DOI:
10.1039/C9RA00906J
(Paper)
RSC Adv., 2019,
9, 17950-17958
Identification of anti-inflammatory components of raw and steamed Panax notoginseng root by analyses of spectrum-effect relationship†
Received
2nd February 2019
, Accepted 28th May 2019
First published on 7th June 2019
Abstract
To identify bioactive components of Panax notoginseng (PN) roots in raw and steamed forms, chemometrics analyses including bivariate correlation analysis and partial least squares regression were used to establish the relationships between the chromatograms and anti-inflammatory effect of PN samples. The chemical fingerprints of PN were obtained by high performance liquid chromatography. The anti-inflammatory activity was evaluated by the TNF-α and IL-6 inhibiting test. According to the results, raw PN (RPN) displayed a stronger anti-inflammatory effect compared with steamed PN (SPN) samples. Chemometrics analyses showed that notoginsenoside R1, ginsenosides Rg1, Re and Rb1 were the major anti-inflammatory components of RPN, whereas ginsenosides 20(S)-Rg3 was the active one of SPN treating inflammation. The pharmacologic verification was consistent with the predicted results. In addition, the contents of anti-inflammatory components in RPN were higher than those in SPN, which might be the reason that RPN exhibited stronger effect on the inflammation induced by lipopolysaccharide in RAW264.7 cells. These findings could be beneficial for choosing differential markers for the quality control of RPN and SPN.
1. Introduction
Panax notoginseng (PN) Burk., a plant in genus Panax (Araliaceae), is a medicinal plant used in Asia for the treatment of trauma, body pain, inflammation and cardiovascular diseases since ancient times.1 Based on the US Dietary Supplement Health and Education Act of 1994,2 herbal medicines and food supplements, including PN root, were classified as dietary supplements. Clinically, there are two forms of PN, of which the raw PN (RPN) is used to promote blood circulation, remove blood stasis, reduce swelling, and treat trauma,1 whereas the steamed one is claimed to be a tonic used to “nourish” blood and to increase the production of various blood cells in anemic conditions.3 Both raw and steamed forms are readily available to the patients as Chinese Proprietary Medicines (CPMs) (finished products such as tablets, capsules, powder).4 Modern researches5,6 have validated such differentiated effects between RPN and steamed PN (SPN), which are closely related to the significant variation in their chemical compositions. Sun, S. et al.7 found that the steaming process could influence the transformation of Rg3 and the SPN extract could inhibit the proliferation of SW-480 human colorectal cancer cells significantly. In our previous studies,8–10 the saponin contents in PN changed significantly with the duration of steaming time. RPN showed stronger hemostasis and anticoagulation activities, while the SPN behaved stronger antioxidation and hematopoiesis effects. However, the raw materials and the steamed ones in the clinic use and market circulation are undifferentiated, or share the same marker components for their quality control (QC). For instance, in Chinese Pharmacopoeia of 2015 edition,11 the marker components for the QC of RPN are ginsenoside Rb1, Rg1, and notoginsenoside R1, which are consistent with QC markers for SPN by China Food and Drug Administration (2012).12 Such undifferentiated determination of marker components for RPN and SPN might bring uncertain curative effects or even adverse reactions in clinic.
Saponins are the major components of PN and considered to be the primary active ones, which exert different pharmacologic effects underlined by varied mechanisms. Fan, J. S. et al.13 reported that PN saponins could inhibit nuclear factor-kappa B (NF-κB) DNA binding activity and reduced secretion of interleukin 6 (IL-6) and macrophage chemoattractant protein 1 in lipopolysaccharide (LPS)-stimulated THP-1 macrophages. Wang, Y. et al.14 held that PN saponins might play an anti-inflammatory effect by inhibiting NF-κB activity and tumor necrosis factor-alpha (TNF-α) mRNA expression. In our previous studies,8 we found that both RPN and SPN showed certain degrees of anti-inflammatory effect on the xylene-induced ear edema model, between which RPN was stronger. Since there are significant difference in the chemical composition between the two forms of PN, we hypothesize that components related to their anti-inflammatory effects could be also different, which has not been identified to our knowledge.
“Spectrum-effect relationship” refers to linking the fingerprint peaks of medicinal materials with specific bioactivities to build a relationship, and then looking for the active components in medicines by analyzing the relationship.15 To overcome the shortcomings of chromatograms which only contain chemical characteristics but little pharmacologic information of components, the analyses of spectrum-effect relationships use data processing techniques such as partial least squares regression (PLSR), partial component regression, or stepwise multivariate linear regression to analyze the weight coefficients of components, monitoring the efficacy despite changes of the peaks and their areas in the fingerprint. Thus, active components related to different activities could be predicted in an effective way for further investigations.
In this study, the approach was used to identify the anti-inflammatory components of RPN and SPN. Since LPS was used to induce RAW264.7 cells inflammation model in a large number of researches about in vitro anti-inflammatory activity,16–20 the inhibition rates of TNF-α and IL-6 of PN during the steaming process were used as the evaluation indicators of the anti-inflammatory effect. Combined with the chemical analysis of RPN and SPN samples in our previous work, the spectrum-effect relationship of PN was constructed by multivariate regression analyses to predict components inhibiting the production of TNF-α and IL-6. Pharmacologic tests were finally performed to validate the predicted results.
2. Materials and methods
2.1. Cell and reagents
RAW264.7 cell was purchased from the Bogu Biotechnology Co., Ltd (Shanghai, China). Dulbecco's modified Eagle's medium (DMEM), fetal bovine serum (FBS), penicillin–streptomycin solution and trypsin were purchased from Biological Industries. LPS was purchased from Sigma-Aldrich, Inc. (St. Louis, MO, USA). TNF-α, IL-6 and MTS cell proliferation Kits were purchased from eBioscience company (USA). The reference standards of ginsenosides Rg1, Re, Rb1, 20(S)-Rg3, and notoginsenoside R1 were obtained from the National Institutes for the Control of Pharmaceutical and Biological Products (Beijing, China).
2.2. Sample preparation
RPN roots were purchased from Yunnan, China. SPN samples were prepared by steaming the crushed RPN in an autoclave (Shanghai, China) for 2, 4, 6, 8, and 10 h at 105, 110, and 120 °C, respectively. The steamed powder was dried to a constant weight in the heating-air drying oven at 45 °C, and then pulverized and sieved through a 40-mesh sieve. PN samples of 5 g, in the powdered form, were extracted with pure water of 50.0 mL by refluxing twice at 80 °C, 2 h for each extraction. The combined solution was filtered and concentrated under reduced pressure to 0.1 g mL−1 of PN. The extract was then diluted with the normal saline to different concentrations.9
2.3. HPLC analyses
The HPLC analyses were carried out according to the method in our previous research.8 “HPLC analyses were done on an Agilent 1260 series system (Agilent Technologies, Santa Clara, CA, USA) consisting of a G1311B Pump, a G1329B autosampler and a G4212B DAD detector. A Vision HT C18 column (250 mm × 4.6 mm, 5 μm) was adopted for the analyses. The mobile phase consisted of A (ultra pure water) and B (acetonitrile). The gradient mode was as follows: 0–20 min, 80% A; 20–45 min, 54% A; 45–55 min, 45% A; 55–60 min, 45% A; 60–65 min, 100% B; 65–70 min, 80% A; and 70–90 min, 80% A. The flow rate was set at 1.0 mL min−1. The detection wavelength was set at 203 nm. The column temperature was set at 30 °C and sample volume was set at 10 μL”.
2.4. Cells culture
The RAW264.7 cells were cultured with DMEM (high sugar) containing 10% FBS and 1% penicillin-streptomycin mixture solution at 37 °C, 5% CO2 and relative humidity of 90%.
2.5. Screening of induced concentration and time of LPS in inflammation model
2.5.1. Screening of optimal concentrations of LPS to induce TNF-α and IL-6. The cells were incubated in 24-well plates (2 × 106 mL−1) and cultured at 37 °C, 5% CO2 and relative humidity of 90%. Blank control wells were set up and the remaining wells were incubated with different concentrations of LPS (0, 0.25, 0.5, 1.0, and 2.0 μg mL−1) after culturing cells for 24 h. Each group was set up in triplicate. The culture medium was collected and the contents of TNF-α and IL-6 were determined by ELISA Kits after 48 h.
2.5.2. Screening of optimal time for LPS to induce TNF-α and IL-6. The cells were cultured in 24-well plates (2 × 106 mL−1) at 37 °C, 5% CO2 and relative humidity of 90%. Control wells were set up and the remaining wells were incubated for different time (0, 12, 24, 36 and 48 h) at the screened optimal concentration. Each group was set up in triplicate. The culture medium was collected and the contents of TNF-α and IL-6 were determined by ELISA Kits after 0, 12, 24, 36, and 48 h, respectively.
2.6. Effects of raw and steamed PN extracts and LPS on cell growth
The cells were cultured in 96-well plates (2 × 104 mL−1) at 37 °C, 5% CO2 and relative humidity of 90%. Control wells were set up and the remaining wells were incubated with different concentrations of PN extracts (0.625, 1.25, 2.50, 5.0, 10.0, 20.0, and 40.0 mg mL−1) and 2.0 μg mL−1 LPS after culturing cells for 24 h. Each group was set up in triplicate. Then the old medium was removed, with 100 μL fresh ones and 20 μL MTS solution added after culturing for 24 h. The absorbance at 490 nm was measured with a spectrophotometer after incubated for 1 h. The inhibition rate of cell growth was calculated according to the following equations: |
Cell growth inhibition rate (%) = (1 − ODdrug/ODcontrol) × 100%
| (1) |
2.7. Effects of PN extracts on the level of TNF-α and IL-6 by LPS
The cells were cultured in 24-well plates (2 × 106 mL−1, 500 μL per well) at 37 °C, 5% CO2 and relative humidity of 90%. After the adherent culture of 24 h, cells were treated with PN extracts of different concentrations (0–4.2 mg mL−1). After 1 h of incubation, LPS of the screened concentration was added in each well. The culture medium was collected after 24 h incubation for the content determination of TNF-α and IL-6 by ELISA kits.
2.8. Multivariate data analyses
2.8.1. BCA. BCA is a multivariate analysis used to describe the degree of closeness, correlation and direction of the linear relationship between two variables. The correlation coefficients are used to determine the relevance between two variables and reflect their predictive or explanatory power.21
2.8.2. PLSR. PLSR is used to find the intrinsic relationship between the independent variables (X) and dependent variables (Y), which are modeled simultaneously by considering the variance of X and the covariance between X and Y.22 In our case, the X matrix is composed of the enhanced fingerprints, and the Y vector is constructed with the reference values of anti-inflammatory effect obtained by measuring the levels of TNF-α and IL-6. Then, X and Y are decomposed in a product of another two matrices of scores and loadings; as described by the following equations:where TPT proximate to the chromatographic data and UQT to the true Y values; notice that the relationship between T and U scores is a summary of the relationship between X and Y. The terms E and F from the equations are error matrices. Thus, the PLS algorithm tries to find potential variables related to the prediction Y to maximize the variation explained in X; i.e., to capture variance and achieve correlation.23
2.9. Pharmacologic test
The predicted components/peaks related to the anti-inflammatory activity of PN were identified by reference standards. Then the anti-inflammatory activity of components with different concentrations (2.5, 5.0, 10, 20, and 40 μmol L−1) was determined using the method described in “Section 2.7”.
2.10. Statistical analyses
All data were expressed as means ± SD. SPSS 21.0 software (Statistical Program for Social Sciences, SPSS inc, Chicago, IL, USA) was applied to carry out the two-tailed unpaired t-test. The IC50 value was fitted by probit regression with the GraphPad Prism 7 (GraphPad Software, USA) for Windows. Umetrics SIMCA-P 11.5 software (Sartorius Stedim Biotech, Sweden) was applied for PLSR analysis. DPS 9.50 software (Data Processing System, China) was used for MLRA. A value of P < 0.05 was considered to be significant difference. A value of P < 0.01 was considered to be highly significant difference.
3. Results and discussion
3.1. HPLC analyses
HPLC fingerprints for 18 batches of PN samples were shown in Fig. S1.†8 Peaks with good segregation, which also occupied large areas from consecutive peaks, were determined as the common peaks of PN samples. Therefore, fifteen peaks were selected by comparing their ultraviolet spectra and HPLC retention time. The method used to identify common peaks refers to reports in similar researches.24,25 The areas of 15 peaks in 18 batches of PN samples were listed in Table S1.†8 “The peak area was defined as “0” for peaks lacked in chromatograms. The coefficients of variance for almost all common peaks were higher than 46.6%. This is due to the diversity in the levels of components contained in samples under different process conditions. The areas of 15 common peaks were used for the following analysis”.8
3.2. Screening of induced concentration and time of LPS in inflammation model
3.2.1. Screening of optimal concentrations of LPS to induce TNF-α and IL-6. RAW264.7 cells are mouse macrophages, which can produce a variety of inflammatory factors including TNF-α, IL-8, IL-6, IL-1 and inflammatory transmitter NO under the stimulation of bacterial products and external harmful substances.26,27 LPS is the major component of the cytoderm of Gram negative bacteria, which can activate monocyte-macrophage system like RAW264.7 cell, resulting in the release of a large number of pro-inflammatory mediators such as TNF-α and IL-1β, causing systemic inflammatory response syndrome and further developing into multiple organ dysfunction, failure, damage or even death.28,29 In this study, LPS was used to induce the cell inflammation model. Then the levels of TNF-α and IL-6 were determined to evaluate the anti-inflammatory activity of RPN and SPN. As shown in Fig. 1A, the level of TNF-α was increased after RAW264.7 cells were stimulated by different concentrations of LPS (0, 0.25, 0.5, 1.0, and 2.0 μg mL−1) for 48 h. Meanwhile, the level of TNF-α in cells treated by LPS of 1.0 μg mL−1 reached the maximum, but then decreased with the increase of LPS concentration, which was consistent with the research of Liu, T. X. et al.30 Based on the above results, 1.0 μg mL−1 was determined as optimal concentration of LPS to induce TNF-α for the subsequent tests.
 |
| Fig. 1 Effects of different concentrations of LPS on levels of TNF-α (A) and IL-6 (B and C) ( ± s, n = 3). | |
As shown in Fig. 1B, the level of IL-6 was gradually increased after RAW264.7 cells were stimulated by different concentrations of LPS (0, 0.25, 0.5, 1.0, and 2.0 μg mL−1) for 48 h. While when the concentration of LPS was further increased (4.0, 6.0, 8.0, and 10.0 μg mL−1), the level of IL-6 was became gradually decreased (Fig. 1C). Therefore, 2.0 μg mL−1 was determined as the optimal concentration of LPS to induce IL-6 for the subsequent tests.
3.2.2. Screening of optimal time for LPS to induce TNF-α and IL-6. As shown in Fig. 2A, the levels of TNF-α were generally increased along with the increase of induced time when LPS at the concentration of 1.0 μg mL−1. After 24 h of treatment, the level of LPS was decreased. Besides, the level of IL-6 showed the similar trend when treated with LPS at the concentration of 2.0 μg mL−1 (Fig. 2B). Therefore, 24 h was determined as optimal time for LPS to induce TNF-α and IL-6 for the subsequent tests.
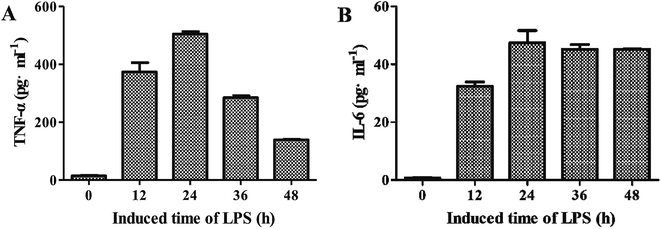 |
| Fig. 2 Effects of different induced time of LPS on levels of TNF-α (A) and IL-6 (B) ( ± s, n = 3). | |
3.3. Effects of raw and steamed PN extracts and LPS on cell growth
MTT was the earliest method to determine cell viability and established by Mosmann in 1983,31 which is simple, rapid and free of radioactive contamination. However, the insoluble formazan produced by the MTT needs DMSO to dissolve. And it is easy to take during the experiment, which will lead to the instability of results. In recent years, other methods such as MTS (3-(4,5-dimethylthiazol-2-yl)-5-(3-carboxymethoxyphenyl)-2-(4-sulfophenyl)-2H-tetrazolium) have emerged to overcome the shortcomings of the MTT.32 Therefore, in our study, MTS cell proliferation assay was used to detect the inhibitory effect of RPN, SPN, and LPS on the proliferation of RAW264.7 cells.
The curves of cell growth after treating RPN, SPN, and LPS were shown in Fig. S2.† The inhibition rate of cell growth was generally increased along with the increase of PN concentration. Compared with the treatment with only PN extracts, the combined use of LPS and PN extracts showed relatively greater inhibition on the cell growth. The IC50 values, the concentration of PN extracts and LPS inhibiting 50% cells, were summarized in Table 1. Also, RPN samples (S1–S3) exhibited higher IC50 values of cell growth inhibition rate, indicating that the cytotoxicity of SPN was greater than RPN at the same concentration. The similar trend of the combined treatment with LPS and RPN/SPN could also be observed. Accordingly, the concentrations lower than the IC50 values were used for the subsequent tests in order to reduce the cytotoxic effects of LPS and PN on cells.
Table 1 The effect of RPN, SPN, and LPS on cell growth (IC50, mg mL−1)a
LPS |
RPN |
SPN steamed at 105 °C |
SPN steamed at 110 °C |
SPN steamed at 120 °C |
1 |
2 |
3 |
2 h |
4 h |
6 h |
8 h |
10 h |
2 h |
4 h |
6 h |
8 h |
10 h |
2 h |
4 h |
6 h |
8 h |
10 h |
− −, without the treatment of LPS; + +, co-treatment of LPS and PN extracts. |
− − |
8.58 |
7.36 |
9.75 |
5.48 |
5.30 |
5.78 |
4.78 |
5.09 |
5.26 |
4.67 |
6.02 |
6.38 |
6.02 |
5.33 |
4.77 |
5.53 |
6.50 |
7.87 |
+ + |
6.71 |
5.08 |
8.09 |
4.38 |
4.86 |
4.72 |
4.13 |
4.18 |
3.87 |
4.30 |
5.72 |
4.65 |
4.38 |
6.02 |
3.95 |
4.28 |
5.58 |
4.71 |
3.4. Effects of RPN and SPN extracts on the levels of TNF-α and IL-6 by LPS
In this study, we explored the effects of RPN and SPN on the levels of inflammatory cytokines induced by LPS. Fig. 3A and B showed the IC50 values of TNF-α and IL-6 inhibition rates, respectively. According to Fig. S2,† the inhibition rates of both RPN and SPN were increased with the increase of the sample concentrations. And when a certain concentration was reached, the inhibition rates achieved the maximum. According to Fig. 3, the SPN exhibited much higher IC50 values of TNF-α and IL-6 inhibition rates than RPN, indicating that RPN had stronger anti-inflammatory activity than steamed ones. In addition, the IC50 value of the SPN sample was increased with the elevation of the steaming temperature and time, suggesting the anti-inflammatory activity of SPN steamed at higher temperature for a longer time was relatively weaker.
 |
| Fig. 3 The IC50 values of RPN and SPN on inhibiting TNF-α (A) and IL-6 (B). | |
3.5. Uncovering active components by multivariate data analyses
3.5.1. BCA. BCA was used to establish the spectrum-effect relationships between the area values of 15 common peaks in the HPLC data and the IC50 of inhibition rates (TNF-α and IL-6). The analysis result was shown in Fig. 4. According to the correlation coefficients, the inhibition rates of TNF-α and IL-6 were both positively correlated with X3, X5, X7, X10, X12, X13, X14 and X15 (P < 0.01). Besides, six peaks of X1, X2, X4, X6, X8 and X11 were significantly negatively correlated with the IC50 of TNF-α and IL-6 inhibition rates (P < 0.01). It suggested that the corresponding components of peaks 1, 2, 4, 6, 8 and 11 might play the major role in the anti-inflammatory activity of PN.
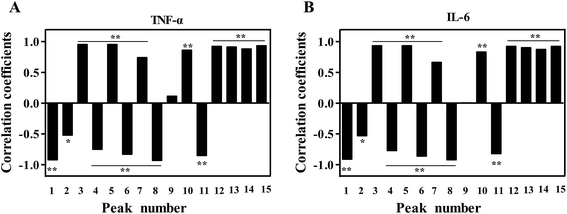 |
| Fig. 4 The correlation coefficients of TNF-α (A) and IL-6 (B) by BCA for the anti-inflammatory activity of PN. | |
3.5.2. PLSR. PLSR models were built from the normalized data matrix X containing the 18 PN fingerprints and the response matrix Y (including Y1 and Y2, which represented the IC50 of TNF-α and IL-6 inhibition rate, respectively). For the established model, two principle components were achieved, with an explained variance of 85.7% for X variable, 89.3% for Y variable, and a predictive ability (Q2) of 84.2% (Table 2), suggesting that the model was excellent. As shown in the regression coefficients plot (Fig. 5), peaks 3, 5, 7, 10 and 12–15 were positively correlated with the IC50 of TNF-α and IL-6 inhibition rate, whereas peaks 1, 2, 4, 6, 8, 9 and 11 were negatively correlated with the IC50 of TNF-α and IL-6 inhibition rate. It should be noted that a negative coefficient did not always mean that the relevant variable made an opposite effect on the anti-inflammatory activity. Thus the predicted IC50 values could not been determined if these variables were increased or decreased.
Table 2 Factor cumulative explained variance of PLSR
Principle |
X variable |
Cumulative X |
Y variable |
Cumulative Y variable |
Q2/cum |
R2 |
1 |
0.528 |
0.528 |
0.471 |
0.471 |
0.860 |
0.615 |
2 |
0.329 |
0.857 |
0.422 |
0.893 |
0.842 |
0.707 |
 |
| Fig. 5 Regression coefficient plots of the anti-inflammatory activity of 15 peaks in the chromatograms of PN. | |
In addition, the importance of the X-variables for the model could be summarized by variable importance for the projection (VIP) values (usually with a threshold > 1.0). Those with small values or no correlation (threshold < 1.0) were removed. Therefore, the components corresponding to the peaks 1–8 and 12 with VIP > 1.0 and high absolute values of coefficients were considered to be closely related to the anti-inflammatory effect of different PN samples (Table 3). Furthermore, the false discovery rate (FDR, usually with a threshold ≤ 0.05) can effectively solve the control of false positive error in multiple comparisons of high-dimensional data, and can significantly improve the efficiency of hypothesis testing.33 Therefore, components corresponding to peaks 1–6, 8 and 12, of which the FDR values were ≤0.05, were predicted to be correlated with the anti-inflammatory effect. Among them, peak 1–4, 6, 8 and 12 had significant correlation with anti-inflammatory effect by P-value correcting (P < 0.05).
Table 3 Variable importance in projection of PLSR for the anti-inflammatory activity of PN
Peak variable |
1 |
2 |
3 |
4 |
5 |
6 |
7 |
8 |
9 |
10 |
11 |
12 |
13 |
14 |
15 |
VIP |
1.13 |
1.11 |
1.01 |
1.09 |
1.11 |
1.13 |
1.04 |
1.11 |
0.91 |
0.87 |
0.45 |
1.11 |
0.39 |
0.72 |
0.19 |
FDR |
0.02 |
0.04 |
0.01 |
0.01 |
0.02 |
0.03 |
0.06 |
0.04 |
0.02 |
0.22 |
0.03 |
0.03 |
0.21 |
0.06 |
0.42 |
P |
0.01 |
0.03 |
0.02 |
0.03 |
0.11 |
0.01 |
0.05 |
0.04 |
0.04 |
0.18 |
0.003 |
0.01 |
0.10 |
0.002 |
0.33 |
3.5.3. Identification of bioactive components corresponding to predicted peaks. Based on BCA and PLSR results, components corresponding to peaks 1–4, 6, 8 and 12 were predicted to be anti-inflammatory ones of PN. By comparing the chromatograms of PN samples with that of the mixture of reference substances (Fig. 6), peaks 1–3, 6 and 12 were identified to be notoginsenoside R1, ginsenosides Rg1, Re, Rb1 and 20(S)-Rg3, respectively. However, components corresponding to peaks 4 and 8 were still unknown.
 |
| Fig. 6 The chromatogram of the mixture of reference substances. Peak 1, 2, 3, 6, and 12 correspond to notoginsenoside R1, ginsenosides Rg1, Re, Rb1, and 20(S)-Rg3, respectively. | |
From Table S1 and Fig. S1B,† peaks 1–3 and 6 were observed in the chromatographic fingerprints of RPN, whereas peak 12 was exclusively existed in the fingerprints of SPN. Therefore, components corresponding to peaks 1–3 and 6 (i.e., notoginsenoside R1, ginsenosides Rg1, Re and Rb1) might play the major role in the anti-inflammatory activity of RPN, and component corresponding to peak 12 (i.e., ginsenosides 20(S)-Rg3) could be the major anti-inflammatory one of SPN. Next, the contents of those components in raw and steamed PN were determined (Fig. 7). According to the results, the levels of notoginsenoside R1, ginsenosides Rg1, Re and Rb1 were decreased along with the increase of steaming temperature and duration of time. Conversely, the level of ginsenosides 20(S)-Rg3 showed the opposite trend, which only existed in SPN samples. Those components could be the differential markers for the individual QC of RPN and SPN.
 |
| Fig. 7 The contents of anti-inflammatory components in RPN and SPN at different steaming temperatures. The points on the scatter plots represent the quantities of the five components in 18 batches of PN samples. Error bars represent mean ± s.e.m. From a two-tailed unpaired Student's t-test, *P < 0.05, **P < 0.01. | |
3.6. Pharmacologic test
In order to verify the predicted five active components and determine their dedication to the anti-inflammatory activity of PN, the inhibition effects of the five components on TNF-α and IL-6 were then tested. As shown in Fig. 8, the sequence of the inhibitory effects of the components on TNF-α was notoginsenoside R1 > ginsenoside Rg1 > ginsenoside Re > ginsenoside Rb1 > ginsenoside 20(S)-Rg3, and the sequence for IL-6 was notoginsenoside R1 > ginsenoside Rg1 > ginsenoside Rb1 > ginsenoside Re > ginsenoside 20(S)-Rg3. The results indicated that the anti-inflammatory activity of ginsenosides Rg1, Re, Rb1, and notoginsenoside R1 were stronger than 20(S)-Rg3, which were consistent with the predicted ones.
 |
| Fig. 8 The measured IC50 values of five PN ginsenosides on the inhibition of TNF-α (A) and IL-6 (B). | |
In our study, BCA and PLSR analyses were used to predict components related to the anti-inflammatory effect of PN. The contents of ginsenosides Rg1, Rb1, and Re, and notoginsenoside R1 in the RPN were decreased gradually with the prolongation of steaming, while ginsenoside 20(S)-Rg3 was formed. That transformation might be due to the dehydration and hydrolyzation of components induced by high temperature. Ginsenosides Rg1, Rb1, Re and notoginsenoside R1 were previously reported to inhibit the production of inflammation-related cytokines,34–37 which coincides with our results and could be the reason that the RPN represented the stronger anti-inflammation effects. Our previous studies8 have shown that there was also significant difference in the anticoagulation and antioxidation effects of RPN and SPN. We hold that the conversion of saponins in the process of steaming accounted for a substantial part for the change in its efficacies. According to the contents and distributions of saponins in PN, ones with stronger activity and higher contents could be determined as the markers related to the corresponding effect of RPN and SPN. The detailed mechanisms underlying the anti-inflammatory activity of PN should be studied in further investigation.
4. Conclusions
In this study, the analysis of spectrum-effect relationship was used to uncover components related to the anti-inflammatory effects of RPN and SPN. There was a significant variation in the chemical composition between the two forms of PN, which led to their difference in the anti-inflammatory effects. The results showed that ginsenosides Rg1, Re, Rb1, and notoginsenoside R1 were the major anti-inflammatory components of RPN, whereas 20(S)-Rg3 played the main role in the anti-inflammation effect of the steamed materials. This research provided an available method for revealing the active material basis of herbal medicines, of which the results could be beneficial for the individual quality control for RPN and SPN, as well as guiding the optimization of processing methods and parameters for this herbal medicine.
Conflicts of interest
There are no conflicts to declare.
Acknowledgements
The study was supported by National Natural Science Foundation of China (81660661), Yunnan Applied Basic Research Key Project (2017ZF001) and National Research Program of China (2017YFC02503).
References
- F. Ge, Z. J. Huang, H. Yu, Y. Wang and D. Q. Liu, Biotechnol. Biotechnol. Equip., 2015, 30, 1–8 Search PubMed.
- 103rd Congress, Dietary supplement health and education act of 1994, Law 103-417, 108 Stat 4325, 1994 Search PubMed.
- C. Z. Gu, J. J. Lv, X. X. Zhang, Y. J. Qiao, H. Yan, Y. Li, H. Wang, H. T. Zhu, H. R. Luo, C. Y. Yang, M. Xu and Y. J. Zhang, J. Nat. Prod., 2015, 78, 1829–1840 CrossRef CAS.
- A. J. Lau, S. O. Woo and L. H. Koh, J. Chromatogr. A, 2003, 1011, 77–87 CrossRef CAS.
- H. L. koh, A. J. Lau and E. C. Yong Chan, Rapid Commun. Mass Spectrom., 2010, 19, 1237–1244 CrossRef PubMed.
- D. Wang, P. Y. Liao, H. T. Zhu, K. K. Chen, M. Xu, Y. J. Zhang and C. R. Yang, Food Chem., 2012, 132, 1808–1813 CrossRef CAS.
- S. Sun, C. Z. Wang, R. Tong, X. L. Li, A. Fishbein, Q. Wang, T. C. He, W. Du and C. S. Yuan, Food Chem., 2016, 118, 307–314 CrossRef.
- Y. Xiong, L. J. Chen, Y. P. Hu and X. M. Cui, Front. Pharmacol., 2017, 8, 754 CrossRef.
- Y. Xiong, L. J. Chen, J. H. Man, Y. P. Hu and X. M. Cui, J. Ginseng Res., 2017, 1–9 Search PubMed.
- Y. Xiong, Y. P. Hu, L. J. Chen, Z. Zhang, Y. Zhang, M. Niu and X. Cui, Front. Pharmacol., 2018, 1514 Search PubMed.
- Chinese Pharmacopoeia Commission, Pharmacopoeia of the People's Republic of China, China Medical Science Press, Beijing, 2015 Search PubMed.
- China Food and Drug Administration, Shusanqi San, China Zhijian Publishing House, Beijing, 2012 Search PubMed.
- J. S. Fan, D. N. Liu, G. Huang, Z. Z. Xu, Y. Jia, H. G. Zhang, X. H. Li and F. T. He, J. Ethnopharmacol., 2012, 142, 732–738 CrossRef CAS PubMed.
- Y. Wang, D. Z. Peng, W. H. Huang, X. Zhou, J. Liu and Y. F. Fang, Burns, 2006, 32, 846–852 CrossRef PubMed.
- G. L. Xu, M. Xie, X. Y. Yang, Y. Song, C. Yan, Y. Yang, X. Zhang, Z. Z. Liu, Y. X. Tian, Y. Wang, R. Jiang, W. R. Liu, X. H. Wang and G. M. She, Molecules, 2014, 19, 17897–17925 CrossRef PubMed.
- A. Rhule, S. Navarro, J. R. Smith and D. M. Shepherd, J. Ethnopharmacol., 2006, 106, 121–128 CrossRef PubMed.
- Y. F. Xian, Y. C. Li, S. P. Ip, Z. X. Lin, X. P. Lai and Z. R. Su, Exp. Ther. Med., 2011, 2, 545–550 CrossRef CAS PubMed.
- L. J. Liu, Y. L. Wang, S. Q. Xu, S. B. Wei and J. X. Li, World J. Integr. Tradit. West. Med., 2015, 10, 1069–1072 Search PubMed.
- L. J. Wang, Z. M. Jiang, P. T. Xiao, J. B. Sun, Z. M. Bi and E. H. Liu, J. Pharm. Biomed. Anal., 2019, 167, 38–48 CrossRef CAS PubMed.
- Q. Liu, Z. Wang, L. L. Liu, P. Li and E. H. Liu, RSC Adv., 2016, 6, 96039–96048 RSC.
- M. F. a. A, Iuliano, Correlation Analysis, Institute for Applied Mathematics (IAC): “Mauro Picone”, Rome, Italy, 2018 Search PubMed.
- H. Martens and T. Naes, Multivariate Calibration, Wiley, New York, NY, 1989 Search PubMed.
- F. Vogt, J. Chem. Educ., 2007, 84, 1926 CrossRef CAS.
- Z. L. Shi, Z. J. Liu, C. S. Liu, M. Q. Wu, H. B. Su, X. Ma, Y. M. Zang, J. B. Wang, Y. L. Zhao and X. H. Xiao, Front. Pharmacol., 2016, 7, 12 Search PubMed.
- Q. F. Zheng, Y. L. Zhao, J. B. Wang, T. T. Liu, B. Zhang, M. Gong, J. Y. Li, H. H. Liu, B. Han, Y. M. Zhang, X. A. Song, Y. G. Li and X. H. Xiao, J. Ethnopharmacol., 2014, 153, 615–623 CrossRef CAS PubMed.
- S. H. Lu, W. L. Hsu, T. H. Chen and T. C. Chou, Int. Immunopharmacol., 2015, 29, 770–778 CrossRef CAS PubMed.
- J. H. Kim, S. Y. Oh, S. B. Han, G. M. Uddin, C. Y. Kim and J. K. Lee, Arch. Pharmacal Res., 2015, 38, 1117–1126 CrossRef CAS PubMed.
- D. H. Harrison, C. A. Welch and J. M. Eddleston, Crit. Care, 2006, 10, R42 CrossRef PubMed.
- X. T. Wang, Y. Tanino, S. Sato, T. Nikaido, K. Misa, N. Fukuhara, A. Fukkuhara, J. Saito, H. Yokouchi, T. Ishida, T. Fujita and M. Munakata, Inflammation, 2015, 38, 828–834 CrossRef CAS PubMed.
- T. X. Liu, J. Li, G. J. Jiang, X. M. Dong, Z. M. Zhu and G. H. Huang, Her. Med., 2017, 36, 601–605 Search PubMed.
- M. Mosmann, J. Immunol. Methods, 1983, 65, 55–63 CrossRef PubMed.
- G. Malich, B. Markovic and C. Winder, Toxicology, 1997, 124, 179–192 CrossRef CAS PubMed.
- Y. Benjamini and Y. Hochberg, J. Royal Stat. Soc., 1995, 57, 289–300 Search PubMed.
- D. F. Qu, H. J. Yu, Z. Liu, D. F. Zhang, Q. J. Zhou, H. L. Zhang and A. F. Du, Vet. Parasitol., 2011, 179, 28–34 CrossRef CAS PubMed.
- C. L. Zhuang, X. Y. Mao, S. Liu, W. Z. Chen, D. D. Huang, C. J. Zhang, B. C. Chen, X. Shen and Z. Yu, Eur. J. Pharmacol., 2014, 740, 480–487 CrossRef CAS PubMed.
- K. W. Lee, S. Y. Jung, S. M. Choi and E. J. Yang, BMC Complementary Altern. Med., 2012, 12, 196 CrossRef CAS.
- B. Sun, J. Xiao, X. B. Sun and Y. Wu, Br. J. Pharmacol., 2013, 168, 1758–1770 CrossRef CAS PubMed.
Footnotes |
† Electronic supplementary information (ESI) available: Chart supplement. See DOI: 10.1039/c9ra00906j |
‡ These authors contributed equally to this work. |
|
This journal is © The Royal Society of Chemistry 2019 |
Click here to see how this site uses Cookies. View our privacy policy here.