DOI:
10.1039/C9RA06267J
(Paper)
RSC Adv., 2019,
9, 39025-39036
High-throughput untargeted metabolomics and chemometrics reveals pharmacological action and molecular mechanism of chuanxiong by ultra performance liquid chromatography combined with quadrupole-time-of-flight-mass spectrometry†
Received
12th August 2019
, Accepted 17th November 2019
First published on 28th November 2019
Abstract
Metabolomics methods can be used to explore the effect mechanisms underlying treatments with traditional medicine. Lung cancer (LC) causes the highest morbidity and mortality among tumors disease, and has become a serious public health problem. Chuanxiong (CX) is a dried rhizome of Ligusticum Chuanxiong Hort., often used in traditional Chinese medicine and has been widely used in the treatment for tumors. However, the pharmacological effect of CX on the metabolism process of LC mice is still unclear. This study used high-throughput untargeted metabolomics aims to discover biomarkers and metabolic pathways of LC as a potential target to provide insight into the pharmacological action and effective mechanism of CX against LC. The precise structural identification of the LC biomarker has been established using ultra performance liquid chromatography (UPLC) combined with quadrupole-time-of-flight-mass spectrometry (Q-TOF-MS) technology. UPLC-Q-TOF-MS and chemometrics methods were used to analyze the blood metabolism of LC model mice, and revealed the intervention effect of CX on LC model mice and potential therapeutic targets. The results showed that the metabolic profile clustering among the groups was obvious, and 31 potential biomarkers were finally locked, involving 7 related metabolic pathways. After treatment with CX, we found that 22 kinds of biomarkers were recalled to the main metabolic pathway which are associated with lipid metabolism. This study provides an effective biomarker reference for early clinical diagnosis of LC, and also provides a foundation for the expansion of new drugs for CX treatment of LC.
1. Introduction
Cancer has become the chief culprit in threatening human health. Primary bronchial lung cancer, referred to as lung cancer (LC), is a malignant tumor with unlimited proliferation of epidermal cells in the alveolar wall, and bronchial or bronchiolar mucosa. It is composed of 80–85% non-small cell lung cancer and a small fraction of small cell LC.1,2 The incidence of LC is high all over the world. It is the form of cancer with the highest morbidity and mortality. The number of LC deaths far exceeds the number of deaths from other major cancers, it has become a serious public health problem.3 Surgical treatment for early LC has a good prognosis, but a large proportion of LC patients have reached the end of diagnosis, where there are few cases that can be operated on.4 Generally, radiotherapy and chemotherapy are the main methods. However, if the symptoms are not cured, the patient's remission period and survival time are not prolonged, accompanied by large side effects.5 Therefore, for the postoperative recovery of ill patients with LC, 80% of them died within one year after diagnosis, and the 5 year survival rate is only 5% to 10%, and the curative effect is unsatisfactory.6,7 At present, imaging methods, sputum cytology and bronchoscopy are frequently used in the diagnosis of LC. These methods easily lead to missed diagnosis and misdiagnosis and require expensive instruments. They are not suitable for early screening of LC. With the high-speed upgrowth of biomarkers, the recognition of tumor markers has become a research hotspot in early diagnosis of malignant tumors.8 Tumor markers refer to a group of substances produced by the biosynthesis, release of tumor cells or the interaction between tumor and host in the process of tumorigenesis and proliferation, which ultimately lead to changes in metabolites in vivo. Therefore, in recent years, emphasis has been placed on early detection and early treatment.9 In the middle and late stage, multi-disciplinary and multi-method comprehensive treatment is advocated to improve the efficacy.10 Therefore, it is an important way to explore and study the method of combining TCM with chemistry medicine in the treatment of LC. In recent years, clinical treatment of LC has tended to combine differentiation of symptoms with differentiation of diseases, strengthening the body and eliminating pathogens. Combined with surgery, it can reduce the side effects of radiotherapy and chemotherapy and improve clinical efficacy, as well as the application of modern scientific means from clinical detection and animal reality.11 Some progress has been made in the study of therapeutic mechanism, which explained TCM shows obvious advantages in the prevention and treatment of LC.12
Because the early symptoms of LC patients are not obvious, and there are no specific and sensitive tumor markers in the current diagnosis.13 Although the combined detection of NSE, CEA, CYFRA21-1 and other tumor markers commonly used in clinic has certain sensitivity and accuracy, their specificity is not high and the positive rate is relatively low, so it is difficult to achieve the purpose of early accurate diagnosis.14,15 Therefore, how to early diagnose LC has become an important problem.16,17 Metabolomics is a technology to study the metabolic pathway of biological system by investigating the changes of metabolites of biological system after being stimulated or disturbed.18 It is another important research content of system biology after genomics, transcriptome and proteomics.19 Metabolomics is characterized by integrity and comprehensiveness, it explains life phenomena that can't be explained by one or more metabolites through the information provided.20,21 Therefore, its clinical application is being paid more and more attention.22 At present, many scholars have successfully used metabolomics methods to find tumor markers of breast cancer, prostate cancer and other malignant tumors.23,24 Therefore, finding accurate and effective LC biomarkers for early diagnosis is essential.
In the treatment of LC, comprehensive treatment has become the mainstream. According to the patient's body condition, the pathological type, the scope of invasion and the development trend of the tumor, the existing treatment methods should be applied in a planned and reasonable way in order to maximize the survival time and improve the quality of life of patients.25 Chinese medicine has become an important part of LC treatment.26,27 Many aspects of TCM, such as stabilizing tumor focus, prolonging survival time with tumor, increasing immune function, improving clinical symptoms, improving quality of life, and alleviating toxic and side effects of radiotherapy and chemotherapy, have shown certain efficacy and advantages.28,29 Because of its advantages, the local remission rate alone can't fully reflect the efficacy characteristics of traditional Chinese medicine.30
Ligusticum Chuanxiong Hort. (CX) is a dried rhizome of Ligusticum Chuanxiong Hort. often used in traditional Chinese medicine.31 It contains volatile oil (mainly phthalide and its dimer), alkaloids, organic acids and polysaccharides.32,33 CX and its chemical parts and monomers have many pharmacological effects on cardiovascular and cerebrovascular system, nervous system, respiratory system and other systems, such as improving vascular endothelial function and coronary artery blood flow, reducing blood flow resistance and blood pressure, anti-oxygen free radical, anti-inflammatory, anti-cancer, anti-platelet aggregation, anti-thrombosis, protecting nerve and so on.34 Blood stasis syndrome is a syndrome element objectively existing in most malignant tumor patients. Therefore, CX and other blood-activating drugs have been widely used in clinical tumor treatment.35 In recent years, basic research shows that CX, in addition to promoting blood circulation and relieving pain, its active ingredient ligustrazine, can play a variety of anti-tumor effects in inhibiting tumor proliferation, invasion, drug resistance and regulating tumor immunity, and should also be widely used in the treatment of LC.36 However, the effect of CX on metabolism spectrum of LC model mice is still unclear.
This work is based on the high-throughput metabolomics research platform and C57BL mice are to be inoculated with Lewis lung cancer cells to establish a model. By comparing the metabolic spectrum changes between model rats and control rats, the serum biomarkers related to Lew is LC model mice are found to provide effective markers for the early diagnosis of LC, and the therapeutic effect of CX on LC and the changes in blood metabolic spectrum are evaluated one step to provide basis for the research and development of anti-LC drugs.
2. Experiments
2.1. Drugs and reagents
Protamine Sulfate Injection (PTM), produced by Shanghai Biochemistry Pharmaceutical Factory, with a specification of 50 mg/5 mL/branch, batch number: 000609; sodium chloride injection was purchased from Sanlian Pharmaceutical Co., Ltd. (Harbin, China); sodium hydroxymethyl cellulose was purchased from Jinan Ou Mi Technology Co., Ltd. (Jinan, China); 95% ethanol was purchased from Nanjing Chemical Reagents Co., Ltd. (Nanjing, China); acetonitrile, methanol and acetone were purchased from Merck Company (chromatographic grade, Merck Company of Germany). Ligusticum wallichii aqueous solution (laboratory configuration) and other reagents were chromatographic grade.
2.2. Model preparation and grouping administration
C57BL tumor-bearing mice inoculated with Lewis LC for 10–14 day (purchased from Shanghai Institute of Medicine, Chinese Academy of Sciences, Shanghai Dong He Zheng Zi no. 153) were sacrificed after cervical vertebra removal, tumor tissues were taken under aseptic conditions, and uniform slurry was prepared according to tumor mass (g) and normal saline (mL) 1
:
3. Another 50 C57BL mice (18–20 g, purchased from the Institute of Animal Reproduction, Chinese Academy of Medical Sciences, license S1XK11-00-0006), male and female, divided into 5 groups according to the principle of random allocation, low dose group aqueous solution of CX 100 mg (kg d)−1 (CXLD) and high dose group aqueous solution of CX 200 mg (kg d)−1 (CXHD). Protamine 60 mg (kg d)−1 group (PTM); normal saline group (control group) and model group, all animals were raised in standard environment (temperature: 18–25 °C, humidity: 45–60%, illumination/darkness alternated every 12 hours). During the whole experiment, mice were allowed to eat freely and take water. Except the control group, mice in each group were inoculated with homogenate subcutaneously in the right axilla, each 0.2 mL. The drug administration was started on the 2nd day after inoculation in each group. Each dose group of CX aqueous solution was given corresponding drugs respectively. The normal saline group was given the same amount of normal saline, 0.2 mL each for 21 days. The protamine group was given once at 60 mg kg−1 on the 2nd day after inoculation and once every 12 h from the 3rd day for 20 day. Blood samples were collected from abdominal aorta on the 22 day. The experimental procedures were approved by the Animal Care and Ethics Committee at Harbin Medical University and all experiments were performed in accordance to the declaration of Helsinki.
2.3. Preparation of drug delivery solution
CX was purchased from Beijing Tong Ren Tang Harbin branch (Harbin, China) and identified as the dried rhizome of Ligusticum Chuanxiong Hort. Drying the medicinal materials, pulverizing, weighing 2 kg, performing hot reflux extraction twice with 8 times of water for 2 h each time, filtering, performing hot reflux extraction for 2 h with 95% ethanol on the residue, cooling naturally, pouring out the ethanol aqueous solution, filtering the residue, mixing with the water extract, vacuum concentrating under reduced pressure, and drying to obtain the prepared sample. Add 0.5% CMC–Na to prepare gastric lavage agent with corresponding concentration (calculated according to the amount of Ligusticum Chuanxiong), and store at 4 °C for usage.
2.4. Collection and preparation of blood samples
Collection. 24 hours after the last administration, all mice were anesthetized by intraperitoneal injection of 1% chloral hydrate solution and injected at a dose of 1 mL/100 g, collecting blood from the abdominal aorta, left to stand for 30 min, centrifuged at 4000 rpm, 4 °C for 15 min, supernatant was taken and split into 5 parts, 1 mL for each part, and frozen in a refrigerator at −80 °C until analysis.
Preparation. Unfreeze the serum sample in an ice bath, take 200 μL of serum sample, add 800 μL of acetonitrile to precipitate protein, vortex for 30 s, 4 °C, 1300 rpm for 15 min, remove supernatant, nitrogen drying, add 200 μL of methanol: water (9
:
1, v/v) mixed solution to the residue, vortex for 60 s, centrifuge at 4 °C, 13
000 rpm for 10 min, take supernatant through a 0.22 μm filter membrane, and take 5 μL of filtrate for analysis.
2.5. Liquid chromatography and mass spectrometric conditions
The UPLC-MS/MS analysis was carried out using a Waters ACQUITY™ ultra-performance liquid chromatography system (Waters Corp., Milford, USA) coupled with a Waters Synapt™ Q-TOF Mass System (Waters Corporation) equipped with an ESI ion source and hybrid Q-TOF mass spectrometer in both ESI− and ESI+ ion mode. Chromatographic column: ACQUITY UPLC™ BEH C18 (2.1 mm × 100 mm, 1.7 um, Waters Company), mobile phase: A phase is an aqueous solution containing 0.1% formic acid, B phase is acetonitrile containing 0.1% formic acid; gradient elution of A and B, 0–1 min, 1% B; 1–8 min, 1–30% B; 8–10 min, 30–99% B; 10–11 min, 99% B; 11–12 min, 99–1% B; 12–13 min, 1% B. Flow rate: 0.4 mL min−1; column temperature: 40 °C; sample room temperature: 4 °C; sample volume: 4 L.
Using electrospray ion source (ESI): detection mode: simultaneous detection of positive and negative ions; scanning mode: metabolite spectrum analysis using full scan (Full Scan), scanning range: 100–1000 amu; metabolite identification using mass spectrometry/mass spectrometry (MS/MS) mode; temperature from the sub source: 120 degrees; capillary voltage: 3 kV (ESI+), 2.8 kV (ESI−); tapered voltage: 25 V (ESI+), 35 V (ESI−); desolvent gas and cone pore gas: nitrogen; desolvent temperature: 400 °C; desolvent gas velocity: 450 L h−1; cone pore gas velocity: 30 L h−1. Collision gas: argon; collision energy: 5–25 eV.
2.6. Multivariate data processing
The metabolic profiles containing three-dimensional information were obtained by using the established blood sample analysis method. The data were processed by Waters Masslynx V4.1 (Waters, USA), and then imported into EZinfo 3.0.3 (Waters, USA) software for principal component analysis (PCA) to generate score vector map (plot) and 3D-plot is used to find potential biomarkers. The farther the distance from the load plot is, the greater the contribution of ions to the change of metabolic profiles is. To further explore the differential metabolites between the two groups, the two groups of data were imported to Orthogonal Partial Least Square-Discriminate Analysis (OPLS-DA) obtained VIP plot, S-plot. Combining with student T-test analysis and VIP > 1 and P < 0.05 were selected as screening conditions and potential biomarkers. By searching for possible structures in HMDB and MTELIN databases, the metabolites of statistical significance were retrieved and compared, and the differential metabolites were determined. Then the metabolic pathways and biological significance of biomarkers were analyzed through such databases as KEGG, MetPA and large databases.
3. Results
3.1. Metabolite spectrum analysis
All mice blood was pretreated according to the serum sample processing method, the serum samples were fully scanned and analyzed under the UPLC-Q-TOF-MS/MS conditions, and the data were collected and analyzed by Masslynx V4.1 software (Waters, United States). The metabolic profile BPI diagram (Fig. S1A and B†) of each group was obtained. Then all the .raw files collected by Masslynx V4.1 were guided into the Progenesis QI software. After peak matching, extraction and standardization, the ions were normalized to obtain a total of 8981 ions (ESI+) and 3763 ions (ESI−), which were then imported into Ezinfo3.0.3 software. Unsupervised principal component analysis (PCA) was carried out on all ions, and score plot (Fig. 1A and B) and loading plot (Fig. 1C and D) were obtained, intra-group clustering between model groups and control groups can be observed, separation between groups. This shows that the endogenous metabolic network of the mice serum has changed obviously after the model was established, and the contribution rate of ions farther from the origin point to the separation between groups in the loading diagram is greater. From the blood metabolomics level, the mouse model of LC was successfully replicated, and the endogenous metabolites of the model group have undergone significant changes.
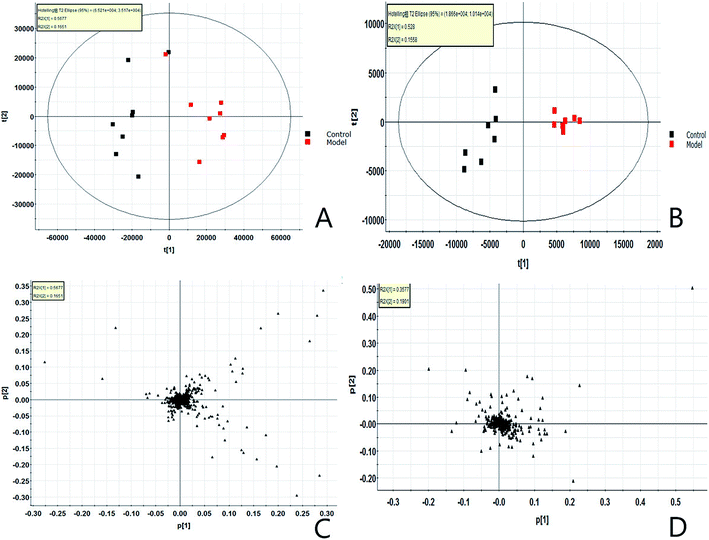 |
| Fig. 1 Score plot and loading plot of serum profile of control group and model group PCA analysis. (A) Score plot in positive ion mode. (B) Score plot in negative ion mode. (C) Loading plotin positive ion mode. (D) Loading plot in negative ion mode. | |
3.2. Identification of potential metabolic biomarkers
In order to find endogenous metabolic products that take an obvious part in the clustering and separation of metabolic groups, the serum samples is analyzed by supervised OPLS-DA to obtain OPLS-DA score plot (Fig. 2A and B) and 3D score plot (Fig. 2C and D), there is significant separation between the two groups in the plot. Further, the S-plot plot (Fig. 3C and D) is obtained. Further, ions with VIP value greater than 1 are selected in VIP scatter plot (Fig. 3A and B), combined with the T-test test results between the groups, finally, ions with VIP > 1 and P < 0.05 are selected for the next step of screening. Elemental composition analysis based on Rt_m/z of selected ions, then through the online network database (HMDB, Metaboanalyst) search, the possible name and chemical structure of the ion product were preliminarily estimated; and the massfragment software attached to the Masslynx V4.1 system was used to prove its effectiveness. This experiment initially identified 31 key metabolites, as shown in ESI Table 1.† The 31 compounds were matched with the MS/MS fragmentation information, and the possible chemical composition and structure were determined. At the same time, TBtools software was used to analyze the content of these potential biomarkers in each other to obtain a heat map (Fig. 4), which more intuitively reflects the change in its content. From the figure we can see that compared with the control group, increased expression of 24 biomarkers in the model group, and 7 decreased. 5 of them had significant differences (P < 0.05), and 26 had extremely significant differences (P < 0.01).
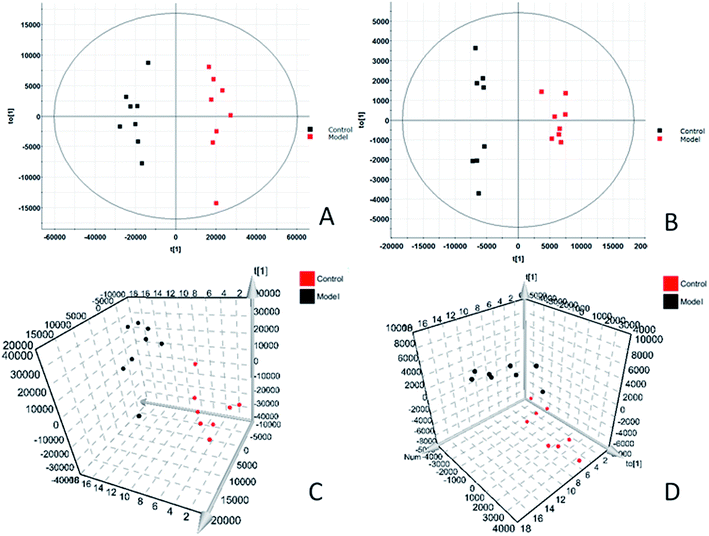 |
| Fig. 2 Score plot and 3D-score plot of serum profile of control group and model group OPLS-DA analysis. (A) OPLS-DA score plot in positive ion mode. (B) OPLS-DA score plot in negative ion mode. (C) 3D-score plot in positive ion mode. (D) 3D-score plot in negative ion mode. | |
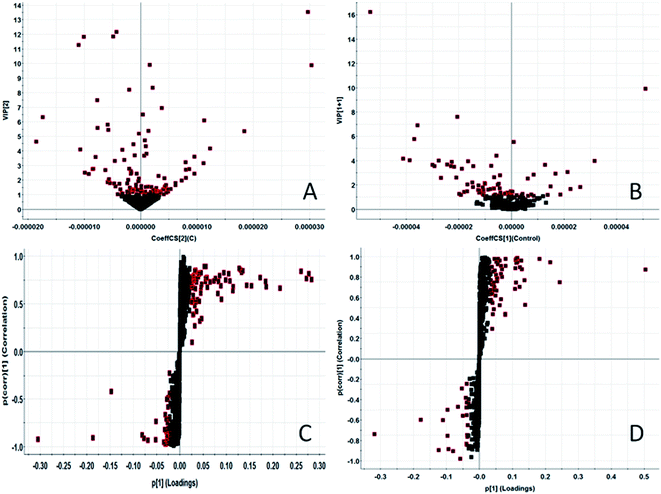 |
| Fig. 3 Metabolomics profiling of lung cancer. (A) VIP-plot serum profile of control group and model group scanned by OPLS-DA analysis in positive ion mode. (B) VIP-plot serum profile of control group and model group scanned by OPLS-DA analysis in negative ion mode. (C) S-plot of serum profile of control group and model group scanned by OPLS-DA analysis in positive ion mode. (D) S-plot of serum profile of control group and model group scanned by OPLS-DA analysis in negative ion mode. | |
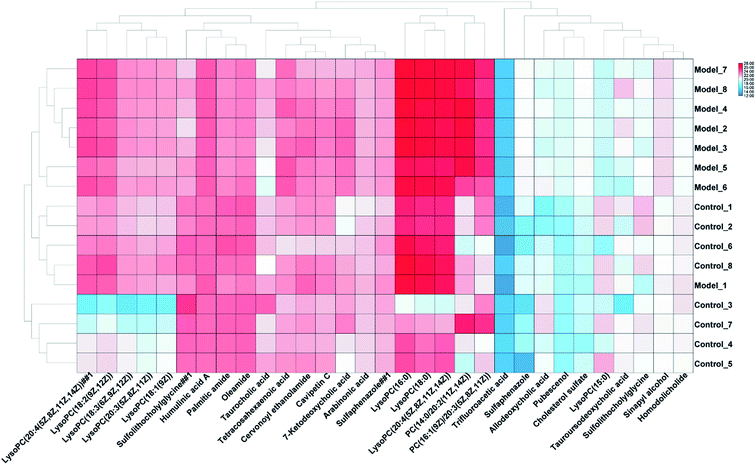 |
| Fig. 4 Changes in potential biomarker content between the control and model groups. | |
3.3. Biological pathway and function analysis
According to the identified 31 serum biomarkers, MetPA (Metabolomics Pathway Analysis) and online database such as KEGG were used to carry out enrichment analysis of related metabolic pathways to find the affected blood metabolic pathways in Lewis LC model mice. MetPA is a specialized network tool for topological analysis of metabolic pathways of potential metabolic biomarkers to help identify disease-associated metabolic pathways and finalized 7 related metabolic pathways (Fig. 5), including glycerophospholipid metabolism; linoleic acid metabolism; taurine and hypotaurine metabolism; alpha-linolenic acid metabolism; sphingolipid metabolism; arachidonic acid metabolism; primary bile acid biosynthesis; steroid hormone biosynthesis. This study shows that these blood endogenous metabolites produce strong disturbance in the whole metabolic track of LC model mice and are closely related to the metabolic mechanism of LC diseases. Among them, the most relevant are glycerophospholipid metabolism and primary bile acid biosynthesis. The network diagram of key potential markers and related metabolic pathways in serum metabolism of LC mice is shown in Fig. 6.
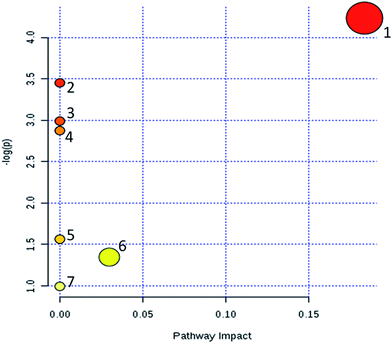 |
| Fig. 5 Metabolic pathway map related to potential biomarkers in mice serum generated by MetaboAnalyst. (1) Glycerophospholipid metabolism. (2) Linoleic acid metabolism. (3) Taurine and hypotaurine metabolism. (4) Alpha-linolenic acid metabolism. (5) Arachidonic acid metabolism. (6) Primary bile acid biosynthesis. (7) Steroid hormone biosynthesis. | |
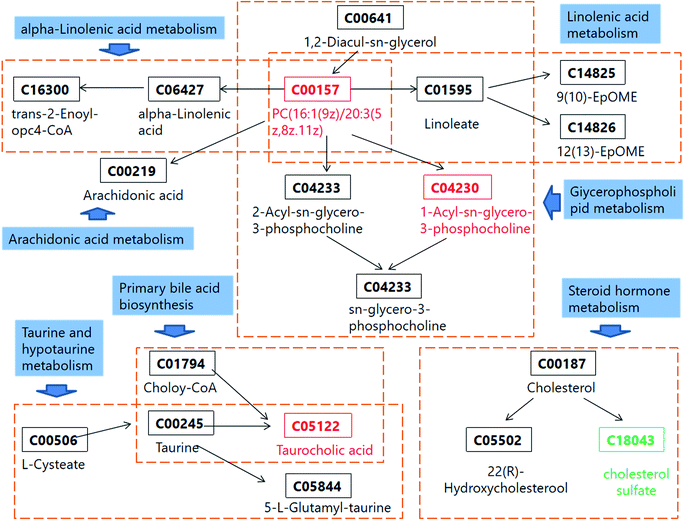 |
| Fig. 6 Correlation networks of the key biomarkers that are abnormally expressed in lung cancer mice on KEGG. In the path shown in the networks, the KEGG identification of each biomarker is displayed. A red box around the KEGG marker indicates that the marker is up-regulated in the model group, a green box indicates that the marker is down-regulated, and a black box indicates that the biomarker is not found in our experiment. The blue background box represents the corresponding metabolic pathway. | |
3.4. Effect of CX on blood metabolic profile of rats
According to the blood sample preparation method, the blood samples of the control group, the model group, the CXHD and CXLD, and the PTM group were separately processed, and the full scan analysis was performed using the UPLC-Q-TOF-MS platform. The PCA analysis was carried out by using the data of each group of QI software and EZinfo3.0 software, and the scores plot which can reflect the trend of changes between groups was obtained (Fig. 7). In the figure, it can be observed that the separation between the groups is obvious, and the control group is significantly separated from the LC model group, indicating that the serum metabolism of the mice has changed significantly after the model replication; CXHD, CXLD and PTC were clustered. The class is obvious, and the vector position is far away from the model group and approaches the control group, showing that using CX to prevent and treat LC model mice, it has a certain retardation effect on the pathological process of model mice. The metabolic expression of CXHD and CXLD was similar to that of the PTM group, further illustrating the effectiveness of CX in the treatment of LC model mice.
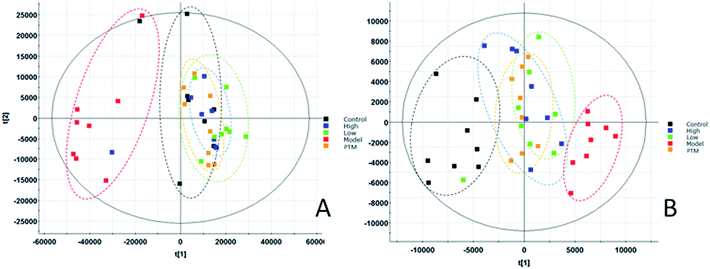 |
| Fig. 7 Metabolomics profiling of control group, model group, CX high dose group, CX low dose group and protamine group. Intra-group clustering and separation between groups, and after drug treatment, stay away from the model group and approach the control group. | |
3.5. Effect of CX on biomarkers in model mice
The change trend of potential biomarkers in Lewis LC model was analyzed after different doses of CX solution and PTM administration. It was found that CX solution intervention could affect potential biomarkers in Lewis LC model and make its content callback towards the control group. This can be seen in the relative changes of potential biomarkers (Fig. S2†), among the 31 potential biomarkers identified, PTM could recall 26 and 21 had statistical differences. CXHD intervention can callback 28, 22 have statistical difference; CXLD intervention can recall 25 cases, and 18 cases have statistical difference. There were 22 common markers that could be recalled at both doses, and 15 of them had significant difference. LysoPC (16:0); PC (14:0/20:2(11Z,14Z)); lysoPC (18:0); lysoPC (20:4(5Z,8Z,11Z,14Z)); PC (16:1(9Z)/20:3(5Z,8Z,11Z)); lysoPC (20:4(5Z,8Z,11Z,14Z)); lysoPC (18:2(9Z,12Z)); tetracosahexaenoic acid; palmitic amide; oleamide; cervonoyl ethanolamide; lysoPC (20:3(5Z,8Z,11Z)); lysoPC (18:3(6Z,9Z,12Z)); lysoPC (18:1(9Z)); trifluoroacetic acid (see ESI Table 2† for details). Studies on the metabolic pathway of potential biomarkers recalled by CX showed that its main regulatory metabolic pathway is glycerophospholipid metabolism; linoleic acid metabolism; alpha-linolenic acid metabolism; arachidonic acid metabolism, etc., and it is suggested that CX may achieve the effect of treating LC by regulating the metabolic disorder of the metabolic pathway and making it tend to be normal.
4. Discussion
In this study, C57BL mice were inoculated with Lewis LC cells to replicate LC model. Based on the analysis method of high-throughput metabolomics, using UPLC-Q-TOF-MS/MS analysis platform and multivariate data analysis method, 31 potential biomarkers and 7 related metabolic pathways of LC were found from the level of mice blood metabolomics. CXHD and CXLD can significantly recall 15 biomarkers at the same time, thus affecting glycerophospholipid metabolism; linoleic acid metabolism; alpha-linolenic acid metabolism; arachidonic acid metabolism and other metabolic pathways prevent LC model mice, thus achieving the purpose of treatment. This study provides the basis for the early diagnosis of LC and the development of new drugs.
Metabolomics method has demonstrated potential in some fields such as cancer, diabetes, toxicology, nutrition, genetic manipulation, environment, disease diagnosis and drug discovery.37,38 In this study, these metabolic pathways are all within the scope of lipid metabolism. The diversity of lipid structure endows it with a variety of important biological functions. Lipid participates in the regulation of a variety of life activities. It is not only an important component of cells and internal organelle membranes, but also have a vital function in the regulation of cell homeostasis in energy conversion, material transportation, information recognition and signal transmission, cell development and differentiation, and cell apoptosis.39,40 More and more basic research, clinical and intervention research, epidemiological investigation and so on show that abnormal lipid metabolism may be an important manifestation in the development of cancer.41,42 Lipids can be divided into two categories according to their structure and function: fats (triacylglycerol and triglyceride) and lipids (phospholipids, glycolipids, cholesterol and cholesterol lipids), linoleic acid; alpha-linolenic acid; arachidonic acid belongs to polyunsaturated fatty acids.43 Fatty acids can synthesize different kinds of complex lipids, glycerol esters can be synthesized in two ways. One is esterification, in which fatty acids are esterified with glycerol by monoacylglycerol acyltransferase (MGAT) and diacylglycerol acyltransferase (DGAT). Another route is the de novo synthesis of triglycerides, also known as the kennedy synthesis route.44 On the other hand, the synthesized TAG can be hydrolyzed into diacylglycerol (DAG), monoacylglycerol (MAG) and glycerol skeleton in reverse order by lipolytic enzyme, ATGL, HSL and MAGL, and free fatty acid can be released at the same time.45,46
Glycerol phospholipid is the most abundant lipid and the main component of biofilm. Glycerol phospholipids contain at least one acyl, alkyl or alkenyl linked fatty acyl side chain, and are divided into PA, PG, PI, PC, PE, PS and other main categories according to different polar heads, and lysophospholipid (lyso-PL) generated after hydrolysis of one fatty acid side chain.47 The metabolism of different phospholipids and their mutual transformation form complex network, which is regulated by a variety of metabolic enzymes. PC and PE are phospholipids with the largest abundance. The key enzymes for their synthesis mainly include genes such as PCYT1a, PCYT2, CHPT1 or CEPT1, EPT1 or CEPT1, and PE can generate PC through PEMT pathway, which becomes another important way for PC source. Abnormal metabolism of phosphatidylcholine (PC) is another marker of cancer cells.48 In ovarian cancer, endometrial cancer and other female tumors, PC changes are especially obvious, suggesting that PC-related metabolic enzymes may act through estrogen and its receptors or be associated with them. Another important way to generate PC is to convert PE into PC through PEMT enzyme. PEMT expression is significantly up-regulated in patients with NSCLC and is positively correlated with short survival time and adverse prognosis.49 Therefore, activated PC metabolic pathway may be necessary for tumor growth and development. However, the expression and activity of PEMT gene are significantly down-regulated in liver cancer, so the metabolic changes of phospholipids in different types of tumors may also be different. The increase in lipid production is mainly to synthesize more cell membrane lipids to meet the rapid proliferation of cancer cells and the soaring demand for energy. FAS, ACC, ACLY, the key genes of lipid production, and SREBP1, the key transcription regulatory factor that regulates their expression and activity, can be used as targets for inhibiting tumor neogenesis, and the growth of tumor cells can be effectively reduced through corresponding gene knock-down or chemical inhibitors.
Mass spectrometry can provide insight into disease pathogenesis,50–53 disease diagnosis,54–69 treatment prognosis,70–81 and monitoring testing.82–85 Mass spectrometry based chinmedomics could be used to discover lipid biomarkers86–88 and exploring the effects and mechanism of chinese herb medicine.89–96 In this study, another key lipid metabolism enzyme closely related to cancer is SCD1, a desaturase that generates MUFA from SFA. Suppressing SCD1 in LC cells can significantly inhibit the proliferation, survival and invasion of cancer cells in vitro and the tumorigenicity in vivo, and this inhibitory ability may hinder cells from passing through G1/S cell cycle and induce programmed cell death through the reduction of MUFA, and have a vital function in the initiation of LC cells. In this study, lysoPC (16:0); PC (16:1(9Z)/20:3(5Z,8Z,11Z)); PC (14:0/20:2(11Z,14Z)) and other phospholipids showed significant increases, indicating that the occurrence and development of LC have an important relationship with abnormal lipid metabolism. However, after CX treatment, the content of metabolic expression of several lipids was significantly decreased, indicating that lipid metabolism may be a potential metabolic pathway for CX treatment LC, lysoPC (16:0); PC (16:1(9Z)/20:3(5Z,8Z,11Z)); PC (14:0/20:2(11Z,14Z)) may be a potential data target, providing a basis for a new method of LC treatment.
5. Conclusion
In this study, we used high-throughput metabolomics method to discover biomarkers and metabolic pathways of LC and insight into the pharmacological action and action mechanism of CX against LC. A total of 31 potential biomarkers were finally discovered, involving 7 related metabolic pathways. After treatment with CX, and we found that 22 biomarkers were regulated and mainly associated with lipid metabolism. This study provides foundation for the exploring drugs for CX treatment of LC.
Conflicts of interest
There are no conflicts to declare.
References
- C. F. Mountain, Revisions in the international system for staging lung cancer, Chest, 1997, 111(6), 1710–1717 CrossRef CAS PubMed.
- P. C. Hoffman, A. M. Mauer and E. E. Vokes, Lung cancer, Lancet, 2000, 355(9202), 479–485 CrossRef CAS.
- C. A. P. Iii, R. T. Burnett, M. J. Thun, E. E. Calle, D. Krewski and K. Ito, et al., Lung cancer,cardiopulmonary mortality, and long-term exposure to fine particulate air pollution, JAMA, 2002, 287(9), 1132–1141 CrossRef PubMed.
- J. Rodriguezcanales, E. Parracuentas and I. I. Wistuba, Diagnosis and molecular classification of lung cancer, Cancer Treat. Res., 2016, 170, 25–46 Search PubMed.
- A. E. Dutkowska and A. Antczak, Comorbidities in lung cancer, Pneumonol. Alergol. Pol., 2016, 84(3), 186–192 CrossRef PubMed.
- Y. Bahader and A. R. Jazieh, Epidemiology of lung cancer, Chest, 2008, 3(6), 592 Search PubMed.
- S. Yang, Z. Zhang and Q. Wang, Emerging therapies for small cell lung cancer, J. Hematol. Oncol., 2019, 12(1), 47 CrossRef PubMed.
- H. Sun, A. Zhang and X. Wang, Potential role of metabolomic approaches for Chinese medicine syndromes and herbal medicine, Phytother. Res., 2012, 26(10), 1466–1471 CAS.
- L. Long, C. Zhao, M. Ozarina, X. Zhao, J. Yang and H. Chen, Targeting immune checkpoints in lung cancer: current landscape and future prospects, Clin. Drug Invest., 2019,(1), 1–13 Search PubMed.
- W. Li, Y. Yang, Z. Ouyang, Q. Zhang, L. Wang and F. Tao, et al., Xiao-ai-ping, a tcm injection, enhances the antigrowth effects of cisplatin on lewis lung cancer cells through promoting the infiltration and function of cd8+ t lymphocytes, Evid.-Based Complementary Altern. Med., 2013, 2013(3), 879512 Search PubMed.
- Z. Y. Yang, X. M. Wu, Y. L. Ou, Y. Ping, L. Jie and X. Y. Song, Effect of tcm combined with chemotherapy on immune function and quality of life of patients with non-small cell lung cancer in stage iii–iv, Chin. J. Integr. Med., 2004, 10(3), 181–186 CrossRef.
- M. Zhu and W. U. Wanyin, Specialized tcm prescription integrated with chemotherapy in the treatment of stages III–IV non-small cell lung cancer: a meta analysis, Tumor, 2013, 33(6) Search PubMed.
- Z. S. Hashemi, S. Khalili, M. Forouzandeh Moghadam and E. Sadroddiny, Lung cancer and mirnas: a possible remedy for anti-metastatic, therapeutic and diagnostic applications, Expert Rev. Respir. Med., 2017, 11(2), 147–157 CrossRef CAS PubMed.
- X. N. Li, A. Zhang and M. Wang, et al., Screening the active compounds of Phellodendri Amurensis cortex for treating prostate cancer by high-throughput chinmedomics, Sci. Rep., 2017, 7, 46234 CrossRef CAS PubMed.
- C. Yingrong, M. Zhihong, M. Lishan, L. Hongwei, W. Bin and Z. Jing, et al., Biomarker identification and pathway analysis by serum metabolomics of lung cancer, BioMed Res. Int., 2015, 2015, 1–9 Search PubMed.
- M. Ros-Mazurczyk, K. Jelonek, M. Marczyk, F. Binczyk, M. Pietrowska and J. Polanska, et al., Serum lipid profile discriminates patients with early lung cancer from healthy controls, Lung Cancer, 2017, S0169500217304361 Search PubMed.
- S. J. S. Cameron, K. E. Lewis, M. Beckmann, G. G. Allison, R. Ghosal and P. D. Lewis, et al., The metabolomic detection of lung cancer biomarkers in sputum, Lung Cancer, 2016, 94, 88–95 CrossRef PubMed.
- A. Zhang, H. Sun, G. Yan, P. Wang and X. Wang, Mass spectrometry-based metabolomics: applications to biomarker and metabolic pathway research, Biomed. Chromatogr., 2016, 30(1), 7–12 CrossRef PubMed.
- A. Zhang, H. Wang and H. Sun, et al., Metabolomics strategy reveals therapeutical assessment of limonin on nonbacterial prostatitis, Food Funct., 2015, 6(11), 3540–3549 RSC.
- H. Fang, A. H. Zhang and H. Sun, et al., High-throughput metabolomics screen coupled with multivariate statistical analysis identifies therapeutic targets in alcoholic liver disease rats using liquid chromatography-mass spectrometry, J. Chromatogr. B: Anal. Technol. Biomed. Life Sci., 2019, 1109, 112–120 CrossRef CAS PubMed.
- A. Zhang, S. Hui and X. Wang, Emerging role and recent applications of metabolomics biomarkers in obesity disease research, RSC Adv., 2017, 7(25), 14966–14973 RSC.
- A. Zhang, H. Sun, G. Yan, P. Wang and X. Wang, Metabolomics for biomarker discovery: moving to the clinic, BioMed Res. Int., 2016, 2015, 1–6 Search PubMed.
- L. R. Euceda, M. K. Andersen, M. B. Tessem, S. A. Moestue, M. T. Grinde and T. F. Bathen, Nmr-based prostate cancer metabolomics, Methods Mol. Biol., 2018, 1786, 237 CrossRef CAS PubMed.
- C. D. Hart, L. Tenori, C. Luchinat and A. Di Leo, Metabolomics in Breast Cancer: Current Status and Perspectives, Adv. Exp. Med. Biol., 2016, 882, 217–234 CrossRef CAS PubMed.
- K. Yao, L. Guoqing, S. Jinhui, L. Cong, W. Jun and L. Jun, et al., Genome-wide profiling reveals that herbal medicine jinfukang-induced polyadenylation alteration is involved in anti-lung cancer activity, Evid.-Based Complementary Altern. Med., 2017, 2017, 1–8 Search PubMed.
- Z. Yan-Juan, Z. Hai-Bo, L. Li-Rong, L. Yi-Hong, Z. Fu-Li and B. Jian-Ping, et al., yin-cold, or, yang-heat, syndrome type of traditional chinese medicine was associated with the epidermal growth factor receptor gene status in non-small cell lung cancer patients: confirmation of a TCM concept, Evid.-Based Complementary Altern. Med., 2017, 2017, 1–7 CrossRef PubMed.
- C. Li, M. Niu, R. Wang, X. W. Zhou, B. Dong, S. Qi, W. Chen, M. Zhang, Y. Shi, R. S. Li and G. Li, The modulatory properties of Si Jun Zi Tang enhancing anticancer of gefitinib by an integrating approach, Biomed. Pharmacother., 2019, 111, 1132–1140 CrossRef CAS PubMed.
- L. Bi, X. Yan, Y. Yang, L. Qian and W. Chen, The component formula of salvia miltiorrhiza and panax ginseng induces apoptosis and inhibits cell invasion and migration through targeting pten in lung cancer cells, Oncotarget, 2017, 8(60), 101599–101613 CrossRef PubMed.
- P. Lü, Inhibitory effects of hyperoside on lung cancer by inducing apoptosis and suppressing inflammatory response via caspase-3 and nf-κb signaling pathway, Biomed. Pharmacother., 2016, 82, 216–225 CrossRef PubMed.
- L. Li, S. Wang, X. Yang, S. Long and S. S. Hann, Traditional chinese medicine, fuzheng kang-ai decoction, inhibits metastasis of lung cancer cells through the stat3/mmp9 pathway, Mol. Med. Rep., 2017, 16(3), 2461–2468 CrossRef CAS PubMed.
- Z. Chen, C. Zhang, F. Gao, Q. Fu and J. Zhang, A systematic review on the rhizome of Ligusticum Chuanxiong Hort. (chuanxiong), Food Chem. Toxicol., 2018, 119, 309–325 CrossRef CAS PubMed.
- Q. Zhang, M. Wang, Q. Wang, H. Zhao, Z. Zhang and H. Yu, et al., Characterization of the potential new phthalides in Ligusticum Chuanxiong Hort. using ultra-performance liquid chromatography coupled with quadrupole time of flight tandem mass spectrometry, J. Sep. Sci., 2017, 40(10), 2123–2130 CrossRef CAS PubMed.
- X. Ran, L. Ma, C. Peng, H. Zhang and L. P. Qin, Ligusticum Chuanxiong Hort: a review of chemistry and pharmacology, Pharm. Biol., 2011, 49(11), 1180–1189 CrossRef CAS PubMed.
- X. Liu, X. Li, S. Ji, X. Cui and M. Li, Screening of bioactive ingredients in Ligusticum Chuanxiong Hort. for protection against myocardial ischemia, Cell. Physiol. Biochem., 2016, 40(3–4), 770–780 CrossRef CAS PubMed.
- J. Hu, X. Jia, X. Fang, P. Li, C. He and M. Chen, Ultrasonic extraction, antioxidant and anticancer activities of novel polysaccharides from chuanxiong rhizome, Int. J. Biol. Macromol., 2016, 85, 277–284 CrossRef CAS PubMed.
- X. Xie, Y. Tian, S. Yin, Y. Lin and G. Tan, Anticancer effects of Ligusticum Chuanxiong Hort. alcohol extracts on hs766t cell, Afr. J. Tradit., Complementary Altern. Med., 2013, 10(6), 542–546 CrossRef PubMed.
- X. Wang, J. Li and A. H. Zhang, Urine metabolic phenotypes analysis of extrahepatic cholangiocarcinoma disease using ultra-high performance liquid chromatography-mass spectrometry, RSC Adv., 2016, 6(67), 63049–63057 RSC.
- Y. Zhang, P. Liu and Y. Li, et al., Exploration of metabolite signatures using high-throughput mass spectrometry coupled with multivariate data analysis, RSC Adv., 2017, 7, 6780–6787 RSC.
- V. Braun and K. Hantke, Lipoproteins: Structure, Function, Biosynthesis., Subcell. Biochem., 2019, 92, 39–77 CrossRef PubMed.
- A. Zhang, H. Sun and P. Wang, et al., Salivary proteomics in biomedical research, Clin. Chim. Acta, 2013, 415, 261–265 CrossRef CAS PubMed.
- P. Salman. and D. C. Cho, Novel Therapeutics Affecting Metabolic Pathways, Am. Soc. Clin. Oncol. Educ. Book, 2019, 39, e79–e87 Search PubMed.
- M. M. Salvador, M. Gómez de Cedrón, J. Merino Rubio, S. F. Martínez, R. S. Martínez and E. Casado, et al., Lipid metabolism and lung cancer, Critical Reviews in Oncology/Hematology, 2017, 112, 31–40 CrossRef PubMed.
- E. Fahy, S. Subramaniam, R. C. Murphy, N. Masahiro., R. H. Raetz Christian, T. Shimizu, S. Friedrich., G. van Meer, J. O. Wakelam Michael and E. A. Dennis, Update of the LIPID MAPS comprehensive classification system for lipids, J. Lipid Res., 2009, S9–S14 CrossRef CAS PubMed.
- F. Gibellini and T. K. Smith, The kennedy pathway-de novo synthesis of phosphatidylethanolamine and phosphatidylcholine, IUBMB Life, 2010, 62(6), 414–428 CrossRef CAS PubMed.
- D. M. Small, The effects of glyceride structure on absorption and metabolism, Annu. Rev. Nutr., 1991, 11(11), 413 CrossRef CAS PubMed.
- H. Cao, A. Zhang and H. Zhang, et al., The application of metabolomics in traditional Chinese medicine opens up a dialogue between Chinese and Western medicine, Phytother. Res., 2015, 29(2), 159–166 CrossRef PubMed.
- M. G. Van, D. R. Voelker and G. W. Feigenson, Membrane lipids: where they are and how they behave, Nat. Rev. Mol. Cell Biol., 2008, 9(2), 112–124 CrossRef PubMed.
- M. Murakami, Novel functions of phospholipase As: overview, Biochim. Biophys. Acta, Mol. Cell Biol. Lipids, 2019, 1864, 763–765 CrossRef CAS PubMed.
- F. Spadaro, C. Ramoni, D. Mezzanzanica, S. Miotti, P. Alberti and S. Cecchetti, et al., Phosphatidylcholine-specific phospholipase cactivation in epithelial ovarian cancer cells, Cancer Res., 2008, 68(16), 6541–6549 CrossRef CAS PubMed.
- A. Zhang, H. Sun and Y. Han, et al., Urinary metabolic biomarker and pathway study of hepatitis B virus infected patients based on UPLC-MS system, PLoS One, 2013, 8(5), e64381 CrossRef CAS PubMed.
- A. Zhang, H. Sun and S. Qiu, et al., Metabolomics in noninvasive breast cancer, Clin. Chim. Acta, 2013, 424, 3–7 CrossRef CAS PubMed.
- A. Zhang, H. Sun and H. Xu, et al., Cell metabolomics, OMICS: J. Integr. Biol., 2013, 17(10), 495–501 CrossRef CAS PubMed; X. Y. Liu, A. H. Zhang and H. Fang, et al., Serum metabolomics strategy for understanding the therapeutic effects of Yin-Chen-Hao-Tang against Yanghuang syndrome, RSC Adv., 2018, 8(14), 7403–7413 RSC.
- A. Zhang, H. Sun and G. Yan, et al., Metabolomics in diagnosis and biomarker discovery of colorectal cancer, Cancer Lett., 2014, 345(1), 17–20 CrossRef CAS PubMed.
- A. Zhang, H. Sun and Y. Han, et al., Exploratory urinary metabolic biomarkers and pathways using UPLC-Q-TOF-HDMS coupled with pattern recognition approach, Analyst, 2012, 137(18), 4200–4208 RSC.
- H. Fang, A. Zhang and J. Yu, et al., Insight into the metabolic mechanism of scoparone on biomarkers for inhibiting Yanghuang syndrome, Sci. Rep., 2016, 6, 37519 CrossRef CAS PubMed.
- X. Li, A. Zhang and H. Sun, et al., Metabolic characterization and pathway analysis of berberine protects against prostate cancer, Oncotarget, 2017, 8, 65022–65041 Search PubMed.
- A. Zhang, H. Sun and G. Yan, et al., Mass spectrometry-based metabolomics: applications to biomarker and metabolic pathway research, Biomed. Chromatogr., 2016, 30(1), 7–12 CrossRef PubMed.
- H. Chu, A. Zhang and Y. Han, et al., Metabolomics approach to explore the effects of Kai-Xin-San on Alzheimer's disease using UPLC/ESI-Q-TOF mass spectrometry, J. Chromatogr. B: Anal. Technol. Biomed. Life Sci., 2016, 1015, 50–61 CrossRef PubMed.
- A. Zhang, H. Sun and G. Yan, et al., Metabolomics for biomarker discovery: moving to the clinic, BioMed Res. Int., 2015, 2015, 1–6 Search PubMed.
- J. Xie, A. H. Zhang and S. Qiu, Identification of the perturbed metabolic pathways associating with prostate cancer cells and anticancer affects of obacunone, J. Proteomics, 2019, 206, 103447 CrossRef CAS PubMed.
- A. Zhang, H. Sun and X. Wang, Emerging role and recent applications of metabolomics biomarkers in obesity disease research, RSC Adv., 2017, 7(25), 14966–14973 RSC.
- H. Xiong, A. H. Zhang and Q. Q. Zhao, et al., Discovery and screening quality-marker ingredients of Panax quinquefolius using chinmedomics approach, Phytomedicine, 2019,(19), 30097–30102 Search PubMed.
- H. Sun, H. Wang and A. Zhang, et al., Berberine ameliorates nonbacterial prostatitis via multi-target metabolic network regulation, OMICS: J. Integr. Biol., 2015, 19(3), 186–195 CrossRef CAS PubMed.
- H. L. Zhang, A. H. Zhang and X. H. Zhou, et al., High-throughput lipidomics reveal mirabilite regulating lipid metabolism as anticancer therapeutics, RSC Adv., 2018, 8(62), 35600–35610 RSC.
- H. Sun, A. Zhang and L. Yang, et al., High-throughput chinmedomics strategy for discovering the quality-markers and potential targets for Yinchenhao decoction, Phytomedicine, 2019, 54, 328–338 CrossRef CAS PubMed.
- H. Sun, A. Zhang and G. Yan, et al., Metabolomic analysis of key regulatory metabolites in hepatitis C virus–infected tree shrews, Mol. Cell. Proteomics, 2013, 12(3), 710–719 CrossRef CAS PubMed.
- A. Zhang, H. Sun and X. Wang, Potentiating therapeutic effects by enhancing synergism based on active constituents from traditional medicine, Phytother. Res., 2014, 28(4), 526–533 CrossRef PubMed.
- Y. Nan, X. Zhou and Q. Liu, et al., Serum metabolomics strategy for understanding pharmacological effects of ShenQi pill acting on kidney yang deficiency syndrome, J. Chromatogr. B: Anal. Technol. Biomed. Life Sci., 2016, 1026, 217–226 CrossRef CAS PubMed.
- A. Zhang, H. Wang and H. Sun, et al., Metabolomics strategy reveals therapeutical assessment of limonin on nonbacterial prostatitis, Food Funct., 2015, 6(11), 3540–3549 RSC.
- A. Zhang, H. Sun and P. Wang, et al., Future perspectives of personalized medicine in traditional Chinese medicine: a systems biology approach, Complement. Ther. Med., 2012, 20(1–2), 93–99 CrossRef PubMed.
- Y. Zhang, P. Liu and Y. Li, et al., Exploration of metabolite signatures using high-throughput mass spectrometry coupled with multivariate data analysis, RSC Adv., 2017, 7, 6780–6787 RSC.
- L. Wang, H. Dong and A. H. Zhang, et al., Exploring the detoxification effects and mechanism of Caowu in prescription using liquid chromatography-high-resolution mass spectrometry-based metabolomics, Open Journal of Proteomics and Genomics, 2018, 3(1), 011–023 Search PubMed.
- A. H. Zhang, H. Sun and G. L. Yan, et al., Chinmedomics: A Powerful Approach Integrating Metabolomics with Serum Pharmacochemistry to Evaluate the Efficacy of Traditional Chinese Medicine, Engineering, 2019, 5, 60–68 CrossRef CAS.
- H. Sun, H. L. Zhang and A. H. Zhang, et al., Network pharmacology combined with functional metabolomics discover bile acid metabolism as a promising target for mirabilite against colorectal cancer, RSC Adv., 2018, 8, 30061–30070 RSC.
- Q. Liang, H. Liu and X. Li, et al., High-throughput metabolomics analysis discovers salivary biomarkers for predicting mild cognitive impairment and Alzheimer's disease, RSC Adv., 2016, 6, 75499–75504 RSC.
- X. Li, Y. Han and A. Zhang, et al., Mechanistic and Therapeutic Advances in Colon Cancer: A Systematic Review, Open Journal of Proteomics and Genomics, 2019, 4(1), 001–012 Search PubMed.
- H. Sun, A. H. Zhang and S. B. Liu, et al., Cell metabolomics identify regulatory pathways and targets of magnoline against prostate cancer, J. Chromatogr. B: Anal. Technol. Biomed. Life Sci., 2018, 1102–1103, 143–151 CrossRef CAS PubMed.
- H. Zhang, A. Zhang and J. Miao, et al., Targeting regulation of tryptophan metabolism for colorectal cancer therapy: a systematic review, RSC Adv., 2019, 9, 3072–3080 RSC.
- A. Zhang, H. Sun and S. Qiu, et al., NMR-based metabolomics coupled with pattern recognition methods in biomarker discovery and disease diagnosis, Magn. Reson. Chem., 2013, 51(9), 549–556 CrossRef CAS PubMed.
- C. C. Feng, A. H. Zhang and J. H. Miao, et al., Recent advances in understanding cross-talk between bile acids and gut microbiota, Open Journal of Proteomics and Genomics, 2018, 3(1), 024–034 Search PubMed.
- A. Zhang, S. Qiu and H. Xu, et al., Metabolomics in diabetes, Clin. Chim. Acta, 2014, 429, 106–110 CrossRef CAS PubMed.
- H. Sun, X. Li and A. Zhang, et al., Exploring potential biomarkers of coronary heart disease treated by Jing Zhi Guan Xin Pian using high-throughput metabolomics, RSC Adv., 2019, 9(20), 11420–11432 RSC.
- A. Zhang, H. Sun and G. Yan, et al., Serum proteomics in biomedical research: a systematic review, Appl. Biochem. Biotechnol., 2013, 170(4), 774–786 CrossRef CAS PubMed.
- X. J. Wang, J. L. Ren and A. H. Zhang, et al., Novel applications of mass spectrometry-based metabolomics in herbal medicines and its active ingredients: current evidence, Mass Spectrom. Rev., 2019, 38(4–5), 380–402 CrossRef PubMed.
- H. Sun, M. Wang and A. Zhang, et al., UPLC-Q-TOF-HDMS Analysis of Constituents in the Root of Two Kinds of Aconitum Using a Metabolomics Approach, Phytochem. Anal., 2013, 24(3), 263–276 CrossRef CAS PubMed.
- A. Zhang, H. Sun and X. Wang, Potentiating therapeutic effects by enhancing synergism based on active constituents from traditional medicine, Phytother. Res., 2014, 28(4), 526–533 CrossRef PubMed.
- G. Yan, A. Zhang and H. Sun, et al., An effective method for determining the ingredients of S huanghuanglian formula in blood samples using high-resolution LC-MS coupled with background subtraction and a multiple data processing approach, J. Sep. Sci., 2013, 36(19), 3191–3199 CAS.
- A. H. Zhang, J. B. Yu and H. Sun, et al., Identifying quality-markers from Shengmai San protects against transgenic mouse model of Alzheimer's disease using chinmedomics approach, Phytomedicine, 2018, 45(18), 84–92 CrossRef CAS PubMed.
- A. Zhang, H. Sun and S. Qiu, et al., Metabolomics insights into pathophysiological mechanisms of nephrology, Int. Urol. Nephrol., 2014, 46(5), 1025–1030 CrossRef CAS PubMed.
- H. Wang, G. Yan and A. Zhang, et al., Rapid discovery and global characterization of chemical constituents and rats metabolites of Phellodendri amurensis cortex by ultra-performance liquid chromatography-electrospray ionization/quadrupole-time-of-flight mass spectrometry coupled with pattern recognition approach, Analyst, 2013, 138(11), 3303–3312 RSC.
- X. Wang, J. Li and A. H. Zhang, Urine metabolic phenotypes analysis of extrahepatic cholangiocarcinoma disease using ultra-high performance liquid chromatography-mass spectrometry, RSC Adv., 2016, 6(67), 63049–63057 RSC.
- A. Zhang, H. Sun and G. Yan, et al., Metabolomics study of type 2 diabetes using ultra-performance LC-ESI/quadrupole-TOF high-definition MS coupled with pattern recognition methods, J. Physiol. Biochem., 2014, 70(1), 117–128 CrossRef CAS PubMed.
- Y. F. Li, S. Qiu and L. J. Gao, et al., Metabolomic estimation of the diagnosis of hepatocellular carcinoma based on ultrahigh performance liquid chromatography coupled with time-of-flight mass spectrometry, RSC Adv., 2018, 8(17), 9375–9382 RSC.
- X. Wang, A. Zhang and X. Zhou, et al., An integrated chinmedomics strategy for discovery of effective constituents from traditional herbal medicine, Sci. Rep., 2016, 6, 18997 CrossRef CAS PubMed.
- H. Sun, A. H. Zhang and Q. Song, et al., Functional metabolomics discover pentose and glucuronate interconversion pathways as promising targets for Yang Huang syndrome treatment with Yinchenhao Tang, RSC Adv., 2018, 8, 36831–36839 RSC.
- Y. Zhao, H. Lv and S. Qiu, et al., Plasma metabolic profiling and novel metabolite biomarkers for diagnosing prostate cancer, RSC Adv., 2017, 7(48), 30060–30069 RSC.
Footnotes |
† Electronic supplementary information (ESI) available. See DOI: 10.1039/c9ra06267j |
‡ These authors contributed equally to this work. |
|
This journal is © The Royal Society of Chemistry 2019 |