DOI:
10.1039/C8NR06526H
(Paper)
Nanoscale, 2019,
11, 1737-1744
Quantitative profiling of CD13 on single acute myeloid leukemia cells by super-resolution imaging and its implication in targeted drug susceptibility assessment†
Received
13th August 2018
, Accepted 12th December 2018
First published on 9th January 2019
Abstract
Quantitative profiling of membrane proteins on the cell surface is of great interest in tumor targeted therapy and single cell biology. However, the existing technologies are either of insufficient resolution, or unable to provide precise information on the localization of individual proteins. Here, we report a new method that combines the use of quantum dot labeling, super-resolution microscopy (structured illumination microscopy, SIM) and software modeling. In this proof-of-principle study, we assessed the biological effects of Bestatin on individual cells from different AML cell lines expressing CD13 proteins, a potential target for tumor targeted therapy. Using the proposed method, we found that the different AML cell lines exhibit different CD13 expression densities, ranging from 0.1 to 1.3 molecules per μm2 cell surface, respectively. Importantly, Bestatin treatment assays shows that its effects on cell growth inhibition, apoptosis and cell cycle change are directly proportional to the density of CD13 on the cell surface of these cell lines. The results suggest that the proposed method advances the quantitative analysis of single cell surface proteins, and that the quantitative profiling information of the target protein on single cells has potential value in targeted drug susceptibility assessment.
Introduction
Quantitative profiling of membrane proteins on the cell surface is a new approach in single cell biology, and progressively draws attention in tumor targeted therapy research.1–3 Traditional methods of protein analysis include western blot, flow cytometry and mass spectrometry. Although these methods have greatly elucidated that how these protein molecules are involved in various biological processes, they are not suitable for a detailed understanding as they failed to explore the detailed phenomena of how individual proteins behave on single cells within a cell population.4,5 Currently available methods for analyzing protein molecules on the surface of cells include multiparameter flow cytometry (MFC),6 flow cytometry and single cell mass spectrometry (SCMS).7 These methods have been useful for understanding of minute amounts of specific proteins, but are not sufficient to perform quantitative in situ protein analysis8–10 because of insufficient resolution and low intensity of fluorescence labeling tags. Super-resolution microscopy technologies, such as STED,11 PALM12 and STORM,13 can have extremely high resolution and were used to analyze protein distribution in situ.14 These methods could be used for quantitative profiling of single protein on single cells but there is no report yet, probably because of some limitations of the methods, such as complexity of instruments and experiments.
Our solution is named super-resolution 3D molecular imaging (3DMI). Among fluorescent labels used for molecular imaging, quantum dots are more and more applied because they feature high brightness and quench resistance.15 Here, we set out to label the single protein with quantum dots in situ that is able to perform imaging by super-resolution microscopy (structural illumination microscope, SIM) and to perform 3D reconstruction by computer simulation, thus allowing quantitative analysis of specific proteins in single cells. Previously, we have combined quantum dot technology with super resolution imaging to analyze biological processes at the single cell level. For instance, we successfully tracked single virions in the host cell,16,17 and monitored integration of a single copy HIV genome into the host chromosome.18
To demonstrate the design, we used acute myeloid leukemia (AML) cell lines NB4, HL60 and KG1, to investigate the distribution and density of the cell surface protein and leukemia marker CD13. The cure rate of AML is currently 35 ± 40% in patients below 60 years and 5 ± 15% above 60 years of age.19 CD13 is found to be upregulated on AML, rendering a special inhibitor for CD13 (Bestatin), a potential targeted drug for related tumor types.20–22 We hypothesized that the difference of CD13 density on the test cell lines would lead to efficiency difference of the targeted-drug treatment, and it was true.
Results
Scheme of labeling CD13 proteins on the cell surface using quantum dots
In order to generate high resolution images of individual CD13 molecules on the cell surface of AML cells, we specifically targeted CD13 using antibody–quantum dot conjugates (ESI Fig. S1†) by sandwich means. TEM images show that the QDs coated with secondary antibody exhibited a homogeneous structure of a similar size of approx. 20 nanometers (Fig. 1b). Fig. 1a shows the labeling scheme. The test cells were incubated with an excess of the primary antibody (Ab1) followed by incubation with the secondary antibody–QD conjugates (Ab2-QDs). TEM analysis revealed that the QD conjugates bind to CD13 on the membrane of the NB4 cell with a distance between two quantum dots of several hundreds of nanometers, which tentatively indicates the dispersity of the CD13 protein on the NB4 cell surface.
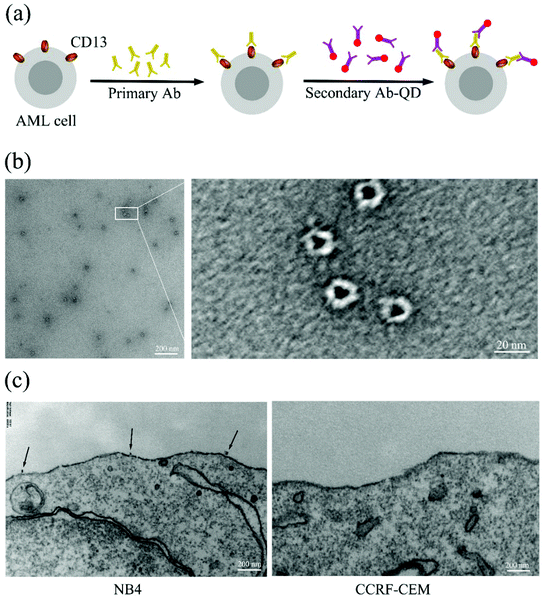 |
| Fig. 1 Schematic illustration of QD staining and EM imaging. (a) Schematic illustration of CD13 labeling. (b) TEM images of the Ab2-QDs. (c) TEM images of the Ab2–QD bond on the CD13 positive cell (NB4) surface (left) and no bonding on the CD13 negative cell (CCRF-CEM) surface (right). | |
Quantum dot labeling and SIM allow single CD13 molecule imaging at super resolution
Fig. 2a shows the single slice (125 nm in thickness) of the whole cell image with the QD-labeled CD13 proteins under a laser scanning confocal microscope (LSCM) and a SIM. As can be seen, the confocal microscope yielded images of CD13 proteins continuously distributed on the cell membrane, in which the single protein resolution is unsatisfactory. The wide field SIM (SIM-WF) considerably improved the discrimination of single proteins, while the SIM imaging yielded superior resolution with CD13 molecules being visible as individual dots on the membrane of AML cells. Fig. 2b shows the whole cell projection images of LSCM, SIM-WF and SIM, respectively. The single quantum dot signal can be distinguished clearly by SIM imaging, which was therefore applied for 3D reconstruction of the cell surfaces and the quantum dot labeling signal patterns of each test cell line.
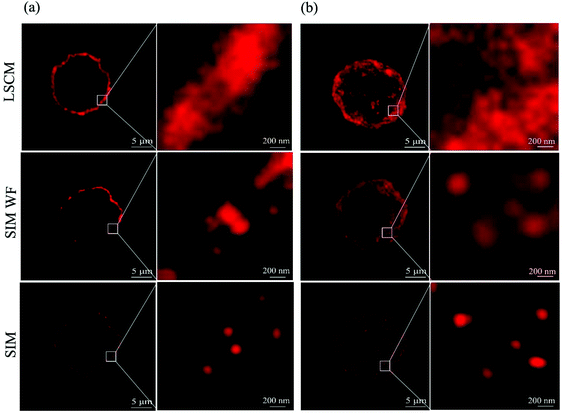 |
| Fig. 2 CD13 images on the surface of a single NB4 cell. (a) Slice section images and their enlarged image under the confocal microscope, SIM wide field and SIM, respectively. (b) Projection images and their enlarged image under the confocal microscope, SIM wide field and SIM, respectively. | |
SIM imaging and QD labeling based analysis reveal cell line-specific CD13 cell surface densities
To compare the density of CD13 on different AML cell lines, we profiled the CD13 density on the surface of AML cells using SIM imaging. Fig. 3a depicts the process in which the image data collected were processed by using Imaris software (ESI S3†). First, QD conjugates emit optical signals as the location information of CD13 proteins collected by SIM microscopy. Second, Imaris software fit the core of all the luminescence points and the surface area of the cell membrane. Using this information, we reconstructed the three-dimensional digital simulation of the distribution pattern of the CD13 protein on the cell surface and were able to calculate the density of CD13 proteins (Fig. 3a, ESI Video S4†). As shown in Fig. 3b, Imaris 3D reconstruction and digital simulation of the three AML cell lines NB4, HL60 and KG1 show that the QD signals of CD13 proteins diffused randomly throughout the cell membrane. The densities of CD13 protein signals in three different subtypes of cells were different, i.e. 1.30 per μm2 in NB4 cells, 0.15 per μm2 in HL60 cells and 0.11 per μm2 in KG1 (Fig. 3c). ANOVA statistical analysis showed that the CD13 density on the surface of NB4 cells is significantly higher than the density of CD13 on HL60 and KG1 cells (p < 0.05, Fig. 3c). These results were verified with the semi-quantitative analysis by flow cytometry (ESI Fig. S2†).
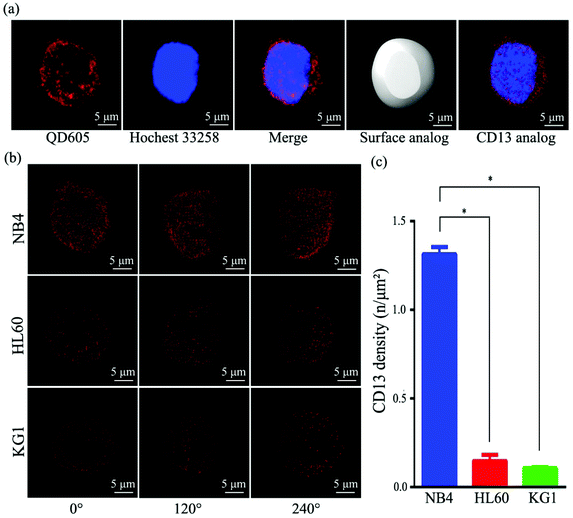 |
| Fig. 3 Quantitative profiling and 3D reconstruction of CD13 on AML cell lines with SIM and software Imaris. (a) Distribution pattern of CD13 on the single cell surface reconstructed from the original SIM image (NB4 as the example). (b) Density and distribution pattern of CD13 on three AML single cells at horizontal viewing angles of 0°, 120° and 240°. (c) Comparison of CD13 density on the surface of single cells for three AML cell lines, using ANOVA. *, p < 0.05. Red, quantum dot 605; blue, Hoechst 33258. | |
CD13 density directly correlates with the degree of effects on cell growth inhibition, apoptosis and cell cycle following Bestatin treatment
On the basis of the CD13 quantification, we hypothesized that the subtypes of AML cells expressing different levels of CD13 molecules differ in their sensitivity to Bestatin. In order to test the relationship between the density of CD13 on the cell membrane and the Bestatin treatment effects, we compared the effects of Bestatin treatment related to growth inhibition, apoptosis and cell cycle in the three AML cell lines. As shown in Fig. 4, the inhibitory effects of increasing concentrations of Bestatin are most prominent in NB4 cells (Fig. 4a), similar to the effects with increasing the treatment times (Fig. 4b), while the control cells (CCRF-CEM, CD13 negative expression) showed no obvious response to the pressure of Bestatin. Treatment of cells with 500 μM Bestatin for 48 h induced apoptosis in NB4 cells. Compared with NB4 cells, HL60 and KG1 cells showed no statistically significant differences over the control cells (CCRF-CEM, CD13 negative expression) (Fig. 5).
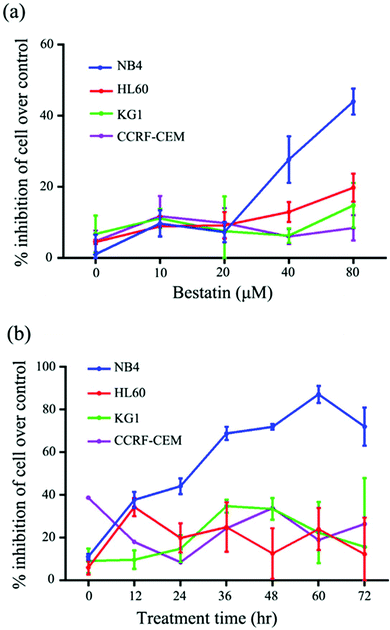 |
| Fig. 4 Inhibition of growth of three AML cells and CD13 negative cell CCRF-CEM by Bestatin. (a) Effect of concentration of Bestatin on inhibition effects in 24 hours. (b) Effect of treatment time by Bestatin (80 μM) on growth inhibition effects. | |
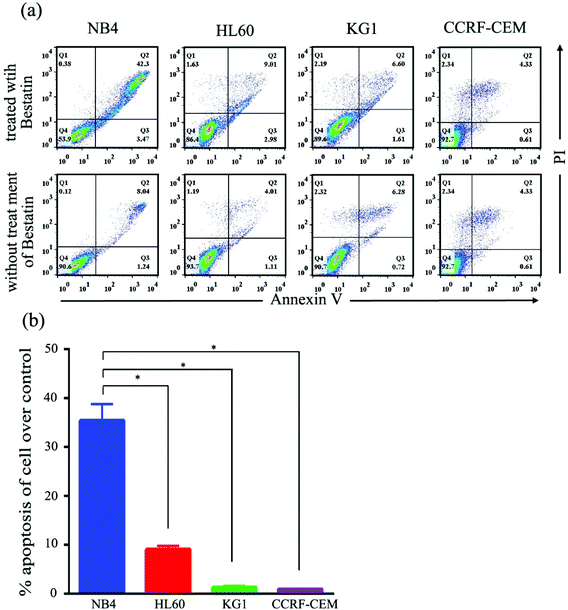 |
| Fig. 5 Flow cytometric analysis of cell apoptosis induced by Bestatin (500 μM, 48 h). (a) Apoptosis diagrams of flow cytometry for the three AML cells and CCRF-CEM treated with or without Bestatin. (b) Comparison of apoptosis between the three AML cells and CCRF-CEM based on the flow cytometry experiment, with ANOVA. *, p < 0.05. | |
In the cell cycle experiment, we treated all four cell lines with Bestatin (500 μM, 48 h) and compared those results with the effects on the controls lacking Bestatin treatment. The flow cytometric analysis showed that the distribution of cells in different cell cycle stages significantly changed in the sub-G1 phase, while it changed little in G0/G1, S and G2/M phases (Fig. 6a and b). NB4 cells treated with Bestatin showed an increase in their sub-G1 population (9.60 ± 1.01%) compared to the control lacking Bestatin treatment (Fig. 6c). In contrast, the percentages of cells in HL60, KG1 and CCRF-CEM cells in their sub-G1 phase were 0.83 ± 0.20%, 2.13 ± 0.87% and 0.40 ± 0.08%, respectively. Statistically, the increased sub-G1 ratio induced by Bestatin in NB4 cells is higher than those in HL60, KG1 and CCRF-CEM cells (Fig. 6c, p < 0.05).
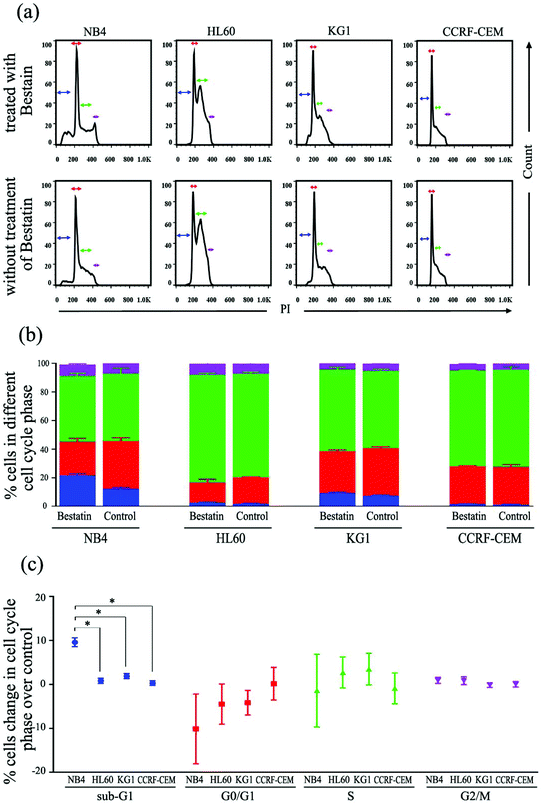 |
| Fig. 6 Flow cytometric analysis of cell cycle changes induced by Bestatin. (a) Propidium iodide (PI) stain indicated cell counts in different cell cycles in the flow cytometric experiment with or without Bestatin treatment (500 μM, 48 h). (b) Proportion (%) of cells in different cell cycle phases observed in the flow cytometric experiment. (c) Changes of the distribution proportion (%) in different cell cycle phases of the four AML cell lines after treatment with Bestatin over the control, with ANOVA. *, p < 0.05. | |
Discussion
Here we show that the QD labeling combined with SIM imaging is a reliable method to realize single membrane protein profiling on single cells. Single molecule imaging using optical microscopy faces two major challenges. The first is to obtain sufficiently high resolution to discriminate between adjacent proteins.23 The second is to design a label that does not quench during the optical scanning procedure.24 SIM combined with QD labeling has the potential to fulfill these conditions. On the one hand, SIM is a convenient super-resolution imaging method which surpasses the physical resolution limit as originally described by Ernst Abbe,25 although there are other advanced microscopy techniques that have even higher resolution. On the other hand, QDs are a kind of advanced semiconductor luminescent material with narrow and bright spectra.26 QDs display an outstanding anti-bleach property; thus samples labeled with QDs are ideal for SIM super resolution imaging and three-dimensional reconstruction. To this end, we were able to view the distribution patterns of the target protein molecules on intact cells, counting the least number of the target on single cells and the least number of it per μm2 cell surface. To our knowledge, this quantitative precision of the surface protein is obtained for the first time and described in our work. However, although we used an excess amount of the labeling reagents there was no guarantee that all CD13 molecules were labeled by the QDs. This is normal in most molecular reactions. So, we prefer to define our counting as “the least number”. Another concern we used to have was that SIM could not distinguish two dot signals if the distance between the QD-labeled target molecules was less than 100 nm, the resolution limit in the XY axis of SIM. Later, we found it unlikely to occur after viewing TEM images, where the distance between two CD13 molecules is several hundreds of nanometers, and distribution patterns of CD13 molecules on cell surfaces in 3D reconstruction images, whereby CD13 molecules were clearly visible as individual dots.
Another major finding is that there is density disparity of target proteins between the test cell lines. This difference not only reveals heterogeneity of the leukemia cells at the molecular phenotype level, but also has significant impact on the sensitivity of distinct AML cell lines to treatment with a specific CD13 inhibitor. The higher the density of the CD13 molecules, the more the sensitivity of the test cells to the Bastatin treatment (Fig. 4–6). Bestatin treatment did not affect the distribution of CD13 molecules on the AML cell membrane. We deduce that Bestatin and primary antibody have different combined epitopes on the CD13 protein. The mechanisms behind remain to be elaborated in dedicated studies.
Conclusion
In conclusion, the combination of QD labeling and SIM super-resolution imaging technology (3DMI) is reliable for profiling of specific protein targets on the surfaces of single cells in situ. Importantly, we show that this technique can be used to quantitatively measure the distribution and the density of individual proteins on the cell membranes. Furthermore, we established a functional correlation between CD13 density and cell biological effects of Bestatin treatment, thus providing an important reference for molecular classification of leukemia types. In the long-term, patients should benefit from more targeted protein profiling, improving clinical effects and avoiding ineffective treatments as well as excessive medication during targeted therapy.
Materials and methods
Cell culture and smear
Four leukemia cell lines of different classification were used in this study. NB4 cells were obtained courtesy of Pro. Xu Chinese Academy of Medical Sciences. HL60, KG1 and CCRF-CEM cells were purchased from Huiying-Bio (Shanghai, China). All the cells were cultured in the RPMI 1640 medium (HyClone), supplemented with 10% fetal bovine serum (FBS), penicillin (100 U ml−1) and streptomycin (100 μg ml−1) under a 5% CO2 humidified atmosphere at 37 °C. After washing three times with phosphate-buffered saline (PBS), the cells were fixed with 4% paraformaldehyde for 15 minutes at room temperature. After the cells were washed three times with the phosphate-buffered saline (PBS), about 5000 cells were centrifuged onto glass slides at 1000 rpm for 5 minutes by using a CytoSpin4 cytocentrifuge (Thermo Fisher).
Transmission electron microscopy
Cells were fixed with 2.5% (vol/vol) glutaraldehyde with Phosphate Buffer (PB) (0.1 M, pH 7.4), and washed four times with PB. Then cells were first immersed in 1% (wt/vol) OsO4 and 1.5% (wt/vol) potassium ferricyanide aqueous solution at 4 °C for 1 h. After washing, the cells were incubated in filtered 1% thiocarbohydrazide (TCH) aqueous solution (Sigma-Aldrich) at room temperature for 30 min, 1% unbuffered OsO4 aqueous solution at 4 °C for 1 h and 1% UA aqueous solution at 4 °C overnight. Then cells were dehydrated through graded alcohol (30, 50, 70, 80, 90, 100, 100%, 5 min) into pure acetone (2 times, 5 min). Samples were infiltrated into graded mixtures (3
:
1, 1
:
1, 1
:
3) of acetone and SPI-PON 812 resin (19.6 ml SPI-PON 812, 6.6 ml DDSA and 13.8 ml NMA), which were then changed to pure resin. Finally, cells were embedded in pure resin with 1.5% BDMA and polymerized for 12 h at 45 °C, 48 h at 60 °C. The ultrathin sections (70 nm per thick) were sectioned with a microtome (Leica EM UC6) and examined by using a transmission electron microscope (FEI Tecnai Spirit 120 kV).
Quantum dot staining
The cells attached to slides were treated with 10% goat serum in PBS for 1 hour to block nonspecific sites. The cells were incubated with mouse anti-human CD13 monoclonal antibody (1
:
300 in blocking solution, Abcam, ab7417) at 4 °C overnight. After washing three times with PBS, the cells were incubated with secondary antibody which is quantum dot conjugated anti-mouse IgG (1
:
200 in blocking solution, Wuhan Jiayuan, CN, QD 605) at room temperature for 1 hour and stained with Hoechst 33258 (Thermo Fisher, H3569) at room temperature for 15 minutes in the dark. After washing three times, the cells were visualized under structured illumination microscopy (DeltaVision OMX V3, API, USA).
Cell growth inhibition analysis
First, NB4, HL60, KG1 and CCRF-CEM cells were harvested after 24 hours for free serum treatment. Second, the cells were seeded into 96-well plates at a density of 5000 cells per well in the Bestatin concentrations of 0, 10, 20, 40 and 80 μM. Finally, the luminescence was detected using a Cytation 3 Cell Imaging Multi Mode Reader (Bio Tek) after incubation for 0, 12, 24, 36, 48, 60 and 72 hours using RealTime GloTM MT Cell Viability Assay (Promega Corporation) following the manufacturer's instructions.
Cell apoptosis analysis with Annexin V staining
The Annexin V-FITC apoptosis detection kit (Biofriend, CN) was used to detect cells undergoing apoptosis after Bestatin treatment following the manufacturer's instruction. NB4, HL60, KG1 and CCRF-CEM cells were incubated in 500 μM Bestatin for 48 hours. After washing three times with the PBS, 100 μL cell suspensions was mixed with 5 μL of Annexin V-FITC and 10 μL of PI and incubated for 15 min at room temperature in the dark. The samples were analyzed by flow cytometry on a FACS Calibur II (BD Biosciences). Cells stained with Annexin V were considered apoptotic cells.
Cell cycle analysis with propidium iodide staining
For cell cycle analysis, cells were incubated with 500 μM Bestatin for 24 h. After washing three times with the PBS, the NB4, HL60, KG1 and CCRF-CEM cells were stained by using a Cell Cycle Rapid Detection kit (dakewei, CN). The stained cells were analyzed by flow cytometry on a FACS Calibur II (BD Biosciences). Each histogram was constructed with data from at least 20
000 events. The flow cytometric analyses were performed using FlowJo software.
Statistical analysis
Data are expressed as means ± SD. The ANOVA (one-way analysis of variance) was used to examine differences between groups in cell proliferation, apoptosis, and cell cycle assay. P < 0.05 was taken as statistically significant. Statistical analyses were performed with GraphPad Prism software, version 6.0 (GraphPad Software, Inc., USA).
Conflicts of interest
The authors declare no competing financial interest.
Acknowledgements
This work was supported by a Grant from the National Key Research and Development Program of China (2017YFA0205500) and the Strategic Priority Research Program of Chinese Academy of Sciences (XDPB, CAS). The authors of this article are very grateful to Shuoguo Li, Xixia Li, Xueke Tan (CBI, IBP, CAS) and Junying Jia, Shuang Sun (FACS center, IBP, CAS) for technical support. We thank Dong Men and Feng Li for valuable discussion.
References
- D. B. Johnson, J. M. Bordeaux, J. Y. Kim, C. A. Vaupel, D. L. Rimm, T. H. Ho, R. W. Joseph, A. I. Daud, R. M. Conry, E. M. Gaughan, L. F. Hernandez-Aya, A. Dimou, P. Funchain, J. W. Smithy, J. S. Witte, S. B. McKee, J. Ko, J. Wrangle, B. Dabbas, S. Tangri, J. Lameh, J. M. Hall, J. Markowitz, J. M. Balko and N. K. Dakappagari, Clin. Cancer Res., 2018, 24(21), 5250–5260 Search PubMed.
- R. S. Croner, M. Sturzl, T. T. Rau, G. Metodieva, C. I. Geppert, E. Naschberger, B. Lausen and M. V. Metodiev, Int. J. Cancer, 2014, 135, 2878–2886 CrossRef CAS PubMed.
- P. I. Imoukhuede and A. S. Popel, Cancer Med., 2014, 3, 225–244 CrossRef CAS PubMed.
- T. E. Angel, U. K. Aryal, S. M. Hengel, E. S. Baker, R. T. Kelly, E. W. Robinson and R. D. Smith, Chem. Soc. Rev., 2012, 41(10), 3912–3928 RSC.
- M. Hamdan and P. G. Righetti, Mass Spectrom. Rev., 2002, 21(4), 287–302 CrossRef CAS PubMed.
- B. S. Edwards, T. Oprea, E. R. Prossnitz and L. A. Sklar, Curr. Opin. Chem. Biol., 2004, 8(4), 392–398 CrossRef CAS PubMed.
- M. H. Spitzer and G. P. Nolan, Cell, 2016, 165(4), 780–791 CrossRef CAS PubMed.
- I. C. Macaulay, C. P. Ponting and T. Voet, Trends Genet., 2017, 33, 155–168 CrossRef CAS PubMed.
- T. Fujii, S. Matsuda, M. L. Tejedor, T. Esaki, I. Sakane, H. Mizuno, N. Tsuyama and T. Masujima, Nat. Protoc., 2015, 10(9), 1445–1456 CrossRef CAS PubMed.
- C. A. Gedye, A. Hussain, J. Paterson, A. Smrke, H. Saini, D. Sirskyj, K. Pereira, N. Lobo, J. Stewart, C. Go, J. Ho, M. Medrano, E. Hyatt, J. Yuan, S. Lauriault, M. Meyer, M. Kondratyev, T. van den Beucken, M. Jewett, P. Dirks, C. J. Guidos, J. Danska, J. Wang, B. Wouters, B. Neel, R. Rottapel and L. E. Ailles, PLoS One, 2014, 9, e105602 CrossRef PubMed.
- J. Hanne, H. J. Falk, F. Gorlitz, P. Hoyer, J. Engelhardt, S. J. Sahl and S. W. Hell, Nat. Commun., 2015, 6, 7127 CrossRef CAS PubMed.
- P. Sengupta, T. Jovanovic-Talisman, D. Skoko, M. Renz, S. L. Veatch and J. Lippincott-Schwartz, Nat. Methods, 2011, 8, 969–975 CrossRef CAS PubMed.
- F. Baumgart, A. M. Arnold, K. Leskovar, K. Staszek, M. Folser, J. Weghuber, H. Stockinger and G. J. Schutz, Nat. Methods, 2016, 13, 661–664 CrossRef CAS PubMed.
- T. Lukes, D. Glatzova, Z. Kvicalova, F. Levet, A. Benda, S. Letschert, M. Sauer, T. Brdicka, T. Lasser and M. Cebecauer, Nat. Commun., 2017, 8, 1731 CrossRef PubMed.
- P. Zrazhevskiy and X. Gao, Nat. Commun., 2013, 4, 1619 CrossRef PubMed.
- F. Li, Z. P. Zhang, J. Peng, Z. Q. Cui, D. W. Pang, K. Li, H. P. Wei, Y. F. Zhou, J. K. Wen and X. E. Zhang, Small, 2009, 5, 718–726 CrossRef CAS PubMed.
- Y. Ma, M. Wang, W. Li, Z. Zhang, X. Zhang, T. Tan, X. E. Zhang and Z. Cui, Nat. Commun., 2017, 8, 15318 CrossRef CAS PubMed.
- Y. Ma, Z. He, T. Tan, W. Li, Z. Zhang, S. Song, X. Zhang, Q. Hu, P. Zhou, Y. Wu, X. E. Zhang and Z. Cui, ACS Nano, 2016, 10, 6273–6282 CrossRef CAS PubMed.
- H. Dohner, D. J. Weisdorf and C. D. Bloomfield, N. Engl. J. Med., 2015, 373, 1136–1152 CrossRef PubMed.
- B. Bauvois and D. Dauzonne, Med. Res. Rev., 2016, 26(1), 88–130 CrossRef PubMed.
- C. Schneider, M. Bayerl, C. Boyer, R. Desai, D. Claxton and A. Van de Louw, Am. J. Respir. Crit. Care Med., 2017, 196(8), 1077–1080 CrossRef CAS PubMed.
- P. Mina-Osorio, Trends Mol. Med., 2008, 14, 361–371 CrossRef CAS PubMed.
- G. Komis, O. Samajova, M. Ovecka and J. Samaj, Trends Plant Sci., 2015, 20(12), 834–843 CrossRef CAS PubMed.
- P. Wu and X. P. Yan, Chem. Soc. Rev., 2013, 42(12), 5489–5521 RSC.
- E. H. Rego, L. Shao, J. J. Macklin, L. Winoto, G. A. Johansson, N. Kamps-Hughes, M. W. Davidson and M. G. Gustafsson, Proc. Natl. Acad. Sci. U. S. A., 2012, 109(3), 135–143 CrossRef PubMed.
- U. Resch-Genger, M. Grabolle, S. Cavaliere-Jaricot, R. Nitschke and T. Nann, Nat. Methods, 2008, 5(9), 763–775 CrossRef CAS PubMed.
Footnotes |
† Electronic supplementary information (ESI) available. See DOI: 10.1039/c8nr06526h |
‡ These authors contributed equally to this work. |
|
This journal is © The Royal Society of Chemistry 2019 |
Click here to see how this site uses Cookies. View our privacy policy here.