181–3191 CrossRef CAS PubMed.
- M. Brannock, G. Leslie, Y. Wang and S. Buetehorn, Desalination, 2010, 250, 815–818 CrossRef CAS.
- Y. Wang, M. Brannock, S. Cox and G. Leslie, J. Membr. Sci., 2010, 363, 57–66 CrossRef CAS.
- F. Zamani, A. W. K. Law and A. G. Fane, J. Membr. Sci., 2013, 429, 304–312 CrossRef CAS.
- Z. Wang, K. Su, T. Shu and W. Wang, Water Sci. Technol., 2017, 76, 2503–2514 CrossRef CAS.
- E. Radaei, X. Liu, K. H. Tng, G. Merendino, F. J. Trujillo, P. R. Bérubé and G. Leslie, Water Res., 2019, 160, 60–69 CrossRef CAS.
- K. V. Sharp and R. J. Adrian, AIChE J., 2001, 47, 766–778 CrossRef CAS.
- C.-L. Liu, Z. Sun, G.-M. Lu and J.-G. Yu, R. Soc. Open Sci., 2018, 5, 171255 CrossRef.
- R. C. Chen, J. Reese and L.-S. Fan, AIChE J., 1994, 40, 1093–1104 CrossRef CAS.
- B. Wang, K. Zhang and R. W. Field, AIChE J., 2018, 64, 2721–2736 CrossRef CAS.
- C. Liu, Z. Sun, G. Lu, X. Song and J. Yu, Flow Meas. Instrum., 2015, 45, 415–420 CrossRef.
- X. Liu, Y. Wang, T. D. Waite and G. Leslie, J. Membr. Sci., 2016, 520, 201–213 CrossRef CAS.
- L. Li, G. A. Al-Muntasheri and F. Liang, Petroleum, 2016, 2, 313–323 CrossRef.
- C.-L. Liu, Z. Sun, G.-M. Lu, X.-F. Song and J.-G. Yu, Can. J. Chem. Eng., 2015, 93, 565–579 CrossRef CAS.
- A. Sokolichin, G. Eigenberger and A. Lapin, AIChE J., 2004, 50, 24–45 CrossRef CAS.
- D. A. Drew, Annu. Rev. Fluid. Mech., 1983, 15, 261–291 CrossRef.
- M. E. Díaz, A. Iranzo, D. Cuadra, R. Barbero, F. J. Montes and M. A. Galán, Chem. Eng. J., 2008, 139, 363–379 CrossRef.
- N. Liu, Q. Zhang, G. L. Chin, E. H. Ong, J. Lou, C. W. Kang, W. Liu and E. Jordan, J. Membr. Sci., 2010, 353, 122–134 CrossRef CAS.
- R. Sengur, G. Deveci, R. Kaya, T. Turken, S. Guclu, D. Y. Imer and I. Koyuncu, Desalin. Water Treat., 2015, 55, 1747–1761 CrossRef CAS.
|
This journal is © The Royal Society of Chemistry 2019 |