DOI:
10.1039/C9NH00097F
(Communication)
Nanoscale Horiz., 2019,
4, 1063-1076
Disease-specific protein corona sensor arrays may have disease detection capacity†
Received
19th February 2019
, Accepted 22nd May 2019
First published on 17th June 2019
Abstract
The earlier any catastrophic disease (e.g., cancer) is diagnosed, the more likely it can be treated, providing improved patient prognosis, extended survival and better quality of life. In early 2014, we revealed that various types of disease can substantially affect the composition/profile of protein corona (i.e., a layer of biomolecules that forms at the surface of nanoparticles upon their interactions with biological fluids). Here, by combining the concepts of disease-specific protein corona and sensor array technology we developed a platform with disease detection capacity using blood plasma. Our sensor array consists of three cross-reactive liposomes, with distinct lipid composition and surface charge. Rather than detecting a specific biomarker, the sensor array provides pattern recognition of the corona protein composition adsorbed on the liposomes. As a feasibility study, sensor array validation was performed using plasma samples obtained from patients diagnosed with five different cancer types (i.e. lung cancer, glioblastoma, meningioma, myeloma, and pancreatic cancer) and a control group of healthy donors. Although no single corona composition is specific for any one cancer type, overlapping but distinct patterns of the corona composition constitutes a unique “fingerprint” for each type of cancer (with a high classification accuracy, i.e. 99.4%). To finally probe the capacity of this sensor array for early detection of cancers, we used cohort plasma obtained from healthy people who were subsequently diagnosed several years after plasma collection with lung, brain, and pancreatic cancers. Our results suggest that the disease-specific protein corona sensor array will not only be instrumental in the screening, detection, and identification of diseases, but may also help identify novel protein pattern markers whose role in disease development and/or disease biology has not been appreciated so far.
New concepts
In 2014, our group introduced the concept of “personalized”/“disease-specific” protein corona. Here, by combining the concepts of “disease-specific” protein corona and sensor array technology, we have created a platform for the detection and identification of diseases (five distinct human cancers were used as a model disease) ex vivo. The protein corona sensor array platform provides a library of corona compositions containing disease signatures. By analyzing the corona compositions of different nanoparticles, using supervised classifiers, we created a unique protein corona pattern which was the “fingerprint” of each type of cancer. Our results revealed that although no single protein corona composition from a single nanoparticle provides this “fingerprint” feature, we found that the pattern of corona composition derived from the nanoparticle sensor array provides a unique “fingerprint” for each type of cancer. To probe the capacity of this platform for very early detection of cancers, we used cohort plasma obtained from healthy people who were later diagnosed with lung, pancreas, and brain cancers several years after plasma collection and the outcomes revealed that the approach could identify and discriminate the cancers. We expect that the protein corona sensor array may also prove useful for the diagnosis of other devastating diseases.
|
It is now well accepted that nanoparticles in contact with biological fluids are quickly surrounded by a selected group of adsorbed proteins that form a corona1,2 whose composition is strongly dependent on the physicochemical properties of the nanoparticles themselves. The majority of these protein corona studies were carried out using commercially available pooled plasma (i.e. combined from multiple sources) derived from donors with a wide range of health conditions and diseases. Concurrently, the focus of these studies was to delineate the adverse effect of the protein corona on nanoparticle function which included implications in immunogenicity, mistargeting, and unpredictable pharmacokinetics and biodistribution.3,4 Taking an orthogonal view while building on these studies we have introduced the concept of “personalized” and “disease-specific” protein corona,5–7i.e. we demonstrated that exposing nanoparticles to human plasma, obtained from healthy subjects and patients with various diseases, caused considerable differences in the protein corona profile of nanoparticles and their corresponding biological fates.8,9 The effect of the disease on the variation of protein corona has been further validated by various groups.10–15
The possibility to measure panels of specific and selective biomarker proteins has the potential to revolutionize cancer screening, detection and monitoring.16 Among emerging tools, transition metal complexes have recently found use as luminescent probes for the detection of protein biomarkers.17–19 With respect to organic dyes, their long-standing phosphorescence allows them to be distinguished from an auto-fluorescent background that is common in biological milieu. Moreover, as phosphorescence of metal complexes changes with local environment, they can act as chemosensors for a variety of analytes. Other promising approaches for cancer detection and staging are photoacoustic imaging20 and plasmonic biosensing.21
The use of sensor arrays has proven very sensitive, specific, robust, and versatile for the detection of a wide range of chemical and biological compounds, where specificity is derived from the pattern of response among an array of cross-reactive sensors rather than from individual sensors for specific (bio)molecules.22 The sensor array strategy has been used to successfully detect and differentiate among diverse families of analytes,23 various foods and beverages,24 pathogenic bacteria and fungi,25,26 biomolecules,27 and even nanoparticles.28
Here, we combined nanoparticle sensor-array technology, which offers the advantage of improved accuracy while not being limited to known disease biomarkers with protein corona and developed a label-free protein corona sensor array for early detection of diseases (here five different types of cancers were selected as a disease model). The sensor array is composed of three different cross-reactive liposomes with various lipid compositions: (i) anionic liposomes made of DOPG (1,2-dioleoyl-sn-glycero-3-phospho-(1′-rac-glycerol)); (ii) cationic liposomes made of a binary mixture of DOTAP (1,2-dioleoyl-3-trimethylammonium-propane) and DOPE (dioleoylphosphatidylethanolamine); (iii) zwitterionic liposomes made of DOPC (dioleoylphosphatidylcholine) and cholesterol. Protein corona profiles were characterized by nano liquid chromatography tandem mass spectrometry (nano-LC MS/MS) after exposure to the plasma of patients diagnosed with five cancers: lung cancer, glioblastoma, meningioma, myeloma and pancreatic cancer. Although no single protein corona composition is specific for any one cancer type, we demonstrate that changes in the corona composition pattern could provide a unique “fingerprint” for each type of cancer. Finally, the nanoparticle sensor-array technology was validated using cohort plasma obtained from healthy people who were subsequently diagnosed with cancer several years after plasma collection.
Results and discussion
Hard corona profiles of the sensor array elements using plasma derived from patients with cancers at early, intermediate, and advanced stages
The composition of the protein corona that is observed on the surface of sensor array elements (nanoparticles) is strongly dependent on the physicochemical properties of those nanoparticles29 and, at the same time, the protein coronas can strongly be affected by the unique type, concentration and conformation of proteins and other biomolecules present in a given patient plasma.5,6 As an initial proof-of-concept, the size and charge of the corona-coated nanoparticles were probed via dynamic light scattering (DLS/nanosight) and transmission electron microscopy (TEM), after incubation with plasma derived from patients with five different types of cancers (i.e., glioblastoma multiforme, lung cancer, meningioma, multiple myeloma, and pancreatic cancer); (see Tables S1, ESI†) and healthy individuals, and the results demonstrated that the physicochemical properties of the corona-coated nanoparticles varied across different types of cancer (Fig. 1A and B).
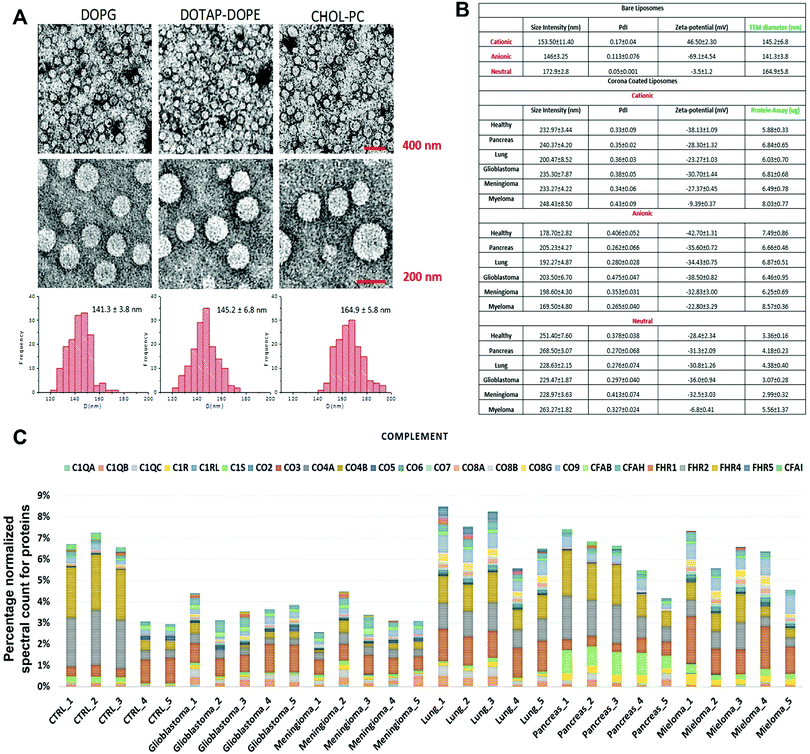 |
| Fig. 1 Protein corona sensor array profiles. (A) TEM images of liposomes with size distribution profiles. (B) Physicochemical properties of different liposomes before and after interactions with human plasma from patients with different diseases. DLS and zeta-potential data on various liposomes before interactions with human plasma and corona complexes (free from excess plasma) obtained following incubation with plasma from healthy subjects and cancer patients (Pdi: polydispersity index from cumulative fitting). (C) Classification of the identified corona proteins from sensor array elements according to their physiological functions in human plasma of healthy individuals and of patients having different types of cancers. (Complement proteins on the surface of cationic liposomes are shown here as an example; other protein categories, including coagulation, tissue leakage, lipoproteins, acute phase, immunoglobulins, and other plasma proteins, are shown in the ESI† Fig. S1A–G). | |
Quantitative evaluation of the total protein adsorbed onto the nanoparticles was performed via the BCA (bicinchoninic acid) or NanoOrange assays, and the results confirmed significant differences in the amounts of adsorbed proteins after incubation in plasma derived from patients with various types of cancers (Fig. 1B). The quantitative evaluation of the total protein adsorbed on the surface of liposomes showed dependency of the protein amount on the cancer type (Fig. 1B). The protein corona composition at the surface of three liposomes was evaluated via liquid chromatography-tandem mass spectrometry (LC-MS/MS) in which the abundance of ∼1800 known proteins was defined (the full raw and analyzed data are provided in Excel files (1–3) in the ESI†). The contribution of individual proteins and their categories (i.e., complement, coagulation, tissue leakage, lipoproteins, acute phase, immunoglobulins, and other plasma proteins) to the corona composition was defined (Fig. 1C and ESI,† Fig. S1A–G). This result demonstrated significant associations between the protein composition and not only the cancer type but also the type of sensor elements (i.e., type of liposome nanoparticles).
According to an extensive body of literature, there are strong relationships between cancer development and variations in protein classes: complement,30–33 coagulation,34–37 tissue leakage,38,39 lipoproteins,40–44 acute phase,45,46 and immunoglobulins.47–50 Therefore, the cross-reactive interactions of these protein categories with nanoparticles may provide unique “fingerprints” for each type of cancer, which would facilitate cancer identification and discrimination. Consequently, one would expect the protein corona sensor array to cross-reactively adsorb a wide range of proteins involved in cancer induction and development that could be used for cancer identification and discrimination. Aside from disease specific proteins, we have recently revealed that the variation of disease related metabolomes in protein solution (e.g., plasma) can substantially change the interaction site of proteins with nanoparticles and can therefore affect protein corona composition.51,52 As cancer development has a capacity to substantially alter the metabolomic composition of plasma,53–58 the cancer extracted plasma can substantially change the protein–nanoparticle interaction sites and therefore alter the protein corona composition.
Development of supervised classification analysis to identify and discriminate among cancers using the protein corona sensor array outcomes
To investigate whether protein corona fingerprints of various sensor elements could be utilized as biosensors and form unique patterns for different diseases, we applied focused classification approaches to proteomic data on three liposomes’ protein corona composition (cationic, anionic, and neutral). Details of the methods are described in the Methods section. A weighted-variable importance in the projection (VIP) score is introduced and applied for ranking of variables based on partial least squares discriminant analysis (PLS-DA) as a linear projection method. Selection of the most relevant variables (protein concentrations) in building the classification model can be guided by a set of obtained ranked variables. In this regard, top ranked variables were added to the model one by one, and the classification error and root mean square error of cross-validation (RMSECV) of the PLS-DA model were monitored. We observed that the classification model has the minimum cross-validation error by using only the top 69 variables (Fig. 2A). The new 69-dimensional variable space was successfully used to discriminate 30 samples (with three replicates) belonging to six classes using PLS-DA with a high classification accuracy (>99%) using 10-fold cross-validation (Fig. 2A). The classification parameters are given in the ESI,† Table S2. The contribution of each single selected protein to the separation of each cancer group (VIP) is plotted on the y-axis (i.e., the VIP value of each variable corresponding to each class) and the x-axis (i.e., the selected variable's number) to provide a visual representation of the relative specificity of the findings (Fig. 2B–F). The proteins with higher VIP scores could be considered the most informative or diagnostic set to discriminate each disease from controls and from among all cancer categories.
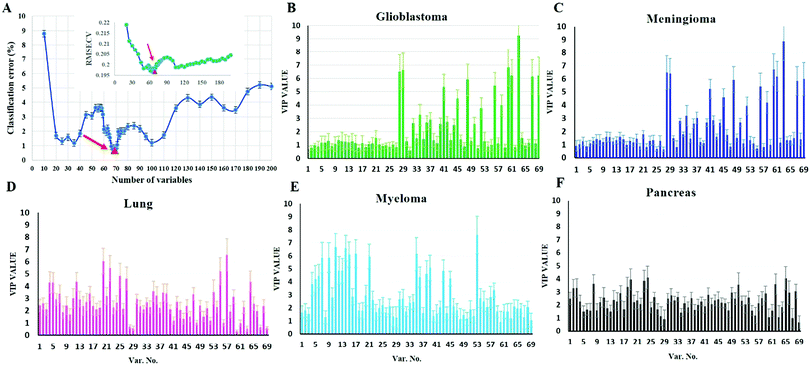 |
| Fig. 2 Predictor discovery and contribution from each individual predictor to separation of each class by PLS discrimination analysis. (A) Predictor exploration by weighted VIP scores was performed by adding the ranked variables to the PLS-DA model one by one and calculating the classification error for internal cross-validation (10-fold). Inset: RMSECV was performed by adding the ranked variables to the PLS-DA model one by one and plotted against a number of variables. Decreasing the classification error and RMSECV led to the discovery of a minimal set of 69 predictors with the highest possible importance for separating each class from the others. (B–F) The contribution of each individual marker to separation of each class based on the PLS discrimination analysis. VIP plot ranking markers of 69 selected variables for their contribution to separation of each class based on the proposed PLS-DA model. VIP score >1 indicates important protein leading to good prediction of class membership, whereas variables with VIP scores <1 indicate unimportant proteins for each class. | |
PLS-DA and the counter-propagation artificial neural network (CPANN) were then applied to the whole samples and selected variables as linear and nonlinear supervised classification approaches, respectively. In agreement with the linear PLS-DA results, the CPANN was also successful in precisely discriminating the six cancers using the selected 69 variables (Fig. 3C and D).
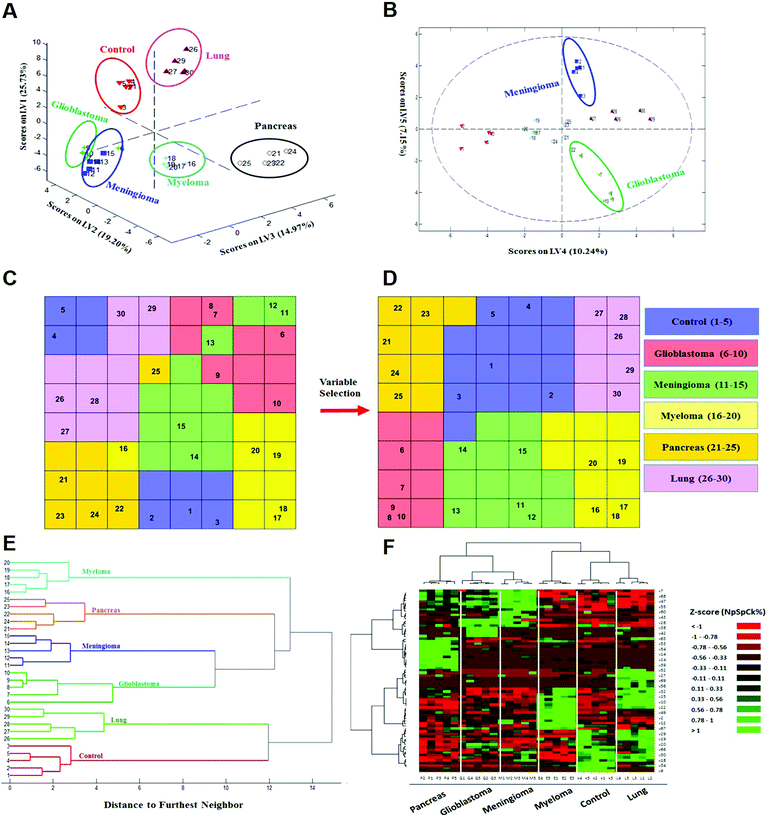 |
| Fig. 3 Identification and discrimination of cancers using protein corona sensor arrays. (A and B) PLS-DA plots showing the separation of different cancerous samples from each other and from controls (n = 30 samples). (A) PLS score-plot obtained using the PLS-toolbox, projecting the objects into the subspace created by the 1st, 2nd, and 3rd latent variables of the model. (B) Objects displayed where the 4th and 5th latent variables of the model are shown. As can be seen, meningioma and glioblastoma cases were not separated in three dimensions appropriately, but they can be separated in the fourth and fifth dimensions of the PLS model. (C and D) Assignation map obtained by using the CPANN with all variables and selected variables. (C) Assignation map obtained by the training of a CPANN network (8 × 8 neurons) using the whole data set (1823 variables). The mapping quality is not good and there are conflicts of different types of cancer in terms of mapping. (D) Assignation map attained by the training of a CPANN network (8 × 8 neurons) using 69 variables. High-dimensional input vectors (samples) are mapped on a two-dimensional network of neurons, preserving similarity and topology. Colors indicate the similarity of a neuron to a specific type of input vector (class type). This map also demonstrates the importance of the predictor selection step and the effect of deletion of non-informative and irrelevant predictors on the model quality. (E and F) 51 proteins identified as capable of distinguishing among the six groups are presented in a ‘Heat Map’ generated using an unsupervised cluster algorithm (agglomerative HCA with furthest neighbor linkage). Visual inspection of both the dendrogram (E) and the heat map (F), based on the raw data of 69 important markers, demonstrates cancer-specific protein corona signature and clear clustering of six groups of samples (five groups of cancerous samples plus normal samples) and also expected similarities among five patients from each group. The heat map also indicates substantial differences in the patterns of variables (markers) of different cancers (each column represents a patient, and each row represents a protein). Higher and lower protein levels are indicated in red and green, respectively; the ID of 69 proteins in the heat map (right y-axis) variables, from top to bottom, are: 7, 1, 68, 8, 47, 36, 55, 37, 60, 48, 43, 50, 28, 51, 38, 3, 42, 58, 63, 46, 53, 31, 54, 17, 14, 44, 24, 21, 39, 40, 52, 5, 27, 11, 69, 65, 56, 57, 32, 16, 15, 13, 10, 26, 22, 62, 49, 6, 2, 41, 12, 45, 67, 59, 29, 4, 19, 64, 20, 33, 66, 61, 30, 23, 18, 35, 34, 25, and 9 (the protein names are provided in Table 1). | |
Next, to further verify and analyze the data, we decided to take advantage of a nonlinear classification and mapping method. Visualizing the feature space can help us understand the hidden structures and topological relationships among the patterns. To reduce the dimensionality of the feature space while preserving the topological relations of the data structure, the CPANN (a supervised a variant of self-organizing maps, SOMs) was used to learn and predict the class membership of the patterns, simultaneously producing a two-dimensional map of “neurons” (the processing units which compete and cooperate to learn the pattern information) and provide valuable information (using a nonlinear approach) about the data structure. Details of the CPANN are provided in the Methods section. Different sizes for the CPANN map were compared using 10-fold cross-validation; a map including 64 (8 × 8) neurons was chosen due to the minimum classification error (ESI,† Fig. S2C). Moreover, the topological structure of data in the high-dimensional space is reflected in the assignation map produced by the CPANN (Fig. 3C). Considering the similarity of the neurons to the input vectors, the map can be partitioned into six distinct zones related to different type of cancers and control samples. Samples with the same class label are mapped onto nearby or the same neurons, which means that the selected variables provide valuable information for discriminating the samples in the feature space. The relative position and orientation of six zones on the map contribute qualitative information on the similarities between types of cancers. To represent the effect of variable selection on the quality of mapping, another CPANN was trained using all 1823 variables, and the resulting map shows that the selected biomarkers (variables) play an important role in discriminating among cancer types and classifying them properly (Fig. 3C and D).
On the basis of the obtained results, both linear and nonlinear models showed high accuracy, deduced from their acceptable specificity, sensitivity, and classification error values. Consistent with these findings, unsupervised clustering (HCA) based on the raw data of 69 markers was able to strongly distinguish various types of cancerous and control samples (Fig. 3E and F). As can be seen in Fig. 3, there is close similarity between the glioblastoma and meningioma groups of samples, implying difficulty in discrimination, most probably related to similar plasma proteomics patterns in these two brain cancers. These results reflect the fact that the plasma concentrations of many proteins in the corona differ considerably, not only among subjects with different types of cancers, but also among healthy individuals.
To illustrate the sensor array's capability for pattern recognition, a set of analyses was performed on the data matrix (all variables) obtained from individual nanoparticles. Importantly, the pattern of cancer-specific fingerprints could not be extracted solely from each class of liposome nanoparticle's PCF (ESI,† Fig. S4). As shown in ESI,† Fig. S4 (ESI†), no one class of liposomes could discriminate all 6 groups of samples as well as the composite response of the full array. The classification error using data obtained individually from anionic, cationic and neutral liposomes is 54%, 24% and 10%, respectively, whereas the combined pattern gave a classification error of only 3%. This substantial reduction in the classification error of the combined pattern is due to the power of the sensory part of the protein corona which provides more proteomics data (even for one specific proteins) for the classifier. Using the nano-sensor array with liposomes that have different chemistries (cationic, anionic, and neutral) combined with pattern-recognition techniques correctly discriminates not only cancerous from control samples, but also each type of cancer under consideration from the others. Notably, 62 proteins out of 69 important variables are unique, because some of the selected proteins are presented in the protein corona profiles of more than one liposome, confirming the key role of those same protein variations [e.g., FCN3 (Ficolin 3)] in different sensor elements. Another specific feature that is presented by using sensor array technology can substantially increase the data dimension of the proteomics outcomes compared to the human plasma proteins. In other words, each protein provides one concentration in human plasma while that specific protein may provide several different concentrations for protein corona profiles of various nanoparticles.
Identification of proteins with crucial roles in cancer detection and discrimination as promising biomarkers for specific types of cancers
The use of biomarkers both before cancer diagnosis (in risk assessment and screening/early detection) and after diagnosis (in monitoring therapy, selecting additional therapy, and detecting recurrence) would yield substantial therapeutic and health-economic benefits.59 To understand the potential biological relevance of the 69 selected proteins that discriminate cancerous samples, we manually searched through previously published reports in PubMed on protein biomarkers of specific types of cancers that are upregulated or downregulated according to different disease stages. The resulting data were compared with the selected proteins in the model to identify matched markers and determine the biological relevance of the proposed model. Interestingly, we noted significant numbers of biomarkers specific to five investigated groups of cancers among the selected predictors that had been reported as specific cancer biomarkers (Table 1).
Table 1 Protein names and biomarkers used for analysis. (A) Protein name and ID of 69 selected variables are listed. Some of the proteins were present in the protein corona of more than one liposome (DOPG, DOTAP and CHOL are denoted by color: green, red, and blue, respectively). (B) Disease-specific biomarkers covered as significant variables by using the proposed models. (C) 8 important markers for cohort samples (the two common variables are colored by red)
After the training of the CPANN, the importance and relevancy of the variables with the produced map can be investigated. A correlation analysis was also performed between the assignation map of the CPANN and 69 weight layers (weight maps) (Fig. 4). Therefore, six correlation coefficients (CCs) can be obtained for each biomarker and these values can show the relevance of that biomarker with the control and cancer classes. The value of a correlation coefficient ranges between −1 and 1 for negative and positive linear correlations respectively. The CC values near to 1 or −1 represent strong correlation and relevancy and a CC value near zero means that there is a weak or non significant correlation between the marker and cancer type. Considering the CC values (ESI,† Table S3), several biomarkers, such as FCN3, CO4A, CO4B, CO7, and C4BPA, can easily be distinguished according to the strong correlation between pancreatic cancer zones on the assignation map and also reported as pancreatic cancer biomarkers in the literature.60–62 Moreover, for lung cancer APOH, CO6, CO8A, CO8G, KNG1, and VTNC have significant correlation with the CPANN assignation map as specific biomarkers.63
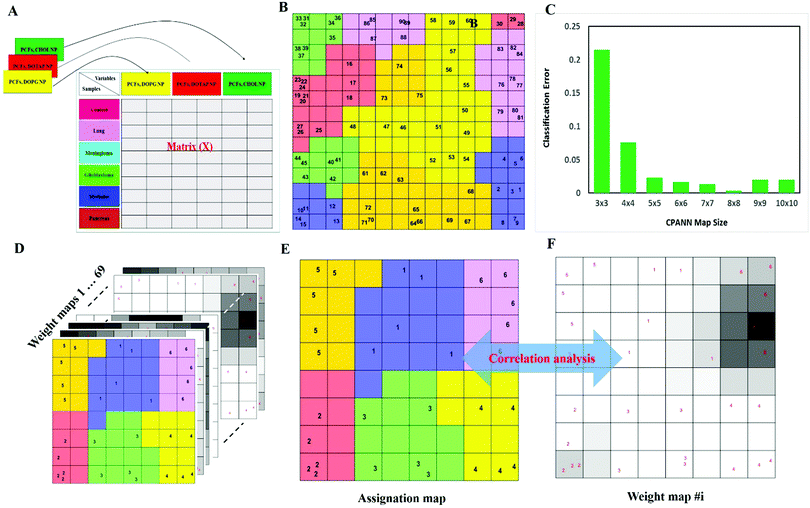 |
| Fig. 4 Data analysis using the CPANN (counter propagation artificial neural network). (A) Schematic representation of unfolding a three-way data matrix into a two-way matrix. (B) Assignation map obtained by the CPANN (14 × 14) trained using 30 samples (three replicates for each samples) with all 1823 variables. Sample numbers are indicated on each neuron. The neuron color (assigned label) is decided based on the similarity between the class label (a 6 × 1 binary vector) and the weight vector in the output layer of the corresponding neuron. Despite using all biomarkers, there are some distinct similarities between samples of the same cancer class. Replicated samples are also mapped on adjacent or the same neurons. (C) Classification error of the CPANN map was calculated at different map size by 10-fold cross validation. (D) The CPANN network has 69 weight layers, which is equal to the number of variables used to train the model. The ith weight layer reflects the effect of the ith variable (biomarker) on the pattern of the assignation map. (E and F) Correlation of the assignation map and 69 weight layers (weight maps) can be calculated which could help identify the biomarkers related to each cancer class. Similarity can be monitored by absolute values of correlation coefficients of two maps. For example, the weight map of biomarker 1282 is highly correlated with the pattern of cancer class 4 on the assignation map, and it could possibly be an important biomarker for the samples obtained from patients with myeloma. Similar inference can be made for the importance of other cancer biomarkers. | |
The high specificity of the selected markers for discriminating among the five groups of cancers, which derives from our protein corona sensor array approach, demonstrates an acceptable level of correlation with the work now under way in the complex cancer proteomics space; therefore, this strategy not only provides a basis for cancer prediction but also translates that promise into reality. It is noteworthy that the discrimination between different cancer groups occurs as a result of the pattern of response of several predictors (and not individual biomarkers) that change simultaneously in a systematic manner, forming patterns unique to each specific type of cancer. On the basis of this evidence, the most informative predictors selected by the proposed model that have not already been reported as cancer-specific biomarkers may have great potential as new diagnosis biomarker candidates. It is noteworthy that the protein corona layer provides different protein concentration compared to the plasma proteins. This means that increasing concentration of cancer specific biomarkers in plasma may not lead to higher participation of that specific protein in the corona composition. However, variation of these cancer specific proteins together with other metabolomic variations may substantially change the interactions of other proteins with the surface of nanoparticles which results in the formation of disease-specific protein corona. To define the role of corona specific proteins in cancer development, the variation and functionality of these promising candidates together with their associated metabolomic pathways in cancer patients should be carefully monitored. By focusing on the unique patterns derived from huge numbers of subjects via a set of informative predictors, researchers should be able to predict cancers at different stages more accurately which is not possible using current methods.
Cohort data analysis
To probe the capacity of this protein corona sensor array technology for early detection of cancers, we used cohort plasma from healthy people who were subsequently diagnosed several years after plasma collection with one of the five types of cancers. Using the cohort samples, we evaluated whether our proposed models, both linear and nonlinear, with 69 selected predictors could be utilized for cancer prediction. The protein corona profiles of the cohort samples are presented in the ESI† (the full raw and analyzed data are provided in four Excel files (4–6) in the ESI†). There were statistically significant differences between protein corona profiles of the cohort samples and previous fresh samples in terms of protein abundance levels and protein types when the proteomics profiles of these two groups of samples were compared. Since sample collection of the cohort samples was at the time of screening of healthy individuals, they were stored frozen for at least ten years. The long-term storage time affects the abundance levels of several proteins, causing decreases or increases in protein concentrations such as coagulation factors, as reported by several groups.64,65 Therefore, we attribute the lack of significant correlation of the cohort results with previous fresh samples that have not been stored for a long time to alteration in protein abundance due to the aging of the samples.
To allow for unbiased classification and prediction of cohort samples, we used two approaches: first, the discriminatory power of the 69 important variables was checked for the cohort samples. Because 15 variables (proteins) out of the 69 markers were absent from the proteomics profile of our protein corona sensor array of cohort samples, classification was performed based on the 54 existing markers and the amount of 15 absent variables in the cohort data matrix was considered zero. Despite such defects and missing markers in the cohort data matrix, both linear and nonlinear models provided proper separation for three groups of cohort samples with reasonable statistics (38% classification error in 10-fold cross validation) (Fig. 5A and C). Second, the cohort samples were classified separately, i.e., not compared with the library of the protein corona sensor array for previous fresh samples. In this regard, the informative markers were selected based on the cohort protein corona profiles in a similar manner as mentioned earlier, and then linear and nonlinear classification approaches were evaluated. Interestingly, the cohort samples could be discriminated by employing both linear and nonlinear classification models using only 8 markers with excellent statistics (the classification error minimized to zero using 8 variables). All detailed results are provided in the ESI,† Table S2, and Fig. 5B and D. As shown in Fig. 5, the cohort samples were significantly discriminated in the score plot of both PLS-DA and the CPANN map.
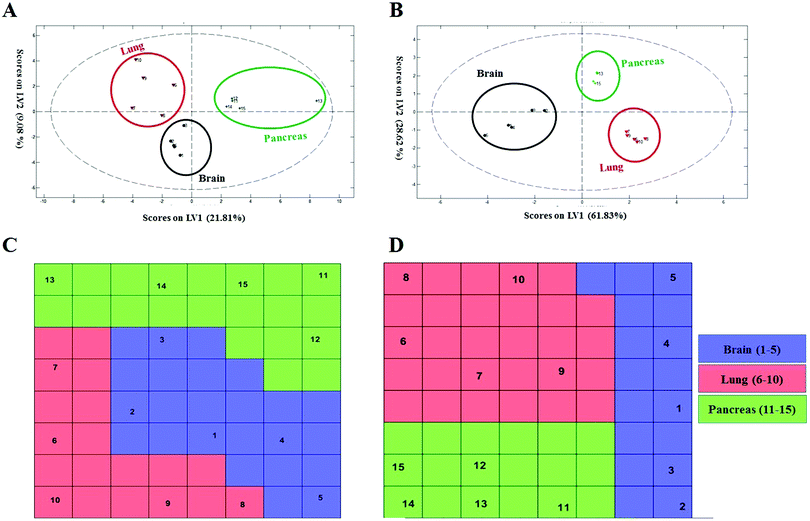 |
| Fig. 5 Classification of cohort samples based on 69 and 8 markers using the linear and nonlinear classification models. (A and B) Discrimination of cohort samples using PLS-DA plots. (A) PLS score-plot obtained by considering 69 important markers, projecting the cohort objects into the subspace created by the 1st and 2nd latent variables of the model. (B) The PLS-DA model is generated using 8 variables projecting the cohort objects into the subspace created by the 1st and 2nd latent variables of the model, using excellent statistics. (C and D) Assignation map obtained by the CPANN with 69 and 8 selected variables. (C) Assignation map obtained by training a CPANN network (8 × 8 neurons) using 69 important markers. (D) Assignation map obtained by training a CPANN network (8 × 8 neurons) using only 8 markers without any misclassifications. Sample numbers are indicated on each neuron. | |
In summary, we have developed a disease-specific protein corona sensor array platform for disease detection using plasma samples. Our sensor array differs from other known sensor arrays that involve individual sensors that detect specific biomolecules. In the present sensor array, the biomolecules do not have to be known, as the system does not rely on the presence or absence of specific biomolecules or amounts of specific disease (here, cancer) markers. This new sensor array detects changes in the composition of the biomolecule coronas associated with different liposome nanoparticle sensor elements. This ability to detect changes in the patterns of the biomolecule corona composition associated with each sensor element allows one to determine a unique biomolecule fingerprint that can differentiate the health or disease states of subjects with high accuracy. As we demonstrated very recently,51,66 variation of other plasma biomolecules (e.g., metabolomes) can substantially change the protein corona composition. This shows that the patterns presented by disease-specific protein coronas should not solely be composed of disease biomarkers, as other disease-specific features (e.g., metabolome variations) can substantially affect the composition of corona around nanoparticles. Using partial least squares discriminant analysis, we were able to discriminate among five cancers and healthy patients with >99% accuracy (n = 90). Results of the cohort samples revealed that the biomolecular fingerprint can even determine a pre-disease state in a subject who will develop one of three cancers at a later time, with an accuracy of >99% (n = 45). This is a significantly different approach to diagnosis compared to systems that detect specific biomarkers associated with a disease or disorder. The present sensor is able to detect a disease early in its development; in other words, it can pre-diagnose the disease before any specific symptoms appear. It is likely that the sensitivity of the protein corona sensor array can further be increased by the addition of more sensor elements (more nanoparticles). It is also obvious that the number and score of the introduced protein patterns for cancer detection in this feasibility study will be changed (and would be more robust) by increasing the numbers/types of patients and/or sensor array elements. It is also noteworthy that this system needs a huge number of patient plasmas in order to end up with ∼0% false negative results as any false disease prediction may cause huge anxiety and unnecessary medical procedures for patients. Beside cancers, the protein corona sensor array may also prove useful for the diagnosis of a wide range of other devastating diseases, where very early detection can significantly improve patients’ survival and quality of life.
Methods
Liposomes
Cholesterol (Chol) was purchased from Sigma Aldrich (St. Louis, MO, USA). DOPC (dioleoylphosphatidylcholine), DOPE (dioleoylphosphatidylethanolamine), DOPG (1,2-dioleoyl-sn-glycero-3-phospho-(1′-rac-glycerol)), and DOTAP (1,2-dioleoyl-3-trimethylammonium-propane) were purchased from Avanti Polar Lipids (Alabaster, AL, USA). Three types of liposomes, labelled DOPG, DOTAP, and CHOL (cationic, anionic, and neutral, respectively), were prepared from DOPG, DOTAP–DOPE (1
:
1 molar ratio), and DOPC–Chol (1
:
1 molar ratio) by dissolving appropriate amounts of the lipids 9
:
1 (v/v) in chloroform:methanol. The chloroform:methanol mixture was evaporated via rotary-evaporation. Lipid films were kept under vacuum overnight and hydrated with 10 mmol l−1 phosphate saline buffer (PBS) (pH 7.4) to a final lipid concentration of 1 mg ml−1. The liposome suspensions obtained were sized by extrusion using a 50 nm polycarbonate carbonate filter by employing an Avanti Mini-Extruder (Avanti Polar Lipids, Alabaster, AL).
Human plasma collection, preparation, and storage
Human plasma (HP) was collected from healthy subjects and cancer patients diagnosed with glioblastoma multiforme, lung cancer, meningioma, myeloma, or pancreatic cancer. Blood sample collection was performed according to the European Directive 2001/20/EC concerning the implementation of good clinical practice in the conduct of clinical trials that is operative in Italy (Decreto legislativo 24 giugno 2003, n. 211). Blood collection from cancer patients was authorized by: the Ethical Committee of Sapienza University of Rome (myeloma), the Ethical Committee of the University of Napoli Federico II (lung cancer), the Azienda Ospedaliera Universitaria Federico II U.O.C. di Neurochirurgia (meningioma and glioblastoma multiforme) and the Ethical Committee of University Campus Bio-Medico di Roma (pancreatic cancer and healthy volunteers). Informed consent was obtained from each participant. In brief, blood was collected by venipuncture of healthy subjects and cancer patients by means of a BD P100 Blood Collection System (Franklin Lakes, NJ, USA) with push-button technology that reduces blood waste while minimizing the risk of contamination. Samples were centrifuged at 1000 × g for 5 min to pellet the blood cells, and the plasma was collected. After confirming the absence of hemolysis, plasma collected from each donor (1 ml) was split into 200 μl aliquots and stored at −80 °C in labeled Protein LoBind tubes until use. Plasma samples collected from cancer patients were stored at −80 °C for no longer than two months before use; these plasmas are called “fresh plasma” in the text. For analysis, the aliquots were thawed at 4 °C and then allowed to warm at room temperature (RT).
Cohort plasma samples
We used human plasma from initially healthy individuals who were subsequently diagnosed with brain, lung, or pancreatic cancers within eight years after plasma collection. The plasma samples were collected through the NIH-funded Golestan Cohort Study, performed by the National Cancer Institute (NCI) in the USA, the International Agency for Research on Cancer (IARC) in France, and the Tehran University of Medical Sciences (TUMS) in Iran. This study involved the collection and storage of plasma from 50
000 healthy subjects, over 1000 of whom went on to develop various types of cancers in subsequent years. Samples from five individuals per cancer were used in this study.67 These important plasma samples provide us the unique opportunity to probe the capacity of our innovative protein corona sensor array for early detection of cancers.
Transmission electron microscopy (TEM)
Liposome formulations have been characterized by TEM as reported previously.68 Briefly, 10 μl of each sample has been deposited onto Formvar-coated grids, negatively stained using 1% uranyl acetate, washed with ultrapure water and air-dried. Measurements have been performed with a Zeiss Libra 120 instrument, and image analysis was performed using ImageJ software.69
Size and zeta-potential
Bare liposomes were incubated with HP (1
:
1 v/v) for 1 hour at 37 °C. Subsequently samples were centrifuged at 14
000 rpm for 15 minutes at 4 °C to pellet liposome–HP complexes. The resulting pellet was washed three times with phosphate-buffered saline (PBS) and resuspended in ultrapure water. For size and zeta-potential measurements, 10 μl of each sample was diluted with 990 μl of distilled water. All size and zeta-potential measurements were performed at RT using a Zetasizer Nano ZS90 system (Malvern, UK) equipped with a 5 mW HeNe laser (wavelength λ = 632.8 nm) and a digital logarithmic correlator. The particle diffusion coefficient D distribution is derived from a deconvolution of the measured intensity autocorrelation function of the sample. D is converted into an effective hydro-dynamic radius RH by using the Stokes–Einstein equation (RH = kBT/6πηD), where kBT is the thermal energy and η is the solvent viscosity. Electrophoretic mobility of the samples, u, was measured via laser Doppler electrophoresis. Zeta-potential was calculated by using the Smoluchowski relation (zeta potential = uη/ε) where η and ε are the viscosity and the permittivity of the solvent phase, respectively. Size and zeta-potential of liposome–HP complexes are given as mean ± standard deviation (S.D.) of five independent measurements.
Protein assay
Liposome formulations were incubated with HP (1
:
1 v/v) for 1 hour at 37 °C. Afterwards, liposome–HP complexes were pelleted at 15
000 × g for 15 minutes at 4 °C and washed three times with PBS. The washed pellet was resuspended in urea 8 mol l−1, NH4CO3 50 mmol l−1. 10 microliters of each sample were added to five wells of a 96-well plate. Protein quantification was performed by adding 150 microliters per well of protein assay reagents (Pierce, Thermo Scientific, Waltham, MA, USA). The multiwell was shaken and incubated at room temperature for 5 minutes. Absorbance was measured using the GloMax Discover System (Promega, Madison, WI, USA) at 660 nm. Background effects were properly corrected, and the protein concentration was calculated using the standard curve. Results are given as mean ± S.D. of five independent replicates.
Protein identification and quantification
The incubation procedure was performed as described elsewhere.70 250 μl of liposome formulations (1 mg ml−1) were incubated with HP (1
:
1 v/v) for 1 hour at 37 °C. Samples were centrifuged at 14
000 × g for 15 min to pellet liposome–HP complexes. It is noteworthy that while bare liposomes cannot be collected via centrifugation at 14
000 × g, the formation of protein corona at the surface of liposomes changes their physicochemical properties which can be collected at this centrifugation rate.8,71–83 The pellet was washed three times with 10 mmol l−1 Tris HCl (pH 7.4), 150 mmol l−1 NaCl, and 1 mmol l−1 EDTA. After washing, the pellet was air dried and resuspended in the digestion buffer. Digestion and peptide desalting were carried out as previously described.84 In brief, the pellet was resuspended in 40 μl of 8 mol L−1 urea, and 50 mmol l−1 NH4HCO3 (pH = 7.8). Afterwards, the protein solution was reduced with 2 μl of 200 mmol l−1 DTT, alkylated with 8 μl of 200 mmol l−1 IAA and newly added with 8 μl of 200 mmol l−1 DTT. Finally, the sample solution was diluted with 50 mmol l−1 NH4HCO3 to obtain a final urea concentration of 1 mol L−1 and digested overnight with 2 μg of trypsin at 37 °C. The enzymatic reaction was stopped by adding TFA. The digested peptides were desalted using the SPE C18 column, reconstituted with a suitable volume of a 0.1% formic acid solution, and stored at −80 °C until analysis. Digested peptides were stored at −80 °C in labeled Protein LoBind tubes for no more than one week. Digested peptides were analyzed by nano-high-performance liquid chromatography (HPLC) coupled to tandem mass spectrometry (MS/MS). NanoHPLC MS/MS analysis was carried out using a Dionex Ultimate 3000 system (Dionex Corporation Sunnyvale, CA, USA) directly connected to a hybrid linear ion trap-Orbitrap mass spectrometer (Orbitrap LTQ-XL, Thermo Scientific, Bremen, Germany) using a nanoelectrospray ion source. Peptide mixtures were enriched on a 300 μm ID × 5 mm Acclaim PepMap 100 C18 precolumn (Dionex Corporation Sunnyvale, CA, USA), employing a premixed mobile phase made up of ddH2O/ACN, 98/2 (v/v) containing 0.1% (v/v) HCOOH, at a flow-rate of 10 μl min−1. Peptide mixtures were then separated via reversed-phase (RP) chromatography. The largest set of peptides was detected using a 3 hour optimized LC gradient composed of mobile phase A of ddH2O/HCOOH (99.9/0.1, v/v) and mobile phase B of ACN/HCOOH (99.9/0.1, v/v). MS spectra of eluting peptides were collected over an m/z range of 350–1700 using a resolution setting of 60
000 (full width at half-maximum at m/z 400), operating in the data-dependent mode. MS/MS spectra were collected for the five most abundant ions in each MS scan. Further details can be found elsewhere.84 For each experimental condition, three independent samples (biological replicates) were prepared, each of which was measured in triplicate (technical replicates), yielding nine measurements for each experimental condition. RAW data files were submitted to Mascot (v1.3, Matrix Science, London, UK) using the Thermo-Finnigan LCQ/DECA RAW file data import filter to perform database searches against the non-redundant Swiss-Prot database (09-2014, 546
000 sequences, Homo Sapiens taxonomy restriction). For the database search, trypsin was specified as the proteolytic enzyme with a maximum of two missed cleavages. Carbamidomethylation was set as the fixed modification of cysteine, whereas oxidation of methionine was chosen as the variable modification. The monoisotopic mass tolerance for precursor ions and fragmentation ions was set to 10 ppm and 0.8 Da, respectively. Charge state of 2+ or 3+ was selected as precursor ions. Proteome output files were submitted to the commercial software Scaffold (v3.6, Proteome Software, Portland, Oregon, USA). Peptide identifications were validated if they surpassed a 95% probability threshold set by the PeptideproPhet algorithm. Protein identifications were accepted if they could be established at >99.0% probability and contained at least two unique peptides. Proteins that contained shared peptides and could not be differentiated on the basis of MS/MS analysis alone were grouped to satisfy the principles of parsimony. Unweighted spectrum counts (USCs) were used to assess the consistency of biological replicates in quantitative analysis, and normalized spectrum counts (NSCs) were used to retrieve protein abundance.
Statistical analysis
All statistical analyses were performed using PLS, Kohonen, and CPANN toolboxes, and graphs were created using Microsoft Excel, XLSTAT, and MATLAB.
Data matrix
Data matrix X (30 × 1823) was generated such that each row of the predictor matrix relating to each individual is derived from all proteins' abundance obtained from the three-protein corona sensor array (ESI,† Fig. S2A). In the preprocessing step, the normalized data in matrix X, relative protein abundance (RPA), were auto-scaled.
Classification and clustering
Partial least squares discriminant analysis (PLS-DA).
Partial least squares discriminant analysis is a well-known multivariate approach regarded as a linear classification and dimension reduction method consisting of two main parts: a structural part, which searches for latent variables as linear combinations of original independent variables (i.e., data matrix X), which have the maximum covariance with the corresponding dependent-variables (i.e., class membership, Y);85,86 the second part is composed of measured components including the latent variables as scores and loadings, which show how the latent variables and the original variables are related. Based on the ability of PLS-DA to reduce the dimensionality of the data, it allows a linear mapping and graphical visualization of the different data patterns. PLS-DA is particularly well suited to deal with highly collinear and noisy patterns. The main problems associated with the large dataset in proteomics are the large number of monitored variables (i.e., proteins) and a relatively small number of samples. Hence, there may be a high redundancy among variables, which renders many of them uninformative and irrelevant to the classification. In this way, eliminating uninformative variables or finding new uncorrelated ones may improve the predictive performance of classification. Since in biomedical applications, such as in the present work, we must not only make decisions about whether a sample belongs to one of a number of known groups, but also determine which variables are most relevant for the best discrimination between classes, a method like PLS-DA is a good approach for finding uncorrelated new latent variables while preserving the variation of the data.87 The importance of the original variables to define latent projections can also be evaluated by the variable importance in the projection (VIP) analysis, in which PLS methods can play a significant role in the selection of a subset of discriminative features.88,89 Moreover, the optimal number of latent variables (LV) was obtained using 10-fold cross validation outcomes.
Identifying the most relevant variables based on weighted VIP.
The partial least squares discriminant analysis (PLS-DA) was used to explore the VIP values associated with variables. VIP is a combined measure of how much a variable contributes to a description of the two sets of data: the dependent (Y) and the independent variables (X). The weights in a PLS model reflect the covariance between the independent and dependent variables, and the inclusion of the weights allows VIP to reflect not only how well the dependent variable is described but also how important that information is for the model of the independent variables.90
An approach based on the VIP score was developed to identify the best subset of variables. VIP scores can be calculated by performing PLS-DA on the dataset. In that approach, VIP scores of variables are calculated 50 times, each time using a random permutation of training and validation sets (random training sets were selected iteratively by considering 80-percent coverage of each class of objects). Considering the most important variables, the large VIP-score values (>2), the top 200 variables can be selected at each repetition and added to the top-variables pool. Afterward, a frequency of occurrence (Freqi) and an average VIP-score
for each variable can be obtained according to the top-variable pool. Thus, the selection of variable i (the high
value and the low
value) is less recommended than variable j (high values for both VIPj and
) because the selection of variable i is more dependent on the training and validation sets than variable j. Therefore, the
value of each variable can be weighted by Freqi, and the most relevant variables can be ranked using weighted
. Fig. 4A is a schematic diagram of the proposed approach. Selection of the most relevant variables to build the classification model can be guided by the obtained ranking as follows: the highly ranked variables were added one by one to the dataset, and the classification error of PLS-DA was calculated to find the minimum number of relevant predictors (Fig. 1A).
Counter-propagation artificial neural network (CPANN)
The counter-propagation artificial neural network (CPANN) is a supervised variant of the self-organizing map that consists of two layers of neurons arranged on a predefined N × N grid. The CPANN can be used to map data from a high-dimensional feature space to a low-dimensional (typically 2) discrete space of neurons as well as to predict the class membership of the unknown samples. The input vectors (sample feature vectors) and corresponding class membership vectors (a binary vector) are presented to the input and output layer of the CPANN, respectively. The weight correction of the neurons in both layers is performed based on competitive learning and cooperation of the neurons.91,92 Hence, similar input vectors can be mapped on the same or adjacent neurons and vice versa. The final assignation map properly reveals the structure of the data in the feature space and preserves the distance of patterns in the low-dimensional grid of neurons. ESI,† Fig. S3C shows a high-quality assignation map of the CPANN using top-ranked biomarkers. According to the distinct regions for each class, the risk of classification errors is minimized. The proper size of the map can be decided by performing 10-fold cross-validation at different map sizes. The trained CPANN can be used to assign a class membership to an unlabeled sample.85 The presence of redundant and uninformative variables in training data will affect the quality of the map and increase the risk of an error of classification (Fig. 3C). The process is a nonlinear mapping, which helps visualize a high-dimensional input object on a two-dimensional neuron grid. It is a self-organized procedure which solves the issue of classification in a transparent way. More details about the CPANN method can be found in the following references.85,93
Hierarchical clustering analysis (HCA).
Hierarchical clustering analysis is an unsupervised method widely used to explore and visualize whole heterogeneous large data sets (like those often used in proteomics) into distinct and homogeneous clusters. In cluster analysis, to identify homogeneous sub-groups, the two important concepts of similarity (determining a numerical value for the similarity between objects and constructing a similarity matrix) and linkages (connection of an object to a group or not) should be defined.94,95 Herein, we applied agglomerative hierarchical clustering using the furthest-neighbor linkage algorithm based on the Euclidean distance similarity for unsupervised analysis using the selected variables. The agglomerative procedure first separates each object into its own individual cluster and then combines the clusters sequentially; similar objects or clusters are merged until every object belongs to only one cluster.
Cohort sample prediction.
The discriminative ability of 69 selected predictors with both linear and nonlinear classification approaches was assessed by cohort sample analysis. To this end, the values related to 69 variables were selected and discrimination of cohort samples was checked by both PLS-DA and the CPANN. Notably, 15 variables (proteins), out of the 69 variables, were absent from the proteomics profile of protein corona sensor arrays of cohort samples; therefore, their amount in the cohort data matrix was zero. Next, the cohort samples were classified separately without involving the library of the protein corona sensor arrays of previous fresh samples. In this regard, the informative markers were selected based on the cohort protein corona profiles in a similar manner as mentioned earlier, and then linear and nonlinear classification approaches were evaluated. Interestingly, the cohort samples could be discriminated by using both linear and nonlinear classification models using only 8 markers with excellent statistics (zero classification errors).
Conflicts of interest
There are no conflicts to declare.
Acknowledgements
The research leading to these results has received funding from AIRC under IG 2017 – ID. 20327 project – P. I. Caracciolo Giulio and the Tehran University of Medical Sciences (TUMS), grant: Internal Grant (2014-2015).
References
- C. Salvador-Morales, L. Zhang, R. Langer and O. C. Farokhzad, Biomaterials, 2009, 30, 2231–2240 CrossRef CAS PubMed.
- M. P. Monopoli, C. Åberg, A. Salvati and K. A. Dawson, Nat. Nanotechnol., 2012, 7, 779 CrossRef CAS PubMed.
- M. Mahmoudi, Trends Biotechnol., 2018, 36, 755–769 CrossRef CAS PubMed.
- M. Mahmoudi, Nat. Nanotechnol., 2018, 13, 775 CrossRef CAS PubMed.
- M. J. Hajipour, S. Laurent, A. Aghaie, F. Rezaee and M. Mahmoudi, Biomater. Sci., 2014, 2, 1210–1221 RSC.
- M. J. Hajipour, J. Raheb, O. Akhavan, S. Arjmand, O. Mashinchian, M. Rahman, M. Abdolahad, V. Serpooshan, S. Laurent and M. Mahmoudi, Nanoscale, 2015, 7, 8978–8994 RSC.
- M. Rahman and M. Mahmoudi, Proc. SPIE, 2015, 9338, 93380V CrossRef.
- D. Caputo, M. Papi, R. Coppola, S. Palchetti, L. Digiacomo, G. Caracciolo and D. Pozzi, Nanoscale, 2017, 9, 349–354 RSC.
- D. Caputo, M. Cartillone, C. Cascone, D. Pozzi, L. Digiacomo, S. Palchetti, G. Caracciolo and R. Coppola, Pancreatology, 2018, 18, 661–665 CrossRef CAS PubMed.
- M. Hadjidemetriou, Z. Al-Ahmady, M. Buggio, J. Swift and K. Kostarelos, Biomaterials, 2019, 188, 118–129 CrossRef CAS PubMed.
- M. Hadjidemetriou, S. McAdam, G. Garner, C. Thackeray, D. Knight, D. Smith, Z. Al-Ahmady, M. Mazza, J. Rogan and A. Clamp, Adv. Mater., 2018, 1803335 Search PubMed.
- B. A. Aguado, J. C. Grim, A. M. Rosales, J. J. Watson-Capps and K. S. Anseth, Sci. Transl. Med., 2018, 10, eaam8645 CrossRef PubMed.
- J. Lazarovits, Y. Y. Chen, F. Song, W. Ngo, A. J. Tavares, Y.-N. Zhang, J. Audet, B. Tang, Q. Lin and M. C. Tleugabulova, Nano Lett., 2018, 19, 116–123 CrossRef PubMed.
- M. Papi and G. Caracciolo, Nano Today, 2018, 21, 14–17 CrossRef CAS.
- G. Caracciolo, D. Caputo, D. Pozzi, V. Colapicchioni and R. Coppola, Colloids Surf., B, 2014, 123, 673–678 CrossRef CAS PubMed.
- R. M. Sallam, Dis. Markers, 2015, 2015, 321370 Search PubMed.
- D.-L. Ma, S. Lin, W. Wang, C. Yang and C.-H. Leung, Chem. Sci., 2017, 8, 878–889 RSC.
- K. Vellaisamy, G. Li, C.-N. Ko, H.-J. Zhong, S. Fatima, H.-Y. Kwan, C.-Y. Wong, W.-J. Kwong, W. Tan and C.-H. Leung, Chem. Sci., 2018, 9, 1119–1125 RSC.
- D.-L. Ma, M. Wang, C. Liu, X. Miao, T.-S. Kang and C.-H. Leung, Coord. Chem. Rev., 2016, 324, 90–105 CrossRef CAS.
- H. J. Knox and J. Chan, Acc. Chem. Res., 2018, 51, 2897–2905 CrossRef CAS PubMed.
- A. Belushkin, F. Yesilkoy and H. Altug, ACS Nano, 2018, 12, 4453–4461 CrossRef CAS PubMed.
- J. R. Askim, M. Mahmoudi and K. S. Suslick, Chem. Soc. Rev., 2013, 42, 8649–8682 RSC.
- S. H. Lim, L. Feng, J. W. Kemling, C. J. Musto and K. S. Suslick, Nat. Chem., 2009, 1, 562–567 CrossRef CAS PubMed.
- B. A. Suslick, L. Feng and K. S. Suslick, Anal. Chem., 2010, 82, 2067–2073 CrossRef CAS PubMed.
- J. R. Carey, K. S. Suslick, K. I. Hulkower, J. A. Imlay, K. R. Imlay, C. K. Ingison, J. B. Ponder, A. Sen and A. E. Wittrig, J. Am. Chem. Soc., 2011, 133, 7571–7576 CrossRef CAS PubMed.
- Y. Zhang, J. R. Askim, W. Zhong, P. Orlean and K. S. Suslick, Analyst, 2014, 139, 1922–1928 RSC.
- F. Ghasemi, M. R. Hormozi-Nezhad and M. Mahmoudi, Anal. Chim. Acta, 2016, 917, 85–92 CrossRef CAS PubMed.
- M. Mahmoudi, S. Lohse, C. J. Murphy and K. S. Suslick, ACS Sens., 2016, 1, 17–21 CrossRef CAS.
- G. Caracciolo, O. C. Farokhzad and M. Mahmoudi, Trends Biotechnol., 2017, 35(5), 257–264 CrossRef CAS PubMed.
- R. Pio, D. Ajona and J. D. Lambris, Semin. Immunol., 2013, 25, 54–64 CrossRef CAS PubMed.
-
R. Pio, L. Corrales and J. D. Lambris, Tumor microenvironment and cellular stress, Springer, 2014, pp. 229–262 Search PubMed.
- S. Ostrand-Rosenberg, Nat. Biotechnol., 2008, 26, 1348 CrossRef CAS PubMed.
- M. Korbelik and P. Cooper, Br. J. Cancer, 2007, 96, 67–72 CrossRef CAS PubMed.
- M. Shoji, W. W. Hancock, K. Abe, C. Micko, K. A. Casper, R. M. Baine, J. N. Wilcox, I. Danave, D. L. Dillehay and E. Matthews, Am. J. Pathol., 1998, 152, 399 CAS.
- C. Huggins, G. M. Miller and E. V. Jensen, Cancer Res., 1949, 9, 177–182 CAS.
- O. Bodansky and G. F. McInnes, Cancer, 1950, 3, 1–14 CrossRef CAS PubMed.
- J. I. Zwicker, B. C. Furie and B. Furie, Crit. Rev. Oncol. Hematol., 2007, 62, 126–136 CrossRef PubMed.
- S. Pan, T. A. Brentnall and R. Chen, Proteomics, 2015, 15, 2705–2715 CrossRef CAS PubMed.
- K. A. Semb, S. Aamdal and P. Oian, J. Clin. Oncol., 1998, 16, 3426–3432 CrossRef CAS PubMed.
- C. Alexopoulos, S. Pournaras, M. Vaslamatzis, A. Avgerinos and S. Raptis, Cancer Chemother. Pharmacol., 1992, 30, 412–416 CrossRef CAS PubMed.
- S. Muntoni, L. Atzori, R. Mereu, G. Satta, M. D. Macis, M. Congia, A. Tedde and A. Desogus, Nutr., Metab. Cardiovasc. Dis., 2009, 19, 218–225 CrossRef CAS PubMed.
- C. Alexopoulos, B. Blatsios and A. Avgerinos, Cancer, 1987, 60, 3065–3070 CrossRef CAS PubMed.
- K. Hasija and H. K. Bagga, Indian J. Clin. Biochem., 2005, 20, 61–66 CrossRef CAS PubMed.
- E. B. Feldman and A. C. Carter, J. Clin. Endocrinol. Metab., 1971, 33, 8–13 CrossRef CAS PubMed.
-
W. S. Orr, J. A. Sandoval, L. H. Malkas and R. J. Hickey, Acute Phase Proteins as Cancer Biomarkers, INTECH Open Access Publisher, 2011 Search PubMed.
- W. W. Pang, P. S. Abdul-Rahman, W. Izlina Wan-Ibrahim and O. Haji Hashim, Int. J. Biol. Markers, 2010, 25, 1 CrossRef CAS PubMed.
- S. E. Senyo, M. L. Steinhauser, C. L. Pizzimenti, V. K. Yang, L. Cai, M. Wang, T.-D. Wu, J.-L. Guerquin-Kern, C. P. Lechene and R. T. Lee, Nature, 2013, 493, 433–436 CrossRef CAS PubMed.
- J. Wang, D. Lin, H. Peng, Y. Huang, J. Huang and J. Gu, Cell Death Dis., 2013, 4, e945 CrossRef CAS PubMed.
- H. P. Vollmers and S. Brändlein, N. Biotechnol., 2009, 25(5), 294–298 CrossRef CAS PubMed.
- G. Lee, J. Clin. Cell. Immunol., 2014, 5(2) DOI:10.4172/2155-9899.1000200.
- M. Tavakol, A. Montazeri, R. Naghdabadi, M. J. Hajipour, S. Zanganeh, G. Caracciolo and M. Mahmoudi, Nanoscale, 2018, 10, 7108–7115 RSC.
- S. Palchetti, L. Digiacomo, D. Pozzi, R. Zenezini Chiozzi, A. L. Capriotti, A. Laganà, R. Coppola, D. Caputo, M. Sharifzadeh and M. Mahmoudi, Adv. Biosyst., 2019, 3, 1800221 CrossRef.
- S. Nishiumi, M. Shinohara, A. Ikeda, T. Yoshie, N. Hatano, S. Kakuyama, S. Mizuno, T. Sanuki, H. Kutsumi and E. Fukusaki, Metabolomics, 2010, 6, 518–528 CrossRef CAS.
- Y. S. Kim, P. Maruvada and J. A. Milner, Future Oncol., 2008, 4(1), 93–102 CrossRef CAS PubMed.
- S. Hori, S. Nishiumi, K. Kobayashi, M. Shinohara, Y. Hatakeyama, Y. Kotani, N. Hatano, Y. Maniwa, W. Nishio and T. Bamba, Lung Cancer, 2011, 74, 284–292 CrossRef PubMed.
- H. Gu, Z. Pan, B. Xi, V. Asiago, B. Musselman and D. Raftery, Anal. Chim. Acta, 2011, 686, 57–63 CrossRef CAS PubMed.
- X. Cheng, X. Liu, X. Liu, Z. Guo, H. Sun, M. Zhang, Z. Ji and W. Sun, Front. Oncol., 2018, 8, 494 CrossRef PubMed.
- E. Reznik, A. Luna, B. A. Aksoy, E. M. Liu, K. La, I. Ostrovnaya, C. J. Creighton, A. A. Hakimi and C. Sander, Cell Syst., 2018, 6, 301–313.e303 CrossRef CAS PubMed.
- J. A. Ludwig and J. N. Weinstein, Nat. Rev. Cancer, 2005, 5, 845–856 CrossRef CAS PubMed.
- S. Pan, R. Chen, D. A. Crispin, D. May, T. Stevens, M. W. McIntosh, M. P. Bronner, A. Ziogas, H. Anton-Culver and T. A. Brentnall, J. Proteome Res., 2011, 10, 2359–2376 CrossRef CAS PubMed.
- J. Ingvarsson, C. Wingren, A. Carlsson, P. Ellmark, B. Wahren, G. Engström, U. Harmenberg, M. Krogh, C. Peterson and C. A. Borrebaeck, Proteomics, 2008, 8, 2211–2219 CrossRef CAS PubMed.
- J. Chen, W. Wu, L. Chen, H. Zhou, R. Yang, L. Hu and Y. Zhao, Pancreatology, 2013, 13, 290–297 CrossRef CAS PubMed.
- C. E. Birse, R. J. Lagier, W. FitzHugh, H. I. Pass, W. N. Rom, E. S. Edell, A. O. Bungum, F. Maldonado, J. R. Jett and M. Mesri, Clin. Proteomics, 2015, 12, 1 CrossRef CAS PubMed.
- K. G. Kugler, W. O. Hackl, L. A. Mueller, H. Fiegl, A. Graber and R. M. Pfeiffer, J. Clin. Bioinf., 2011, 1, 1 CrossRef PubMed.
- S. Enroth, G. Hallmans, K. Grankvist and U. Gyllensten, EBioMedicine, 2016, 12, 309–314 CrossRef PubMed.
- S. Palchetti, L. Digiacomo, D. Pozzi, R. Zenezini Chiozzi, A. L. Capriotti, A. Laganà, R. Coppola, D. Caputo, M. Sharifzadeh and M. Mahmoudi, Adv. Biosyst., 2019, 3(2), 1800221 CrossRef.
- A. Pourshams, H. Khademi, A. F. Malekshah, F. Islami, M. Nouraei, A. R. Sadjadi, E. Jafari, N. Rakhshani, R. Salahi and S. Semnani, Int. J. Epidemiol., 2010, 39, 52–59 CrossRef PubMed.
- V. Palmieri, D. Lucchetti, I. Gatto, A. Maiorana, M. Marcantoni, G. Maulucci, M. Papi, R. Pola, M. De Spirito and A. Sgambato, J. Nanopart. Res., 2014, 16, 1–8 CrossRef CAS.
- J. Schindelin, I. Arganda-Carreras, E. Frise, V. Kaynig, M. Longair, T. Pietzsch, S. Preibisch, C. Rueden, S. Saalfeld and B. Schmid, Nat. Methods, 2012, 9, 676–682 CrossRef CAS PubMed.
- A. L. Capriotti, G. Caracciolo, G. Caruso, C. Cavaliere, D. Pozzi, R. Samperi and A. Laganà, Anal. Bioanal. Chem., 2013, 405, 635–645 CrossRef CAS PubMed.
- C. Corbo, R. Molinaro, F. Taraballi, N. E. Toledano Furman, K. A. Hartman, M. B. Sherman, E. De Rosa, D. K. Kirui, F. Salvatore and E. Tasciotti, ACS Nano, 2017, 11, 3262–3273 CrossRef CAS PubMed.
- C. Corbo, R. Molinaro, F. Taraballi, N. E. T. Furman, M. B. Sherman, A. Parodi, F. Salvatore and E. Tasciotti, Int. J. Nanomed., 2016, 11, 3049 CrossRef CAS PubMed.
- L. Digiacomo, D. Pozzi, H. Amenitsch and G. Caracciolo, Biomater. Sci., 2017, 5, 1884–1888 RSC.
- A. Bigdeli, S. Palchetti, D. Pozzi, M. R. Hormozi-Nezhad, F. Baldelli Bombelli, G. Caracciolo and M. Mahmoudi, ACS Nano, 2016, 10, 3723–3737 CrossRef CAS PubMed.
- V. Colapicchioni, M. Tilio, L. Digiacomo, V. Gambini, S. Palchetti, C. Marchini, D. Pozzi, S. Occhipinti, A. Amici and G. Caracciolo, Int. J. Biochem. Cell Biol., 2016, 75, 180–187 CrossRef CAS PubMed.
- D. Pozzi, G. Caracciolo, L. Digiacomo, V. Colapicchioni, S. Palchetti, A. Capriotti, C. Cavaliere, R. Z. Chiozzi, A. Puglisi and A. Laganà, Nanoscale, 2015, 7, 13958–13966 RSC.
- G. Caracciolo, F. Cardarelli, D. Pozzi, F. Salomone, G. Maccari, G. Bardi, A. L. Capriotti, C. Cavaliere, M. Papi and A. Laganà, ACS Appl. Mater. Interfaces, 2013, 5, 13171–13179 CrossRef CAS PubMed.
- M. Schittmayer, K. Fritz, L. Liesinger, J. Griss and R. Birner-Gruenberger, J. Proteome Res., 2016, 15, 1222–1229 CrossRef CAS PubMed.
- K. K. Chereddy, R. Coco, P. B. Memvanga, B. Ucakar, A. des Rieux, G. Vandermeulen and V. Préat, J. Controlled Release, 2013, 171, 208–215 CrossRef CAS PubMed.
- A. L. Capriotti, G. Caracciolo, G. Caruso, C. Cavaliere, D. Pozzi, R. Samperi and A. Laganà, Anal. Bioanal. Chem., 2010, 398, 2895–2903 CrossRef CAS PubMed.
- G. Caracciolo, L. Callipo, S. C. De Sanctis, C. Cavaliere, D. Pozzi and A. Laganà, Biochim. Biophys. Acta, Biomembr., 2010, 1798, 536–543 CrossRef CAS PubMed.
- S. Motta, V. Rondelli, L. Cantu, E. Del Favero, M. Aureli, D. Pozzi, G. Caracciolo and P. Brocca, Colloids Surf., B, 2016, 141, 170–178 CrossRef CAS PubMed.
- D. Pozzi, V. Colapicchioni, G. Caracciolo, S. Piovesana, A. L. Capriotti, S. Palchetti, S. De Grossi, A. Riccioli, H. Amenitsch and A. Laganà, Nanoscale, 2014, 6, 2782–2792 RSC.
- A. L. Capriotti, G. Caracciolo, C. Cavaliere, C. Crescenzi, D. Pozzi and A. Laganà, Anal. Bioanal. Chem., 2011, 401, 1195–1202 CrossRef CAS PubMed.
- D. Ballabio and M. Vasighi, Chemom. Intell. Lab. Syst., 2012, 118, 24–32 CrossRef CAS.
- C. M. Andersen and R. Bro, J. Chemom., 2010, 24, 728–737 CrossRef CAS.
- R. G. Brereton and G. R. Lloyd, J. Chemom., 2014, 28, 213–225 CrossRef CAS.
- F. Marini, A. Roncaglioni and M. Novic, J. Chem. Inf. Model., 2005, 45, 1507–1519 CrossRef CAS PubMed.
- I.-G. Chong and C.-H. Jun, Chemom. Intell. Lab. Syst., 2005, 78, 103–112 CrossRef CAS.
- D. Ballabio and V. Consonni, Anal. Methods, 2013, 5, 3790–3798 RSC.
- J. Zupan, M. Novič and I. Ruisánchez, Chemom. Intell. Lab. Syst., 1997, 38, 1–23 CrossRef CAS.
- J. Zupan, M. Novič and J. Gasteiger, Chemom. Intell. Lab. Syst., 1995, 27, 175–187 CrossRef CAS.
- D. Ballabio, M. Vasighi and P. Filzmoser, Anal. Chim. Acta, 2013, 765, 45–53 CrossRef CAS PubMed.
- J. Almeida, L. Barbosa, A. Pais and S. Formosinho, Chemom. Intell. Lab. Syst., 2007, 87, 208–217 CrossRef CAS.
- B. Meunier, E. Dumas, I. Piec, D. Bechet, M. Hebraud and J.-F. Hocquette, J. Proteome Res., 2007, 6, 358–366 CrossRef CAS PubMed.
Footnotes |
† Electronic supplementary information (ESI) available. See DOI: 10.1039/c9nh00097f |
‡ These authors contributed equally to this work. |
|
This journal is © The Royal Society of Chemistry 2019 |
Click here to see how this site uses Cookies. View our privacy policy here.