DOI:
10.1039/C9RA01523J
(Paper)
RSC Adv., 2019,
9, 16884-16891
Identification and characterization of differentially expressed miRNAs in HepG2 cells under normoxic and hypoxic conditions†
Received
28th February 2019
, Accepted 23rd May 2019
First published on 29th May 2019
Abstract
MicroRNAs (miRNAs) are important post-transcriptional regulators involved in hypoxia conditions; however, their roles in HepG2 cells remain poorly understood. Our previous study showed that hypoxia treatment modulated gene expression accompanied by with HepG2 cell proliferation arrest and increased cell death. To better understand the mechanism of phenotypic changes of HepG2 under hypoxia conditions; we conducted a comparative RNA sequencing to identify differentially expressed miRNAs between hypoxia treatment and control cells. In total, 165 differentially expressed miRNAs were identified, among which the expression of 114 miRNAs were up-regulated and that of 51 miRNAs were down-regulated in hypoxia treated HepG2 cells. Expression profiles of eleven randomly selected miRNAs were validated by qRT-PCR. Furthermore, 19
367 annotated target genes of differentially expressed miRNAs were predicted by bioinformatics tools. The Gene Ontology analysis indicated that the molecular function of target genes was primarily related to binding and catalytic activity, and that the Kyoto Encyclopedia of Genes and Genomes annotation for target genes were further classified into pathways involved in cellular processes, metabolism, organismal systems, genetic information processing, human disease and environmental information processing. Among the environmental information processing, certain pathways associated with cell proliferation and apoptosis, such as the hippo signalling pathway, wnt signalling pathway, MAPK signalling pathway and Jak-STAT signaling pathways, represented potential factors in the response to hypoxia treatment. In conclusion, the expression profiles of miRNA in HepG2 cells were significantly altered under hypoxia conditions; which were closely related to cell proliferation arrest and apoptosis. Our findings expand our understanding of miRNAs function in regulating cell fate under hypoxia conditions.
Introduction
MicroRNAs (miRNAs) represent the largest family of short non-coding RNA molecules, with a length of 19–23 nucleotides.1 MiRNAs regulate the expression of target genes in a post transcriptional manner, through incomplete base pairing with the 3′ UTR of target mRNAs, inhibiting translation and/or causing mRNA degradation.2 These may contribute to the regulation of a wide variety of cellular functions. More specifically, miRNAs have recently emerged as important regulators of cellular responses under hypoxia. Hypoxia-induced miR-137 production regulates retinal ganglion cell apoptosis by targeting Notch1.3 MiR-145 aggravates hypoxia induced injury via targeting Rac1 in H9c2 cells.4 MiR-485 inhibits the malignant behavior of glioblastoma cells by targeting p21-activated kinase 4 (PAK4).5 MiR-320 may regulate the development of autophagy by targeting HIF-1α in retinoblastoma under hypoxia conditions.6 miR-210 and miR-155 are related to cell cycle, proliferation and autophagy.7–9 MiR-338-3p inhibits hepatocarcinoma cells proliferation by targeting hypoxia-induced factor 1α.10 MiR-26a modulates the angiogenesis of hepatocellular carcinoma through the PIK3C2α/Akt/HIF-1α/VEGFA pathway.11 Nevertheless, miRNAs expression profiles in HepG2 cells under hypoxic conditions are still unclear.
Hypoxia is commonly observed in malignant neoplastic tissue as tumors increase in size but lack neurovascularization.12 In our previous study, we found that hypoxia treatment could modulate gene expression in HepG2 cells accompanied with cell proliferation arrest and increased cell death.13 To better understand the biological function of miRNAs in the context of HepG2 under hypoxic condition, Illumina deep sequencing was applied to identify differentially expressed miRNAs by comparing small RNA libraries obtain from hypoxia treatment and control cells. Gene Ontology (GO) annotation, Clusters of Orthologous Groups (COG) and Kyoto Encyclopedia of Genes and Genomes (KEGG) analyses showed that the putative miRNA target genes were involved in a variety of biological pathways, among which cell proliferation and apoptosis related pathways, as well as the hippo, wnt, MAPK and Jak-STAT signalling pathways, may play a role in response to hypoxia treatment. These results will provide novel insights into the mechanism of miRNA-mediated gene expression under hypoxia treatment and shed light on miRNA therapeutic targets of hepatocellular carcinoma.
Results
Construction of small RNA libraries
To identify the differentially expressed miRNAs in HepG2 cells response to hypoxia, six small RNA libraries representing the normoxic groups (N1, N2, N3) and hypoxia treatment groups (H1, H2, H3) were constructed from total RNA and subject to Illumina small RNA sequencing. After removing contaminant reads, 33.8, 26.1, 27.8, 35.9, 30.0 and 38.4 million clean reads were generated in the N1, N2, N3, H1, H2 and H3 samples, respectively (Table S1†). Further, these clean reads were compared with the Silva, Rfam, GtRNAdb and Repbase databases to filter the non-coding RNAs such as tRNAs, rRNAs, snRNAs and snoRNAs. A total of 92.36% (N1), 92.19% (N2), 90.95% (N3), 91.72% (H1), 75.34% (H2) and 90.37% (H3) distinct reads of the total small RNAs were identified as conserved miRNAs and were applied to subsequent analyses (Table S2†).
Next, the filtered reads were aligned against the human genome (GRCh37, http://grch37.ensembl.org/Homo_sapiens/Info/Index), and the mapped reads were selected to identify known or novel miRNAs by miRDeep2 software.14 In total, 2118 miRNAs were successfully identified in six libraries, and among them, 1472 were known miRNAs and 646 were novel miRNAs (Table S3†). The small RNA length distribution of the known and predicted miRNAs showed that the most abundant species were all 22 nt long, a typical size range for Dicer-derived products (Fig. 1).
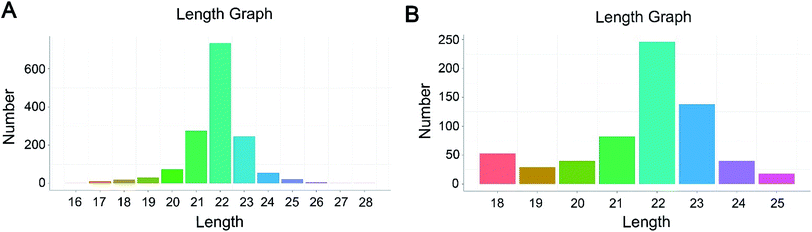 |
| Fig. 1 Frequency distribution of sequence lengths of the known miRNAs (A) and novel miRNAs (B). | |
Differentially expressed miRNAs in HepG2 cells under normoxic and hypoxic conditions
The expression level of miRNAs expressed in normoxic and hypoxic conditions were quantified by TPM. If the TPM with |log
2FC| ≥ 1 and FDR adjusted p-value < 0.05, the miRNAs were considered as differentially expressed. Compared with libraries of N1 to H1, N2 to H2, N3 to H3, 29, 72 and 133 miRNAs were up-regulated, while 37, 23 and 73 were down-regulated, respectively (Tables S4–S6†). Additionally, 69 miRNAs were differentially expressed in at least two pairwise comparisons (Fig. 2). The hierarchical clustering of DE miRNAs classifies the samples into normoxic and hypoxic group based on the miRNAs expression. The results showed that the six samples could be sorted into two distinct groups based on hypoxia treatment (Fig. 3).
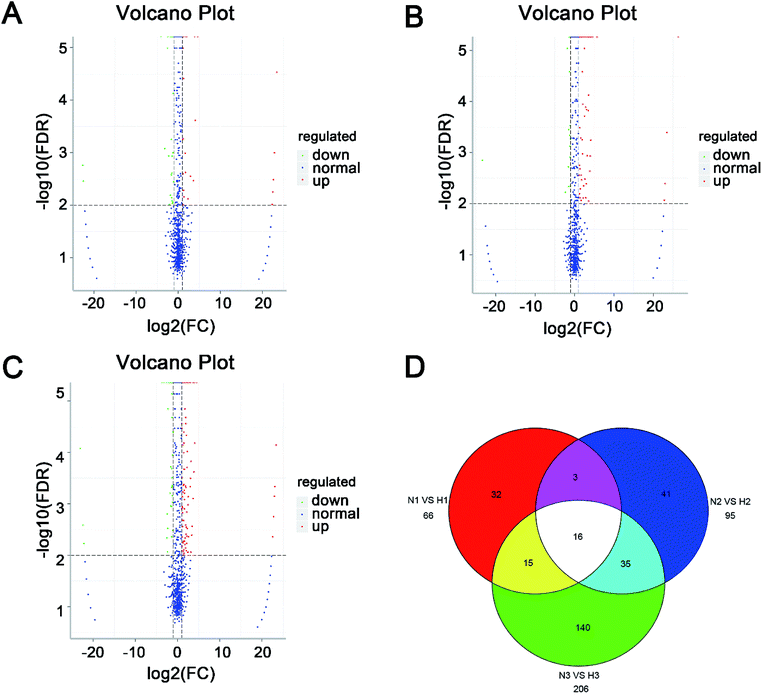 |
| Fig. 2 Volcano plot and Venn diagram of differentially expressed miRNAs in six libraries. (A) Libraries N1 vs. H1. (B) Libraries N2 vs. H2. (C) Libraries N3 vs. H3. (D) Venn diagram of total differentially expressed miRNAs. | |
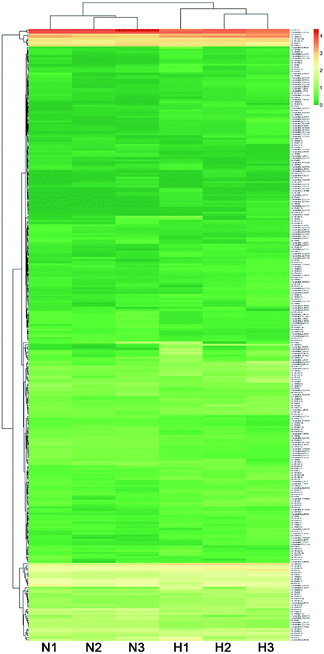 |
| Fig. 3 Hierarchical clustering of DE miRNAs among six libraries. | |
Validation of miRNAs expression by qRT-PCR
The expression level of eleven differentially expressed conserved miRNAs were selected and verified by qRT-PCR (Fig. 4). Seven (miR-210-3p, miR-210-5p, miR-139-3p, miR-935, miR-206, miR-615-5p and miR-873-5p) were common between the three sets of libraries and another four (miR-194-5p, miR-449a, miR-19a-3p and miR-1277) were common between at least one set of libraries. Consistent with the miRNA sequencing data, the expression profile of these twelve miRNAs showed a similar pattern of expression in the pairwise comparisons, despite a slight difference in fold change, which further confirmed that our sequencing data were reproducible and reliable.
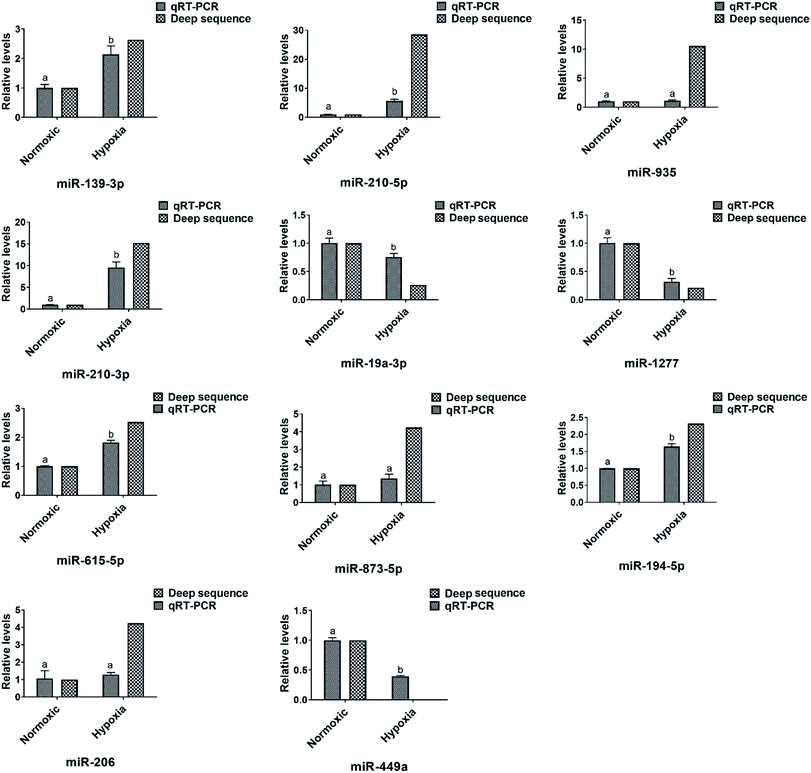 |
| Fig. 4 Relative expression levels of six selected miRNAs in the three libraries according to qRT-PCR and high-throughput sequencing. The expression levels of miRNAs in the normoxic library were set to 1. Values were described as mean ± SD (n = 3). Differences were determined by one-way analysis of variance (ANOVA). Different letters above pillars indicated statistical significance at statistical significance at the level of p < 0.01. | |
Target genes prediction of the total and differentially expressed miRNAs
To illustrate their functions of the miRNAs identified in the present study, target genes prediction was carried out using miRanda and RNAhybrid methods. In total, 19
564 genes were identified in the current study as potential targets of the 1589 miRNAs from six libraries (Table S7†). Among them, 19
367 genes were annotated by searching against the Nr, Swiss-Prot, GO, COG, KEGG, KOG and Pfam databases (Table S8†). Annotated target genes of differentially expressed miRNAs in pairwise comparisons are shown in Table S9.†
GO, COG and KEGG pathway annotation of miRNA target genes
According to the GO classification system, the target genes were classified into three major functional categories (biological process, cellular component and molecular function) and 62 subcategories (Fig. S1, Table S10†). Genes involved in “cell part” (11
978), “cell” (11
970), “organelle” (8930), and “membrane” (6562) groups were notably represented in the cellular component category. In the category of molecular function,” binding” (9930) was the most commonly represented category, followed by “catalytic activity” (4638), “molecular transducer activity” and “receptor activity” (1370). Among the biological processes, a significant proportion of clusters were assigned to “cellular process” (10
107), “single-organism process” (9664), “biological regulation” (8263) and “metabolic process” (6994).
To classify orthologous genes products, the target genes of the differentially expressed miRNAs were subdivided into 25 COG classifications. Among them, the cluster of “General function prediction only” represented the largest group, followed by “Transcription” and “Replication, recombination and repair” (Fig. S2†).
KEGG pathway analysis for the target genes classified them into six biochemical pathways, namely, cellular processes, metabolism, organism systems, genetic information processing, human disease and environmental information processing, in the three pairwise comparisons (N1 vs. H1, N2 vs. H2, N3 vs. H3) (Fig. S3†).
Among the environmental information processing pathways, the hippo, wnt, MAPK and Jak-STAT signalling pathways, represented potential factors in response to hypoxia treatment. In total, 28 proteins involved in these four pathways were identified as the target of DE miRNAs list in Table 1 (target proteins were predicted by TargetScanHuman, http://www.targetscan.org/vert_71/), 20 of which have known functions in cell proliferation and apoptosis, while the rest 8 proteins function in cell proliferation and apoptosis needs to be further studied.
Table 1 List of DE miRNAs targeted proteins involved in cell proliferation and apoptosis related signal pathways
Index |
Signal pathway name |
DE miRNA name |
Up/down |
Target protein |
Target protein ID |
Target proteins function in cell proliferation and apoptosis |
1 |
Hippo signaling pathway |
miR-216a-3p |
Up |
CRB1 |
NP_957705 |
Bulgakova et al., 2009 15 |
miR-424-5p |
Up |
LATS2 |
NP_055387 |
Nishiyama et al., 1999;16 |
Avruch et al., 2012 17 |
miR-27a-3p |
Up |
YAP |
NP_001123617 |
Zhao et al., 2010;18 |
Zhao et al., 2008;19 |
Schlegelmilch et al., 2011 20 |
miR-9-5p |
Up |
FRMD6 |
NP_001253975 |
Angus et al., 2012 21 |
miR-193a-3p |
Down |
AJUBA |
NP_116265 |
Hirota et al., 2003;22 |
Das et al., 2010 23 |
miR-141-3p |
Up |
DLG3 |
NP_066943 |
Makino et al., 1997 24 |
2 |
Wnt signaling pathway |
miR-122-5p |
Down |
FRAT1 |
NP_005470 |
van Amerongen et al., 2004 25 |
miR-29b-3p |
Down |
FRAT2 |
NP_036215 |
Saitoh et al., 2001 26 |
miR-145-5p |
Down |
SENP2 |
NP_067640 |
— |
miR-194-5p |
Up |
CHD8 |
NP_001164100 |
— |
miR-27a-3p |
Up |
LRP5 |
NP_002326 |
Li et al., 2005;27 |
|
Dong et al., 1998 28 |
miR-424-5p |
Up |
LRP6 |
NP_002327 |
Li et al., 2005;27 |
Dong et al., 1998 28 |
miR-129-5p |
Up |
CXXC4 |
NP_079488 |
— |
miR-873-5p |
Up |
CTNNBIP1 |
NP_001012329 |
— |
miR-362-3p |
Up |
RUVBL1 |
NP_003698 |
Shen et al., 2000;29 |
Gartner et al., 2003 30 |
miR-141-3p/miR-194-5p |
Up/up |
SOX17 |
NP_071899 |
— |
miR-19b-3p/miR-19a-3p/miR-122-5p/miR-194-5p |
Down/down/down/Up |
MMP7 |
NP_002414 |
Fanjul-Fernandez et al., 2010;31 |
Amalinei et al., 2007 32 |
3 |
MAPK signaling pathway |
miR-29b-3p |
Down |
DUSP2 |
NP_004409 |
Bermudez et al., 2010 33 |
miR-122-5p |
Down |
DUSP4 |
NP_001385 |
Keyse et al., 2008;34 |
Patterson et al., 2009 35 |
miR-145-5p |
Down |
MAP4K4 |
NP_001229488 |
— |
miR-181a-2-3p |
Up |
MAP4K1 |
NP_009112 |
— |
miR-19a-3p/miR-19b-3p/miR-301b-3p |
Down/down/down |
MAP3K12 |
NP_006292 |
Craig et al., 2008 36 |
miR-34a-3p/miR-30b-3p |
Up/up |
PPP5D1 |
NP_001192210 |
von Kriegsheim et al., 2006 37 |
4 |
Jak-STAT signaling pathway |
miR-216a-5p |
Up |
JAK1 |
NP_001307852 |
Pencik et al., 2016 38 |
miR-1275/miR-625-5p |
Up/up |
PIAS3 |
NP_006090 |
— |
miR-499a-5p |
Down |
CDKN1A |
NP_000380 |
Abbas et al., 2009;39 |
Harper et al., 1993 40 |
miR-5000-3p |
Up |
MYC |
NP_002458 |
Bellosta et al., 2005 41 |
miR-424-5p/miR-141-3p |
Up/up |
CCND2 |
NP_001750 |
Xiong et al., 1992;42 |
Sweeney et al., 1997;43 |
Jena et al., 2002 44 |
Discussion
Increasing evidence suggests that miRNAs are involved in human cancer, and thus are promising therapeutic targets. The facts that various miRNAs are strikingly deregulated in many kinds of cancer cells under hypoxia stress strongly suggests that they may play important roles in cancer development.45–48 However, the precise roles of miRNAs in hepatocellular carcinoma development under hypoxia stress are far from clear. In the present study, we performed a high-throughput RNA sequencing to identify miRNAs in HepG2 cells response to the hypoxia treatment and performed an integrative analysis of their target genes and dynamic of biological process, which provides deeper insight into the expression profiles of miRNAs in HepG2 cells under hypoxia stress.
In the present study, we found that hypoxia-regulated-miRNAs, miR-210-3p and miR-210-5p, were upregulated in hypoxia treated HepG2 cells compared with normal cells (Table S2† and Fig. 4). Consistently, Yang (2012) reported that miR-210 was upregulated in hypoxic human hepatoma cells in vitro and inhibition of miR-210 cause proliferation arrest and cell apoptosis.49 Taken together, the present study successfully achieved hypoxia in vitro.
Our previous study demonstrated that adenylate kinase isozyme 4 (AK4) was downregulated in HepG2 cells accompanied with cell proliferation and cell cycle arrest under hypoxia stress;13 however, its molecular regulation is still unclear. Among the DE miRNAs in the present study, miR-34c-5p, miR-362-3p; miR-320d, miR-190b; and miR-199a-3p, miR-503-5p, miR-9-5p and miR-424-5p were predicted to target AK4 mRNA by miRDB, miRanda and targetscan, respectively. These miRNAs were all upregulated in at least one pairwise comparison (Table S2†). Similarly, Wang (2018) reported that miR-199a-3p affects the multi-chemoresistance of osteosarcoma by targeting AK4.50 Therefore, DE miRNAs from the present study have good theory guidance and reference value to the rational design of the clinical therapy against hepatocarcinoma.
GO annotation illustrated that the predicted target genes of differentially expressed miRNAs were mainly classified into biological processes related to cellular process, single-organism process, biological regulation, metabolic process, and response to stimulus. The COG annotation classified the target proteins into transcription; replication, recombination and repair; and signal transduction mechanisms. Interestingly, KEGG pathway annotation of target genes identified three cell proliferation and cell cycle related pathways, including the hippo, wnt and MAPK signalling pathways. The hippo signaling pathway controls diverse aspects of cell proliferation, survival, and morphogenesis in cancer cells.51,52 hsa-miR-27a-3p and hsa-miR-1277-5p may negatively regulate cell proliferation and cell apoptosis by inhibiting the expression of enzymes such as yes associated protein 1 (YAP1) and large tumor suppressor kinase 1/2 (Lats1/2) which have been found to take part in cell proliferation and apoptosis.18,53
Wnt proteins are secreted morphogens that are required for basic developmental processes, such as cell-fate specification, progenitor-cell proliferation and the control of asymmetric cell division, in many different species and organs.54 In the present study, 10 target gene related enzymes of upregulated miRNA, including cerberus 1 (Cer-1), secreted frizzled related protein (SFRP), porcupine O-acyltransferase (Porc), sclerostin (SOST), frequently rearranged in advanced T-cell lymphomas 1 (FRAT1), SUMO specific peptidase 2 (SENP2), chromodomain helicase DNA binding protein 8 (CHD8), VANGL planar cell polarity protein 2 (VANGL2), prickle planar cell polarity protein (Prickle) and dishevelled associated activator of morphogenesis 1 (Daam1) are involved in the Wnt signaling pathway, suggesting that some miRNAs might regulate cell proliferation and the cell cycle via the suppression of gene expression involved in the Wnt pathway.
The mitogen-activated protein kinase (MAPK) cascade is a highly conserved pathway module that is involved in various cellular functions, including cell proliferation, differentiation and migration.55 Eleven miRNA target gene related enzymes were associated with stress-activated JNK and p38 MAPK pathways, which are crucial for cell proliferation and the cell cycle. Among the 11 enzymes, six, HGK, HPK1, LZK, MUK, PTP and MKP, were related to upregulated miRNA target genes, two, GCK and JIP1/2 were related to downregulated miRNA target genes, and three, JIP3, PP5 and TAO1/2 were related to both upregulated and downregulated miRNA target genes. Taken together, the JNK and p38 MAPK pathways might play an important role in the balance between proliferation and the cell cycle under hypoxia stress.
In summary, (1) the current study clearly demonstrates the differentially expressed miRNA patterns in HepG2 cells under hypoxia and normoxic conditions; (2) in total, 20 target proteins of DE miRNAs involved in environmental information processing pathways were found functions in cell proliferation and apoptosis, suggesting DE miRNAs play important roles in regulation of cellular function under hypoxia condition; (3) the present findings may provide a theoretical foundation for miRNA therapeutic targets screening of hepatocellular carcinoma.
Materials and methods
Cell culture
HepG2 cells were obtained from ATCC and cultured in Dulbecco Modified Eagle's Minimal essential medium supplemented with 10% FBS, 1% penicillin/streptomycin (all from Hyclone, UT, USA).
Hypoxia treatment
HepG2 cells were seeded into 60 mm2 culture plates and incubated for 24 h in a humidified atmosphere containing 5% CO2 at 37 °C. For the hypoxia treatment, cells were transferred into a modular incubation chamber (Billups-Rothenberg, San Diego, CA, USA) with 5% CO2 and 1% O2 balanced with N2 at 37 °C for 72 h. Normoxic control cells were incubated under the regular conditions.
Sample collection and preparation
Cells under normoxic conditions (N1, N2 and N3) and hypoxia conditions (H1, H2 and H3) had three biological replicates. Both groups of cells (N1 and H1, N2 and H2 and N3 and H3) were quickly harvested by a cell scraper after the indicated period, then frozen in liquid nitrogen and stored at −80 °C. Total RNA was extracted using Trizol reagent (Invitrogen, Carlsbad, CA, USA) following the manufacturer's protocol. Nanodrop, Qubit 2.0 and the Agilent 2100 bioanalyzer were used respectively to detect the purity of RNA samples, as well as their concentration and integrity, to ensure that only high-quality samples were sequenced. RNA purity was checked using the NanoPhotometer® spectrophotometer (IMPLEN, CA, USA). RNA concentration was measured using the Qubit® RNA Assay Kit on a Qubit®2.0 Fluorometer (Life Technologies, CA, USA). RNA integrity was assessed using the RNA Nano 6000 Assay Kit on the Agilent Bioanalyzer 2100 system (Agilent Technologies, CA, USA).
Library preparation for small RNA sequencing
A total amount of 1.5 μg RNA per sample was used as input material for the RNA sample preparations. Sequencing libraries were generated using the NEBNext® Ultra™ small RNA Sample Library Prep Kit for Illumina® (NEB, USA) following the manufacturer's recommendations and index codes were added to attribute sequences to each sample.
Bioinformatics analysis of small RNA sequences
Clustering of the index-coded samples was performed on a cBot Cluster Generation System using TruSeq PE Cluster Kit v4-cBot-HS (Illumina) according to the manufacturer's instructions. After cluster generation, the library preparations were sequenced on an Illumina Hiseq 2500 platform and paired-end reads were generated. Using Bowtie tools software, the Clean Reads respectively with Silva database, GtRNAdb database, Rfam database and Repbase database sequence alignment, filter ribosomal RNA (rRNA), transfer RNA (tRNA), small nuclear RNA (snRNA), small nucleolar RNA (snoRNA) and other ncRNA and repeats. The remaining reads were used to detect known miRNAs and new miRNAs were predicted by comparing with known miRNAs from miRBase. Randfold tools software was used for new miRNA secondary structure prediction. MiRNA target gene function was annotated based on the following databases: Nr (NCBI non-redundant protein sequences); Pfam (Protein family); KOG/COG (Clusters of Orthologous Groups of proteins); Swiss-Prot (a manually annotated and reviewed protein sequence database); KO (KEGG Ortholog database); GO (Gene Ontology).
Analysis of differentially expressed miRNAs
Differential expression analysis of miRNA under normoxic and hypoxic conditions was performed using the DESeq R package (1.10.1). DESeq provides statistical pipelines for determining differential expression in digital miRNA expression data using a model based on the negative binomial distribution. The resulting p-values were adjusted using the Benjamini and Hochberg's approach for controlling the false discovery rate. miRNAs with an adjusted p < 0.05 found by DESeq were assigned as differentially expressed.
GO and KEGG pathway annotation analyses
Gene Ontology (GO) analysis of target genes was performed to predict the potential biological processes and functions that were most likely to be affected by miRNAs using Database for Annotation, Visualization and Integration Discovery (DAVID) (Ashburner et al., 2000
56). Significant GO categories, biological functions and different canonical pathways were analyzed for miRNA specific targets as well as for all screened targets based on significant over-representation of genes using the Fisher test. Then, the miRNA target genes were annotated in KEGG pathways using DAVID (Kanehisa et al., 2004
57).
qPCR validation of identified miRNAs
The relative expression of five miRNAs (miR-210-3p, miR-210-5p, miR-935, miR-873-5p and miR-139-3p) were randomly selected and analyzed by quantifying the miRNA stem-loop. Total RNAs were isolated using Trizol reagent (Invitrogen, CA, USA), following the cDNA generation using 1 μg of total RNA by reverse transcription kit (Toyobo, Osaka, Japan). Quantitative real-time PCR (qRT-PCR) was performed on an ABI Step One Plus system (Applied Biosystems, Foster, CA, USA) by using qRT-PCR Reagents provided by Toyobo. The stem-loop primers are shown in the ESI (Table S11†). All reactions were performed in triplicates with reaction volume of 20 μL (10 μL of 2× THUNDERBIRD® SYBR® qPCR mix, 0.4 μL of PCR forward primer, 0.4 μL of PCR reverse primer, 2 μL of cDNA and 7.2 μL of nuclease-free H2O) and were then incubated (95 °C for 10 min, then 40 cycles at 95 °C for 10 s, 60 °C for 30 s). The comparative Ct method and the internal control miRNA gene U6 were used for the calculations of the relative expression levels of the genes. All the qPCR reactions yielded a single peak on the dissociation curve, indicating specific amplifications.
Statistical analysis
Data from the qRT-PCR analyses are presented as mean ± SD. One-way ANOVA was performed to examine the differential expressions of miRNAs. p-Values < 0.05 were considered statistically significant.
Conflicts of interest
The authors declare that they have no conflicts of interest.
Acknowledgements
This work was supported by the Natural Science Foundation of Heilongjiang Province (grant no. C2016043), the Heilongjiang Provincial Key Laboratory of Prevention and Control of Bovine Diseases (grant no. PCBD201709), the Talent Introduction Foundation of Heilongjiang Bayi Agricultural University (grant no. XYB2013-13), the Heilongjiang Province Postdoctoral Science Foundation (grant no. LBH-Q17139 and LBH-Z18259), the Program for Young Scholars with Creative Talents in HeiLongJiang BaYi Agricultural University (grant no. CXRC2017005), the State Key Laboratory of Veterinary Biotechnology Foundation (SKLVBF201908), the National Natural Science Foundation of China (grant no. 31772695). We thank International Science Editing (http://www.internationalscienceediting.com) for editing this manuscript.
References
- M. Ha and V. N. Kim, Nat. Rev. Mol. Cell Biol., 2014, 15, 509–524 CrossRef CAS PubMed.
- M. van Kouwenhove, M. Kedde and R. Agami, Nat. Rev. Cancer, 2011, 11, 644–656 CrossRef CAS PubMed.
- H. Li, Z. Zhu, J. Liu, J. Wang and C. Qu, Int. J. Mol. Med., 2018, 41, 1774–1782 CAS.
- X. Wang, Y. Zhang, H. Wang, G. Zhao and X. Fa, Cell. Physiol. Biochem., 2017, 43, 1974–1986 CrossRef CAS PubMed.
- K. Mao, D. Lei, H. Zhang and C. You, Int. J. Oncol., 2017, 51, 1521–1532 CrossRef CAS PubMed.
- Y. Liang, X. Chen and Z. Liang, Exp. Ther. Med., 2017, 14, 2367–2372 CrossRef CAS PubMed.
- S. Yin, S. Yang, X. Pan, A. Ma, J. Ma, H. Pei, Y. Dong, S. Li, W. Li and X. Bi, Mol. Med. Rep., 2018, 18, 2798–2806 CAS.
- X. Lin, L. Chen, H. Li, Y. Liu, Y. Guan, X. Li, Z. Jia, X. Lin, J. Jia, Y. Sun and D. Xiao, Am. J. Physiol.: Gastrointest. Liver Physiol., 2018, 315, G443–G453 CrossRef CAS PubMed.
- Z. Wang, M. Deng, Z. Liu and S. Wu, Oncol. Rep., 2017, 37, 3010–3018 CrossRef CAS PubMed.
- H. Xu, L. Zhao, Q. Fang, J. Sun, S. Zhang, C. Zhan, S. Liu and Y. Zhang, PLoS One, 2014, 9, e115565 CrossRef PubMed.
- Z. T. Chai, J. Kong, X. D. Zhu, Y. Y. Zhang, L. Lu, J. M. Zhou, L. R. Wang, K. Z. Zhang, Q. B. Zhang, J. Y. Ao, M. Wang, W. Z. Wu, L. Wang, Z. Y. Tang and H. C. Sun, PLoS One, 2013, 8, e77957 CrossRef CAS PubMed.
- P. Vaupel, Microvasc. Res., 1977, 13, 399–408 CrossRef CAS PubMed.
- F. Kong, B. Binas, J. H. Moon, S. S. Kang and H. J. Kim, Arch. Biochem. Biophys., 2013, 533, 11–17 CrossRef CAS PubMed.
- M. R. Friedlander, S. D. Mackowiak, N. Li, W. Chen and N. Rajewsky, Nucleic Acids Res., 2012, 40, 37–52 CrossRef PubMed.
- N. A. Bulgakova and E. Knust, J. Cell Sci., 2009, 122, 2587–2596 CrossRef CAS PubMed.
- Y. Nishiyama, T. Hirota, T. Morisaki, T. Hara, T. Marumoto, S. Iida, K. Makino, H. Yamamoto, T. Hiraoka, N. Kitamura and H. Saya, FEBS Lett., 1999, 459, 159–165 CrossRef CAS PubMed.
- J. Avruch, D. Zhou, J. Fitamant, N. Bardeesy, F. Mou and L. R. Barrufet, Semin. Cell Dev. Biol., 2012, 23, 770–784 CrossRef CAS PubMed.
- B. Zhao, L. Li, Q. Lei and K. L. Guan, Genes Dev., 2010, 24, 862–874 CrossRef CAS PubMed.
- B. Zhao, Q. Y. Lei and K. L. Guan, Curr. Opin. Cell Biol., 2008, 20, 638–646 CrossRef CAS PubMed.
- K. Schlegelmilch, M. Mohseni, O. Kirak, J. Pruszak, J. R. Rodriguez, D. Zhou, B. T. Kreger, V. Vasioukhin, J. Avruch, T. R. Brummelkamp and F. D. Camargo, Cell, 2011, 144, 782–795 CrossRef CAS PubMed.
- L. Angus, S. Moleirinho, L. Herron, A. Sinha, X. Zhang, M. Niestrata, K. Dholakia, M. B. Prystowsky, K. F. Harvey, P. A. Reynolds and F. J. Gunn-Moore, Oncogene, 2012, 31, 238–250 CrossRef CAS PubMed.
- T. Hirota, N. Kunitoku, T. Sasayama, T. Marumoto, D. Zhang, M. Nitta, K. Hatakeyama and H. Saya, Cell, 2003, 114, 585–598 CrossRef CAS PubMed.
- M. Das Thakur, Y. Feng, R. Jagannathan, M. J. Seppa, J. B. Skeath and G. D. Longmore, Curr. Biol., 2010, 20, 657–662 CrossRef CAS PubMed.
- K. Makino, H. Kuwahara, N. Masuko, Y. Nishiyama, T. Morisaki, J. Sasaki, M. Nakao, A. Kuwano, M. Nakata, Y. Ushio and H. Saya, Oncogene, 1997, 14, 2425–2433 CrossRef CAS PubMed.
- R. van Amerongen, H. van der Gulden, F. Bleeker, J. Jonkers and A. Berns, J. Biol. Chem., 2004, 279, 26967–26974 CrossRef CAS PubMed.
- T. Saitoh, J. Moriwaki, J. Koike, A. Takagi, T. Miwa, K. Shiokawa and M. Katoh, Biochem. Biophys. Res. Commun., 2001, 281, 815–820 CrossRef CAS PubMed.
- X. Li, Y. Zhang, H. Kang, W. Liu, P. Liu, J. Zhang, S. E. Harris and D. Wu, J. Biol. Chem., 2005, 280, 19883–19887 CrossRef CAS PubMed.
- Y. Dong, W. Lathrop, D. Weaver, Q. Qiu, J. Cini, D. Bertolini and D. Chen, Biochem. Biophys. Res. Commun., 1998, 251, 784–790 CrossRef CAS PubMed.
- X. Shen, G. Mizuguchi, A. Hamiche and C. Wu, Nature, 2000, 406, 541–544 CrossRef CAS PubMed.
- W. Gartner, J. Rossbacher, B. Zierhut, T. Daneva, W. Base, M. Weissel, W. Waldhausl, M. S. Pasternack and L. Wagner, Cell Motil. Cytoskeleton, 2003, 56, 79–93 CrossRef CAS PubMed.
- M. Fanjul-Fernandez, A. R. Folgueras, S. Cabrera and C. Lopez-Otin, Biochim. Biophys. Acta, 2010, 1803, 3–19 CrossRef CAS PubMed.
- C. Amalinei, I. D. Caruntu and R. A. Balan, Rom. J. Morphol. Embryol., 2007, 48, 323–334 Search PubMed.
- O. Bermudez, G. Pages and C. Gimond, Am. J. Physiol.: Cell Physiol., 2010, 299, C189–C202 CrossRef CAS PubMed.
- S. M. Keyse, Cancer Metastasis Rev., 2008, 27, 253–261 CrossRef CAS PubMed.
- K. I. Patterson, T. Brummer, P. M. O'Brien and R. J. Daly, Biochem. J., 2009, 418, 475–489 CrossRef CAS PubMed.
- E. A. Craig, M. V. Stevens, R. R. Vaillancourt and T. D. Camenisch, Dev. Dyn., 2008, 237, 3102–3114 CrossRef CAS PubMed.
- A. von Kriegsheim, A. Pitt, G. J. Grindlay, W. Kolch and A. S. Dhillon, Nat. Cell Biol., 2006, 8, 1011–1016 CrossRef CAS PubMed.
- J. Pencik, H. T. Pham, J. Schmoellerl, T. Javaheri, M. Schlederer, Z. Culig, O. Merkel, R. Moriggl, F. Grebien and L. Kenner, Cytokine, 2016, 87, 26–36 CrossRef CAS PubMed.
- T. Abbas and A. Dutta, Nat. Rev. Cancer, 2009, 9, 400–414 CrossRef CAS PubMed.
- J. W. Harper, G. R. Adami, N. Wei, K. Keyomarsi and S. J. Elledge, Cell, 1993, 75, 805–816 CrossRef CAS PubMed.
- P. Bellosta, T. Hulf, S. Balla Diop, F. Usseglio, J. Pradel, D. Aragnol and P. Gallant, Proc. Natl. Acad. Sci. U. S. A., 2005, 102, 11799–11804 CrossRef CAS PubMed.
- Y. Xiong, J. Menninger, D. Beach and D. C. Ward, Genomics, 1992, 13, 575–584 CrossRef CAS PubMed.
- K. J. Sweeney, B. Sarcevic, R. L. Sutherland and E. A. Musgrove, Oncogene, 1997, 14, 1329–1340 CrossRef CAS PubMed.
- N. Jena, M. Deng, E. Sicinska, P. Sicinski and G. Q. Daley, Cancer Res., 2002, 62, 535–541 CAS.
- R. Rupaimoole, G. A. Calin, G. Lopez-Berestein and A. K. Sood, Cancer Discovery, 2016, 6, 235–246 CrossRef CAS PubMed.
- C. Blick, A. Ramachandran, R. McCormick, S. Wigfield, D. Cranston, J. Catto and A. L. Harris, Br. J. Cancer, 2015, 113, 634–644 CrossRef CAS PubMed.
- M. Winther, J. Alsner, B. S. Sorensen, C. F. Wittrup, T. Tramm, L. Baeksgaard, K. Hofland, E. Holtved and M. Nordsmark, Anticancer Res., 2016, 36, 721–730 CAS.
- T. Rosenberg, M. Thomassen, S. S. Jensen, M. J. Larsen, K. P. Sorensen, S. K. Hermansen, T. A. Kruse and B. W. Kristensen, CNS Oncol., 2015, 4, 25–35 CrossRef CAS PubMed.
- W. Yang, T. Sun, J. Cao, F. Liu, Y. Tian and W. Zhu, Exp. Cell Res., 2012, 318, 944–954 CrossRef CAS PubMed.
- W. Lei, C. Yan, J. Ya, D. Yong, B. Yujun and L. Kai, BMC Cancer, 2018, 18, 631 CrossRef PubMed.
- A. L. Zygulska, K. Krzemieniecki and P. Pierzchalski, J. Physiol. Pharmacol., 2017, 68, 311–335 CAS.
- Z. Meng, T. Moroishi and K. L. Guan, Genes Dev., 2016, 30, 1–17 CrossRef CAS PubMed.
- G. Gogl, K. D. Schneider, B. J. Yeh, N. Alam, A. N. Nguyen Ba, A. M. Moses, C. Hetenyi, A. Remenyi and E. L. Weiss, PLoS Biol., 2015, 13, e1002146 CrossRef PubMed.
- K. Saito-Diaz, T. W. Chen, X. Wang, C. A. Thorne, H. A. Wallace, A. Page-McCaw and E. Lee, Growth Factors, 2013, 31, 1–31 CrossRef CAS PubMed.
- Z. Chen, T. B. Gibson, F. Robinson, L. Silvestro, G. Pearson, B. Xu, A. Wright, C. Vanderbilt and M. H. Cobb, Chem. Rev., 2001, 101, 2449–2476 CrossRef CAS PubMed.
- M. Ashburner, C. A. Ball, J. A. Blake, D. Botstein, H. Butler, J. M. Cherry, A. P. Davis, K. Dolinski, S. S. Dwight, J. T. Eppig, M. A. Harris, D. P. Hill, L. Issel-Tarver, A. Kasarskis, S. Lewis, J. C. Matese, J. E. Richardson, M. Ringwald, G. M. Rubin and G. Sherlock, Nat. Genet., 2000, 25, 25–29 CrossRef CAS PubMed.
- M. Kanehisa, S. Goto, S. Kawashima, Y. Okuno and M. Hattori, Nucleic Acids Res., 2004, 32, D277–D280 CrossRef CAS PubMed.
Footnote |
† Electronic supplementary information (ESI) available. See DOI: 10.1039/c9ra01523j |
|
This journal is © The Royal Society of Chemistry 2019 |