DOI:
10.1039/C9RA01095E
(Paper)
RSC Adv., 2019,
9, 15949-15956
Ligand discrimination during virtual screening of the CB1 cannabinoid receptor crystal structures following cross-docking and microsecond molecular dynamics simulations†
Received
12th February 2019
, Accepted 16th May 2019
First published on 21st May 2019
Abstract
The therapeutic potential of the CB1 cannabinoid receptor remains underexploited with only a few synthetic ligands on the market. The crystal structures of both the inactive and active-state CB1 receptor have recently been solved, allowing for unprecedented opportunities in structure-based drug discovery applications such as virtual screening. In this study, we have investigated the virtual screening performance of the active and inactive-state CB1 crystal structures and their ability to discriminate between agonist and inverse agonist/antagonist ligands. The ligands of inactive and active-state CB1 receptor crystal structures were then swapped via cross-docking and the resulting structures were subjected to microsecond molecular dynamics (MD) simulations, followed by virtual screening of the MD-extracted structures. The original crystal structures were found to be biased towards ligands matching their activation state during virtual screening. MD simulations of the cross-docked CB1 structures resulted in a minor shift of receptor conformation towards the inactive state for the active-state CB1 structure complexed with the inverse agonist taranabant. Effects on virtual screening were more pronounced, as MD simulations of the cross-docked receptor–ligand complexes reversed the ligand bias in virtual screening observed with the original crystal structures. The simulations also produced receptor conformations that outperformed the crystal structures in virtual screening and in predicting the binding pose of the cognate ligand. The findings of this study highlight the potential of cross-docking and MD simulations to reverse the ligand bias of crystal structures, which may be useful when the crystal structure of only one activation state is available.
1. Introduction
Cannabis, also commonly known as marijuana, has been well-known for both its therapeutic and recreational uses for hundreds of years. The pharmacological properties of cannabis are mediated primarily by the action of Δ9-tetrahydrocannabinol (Δ9-THC) on the endocannabinoid system, which itself comprises two closely-related cannabinoid receptors: CB1 and CB2, which belong to the G protein-coupled receptor (GPCR) superfamily.1,2 The CB1 receptor is distributed primarily in the central nervous system and is the most abundant GPCR in the brain but can also be found in peripheral tissues, while the CB2 receptor is found primarily on immune cells.3 Due to its role in multiple physiological processes, the CB1 cannabinoid receptor represents a promising therapeutic target, and several cannabinoid-based therapies have received marketing approval for conditions such as spasticity in multiple sclerosis, chemotherapy-induced nausea and vomiting, and certain types of epilepsy.4 These currently-approved therapies are primarily based on the “classical” cannabinoid structure similar to the agonist Δ9-THC, and while other scaffolds of cannabinoid receptor-active compounds have been identified, none have been able to achieve clinical success thus far.5 This has in part led to the continuing debate around the legalization of medical marijuana which continues to gain traction in several countries despite courting significant controversy.6 It is not only CB1 agonists that are of clinical value; CB1 antagonists such as rimonabant have also been previously used for the treatment of obesity but unfortunately its marketing approval was withdrawn due to concerns over psychiatric adverse events.7 The current state of cannabinoid receptor-based therapies demonstrate that there is still a need for the discovery of novel synthetic ligands targeting the CB1 receptor which can exploit its therapeutic potential and minimise adverse events, without the controversy associated with cannabis.
For many years, structure-based drug discovery efforts targeting the CB1 receptor were hampered by the lack of an experimentally-determined crystal structure due to the well-known technical difficulties involved in producing diffraction-quality crystals of GPCRs for X-ray crystallography.8 As such, researchers frequently utilized CB1 receptor homology models constructed based on the limited GPCR crystal structures available for studying structure–function relationships, activation mechanisms, predicting ligand binding, and for drug discovery applications such as virtual screening.9–15 However, the performance of homology models has been shown to be inferior to crystal structures in drug discovery applications such as predicting the binding poses of ligands and in virtual screening.16,17 Following several recent breakthroughs in GPCR crystallography, the crystal structure of the inactive-state CB1 receptor bound to the inverse agonist taranabant and antagonist AM6538 was finally solved in late 2016.18,19 This was followed in 2017 by the crystal structure of the active-state CB1 receptor bound to the agonists AM11542 and AM841.20
From a structure-based drug design perspective, the resolution of these crystal structures allows for the unprecedented opportunity to study CB1 receptor structure–activity relationships at a high level of accuracy that was previously unachievable. Similarly, these crystal structures also allow for the application of other computational methods such as virtual screening and molecular dynamics simulations in order to study conformational changes induced by ligand binding. The availability of both CB1 receptor activation states also enables several intriguing questions to be answered. Specifically, we were interested in investigating the ability of the different CB1 crystal structures' activation states to discriminate between ligands with different functional effects in virtual screening. We were also interested in studying the effect of agonist binding on the inactive-state crystal structure and vice versa following unbiased molecular dynamics simulations. To this end, we have in this study investigated the virtual screening performance of the CB1 receptor crystal structures using agonist and inverse agonist/antagonist datasets. We then swapped the agonist and inverse agonist ligands of the active and inactive-state CB1 receptor crystal structures and performed microsecond molecular dynamics simulations on the resulting structures, subsequently extracting multiple receptor conformations and assessing virtual screening performance at each conformation, in order to study the effect of ligand binding on receptor conformation and ligand discrimination.
2. Methods
2.1 Ligand and decoy set selection
A diverse set of 50 agonist and 50 inverse agonist/antagonist ligands with Ki values <100 nM were selected from the ChEMBL database (Table S1†).21 Agonist and inverse agonist/antagonist classification was based on functional assay data from the ChEMBL database. A total of 50 matching decoys per active ligand was then selected using the Directory of Useful Decoys-Enhanced,22 giving a total of 2500 decoy molecules. Ligands were then prepared for docking using LigPrep,23 with appropriate protonation states at pH 7.0 assigned using Epik.24 For brevity, the inverse agonist/antagonist dataset is hereinafter referred to simply as the antagonist dataset.
2.2 Cross docking of crystal structure ligands
The crystal structures of inactive-state (PDB 5U09) and active-state (PDB 5XRA) CB1 receptor were obtained from the Protein Data Bank. The bound ligands (taranabant in inactive-state, AM11542 in active-state) were removed. The receptors were then prepared using the Protein Preparation workflow implemented in Schrodinger's Maestro.25 Non-ligand small molecules and crystallographic waters were removed. The stabilizing fusion proteins that replaced ICL3 in the crystal structures were removed, mutant residues were reverted back to wild-type, and missing side chain residues were modelled using Prime.26 Appropriate protonation and tautomeric states were assigned using Epik,24 hydrogen bond networks were optimized, and the resulting structure was energy minimized. The ligands were then cross-docked into the opposing-state structure (i.e. taranabant docked into active-state, AM11542 docked into inactive-state) using Glide with Schrodinger's Induced Fit Docking (IFD) protocol.27 The docking pose with lowest RMSD relative to the crystallographic ligand following optimal alignment of the receptor transmembrane helices was then selected for molecular dynamics simulations. The definition of the transmembrane regions were obtained from GPCRdb.28 Hereinafter, the inactive-state CB1 receptor with docked agonist AM11542 is referred to as CB1-AM11542, whereas the active-state CB1 receptor with docked inverse agonist taranabant is referred to as CB1*-taranabant.
2.3 Molecular dynamics simulations
All molecular dynamics simulations were conducted using GROMACS 201829 with the Amber ff99SB-ILDN* forcefield, supplemented with Slipids parameters for lipids and the General Amber Force Field (GAFF) for ligand parameters.30–32 Ligand topologies were generated using Acpype with partial charges calculated using the AM1-BCC method.33 Both receptor–ligand complexes were the embedded into a pre-equilibrated POPC membrane bilayer following alignment according to the Orientations of Proteins in Membrane database.34 The system was solvated with TIP3P water and 0.15 M NaCl was added to neutralize the system before energy minimization. Equilibration simulations were conducted for 100 ns using the NPT ensemble at 300 K using the Berendsen thermostat and 1 atm using a semi-isotropic Parinello–Rahman barostat, with position restraints using a force constant of 1000 kJ mol−1 nm−2 applied on the receptor–ligand complex. A time step of 2 fs was used and all bonds involving hydrogen atoms were constrained using the LINCS algorithm.35 Cutoffs of 10 Å were applied for short-range van der Waals and electrostatic interactions, while long-range electrostatic interactions were calculated using particle mesh Ewald.36 Following equilibration, unrestrained production simulations were then run at 300 K and 1 atm with the Nose Hoover thermostat and Parinello–Rahman barostat. Production simulations were run for 1 μs using three independent replicates for both receptor–ligand complexes, giving a total 6 μs of simulation time. RMSD values for the CB1 receptor relative to both the original active and inactive-state crystal structures were calculated following optimal superimposition of the transmembrane helices' backbone, whereas ligand RMSD was calculated relative to their respective crystal structures (5U09 for taranabant, 5XRA for AM11542) following the same superimposition procedure. Binding pocket volumes were calculated using SiteMap.37
2.4 Virtual screening
Receptor conformations were extracted from MD simulations every 50 ns for virtual screening. As IFD has been shown to improve virtual screening results,16 side chain conformation was optimized using IFD with the relevant ligand prior to each virtual screening (taranabant for antagonist dataset, AM11542 for agonist dataset), with the exception of the crystal structures. The receptor–ligand pose obtained from IFD with the lowest ligand RMSD relative to the original crystal structure was then selected for virtual screening. Virtual screening was then conducted using Glide with the SP scoring function with default settings.38 Grids were centered on the receptor orthosteric binding site as defined in the crystal structures. The top docking pose for each ligand was then ranked according to their docking scores. Virtual screening performance was assessed using the area under the curve (AUC) of receiver operating characteristic (ROC) curves. We report these values as adjusted logAUC,39 which includes an additional semilog transformation on the x-axis to provide further weight to early enrichment, followed by subtraction of the area of the random curve (equivalent to 14.46%). Using adjusted logAUC, random enrichment is therefore 0%, while positive values indicate a performance better than random selection and negative values indicate a performance that is worse than random selection.
3. Results and discussion
3.1 Receptor conformation and ligand binding
We were able to reproduce the binding pose of the agonist AM11542 using the inactive-state CB1 crystal structure to a relatively similar degree (RMSD 2.74 Å) using IFD (Fig. 1A). The orientation of the ligand was accurate, with only a slight displacement in the position of the tricyclic terpenoid ring and alkyl chain. In contrast, the lowest RMSD obtained via IFD of taranabant to the active-state CB1 receptor was 6.68 Å (Fig. 1B) but despite this, the relative orientations of cyanophenyl, chlorophenyl, and trifluoromethylpyridine moieties of taranabant were found to be correct. The high RMSD value could partially be attributed to the size of the binding pocket in the active-state crystal structure (which is up to 53% smaller than the inactive-state), primarily due to the inward movement of TM1 and TM2, thus inhibiting proper positioning of taranabant in the putative access channel between TM1 and TM7 in the active-state crystal structure.
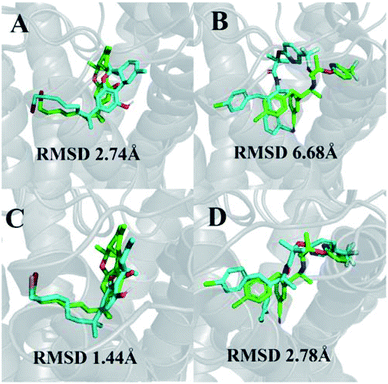 |
| Fig. 1 (A) Best pose of AM11542 following docking to the inactive-state CB1 crystal structure (PDB 5U09); (B) best pose of taranabant following docking to the active-state CB1 crystal structure (PDB 5XRA); (C) lowest RMSD pose of AM11542 following MD simulations with inactive-state CB1 crystal structure; (D) best pose of taranabant following docking to structures extracted from MD simulations. The crystal structure ligand poses are shown in green, while ligand poses from docking and MD simulations are shown in cyan. RMSD values were calculated relative to the crystal structure ligand following optimal superimposition of the receptor transmembrane backbone. | |
The receptor transmembrane backbone RMSD in both sets of simulations and relative to both crystal structures following microsecond molecular dynamics simulations are shown in Fig. 2. The CB1-AM11542 simulations showed that the RMSD values of all three replicates increased relative to both crystal structures, indicating the receptor was exploring conformations that were dissimilar to both activation states. The CB1*-taranabant simulations similarly showed that transmembrane backbone RMSD deviated from the original active-state crystal structure, as expected, with two replicates showing a significant increase in RMSD towards the end of the simulation. In tandem, these two replicates also showed reductions in their RMSD relative to the inactive-state crystal structure, with replicate 2 achieving an average RMSD of <3.0 Å following a transition period between 450 ns to 700 ns, indicating the receptor adopted a conformation more similar to the inactive-state following molecular dynamics simulations with a bound inverse agonist; this was despite the high initial RMSD values observed following cross-docking of taranabant. Analysis of the individual helix RMSDs in comparison with original active-state structure showed that in replicate 2, this transition was due to slight inward movement of the intracellular end of TM6 (which also showed the most movement in all replicates), while in replicate 3, the receptor demonstrated an outward movement of the extracellular portion of TM1 towards the end of the simulation (Fig. 3 and S1†). Both observations were consistent with the key differences in TM bundle arrangement that were observed when comparing the original inactive and active state crystal structures,18,20 and therefore support a transition from active to inactive state. While the reduction in RMSD relative to the opposing activation state here was minor, these observations highlight the potential ability of unbiased molecular dynamics simulations to sample and study receptor activation or inactivation via cross docking of the relevant ligand, which may be useful in scenarios where the crystal structure of only one activation state is available. In order to investigate if the RMSD relative to the inactive state could be further reduced, we extended the simulation for replicate 2, continuing the simulation for 1 μs and also generating two new replicates using the final conformation, which were then run for 1 μs each using new velocities. The transmembrane backbone RMSD remained fairly constant throughout these extended simulations and showed no further reduction, indicating that a potential local minimum in the potential energy landscape may have been reached.
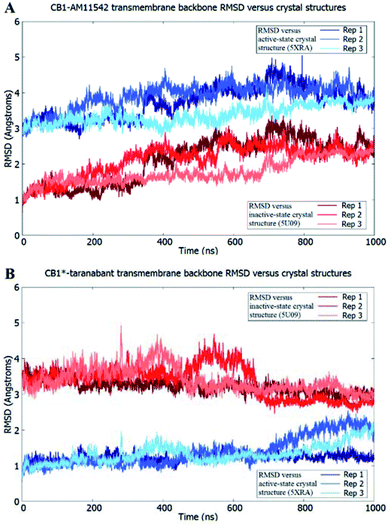 |
| Fig. 2 Receptor transmembrane backbone RMSD of (A) CB1-AM11542 and (B) CB1*-taranabant following 1 μs of molecular dynamics simulations relative to the CB1 crystal structures. RMSD values relative to the inactive-state crystal structure (PDB 5U09) are shown in shades of red, while RMSD values relative to the active-state crystal structure (PDB 5XRA) are shown in shades of blue. | |
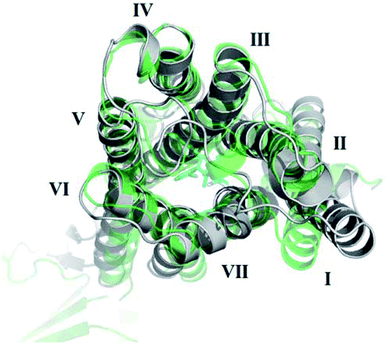 |
| Fig. 3 Outward movement of TM1 of the active-state CB1 cannabinoid receptor following MD simulations with the inverse agonist taranabant. The conformation of the original active-state crystal structure is shown in green, while the final conformation following MD simulations is shown in grey. | |
3.2 Improvements in ligand binding RMSD following MD simulations
In terms of ligand binding, the RMSD of AM11542 and taranabant remained relatively stable throughout all simulations, with fluctuations in the range of 2 Å observed. However, certain conformations of AM11542 sampled showed significant improvement over the initial docked pose, reaching a minimum RMSD of 1.44 Å and essentially replicating the crystallographic binding pose (Fig. 1C). The RMSD of taranabant remained similarly stable throughout all simulations. The minimum RMSD achieved was 5.76 Å, which, while lower than the docked pose, remained relatively inaccurate compared to the crystallographic pose.
Structures extracted from the MD simulations were subjected to IFD with AM11542 and taranabant prior to virtual screening (RMSD values shown in Table S2†). For the CB1-AM11542 simulations, the majority of IFD poses with AM11542 resulted in RMSD values that were higher compared to initial cross-docking (2.74 Å), with the lowest RMSD following IFD obtained for AM11542 demonstrating a slight improvement at 2.43 Å. For IFD with taranabant, the minimum RMSD achieved was 2.78 Å, which was highly similar to the crystallographic pose (Fig. 1D). This was intriguing given that this was a significant improvement over redocking taranabant to the original inactive-state crystal structure, where the best RMSD obtained was 4.74 Å. Similarly, the CB1*-taranabant simulation produced structures that when subjected to IFD offered improvements over the original active-state crystal structure docking, but the differences were less pronounced. The minimum RMSD for AM11542 using simulation structures was 0.54 Å (compared to RMSD 0.63 Å in the active-state crystal structure), while the minimum RMSD for taranabant using simulation structures was 5.72 Å (compared to 6.68 Å using the active-state crystal structure). Nevertheless, these findings reinforce the precedent that molecular dynamics simulations can improve docking poses by either enhancing conformational sampling through the simulation or producing multiple receptor conformations for docking.40,41
3.3 Ligand discrimination in virtual screening
As expected, both original crystal structures showed virtual screening performance matching their activation states. The inactive-state crystal structure (5U09) demonstrated a logAUC of 7.8% for the antagonist dataset versus a logAUC of 5.9% for the agonist dataset. The active-state crystal structure (5XRA) showed a significantly stronger preference for agonists, with a logAUC of 20.5% for the agonist dataset compared to a logAUC 0.5% for the inverse antagonist dataset. Given that the size of the binding pocket in the active-state crystal structure is significantly smaller than the inactive-state,18–20 it was unsurprising that the level of ligand selectivity shown for the active-state receptor was higher while the inactive-state receptor appeared to be able to less selective and accommodated a more diverse range of ligands. The virtual screening performance of both receptor–ligand complexes using agonists and antagonist datasets following microsecond unbiased molecular dynamics simulations are shown in Fig. 4. For CB1-AM11542, the average logAUC across all three replicates was 0.9% ± 4.9% for antagonists and 12.3% ± 5.4% for agonists, with a maximum value of 14.5% for antagonists and 28.3% for agonists. For CB1*-taranabant, the average logAUC across all three replicates was −0.9% ± 3.7% for antagonists and 13.1% ± 5.1 for agonists, with a maximum value of 8.5% for antagonists and 28.6% for agonists. When considering the average logAUC values it is worth taking into consideration that each replicate was independent and therefore sampled different regions of phase space, as we confirmed using principal components analysis (Fig. S2†). Individual logAUC values showed significant variation, and there was no clear correlation with receptor transmembrane backbone RMSD values. Similarly, there was no clear trend between ligand RMSD following IFD and virtual screening performance (i.e. MD structures that best reproduced the crystallographic ligand pose following IFD did not necessarily perform best in virtual screening), a finding similar to that reported with other GPCRs.16 The ability of the different conformations of the CB1 receptor to discriminate between different ligand classes has also recently been shown using three individual ligands and MM/PBSA calculations,42 but the results of this study clearly indicate that the propensity for this bias extends across various cannabinoid ligands and to virtual screening applications. The virtual screening performance of both receptor–ligand complexes following molecular dynamics observed here was intriguing for several reasons. In CB1-AM11542 we observed a clear shift of preference for antagonists to agonists in virtual screening compared to the original inactive-state crystal structure. Starting from a slight preference towards antagonists (logAUC of 7.8% for agonists vs. 5.9% for antagonists) in the crystal structure, the average logAUC across all MD structures was higher for agonists compared to antagonists. 90.5% of the MD structures showed improved performance for agonists, while performance for antagonists decreased relative to the crystal structure in 92.1% of the MD structures. In CB1*-taranabant this shift was less pronounced as the average logAUC was still higher for the agonist dataset in absolute terms, although we noted a significant reduction in average logAUC for agonists compared to the crystal structure, while the average logAUC for antagonists remained little changed. At the individual level however, the majority of the MD structures (93.7%) showed a decrease in logAUC for agonists compared to crystal structure, whereas 28.4% of MD structures showed improved performance compared to the crystal structure for the antagonist dataset. Additionally, it was noteworthy that both simulations produced receptor conformations that outperformed the crystal structures for both agonist and antagonist datasets, regardless of the ligand bound and time of simulation. One potential explanation for this observation is that notwithstanding the limitations of crystallographic resolution, the crystal structures themselves represent a receptor conformation that is primarily favorable for binding of the single co-crystallized ligand. Given the dynamic nature of receptor–ligand binding and that different classes of ligands may have correspondingly distinct bound receptor conformations,43 structures produced from MD ensembles therefore produce receptor conformations that are overall more favorable for binding a more diverse group of ligands, and therefore perform better in typical virtual screening exercises which employ a diverse range of actives.
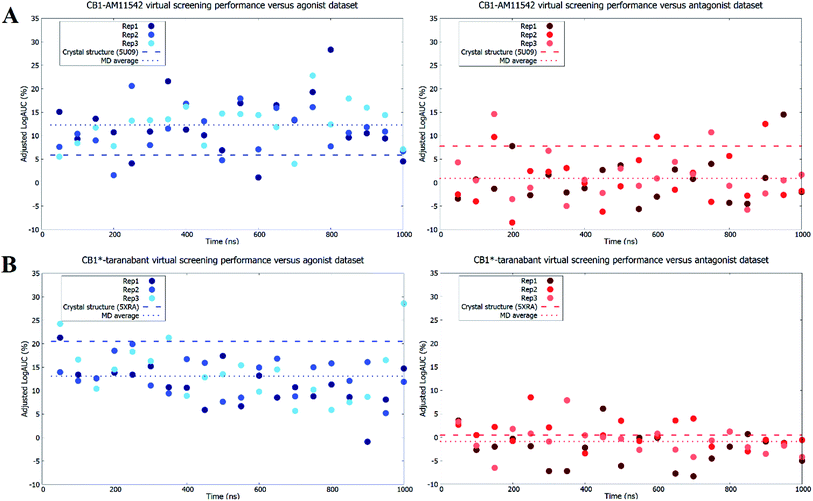 |
| Fig. 4 Virtual screening performance throughout molecular dynamics simulations of (A) CB1-AM11542 structures and (B) CB1*-taranabant structures using agonist and inverse agonist/antagonist datasets. Adjusted logAUC values using agonist datasets are shown as blue dots, while values using inverse agonist/antagonist datasets are shown as red dots. The virtual screening performance of the original crystal structures and the average across all replicates are shown as dashed lines. | |
Finally, when we repeated the virtual screenings but without the IFD step prior to virtual screening we observed similar trends, although the average and individual adjusted logAUC values were lower. The exception to this was the average adjusted logAUC value for the agonist dataset in CB1-AM11542, which was slightly higher (Table S3†). In general, this further reinforces previous findings that IFD improves absolute virtual screening performance.16 Likewise, the same trends were also observed when we attempted to investigate the ability of the models to discriminate between agonist and antagonists ligands directly. This was achieved by removing the decoys and considering only the opposing ligand class as inactives during virtual screening (e.g. when screening for agonist ligands only the antagonist ligands were used as inactives and vice versa). In this case, despite the fact that the “inactives” were actually known binders, the crystal structures retained their respective biases for ligands matching their functional state. We also observed the same reversal in ligand bias following molecular dynamics simulations, and similarly to the full virtual screening dataset some individual models outperformed the crystal structures (Table S4 and Fig. S3†). The degree of reversal seen for the antagonist dataset in the CB1*-taranabant simulations was also relatively minor and remained negative in terms of average adjusted logAUC, mirroring the performance observed when using the full dataset with decoys. These observations therefore further substantiate our findings with the original virtual screening datasets.
The primary caveat to the observations here was that the initial crystal structures already showed some preference towards agonist ligands, which was possibly due to the differences in size of the binding pocket in their respective activation states. When we calculated the size of the ligand binding pocket for each IFD-optimized structure used in virtual screening, correlations between binding pocket volume and adjusted logAUC values were generally poor for the antagonist datasets. In contrast, for the agonist datasets we noted an inverse correlation in most replicates between binding pocket volume and logAUC (r values ranging from −0.53 to −0.14), further suggesting that the binding pocket volume may be a primary contributing factor to the virtual screening bias seen in the active-state crystal structure. Overall, the change in binding pocket volumes were consistent with the bound ligand size (i.e. binding pocket volume reduced for CB1-AM11542 and increased for CB1*-taranabant when compared to the initial crystal structures) but fluctuated consistently in the range of 400–700 Å3.
In summary, these findings highlight the ability of molecular dynamics simulations to not only improve the virtual screening performance of crystal structures as has been previously demonstrated,44 but also to potentially reverse the preference for agonist and inverse agonist/antagonist ligands. Given the technical difficulties involved in membrane protein crystallography, molecular dynamics may be useful in scenarios where only a single activation state crystal structure is available but does not match the desired ligands' functional activity.
4. Conclusion
The therapeutic potential of the CB1 cannabinoid receptor remains underexploited due to the controversy associated with medical marijuana use and adverse effects associated with previously marketed CB1 receptor ligands. With the release of both inactive and active-state crystal structures of the CB1 receptor, structure-based drug discovery applications such as virtual screening involving the CB1 receptor is likely to increase in an effort to discover novel synthetic ligands with potential medicinal properties. In this study we have investigated the virtual screening performance of the inactive and active-state CB1 crystal structures and their ability to discriminate between agonist and inverse agonist/antagonist ligands. We then swapped the ligands of inactive and active-state CB1 receptor crystal structures and subjected the resulting complexes to microsecond molecular dynamics simulations, followed by virtual screening using agonist and inverse agonist/antagonist ligand datasets. Our results indicate that the crystal structures are biased towards ligands matching their activation state, but with the bias for agonist ligands being significantly more prominent in the active-state CB1 structure. Swapping the ligands via cross-docking and running unbiased microsecond molecular dynamics simulations had only minor effects in shifting receptor conformation towards the opposing activation state, but its effect on virtual screening performance was more pronounced. We observed a shift in ligand bias during virtual screening for both receptor–ligand complexes when compared to their respective crystal structures. The MD simulations also produced receptor conformations that outperformed the original crystal structures in virtual screening and in predicting the binding pose of the cognate ligand. The findings reported here may therefore serve to inform prospective virtual screenings and other structure-based drug design applications targeting the CB1 receptor and GPCRs in general.
Conflicts of interest
There are no conflicts to declare.
Acknowledgements
This study received no specific funding.
References
- A. C. Howlett, C. S. Breivogel, S. R. Childers, S. A. Deadwyler, R. E. Hampson and L. J. Porrino, Neuropharmacology, 2004, 47, 345–358 CrossRef CAS PubMed.
- R. Fredriksson, M. C. Lagerstrom, L. G. Lundin and H. B. Schioth, Mol. Pharmacol., 2003, 63, 1256–1272 CrossRef CAS PubMed.
- R. G. Pertwee, A. C. Howlett, M. E. Abood, S. P. H. Alexander, V. Di Marzo, M. R. Elphick, P. J. Greasley, H. S. Hansen, G. Kunos, K. Mackie, R. Mechoulam and R. A. Ross, Pharmacol. Rev., 2010, 62, 588–631 CrossRef CAS PubMed.
- P. J. Robson, Drug Test. Anal., 2014, 6, 24–30 CrossRef CAS PubMed.
- R. G. Pertwee, Br. J. Pharmacol., 2009, 156, 397–411 CrossRef CAS PubMed.
- J. M. Bostwick, Mayo Clin. Proc., 2012, 87, 172–186 CrossRef PubMed.
- European Medicines Agency. Doc. Ref. EMEA/537153/2008 available from: https://www.ema.europa.eu/documents/medicine-qa/questions-answers-recommendation-suspend-marketing-authorisation-acomplia-rimonabant_en.pdf, accessed 13 January 2019.
- D. M. Rosenbaum, S. G. F. Rasmussen and B. K. Kobilka, Nature, 2009, 459, 356–363 CrossRef CAS PubMed.
- C. Montero, N. E. Campillo, P. Goya and J. A. Páez, Eur. J. Med. Chem., 2005, 40, 75–83 CrossRef CAS PubMed.
- T. Tuccinardi, P. L. Ferrarini, C. Manera, G. Ortore, G. Saccomanni and A. Martinelli, J. Med. Chem., 2006, 49, 984–994 CrossRef CAS PubMed.
- A. Gonzalez, L. S. Duran, R. Araya-Secchi, J. A. Garate, C. D. Pessoa-Mahana, C. F. Lagos and T. Perez-Acle, Bioorg. Med. Chem., 2008, 16, 4378–4389 CrossRef CAS PubMed.
- R. Singh, D. P. Hurst, J. Barnett-Norris, D. L. Lynch, P. H. Reggio and F. Guarnieri, J. Pept. Res., 2002, 60, 357–370 CrossRef CAS.
- R. Ai and C. A. Chang, J. Mol. Graph. Model., 2012, 38, 155–164 CrossRef CAS PubMed.
- S. D. McAllister, G. Rizvi, S. Anavi-Goffer, D. P. Hurst, J. Barnett-Norris, D. L. Lynch, P. H. Reggio and M. E. Abood, J. Med. Chem., 2003, 46, 5139–5152 CrossRef CAS PubMed.
- D. Latek, M. Kolinski, U. Ghoshdastider, A. Debinski, R. Bombolewski, A. Plazinska, K. Jozwiak and S. Filipek, J. Mol. Model., 2011, 17, 2353–2366 CrossRef CAS PubMed.
- J. S. E. Loo, A. L. Emtage, K. W. Ng, A. S. J. Yong and S. W. Doughty, J. Mol. Graph. Model., 2018, 80, 38–47 CrossRef CAS PubMed.
- T. Beuming and W. Sherman, J. Chem. Inf. Model., 2012, 52, 3263–3277 CrossRef CAS PubMed.
- Z. Shao, J. Yin, K. Chapman, M. Grzemska, L. Clark, J. Wang and D. M. Rosenbaum, Nature, 2016, 540, 602–606 CrossRef CAS PubMed.
- T. Hua, K. Vemuri, M. Pu, L. Qu, G. W. Han, Y. Wu, S. Zhao, W. Shui, S. Li, A. Korde, R. B. Laprairie, E. L. Stahl, J. H. Ho, N. Zvonok, H. Zhou, I. Kufareva, B. Wu, Q. Zhao, M. A. Hanson, L. M. Bohn, A. Makriyannis, R. C. Stevens and Z. J. Liu, Cell, 2016, 167, 750–762 CrossRef CAS PubMed.
- T. Hua, K. Vemuri, S. P. Nikas, R. B. Laprairie, Y. Wu, L. Qu, M. Pu, A. Korde, S. Jiang, J. H. Ho, G. W. Han, K. Ding, X. Li, H. Liu, M. A. Hanson, S. Zhao, L. M. Bohn, A. Makriyannis, R. C. Stevens and Z. J. Liu, Nature, 2017, 547, 468–471 CrossRef CAS PubMed.
- A. Gaulton, A. Hersey, M. L. Nowotka, A. Patricia Bento, J. Chambers, D. Mendez, P. Mutowo, F. Atkinson, L. J. Bellis, E. Cibrian-Uhalte, M. Davies, N. Dedman, A. Karlsson, M. P. Magarinos, J. P. Overington, G. Papadatos, I. Smit and A. R. Leach, Nucleic Acids Res., 2017, 45, D945–D954 CrossRef CAS PubMed.
- M. M. Mysinger, M. Carchia, J. J. Irwin and B. K. Shoichet, J. Med. Chem., 2012, 55, 6582–6594 CrossRef CAS PubMed.
- LigPrep, Schrödinger, LLC, New York, 2018 Search PubMed.
- J. C. Shelley, A. Cholleti, L. L. Frye, J. R. Greenwood, M. R. Timlin and M. Uchimaya, J. Comput. Aided Mol. Des., 2007, 21, 681–691 CrossRef CAS PubMed.
- Maestro, Schrödinger, LLC, New York, 2016 Search PubMed.
- M. P. Jacobson, D. L. Pincus, C. S. Rapp, T. J. F. Day, B. Honig, D. E. Shaw and R. A. Friesner, Proteins Struct. Funct. Genet., 2004, 55, 351–367 CrossRef CAS PubMed.
- W. Sherman, T. Day, M. P. Jacobson, R. A. Friesner and R. Farid, J. Med. Chem., 2006, 49, 534–553 CrossRef CAS PubMed.
- C. Munk, V. Isberg, S. Mordalski, K. Harpsøe, K. Rataj, A. S. Hauser, P. Kolb, A. J. Bojarski, G. Vriend and D. E. Gloriam, Br. J. Pharmacol., 2016, 16, 2195–2207 CrossRef PubMed.
- M. J. Abraham, T. Murtola, R. Schulz, S. Páll, J. C. Smith, B. Hess and E. Lindah, SoftwareX, 2015, 1–2, 19–25 CrossRef.
- K. Lindorff-Larsen, S. Piana, K. Palmo, P. Maragakis, J. L. Klepeis, R. O. Dror and D. E. Shaw, Proteins, 2010, 78, 1950–1958 CAS.
- J. P. M. Jämbeck and A. P. Lyubartsev, J. Phys. Chem. B, 2012, 116, 3164–3179 CrossRef PubMed.
- J. Wang, R. M. Wolf, J. W. Caldwell, P. A. Kollman and D. A. Case, J. Comput. Chem., 2004, 25, 1157–1174 CrossRef CAS PubMed.
- A. W. Sousa Da Silva and W. F. Vranken, BMC Res. Notes, 2012, 5, 367 CrossRef PubMed.
- M. A. Lomize, I. D. Pogozheva, H. Joo, H. I. Mosberg and A. L. Lomize, Nucleic Acids Res., 2012, 40, D370–D376 CrossRef CAS PubMed.
- B. Hess, H. Bekker, H. J. C. Berendsen and J. J. G. E. M. Fraaije, J. Comput. Chem., 1997, 18, 1463–1472 CrossRef CAS.
- U. Essmann, L. Perera, M. L. Berkowitz, T. Darden, H. Lee and L. G. Pedersen, J. Chem. Phys., 1995, 103, 8577–8593 CrossRef CAS.
- T. A. Halgren, J. Chem. Inf. Model., 2009, 43, 377–389 CrossRef PubMed.
- R. a. Friesner, J. L. Banks, R. B. Murphy, T. a Halgren, J. J. Klicic, D. T. Mainz, M. P. Repasky, E. H. Knoll, M. Shelley, J. K. Perry, D. E. Shaw, P. Francis and P. S. Shenkin, J. Med. Chem., 2004, 47, 1739–1749 CrossRef CAS PubMed.
- M. M. Mysinger and B. K. Shoichet, J. Chem. Inf. Model., 2010, 50, 1561–1573 CrossRef CAS PubMed.
- R. E. Amaro, J. Baudry, J. Chodera, Ö. Demir, J. A. McCammon, Y. Miao and J. C. Smith, Biophys. J., 2018, 114, 1–8 CrossRef PubMed.
- R. E. Amaro, R. Baron and J. A. McCammon, J. Comput. Aided Mol. Des., 2008, 22, 693–705 CrossRef CAS PubMed.
- S. W. Jung, A. E. Cho and W. Yu, Sci. Rep., 2018, 8, 13787 CrossRef PubMed.
- S. Kumar, B. Ma, C. J. Tsai, N. Sinha and R. Nussinov, Protein Sci., 2010, 9, 10–19 CrossRef PubMed.
- F. Feixas, S. Lindert, W. Sinko and J. A. McCammon, Biophys. Chem., 2014, 186, 31–45 CrossRef CAS PubMed.
Footnote |
† Electronic supplementary information (ESI) available. See DOI: 10.1039/c9ra01095e |
|
This journal is © The Royal Society of Chemistry 2019 |
Click here to see how this site uses Cookies. View our privacy policy here.