DOI:
10.1039/C8NH00233A
(Minireview)
Nanoscale Horiz., 2019,
4, 365-377
Artificial intelligence in nanomedicine
Received
10th August 2018
, Accepted 4th October 2018
First published on 4th October 2018
Abstract
The field of nanomedicine has made substantial strides in the areas of therapeutic and diagnostic development. For example, nanoparticle-modified drug compounds and imaging agents have resulted in markedly enhanced treatment outcomes and contrast efficiency. In recent years, investigational nanomedicine platforms have also been taken into the clinic, with regulatory approval for Abraxane® and other products being awarded. As the nanomedicine field has continued to evolve, multifunctional approaches have been explored to simultaneously integrate therapeutic and diagnostic agents onto a single particle, or deliver multiple nanomedicine-functionalized therapies in unison. Similar to the objectives of conventional combination therapy, these strategies may further improve treatment outcomes through targeted, multi-agent delivery that preserves drug synergy. Also, similar to conventional/unmodified combination therapy, nanomedicine-based drug delivery is often explored at fixed doses. A persistent challenge in all forms of drug administration is that drug synergy is time-dependent, dose-dependent and patient-specific at any given point of treatment. To overcome this challenge, the evolution towards nanomedicine-mediated co-delivery of multiple therapies has made the potential of interfacing artificial intelligence (AI) with nanomedicine to sustain optimization in combinatorial nanotherapy a reality. Specifically, optimizing drug and dose parameters in combinatorial nanomedicine administration is a specific area where AI can actionably realize the full potential of nanomedicine. To this end, this review will examine the role that AI can have in substantially improving nanomedicine-based treatment outcomes, particularly in the context of combination nanotherapy for both N-of-1 and population-optimized treatment.
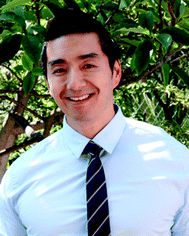
Dean Ho
| Dean Ho is a Provost's Chair Professor of Biomedical Engineering and Pharmacology, Director of SINAPSE, and member of the Biomedical Institute for Global Health Research and Technology (BIGHEART) at the National University of Singapore. Prof. Ho has led multiple first-in-human clinical studies to validate CURATE.AI-driven optimization of combination therapy, and nanomedicine platforms for drug delivery. He is a Fellow of the American Institute of Medical and Biological Engineering (AIMBE). |
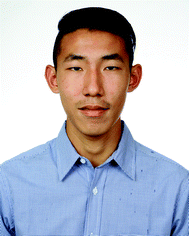
Peter Wang
| Peter Wang is a research intern at Singapore Institute for Neurotechnology (SINAPSE) at the National University of Singapore. His research with Prof. Dean Ho focuses on developing and implementing CURATE.AI, an artificial intelligence platform that optimizes combination therapy. Peter was a UCLA Alumni Scholar, UCLA Samueli Engineering Research Scholar, and APIASF Scholar. |
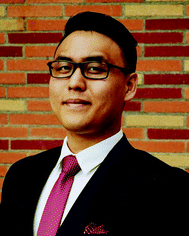
Theodore Kee
| Theodore Kee holds an MS in Bioengineering from UCLA, and he is currently a research associate at NUS Biomedical Engineering. His background focused on developing novel therapeutic combinations for a myriad of indications, including oncology. Currently, Theodore coordinates the clinical trials in the team of Prof. Dean Ho to expand the clinical implementation of CURATE.AI. |
1 Introduction
A broad collection of nanomedicine platforms has been explored for drug delivery and imaging applications.1–18 These have included polymer, metal, lipid, silicon, natural compound, and carbon-based platforms.12,19–54 Indications addressed by these nanomedicine-functionalized therapies and imaging compounds have included multiple types of cancer, cardiovascular disease, regenerative medicine, and others.55–65 Benefits that have been demonstrated through nanotechnology-enhanced compounds include the ability to overcome efflux-mediated drug resistance, and improved drug delivery localization through various targeting moieties, as well as increased magnetic resonance imaging contrast efficiency.66–74
Since the emergence of the field of nanomedicine, major strides have been made in terms of harnessing diverse chemistry to simultaneously functionalize nanomaterial platforms with multiple agents.75–78 Prior studies have included the use of aptamers and small molecule chemotherapies as well as siRNA and small molecules to target various cancers.79,80 The coordinated co-delivery of different classes of nanomaterials that have each been functionalized with different therapies has also been shown to improve treatment outcomes.81–83 Multilayered approaches have also been used to control administration schedules, enabling sequential drug delivery-based improvements in efficacy.59,84,85 These capabilities have led to the expanded investigation of nanomedicine platforms for combination therapy, or the co-delivery of therapies to address multiple aberrant disease pathways to increase treatment efficacy.86–89 Importantly, combination therapy represents a cornerstone of treatment strategies for indications ranging from oncology to infectious diseases and regenerative medicine. The use of 2-, 3-, and 4-drug combinations has led to marked improvements in objective response rate (ORR), and other key endpoints such as overall survival (OS) for oncology, and driven landmark advances in human immunodeficiency virus (HIV), autoimmune deficiency (AIDs), and tuberculosis (TB) therapy, among many other globally prevalent diseases.90–92
In the area of clinical nanomedicine, the past several years has also witnessed the successful advancement of multiple platforms into clinical studies and approval. For example, a PLGA–docetaxel complex was studied for breast cancer.59 A gold nanoshell approach has been clinically assessed for photodynamic therapy.93–95 A spherical nucleic acid (SNA) platform is currently in clinical trials for glioblastoma therapy.96 Clinical trials to assess the use of nanodiamond (ND)-embedded biomaterials for wound healing and anti-infection in oral health and other indications have also been initiated.97–99 With regard to approved nanomedicine formulations, in addition to pegylated liposomal doxorubicin (DOXIL®), albumin-functionalized paclitaxel (Abraxane®) has been approved for multiple indications such as pancreatic, lung, and breast cancer.100 Recently, liposomal irinotecan has also been approved for metastatic pancreatic cancer, and liposomal cytarabine and daunorubicin (CPX-351) has been approved for acute myeloid leukemia.101–103
Achieving therapeutic synergism has long served as a key objective in the design of nano-modified and unmodified combination therapies. In the context of drug development, synergism is defined as the effects of integrating 2 or more drugs superceding those observed by combining the individual effects of each drug.104–106 It is important to note that the degree of drug synergy that is achieved in a combination regimen is not always quantified. Furthermore, it is important to note that even if synergy is achieved, global optimization represents a different outcome. Specifically, drug synergy during combination therapy may improve efficacy and safety compared to monotherapy, but globally optimized efficacy and safety is expected to far outperform synergistic drug interactions. Identifying these parameters represents an area where AI can uniquely excel to realize major advances in drug development.
Synergistic multi-therapeutic delivery using nanomaterials has clearly mediated improved outcomes in preclinical and clinical studies compared to unmodified standard of care regimens.2,23,107–165 In addition to delivering drug compounds, nanomaterials can also be harnessed for other means of stimulus-based intervention, such as photothermal therapy. However, achieving interventional synergy and globally optimized therapy are very different outcomes. More specifically, while synergy is a relative classification that can improve efficacy, globally optimized therapy represents the best possible treatment outcome from a pool of candidate therapies and inputs, and implicitly optimized synergy as well. This is due to the fact that multi-drug delivery and its coupling with other potential nanomedicine-mediated intervention such as photothermal therapy effectively creates a prohibitively large parameter space, where the modulation of drug-dose ratios, and potentially the parameters used for stimulus-based intervention, substantially alters treatment efficacy and safety. In fact, optimal dose selection can often change the drugs that comprise a globally optimized combination. This effectively creates a quandary where global optimization is a function of simultaneously selecting the right drugs and their doses from a pool of conventional therapies (Fig. 1). Therefore, a byproduct of traditional screening and matrix and dose escalation-based combination therapy design is an inability to reconcile this aforementioned parameter space. As such, while synergy is achieved, global optimization is virtually precluded. Further complicating these issues are the fact that drug synergism and antagonism are dynamic, and are time-dependent, dose-dependent, and patient-specific. These factors demonstrate that the drugs that comprise a combination as well as dynamic dose modulation collectively drive globally optimized treatment that is sustained and continuous. As such, fixed-dose drug delivery, even when synergistic, can result in drug development failure and low treatment response rates. Therefore, while achieving synergy is often viewed as a sufficient threshold for improving combination therapy, attaining global optimization represents an important opportunity to overcome the barriers that have confronted drug development since its inception and will ultimately impact nanomedicine development as well, particularly since the use of nanomaterial vehicles can add many more dimensions of complexity to the design of optimal combinations as the materials themselves can also impart therapeutic activity.
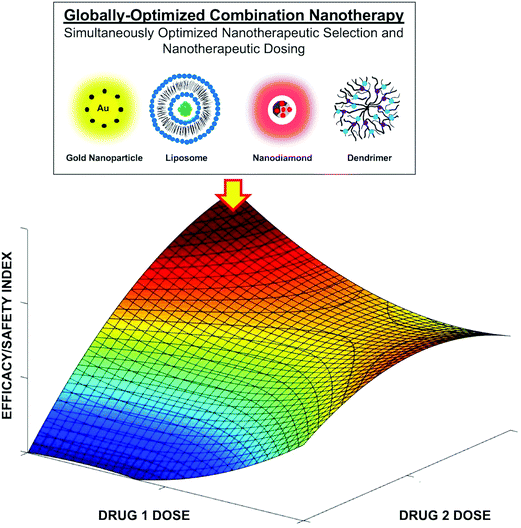 |
| Fig. 1 Harnessing artificial intelligence to optimize nanomedicine. A schematic doublet nanotherapy drug interaction map is shown. Using rationally-designed combination nanotherapy configurations for initial calibration experiments, artificial intelligence can be used to construct three-dimensional profiles that pinpoint globally optimized drug combinations. Importantly, this approach uniquely achieves global optimization by simultaneously identifying both the nanotherapeutics that comprise the combination, as well as the respective nanotherapeutic dosages in a mechanism-independent fashion. Furthermore, artificial intelligence can be used for multi-parametric optimization, where efficacy and safety can be simultaneously optimized. | |
The emergence of AI ultimately presents a feasible opportunity to realize the full potential of combinatorial nanomedicine.47,166–191 This is due to the fact that AI-determined combination parameters can achieve globally optimal therapeutic outcomes independent of complex disease mechanism information. This review will highlight specific examples where insurmountably large drug and dose spaces can be rationally reconciled into actionable and optimized combination therapy for population-wide or personalized medicine using AI. These studies will likely serve as a foundation for overcoming low response rates and trial failures that are a result of sub-optimal drug selection in combination design and the dynamic nature of drug synergism and antagonism. Ultimately, AI will serve as a key catalyst towards more drug approvals, markedly improved outcomes, and improved patient accessibility to affordable nanomedicines.
2 How artificial intelligence (AI) can transform nanomedicine
When good drugs are delivered at sub-optimal doses, they can mediate sub-optimal responses. When drugs that were not originally considered as candidate compounds for combination therapy are delivered at optimal dosages, the resulting treatment outcomes may be globally optimized, far superseding those observed with drugs that were perceived to be ideally suited for co-administration.47,166–192 This quandary resides at the heart of conventional drug development, and will also impact nanomedicine drug development. For example, drug synergy is a key driver of combination therapy design. Subsequent nanomedicine-based delivery of synergistic therapies represents a major effort in the field due to the potential for carrier platforms to preserve synergy at the site of activity.
Achieving optimized combination nanotherapy will ultimately require more than drug synergism. Instead, realizing optimal treatment outcomes relies on 2 stages of development. Firstly, the drug and dose space will need to be interrogated in order to identify drugs and their corresponding dosages that optimize efficacy and safety. Second, once a selected combination therapy is administered, synergy becomes a dynamic process that changes over time. The dose-dependent and patient-specific nature of synergy will then necessitate the dynamic dosing of combination therapies in order to sustain optimization during the course of treatment. Addressing both of these stages in a clinical setting using agnostic, AI-enabled approaches that implicitly account for factors such as drug targeting, ratiometric delivery, and other properties that are enabled by nanotechnology-mediated delivery as well as dynamic patient response to treatment would require unprecedented levels of actionability during drug administration. Conventional approaches that utilize –omics based information have seen success in pairing drugs with patients. However, dynamically modulating treatment requires an even higher degree of actionability in the clinic. This requirement represents an opportunity for the field of artificial intelligence (AI).
Furthermore, nanotechnology has also been used to improve drug targeting to specific regions as a means of preserving potential drug synergies. However, patient-specific requirements for drug exposure at disease sites vary greatly. Also, drug synergy observed at specific doses for one patient may in fact result in drug antagonism for another patient.166 During the course of treatment, synergy can also transform into antagonism in the same patient. The convergence of AI with nanomedicine can be used to address these challenges, particularly since AI can agnostically optimize treatment in a mechanism-independent manner. By directly correlating inputs (e.g. drug selection, dose selection, stimuli-responsive material selection, frequency and amplitude stimulus, etc.) with outputs (efficacy, safety), which are a function of targeting and localization effects, AI can serve as a powerful means of rationally reconciling all of these factors to optimize combination nanotherapy for both individuals and populations.
Harnessing AI to overcome many of the challenges confronted in medicine has seen important advances. For example, deep learning algorithms developed for melanoma diagnosis have shown comparable accuracy with clinician-driven standard of care diagnostic procedures.193–195 Methodologies relevant to AI have also been used to predict the efficacy of drug combinations based on drug synergy.
2.1 Nanomedicine optimization
Recent advances in combinatorial nanomedicine have already served as suitable opportunities for interfacing with AI-based optimization. For example, the combination delivery of multiple classes of nanoparticles was used to enhance the localization and efficacy of tumor treatment. Specifically, gold nanoparticles were initially used to localize at tumor sites. Photothermal heating of the particles via infrared light was used to trigger a clotting cascade. A second class of nanoparticles, which were liposomes that were loaded with doxorubicin and coated with an analog for Factor XIII, a protein that mediates fibrin crosslinking during clotting, was then administered. The clotting process substantially increased the binding sites for this complex resulting in a 40-fold increase in drug localization at the tumor site and markedly improved treatment efficacy.196
Another example of co-delivery to enhance tumor penetration involves the co-administration of iRGD, a tumor-penetrating peptide, and irinotecan-loaded multifunctional mesoporous silica nanoparticles (MSNPs), also referred to as silicasomes. Unconjugated iRGD, a cyclic tumor-penetrating peptide, enhances transcytosis of the carrier silicasomes by engaging with the NRP-1 receptor, thereby upregulating a nutrient-transport pathway. The co-administration of iRGD demonstrated a 3- to 4-fold increased silicasome uptake by highly aggressive pancreatic ductal adenocarcinoma (PDAC) tumors and improved treatment outcomes, decreasing metastasis and increasing overall survival time.197
Nanoparticles can effectively stabilize and deliver synergistic combinations as demonstrated with PLGA–PEG nanoparticles encapsulating and co-localizing two different classes of agents, cisplatin-prodrug and siRNA. In the combination, the siRNA, siREV1 and siREV3L, suppress the expression of the translesion DNA synthesis (TLS) pathway, an error-prone mechanism that allows tumor cells to repair chemotherapy-induced DNA damage and predisposes the cells to develop drug-resistance. Concurrent administration of siREV1, siREV3L, and cisplatin–prodrug within PLGA–PEG nanoparticles was shown to inhibit further tumor growth and increase overall survival time in the murine models by accommodating the siRNA-mediated downregulation of TLS activity involved in acquired mutagenic drug-resistance, sensitizing the cell to the controlled release of cytotoxic cisplatin.198 Nanoparticles can also be used for the delivery of therapies which synergistically interact with other modalities of treatment. One such example is the multi-functional up-conversion core/porous silica shell nanotheranostics (UCSNs) with a core consisting of dual-functional-up-conversion nanoparticles (UCNPs) encapsulated with cisplatin, shown to clinically enhance radiotherapy, and a porous silica layer as the outer shell for precise targeting using magnetic imaging. UCSNs were shown to achieve efficient delivery of cisplatin to tumors for effective synergistic radio-/chemotherapy and luminescence/magnetic dual-mode imaging for targeting tumors.107
With recent innovations in multilayered approaches, layer-by-layer (LbL) nanoparticle carriers were synthesized that enhanced therapeutic efficacy against NSCLC tumors displaying overexpression of oncogene KRAS along with a defective p53 tumor suppression pathway. The LbL platform allowed for staged cargo release by consecutive layering of oppositely charged particles upon a charged core, in this case a liposomal encapsulation of cisplatin. The two nucleic acids, siKRAS intended for KRAS knockout and miR34a, a p53 downstream activator, were then sequentially layered on top, offering synergistic gene-mediated “priming” of the tumor cell. With tumor defense mechanisms averted ahead of cisplatin delivery, mouse survival was extended to 23.5 days under combination therapy, in comparison to 15.5 and 9.0 days with cisplatin and RNA monotherapy alone, respectively.199
Taken together, the multiple agents delivered using nanotechnology platforms have been shown to work in concert to address different disease pathways or targets. While nanomedicine-based combination therapy strategies have improved treatment efficacy, interfacing nanotechnology-based drug delivery with AI can realize substantial improvements in treatment outcomes. This is due to the fact that nanomedicine-based combination therapy is subject to the same constraints as unmodified combination therapy, where fixed-dose, multi-drug delivery can be challenged by changes in drug synergy in response to patient heterogeneity. In a recent study, AI was used to globally optimize the drug-dose ratios of a 4-drug combination therapy regimen consisting of nanodiamond–doxorubicin, nanodiamond–bleomycin, nanodiamond–mitoxantrone, and unmodified paclitaxel. Multiple breast cancer cell lines (MDA-MB-231, MCF-7, BT20) and multiple control cell lines (IMR90, H9C2, MCF-10A) were simultaneously interrogated. The optimization objective of this study was to identify the doses of each drug in the combination that would result in maximal cancer cell apoptosis and maximal control cell line viability. The Latin Hypercube Sampling modality experimentally identified phenotypic response surfaces from which the optimal doses could be immediately pinpointed. Importantly, this study showed that AI-optimized nanomedicine drug combinations outperformed AI-optimized unmodified drug combinations, unmodified and nanomedicine-modified monotherapies, as well as arbitrarily designed nanomedicine combinations. In addition, it was shown that a majority of these arbitrary nanomedicine combinations were more toxic than efficacious. Interestingly, it should be noted that doxorubicin, mitoxantrone, and bleomycin are similar therapies. Conventional drug development implies that combinations containing these drugs would result in an additive effect. However, this study showed that identifying optimal drug-dose ratios resulted in substantially improved efficacy beyond an additive effect. This demonstrated the importance of dose optimization in combination therapy design.174
Importantly, issues such as nanodrug formulation, release profiles, targeting, localization efficiency, and other factors are all important to consider when developing a nanomedicine complex. However, a highly compelling attribute of AI is that its optimization processes implicitly account for these properties, since AI can directly optimize for treatment efficacy, which is a function of all of these elements. Hence, they are already accounted for when the optimization process is implemented.
2.2 Optimizing the drug development pipeline with artificial intelligence
Drug development conventionally relies on target-based discovery of novel compounds, followed by screening and dose finding studies for safety and efficacy. During the screening process, compounds with no apparent treatment efficacy are often removed from the screen. A challenge that is virtually insurmountable when addressed using brute force interrogation is the fact that the drug and dose space is prohibitively large. As such, even if a drug may not mediate apparent efficacy alone, the drug may be capable of mediating optimal efficacy when delivered in combination with the right drugs at the right doses. Identifying this combination using standard screening is challenging. This is a facet of drug development, both nano- and non-nano-, that can be particularly enhanced via AI.47,166–191 For example, in a recent study assessing anti-angiogenic agents for preclinical ovarian cancer treatment, drugs that displayed no apparent efficacy as monotherapies at specific doses resulted in substantial decreases in tumor burden when delivered in combination at the exact same doses.179,187,188 This finding demonstrated the role that AI can play in identifying powerful drug combinations from prohibitively large parameter spaces, which will also undoubtedly impact the nanomedicine domain.
In another recent study, a pool of 14 candidate therapies to address a macrophage model of Mycobacterium tuberculosis (MTB) infection was interrogated with an AI platform, revealing a ranked list of agnostically identified drug combinations that resulted in a substantially enhanced efficacy over clinical standards. Importantly, the top ranked combinations included clofazimine, a third line drug, while none of the top ranked combinations contained rifampin or isoniazid. Importantly, extensively drug-resistant MTB and drug-resistant MTB are often non-responsive to rifampin and isozianid. As such, the AI-optimized combinations may be broadly applicable towards hard-to-treat MTB infections. These combinations were subsequently re-optimized in preclinical studies, which revealed substantially different drug-dose ratios required to optimize MTB infection clearance. This was a particularly important finding, since this re-optimization process reduced the time to relapse-free cure by 75%.169,186
In the field of oncology, combination therapy design strives to achieve drug synergy at fixed doses during initial in vitro experiments. These fixed-dose combination therapies are either directly translated into preclinical validation, or titration and matrix-based studies are developed to assess additional drug-dose ratios for subsequent preclinical validation. While these strategies have resulted in eventual clinical studies that have seen success, many studies fail in the clinic even when a preclinical combination therapy design revealed highly synergistic drug interactions. A recent study harnessed a powerful AI platform called Quadratic Phenotypic Optimization Platform (QPOP) to interrogate a large pool of potential drugs to design a novel combination therapy against bortezomib-resistant multiple myeloma. This pool of therapies included the clinical standards of care, including bortezomib, dexamethasone, lenalidomide, and melphalan. However, mechanism-independent, AI-driven optimization showed that the top ranked combination consisted of decitabine and mitomycin C.173 Individually, these therapies mediated no apparent efficacy towards the bortezomib-resistant multiple myeloma model. However, when combined at the right drug-dose ratio, this combination resulted in superior efficacy over the clinical standards. In addition, this study harnessed AI to preclinically re-optimize the combination to successfully translate the multi-drug regimen through in vivo validation. It is important to note that both the in vitro and preclinical re-optimization processes were able to simultaneously take into account both efficacy and safety, and that there was a clear need to re-identify proper drug-dose ratios in vivo to ensure that the combinations were more efficacious than they were toxic. This was a critical capability that was uniquely enabled by the QPOP AI platform. Patient samples were also interrogated using QPOP. Interestingly, QPOP analysis showed that patient samples displayed highly individualized responses to the different combination therapies (Fig. 2). This was achieved within days, without complex mechanism-based studies. As such, QPOP may also serve as an actionable platform to design patient-specific regimens. This multi-parametric global optimization approach will ultimately overcome many of the barriers that face the drug development process, and will ultimately result in efficacious and safer therapies being developed at a much faster rate. This will re-define the drug development roadmap, translating into improved patient access to affordable and effective treatment options.
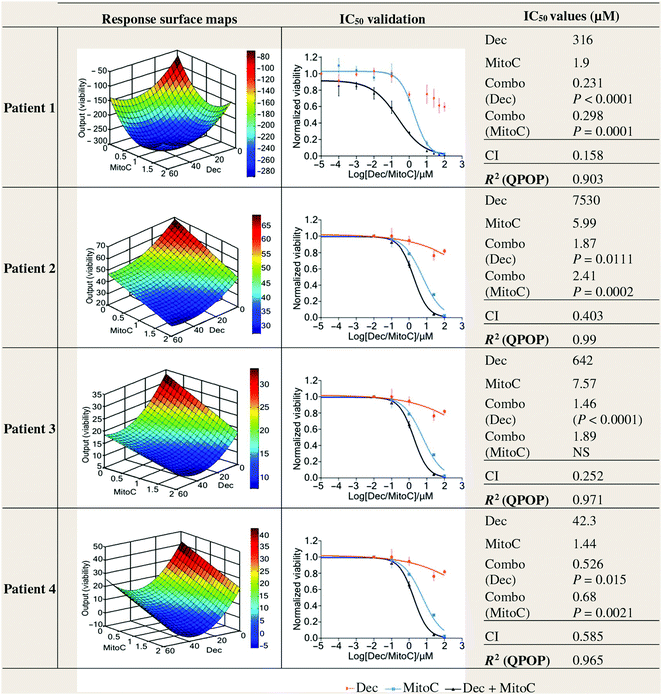 |
| Fig. 2 Artificial intelligence-driven optimization of novel combination therapies. The quadratic phenotypic optimization platform (QPOP) was utilized to agnostically identify drug combinations that were simultaneously optimized for both drug selection and drug dosage. The multi-drug regimens identified using QPOP markedly outperformed the standard of care regimens. Furthermore, patient-specific regimens, as shown, could be actionable determined for individualized combination therapy development. Figure reprinted with permission from the American Association for the Advancement of Science.173 | |
2.3 Artificial intelligence-guided therapy in the clinic
In addition to nanomedicine-based combination therapy design, AI will play a major role in optimizing the administration of nanotechnology-modified as well as unmodified drug combinations. As previously mentioned, synergy is time-dependent, dose-dependent, and patient specific. Therefore, even when synergy is observed in a fixed-dose combination, this synergy can change drastically, or even switch to antagonism during the course of treatment. This challenge accounts for sub-optimal response rates when fixed-dose treatment is administered to an inherently heterogeneous patient population.
Multiple methodologies have been explored for clinical decision support to guide treatment in the clinic.200–208 These have included big data-driven approaches, where electronic medical records of patient treatment outcomes, genetic and broader –omics profiling, and other information has been used for drug selection. These strategies collectively represent an important first step towards using valuable databases of information to refine the regimen design process, which may improve broader patient population efficacy and safety. However, when regimens are selected, the issue of changing synergies and evolving patient responses to therapy still remain as key challenges. To address this barrier, strategies to modulate in vivo drug dosing in oncology and other indications have been explored. These have involved evolutionary dosing, as well as other Bayesian and population-based algorithms. These approaches typically involve the use of multiple data points based on feedback in order to attempt convergence towards improved outcomes. In certain indications, this is a barrier to translation particularly when the search process can contribute to substantial declines in patient health. The treatment process is further confounded given the very large parameter spaces that are created when multiple drugs are delivered during combination therapy.
Taking progress towards optimized dosing in oncology as an example, dose reductions are often implemented to manage treatment-related toxicity, as opposed to increasing efficacy in combination therapy. Emerging studies are showing that it is possible to increase efficacy while reducing drug dosing in combination therapy. A main challenge that must be addressed in order to firmly translate this practice into the clinic is the establishment of a dosing approach that can definitely correlate the right dose reduction with the best possible treatment outcome at a specific time point. This challenge can be addressed via recent advances in AI.
A recent clinical study has shown that actionable and optimized dosing at the N-of-1 level, that is, using only the data of a single patient undergoing combination therapy, is achievable in the clinic. In this study, liver transplant immunosuppression was optimized using CURATE.AI, a powerful technology platform comprised of dosing strategies such as parabolic personalized dosing (PPD).166 In particular, this study aimed to dynamically identify optimal doses of tacrolimus, a standard of care immunosuppressant that would achieve targeted trough levels of tacrolimus, which is the surrogate marker for immunosuppression efficacy. The study was divided into two arms, with the control arm based on conventional titration of tacrolimus, and the treatment arm based on CURATE.AI-guided dosing. The CURATE.AI arm outperformed the control arm in all metrics, such as the duration of time spent within the target tacrolimus trough levels. Importantly, CURATE.AI-treated patients were discharged, on average, nearly one month earlier compared to the control arm patients. These metrics show that CURATE.AI may be effective at improving patient outcomes and reducing the cost of care while serving as a scalable platform for broad clinical implementation.
In addition to the enhanced treatment efficacy mediated by CURATE.AI, this study showed that substantial inter-patient variability existed with regards to drug interactions. In particular, changes in drug synergy were observed during the course of treatment. Of note, intra-patient changes in drug synergy were also observed, whereby drugs that appeared to be synergistic at the start of treatment also appeared to become antagonistic several weeks following treatment (Fig. 3). Therefore, these findings demonstrated a clear need for dynamically modulated drug dosing in order to continuously optimize treatment and improve upon sub-optimal response rates from fixed-dose treatment. Of note, the dynamic nature of drug synergy and antagonism shows that the re-acquisition of efficacy from a perceived failure in combination therapy is possible when optimized modulated dosing is implemented.
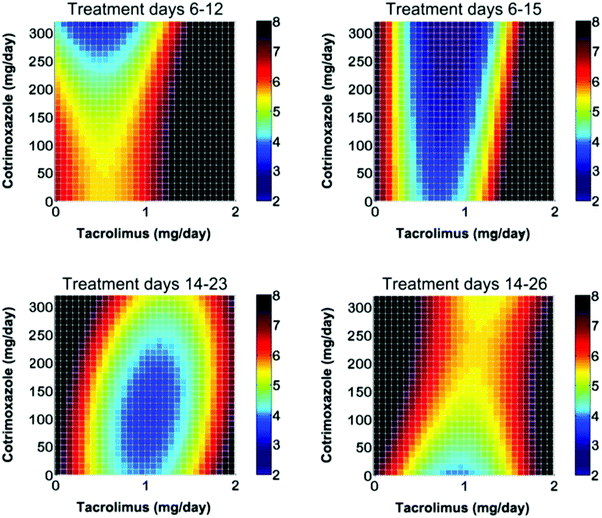 |
| Fig. 3 Intra-patient changes in drug synergy necessitate N-of-1 medicine. Combination therapy is often administered in fixed doses. In a previous clinical study, intra-patient changes in drug synergy were observed, which clearly demonstrate a need to dynamically modulate multi-drug dosing when possible in order to sustain the optimization of combination therapy treatment outcomes. Figure reprinted with permission from the American Association for the Advancement of Science.166 | |
CURATE.AI has also been harnessed to optimize N-of-1 combination therapy for advanced cancer treatment.192 In a recent case report, a metastatic castration-resistant prostate cancer (MCRPC) patient was given a regimen of BET bromodomain inhibitor ZEN-3684 and enzalutamide. The treatment objectives included prostate specific antigen (PSA) reduction as well as the prevention of disease progression, which was monitored using computed tomography (CT) imaging. Following initial clinical-guided dose adjustments, a CURATE.AI profile utilizing only the patient's own data was constructed (Fig. 4). The profile suggested a 50% reduction in drug dosing that resulted in the lowest levels of PSA observed in the study. Subsequent monitoring of PSA levels was used to update the CURATE.AI profile, which suggested continuous modulation of ZEN-3694 and enzalutamide dosing to sustain the optimization of combination therapy. Over the course of an ongoing study that has lasted for more than 16 months, a durable response with halted disease progression was achieved. This study serves as a gateway towards the dynamic modulation of combination therapy dosing to continuously optimize treatment responses. The downstream integration of platforms such as CURATE.AI with emerging clinical study designs such as basket studies are likely to provide strong foundations for AI-driven improvements in response rates for future nanomedicine and drug development programs.
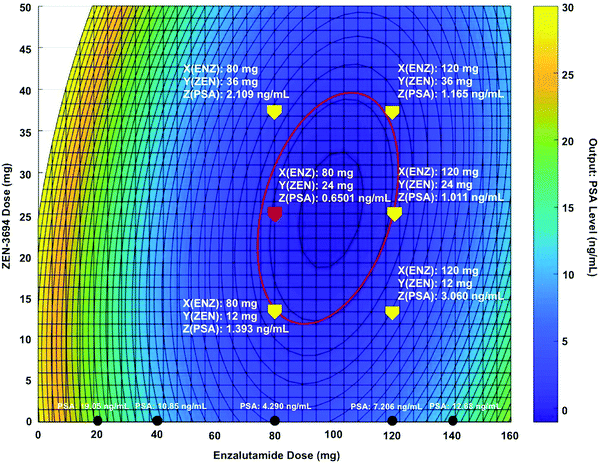 |
| Fig. 4 Artificial intelligence has been successfully utilized for dynamic modulation of combination therapy in an advanced cancer patient. Specifically, the dosages of BET bromodomain inhibitor ZEN-3694 and enzalutamide were dynamically adjusted using CURATE.AI, resulting in a durable patient response and halted disease progression. Platforms such as CURATE.AI will become increasingly important with regards to using N-of-1 medicine to improve response rates for novel unmodified and nanotechnology-modified drug combinations. Importantly, approaches such as CURATE.AI can also implicitly account for multiple factors that are associated with nanomedicine, such as drug targeting, and stimulus-driven intervention to agnostically optimize treatment outcomes. Figure reprinted with permission from John Wiley & Sons.192 | |
3 Conclusions
The field of nanomedicine is harnessing a collection of versatile carriers to improve the targeting of disease sites and localization of drug delivery. These carriers have recently served as gateways towards combinatorial nanotherapy to further increase treatment efficacy by simultaneously addressing multiple disease pathways. Combination therapy using nanomedicine, however, will be confronted by the same challenges faced by conventional drug development roadmaps. These include the preservation of drug synergy, improved targeting efficiency, and rationally designed drug exposure. Achieving drug synergy is a promising first step towards enhanced treatment outcomes, but synergy and optimization are very different endpoints. Combination therapy design opens up a very large drug and dose parameter space that will need to be reconciled in order to globally optimize treatment. In addition, patients vary substantially with regard to the dosages needed to attain drug synergy, as well as the degree of drug exposure needed to reach optimal treatment outcomes. These parameters also vary over time within the same patient. The emergence of AI will play a critical role in reconciling this space into an actionable treatment response, and the recent studies highlighted in this work serve as a strong foundation for a re-defined combination therapy landscape that will be markedly enhanced with nanotechnology-modified therapeutics.
Conflicts of interest
D. H. and T. K. are co-inventors of issued and pending patents pertaining to artificial intelligence and nanodiamond platforms.
Acknowledgements
D. H. and T. K. gratefully acknowledge funding support from the National University of Singapore. D. H. acknowledges support from the V Foundation for Cancer Research, Wallace H. Coulter Foundation Translational Research Award, and Fulbright Core U.S. Scholar Program.
References
- K. Glinel, A. Behrens, R. S. Langer, A. Jaklenec and A. M. Jonas, Biomacromolecules, 2018 DOI:10.1021/acs.biomac.8b00735.
- V. Sainz, L. I. Moura, C. Peres, A. I. Matos, A. S. Viana, A. M. Wagner, J. E. V. Ramirez, T. Barata, M. Gaspar and S. Brocchini, Acta Biomater., 2018, 76, 193–207 CrossRef CAS PubMed.
- J. T. Peters, S. S. Hutchinson, N. Lizana, I. Verma and N. A. Peppas, Chem. Eng. J., 2018, 340, 58–65 CrossRef CAS.
- H. Cheng, R. Chabok, X. Guan, A. Chawla, Y. Li, A. Khademhosseini and H. L. Jang, Acta Biomater., 2018, 69, 342–351 CrossRef CAS PubMed.
- A. S. Barnard, Nanoscale, 2018, 10, 8893–8910 RSC.
- E. S. Kang, Y.-T. Kim, Y.-S. Ko, N. H. Kim, G. Cho, Y. H. Huh, J.-H. Kim, J. Nam, T. T. Thach and D. Youn, ACS Nano, 2018, 12, 6554–6562 CrossRef CAS PubMed.
- S. Claveau, J.-R. Bertrand and F. Treussart, Micromachines, 2018, 9, 247 CrossRef.
- D. A. Simpson, E. Morrisroe, J. M. McCoey, A. H. Lombard, D. C. Mendis, F. Treussart, L. T. Hall, S. Petrou and L. C. Hollenberg, ACS Nano, 2017, 11, 12077–12086 CrossRef CAS PubMed.
-
F. Ziem, M. Garsi, H. Fedder and J. Wrachtrup, arXiv preprint arXiv:1807.08343, 2018.
- O. G. Hayes, J. R. McMillan, B. Lee and C. A. Mirkin, J. Am. Chem. Soc., 2018, 140, 9269–9274 CrossRef CAS PubMed.
- J. E. Coffman, S. W. Metz, A. Brackbill, M. Paul, M. J. Miley, J. DeSimone, J. C. Luft, A. de Silva and S. Tian, Bioconjugate Chem., 2018, 29, 1544–1552 CrossRef CAS PubMed.
- L. Urbano, L. Clifton, H. K. Ku, H. Kendall-Troughton, K.-K. A. Vandera, B. F. Matarese, T. Abelha, P. Li, T. Desai and C. A. Dreiss, Langmuir, 2018, 34, 6125–6137 CrossRef CAS PubMed.
- T. Stewart, W. T. Koval, S. A. Molina, S. M. Bock, J. W. Lillard Jr, R. F. Ross, T. A. Desai and M. Koval, Exp. Cell Res., 2017, 355, 153–161 CrossRef CAS PubMed.
- M. Liu, S. Shen, D. Wen, M. Li, T. Li, X. Chen, Z. Gu and R. Mo, Nano Lett., 2018, 18, 2294–2303 CrossRef CAS PubMed.
- L. Zhu, J. Zhou, G. Xu, C. Li, P. Ling, B. Liu, H. Ju and J. Lei, Chem. Sci., 2018, 9, 2559–2566 RSC.
- X. Shen, B. Wang, K. Li, L. Wang, X. Zhao, F. Xue, R. Shi and J. Zheng, J. Invest. Dermatol., 2018, 138, 2024–2032 CrossRef CAS PubMed.
- S. T. Koshy, D. K. Zhang, J. M. Grolman, A. G. Stafford and D. J. Mooney, Acta Biomater., 2018, 65, 36–43 CrossRef CAS PubMed.
- O. Veiseh, J. W. Gunn and M. Zhang, Adv. Drug Delivery Rev., 2010, 62, 284–304 CrossRef CAS PubMed.
- D. S. Spencer, B. C. Luu, D. W. Beckman and N. A. Peppas, J. Polym. Sci., Part A: Polym. Chem., 2018, 56, 1536–1544 CrossRef CAS.
- A. M. Wagner, D. S. Spencer and N. A. Peppas, J. Appl. Polym. Sci., 2018, 135, 46154 CrossRef PubMed.
- X. Chen, Z. Chen, B. Hu, P. Cai, S. Wang, S. Xiao, Y. L. Wu and X. Chen, Small, 2018, 14, 1703164 CrossRef PubMed.
- J. M. Buriak, P. V. Kamat, K. S. Schanze, A. P. Alivisatos, C. J. Murphy, G. C. Schatz, G. D. Scholes, P. J. Stang and P. S. Weiss, Chem. Mater., 2017, 29, 8915–8917 CrossRef CAS.
- V. Mirshafiee, B. Sun, C. H. Chang, Y.-P. Liao, W. Jiang, J. Jiang, X. Liu, X. Wang, T. Xia and A. E. Nel, ACS Nano, 2018, 12, 3836–3852 CrossRef CAS PubMed.
- E. R. Evans, P. Bugga, V. Asthana and R. Drezek, Mater. Today, 2018, 21, 673–685 CrossRef CAS PubMed.
- H. Cheng, N. Yang, Q. Lu, Z. Zhang and H. Zhang, Adv. Mater., 2018, 1707189 CrossRef PubMed.
- M. Tiffany and F. C. Szoka, J. Drug Targeting, 2016, 24, 857–864 CrossRef CAS PubMed.
- K. S. Park, H. Kim, S. Kim, K. Lee, S. Park, J. Song, C. Min, F. Khanam, R. Rashu and T. R. Bhuiyan, ACS Nano, 2017, 11, 11425–11432 CrossRef CAS PubMed.
- H. Huang, E. Pierstorff, E. Osawa and D. Ho, Nano Lett., 2007, 7, 3305–3314 CrossRef CAS PubMed.
- M. Chen, X.-Q. Zhang, H. B. Man, R. Lam, E. K. Chow and D. Ho, J. Phys. Lett., 2010, 1, 3167–3171 CAS.
- R. Lam, M. Chen, E. Pierstorff, H. Huang, E. Osawa and D. Ho, ACS Nano, 2008, 2, 2095–2102 CrossRef CAS PubMed.
- M. Chen, E. D. Pierstorff, R. Lam, S.-Y. Li, H. Huang, E. Osawa and D. Ho, ACS Nano, 2009, 3, 2016–2022 CrossRef CAS PubMed.
- H. Huang, M. Chen, P. Bruno, R. Lam, E. Robinson, D. Gruen and D. Ho, J. Phys. Chem. B, 2009, 113, 2966–2971 CrossRef CAS PubMed.
- X.-Q. Zhang, M. Chen, R. Lam, X. Xu, E. Osawa and D. Ho, ACS Nano, 2009, 3, 2609–2616 CrossRef CAS PubMed.
- R. A. Shimkunas, E. Robinson, R. Lam, S. Lu, X. Xu, X.-Q. Zhang, H. Huang, E. Osawa and D. Ho, Biomaterials, 2009, 30, 5720–5728 CrossRef CAS PubMed.
- X.-Q. Zhang, T. T. Trinh, R. A. van Santen and A. P. Jansen, J. Am. Chem. Soc., 2011, 133, 6613–6625 CrossRef CAS PubMed.
- X.-Q. Zhang, X. Xu, R. Lam, D. Giljohann, D. Ho and C. A. Mirkin, ACS Nano, 2011, 5, 6962–6970 CrossRef CAS PubMed.
- X.-Q. Zhang, J. Intra and A. K. Salem, Bioconjugate Chem., 2007, 18, 2068–2076 CrossRef CAS PubMed.
- L. M. Manus, D. J. Mastarone, E. A. Waters, X.-Q. Zhang, E. A. Schultz-Sikma, K. W. MacRenaris, D. Ho and T. J. Meade, Nano Lett., 2009, 10, 484–489 CrossRef PubMed.
- X.-Q. Zhang, X.-L. Wang, S.-W. Huang, R.-X. Zhuo, Z.-L. Liu, H.-Q. Mao and K. W. Leong, Biomacromolecules, 2005, 6, 341–350 CrossRef CAS PubMed.
- X.-Q. Zhang, E. Iype, S. V. Nedea, A. P. Jansen, B. M. Szyja, E. J. Hensen and R. A. van Santen, J. Phys. Chem. C, 2014, 118, 6882–6886 CrossRef CAS.
- X.-Q. Zhang, T. T. Trinh, R. A. van Santen and A. P. Jansen, J. Phys. Chem. C, 2011, 115, 9561–9567 CrossRef CAS.
- X.-Q. Zhang, R. A. van Santen and E. J. Hensen, ACS Catal., 2014, 5, 596–601 CrossRef.
- N. Rammohan, K. W. MacRenaris, L. K. Moore, G. Parigi, D. J. Mastarone, L. M. Manus, L. M. Lilley, A. T. Preslar, E. A. Waters, A. Filicko, C. Luchinat, D. Ho and T. J. Meade, Nano Lett., 2016, 16, 7551–7564 CrossRef CAS PubMed.
- L. M. Manus, D. J. Mastarone, E. A. Waters, X.-Q. Zhang, E. A. Schultz-Sikma, K. W. MacRenaris, D. Ho and T. J. Meade, Nano Lett., 2009, 10, 484–489 CrossRef PubMed.
- E. K.-H. Chow and D. Ho, Sci. Transl. Med., 2013, 5, 216rv214 Search PubMed.
- E. K. Chow, X.-Q. Zhang, M. Chen, R. Lam, E. Robinson, H. Huang, D. Schaffer, E. Osawa, A. Goga and D. Ho, Sci. Transl. Med., 2011, 3, 73ra21 Search PubMed.
- D. Ho, C.-H. K. Wang and E. K.-H. Chow, Sci. Adv., 2015, 1, e1500439 Search PubMed.
- H.-J. Kim, K. Zhang, L. Moore and D. Ho, ACS Nano, 2014, 8, 2998–3005 CrossRef CAS PubMed.
- L. Moore, J. Yang, T. T. H. Lan, E. Osawa, D.-K. Lee, W. D. Johnson, J. Xi, E. K.-H. Chow and D. Ho, ACS Nano, 2016, 10, 7385–7400 CrossRef CAS PubMed.
- Y. Li and J. Shi, Adv. Mater., 2014, 26, 3176–3205 CrossRef CAS PubMed.
- D. B. Chithrani, S. Jelveh, F. Jalali, M. van Prooijen, C. Allen, R. G. Bristow, R. P. Hill and D. A. Jaffray, Radiat. Res., 2010, 173, 719–728 CrossRef CAS PubMed.
- S. Wang, X. Li, Y. Chen, X. Cai, H. Yao, W. Gao, Y. Zheng, X. An, J. Shi and H. Chen, Adv. Mater., 2015, 27, 2775–2782 CrossRef CAS PubMed.
- Q. He and J. Shi, Adv. Mater., 2014, 26, 391–411 CrossRef CAS PubMed.
- M. Wu, Q. Meng, Y. Chen, Y. Du, L. Zhang, Y. Li, L. Zhang and J. Shi, Adv. Mater., 2015, 27, 215–222 CrossRef CAS PubMed.
- J. Y. R. Bang, C. Ting, P. Wang, T. Kim, K. K. Wang, T. Kee, D. Miya, D. Ho and D.-K. Lee, J. Oral. Implantol., 2018, 44, 207–211 CrossRef PubMed.
- P. Wang, W. Su and X. Ding, Diamond Relat. Mater., 2018, 88, 43–50 CrossRef CAS.
- B. Kim, H.-B. Pang, J. Kang, J.-H. Park, E. Ruoslahti and M. J. Sailor, Nat. Commun., 2018, 9, 1969 CrossRef PubMed.
- C. Zavaleta, D. Ho and E. J. Chung, SLAS Technol., 2018, 23, 281–293 Search PubMed.
- C. J. Bowerman, J. D. Byrne, K. S. Chu, A. N. Schorzman, A. W. Keeler, C. A. Sherwood, J. L. Perry, J. C. Luft, D. B. Darr and A. M. Deal, Nano Lett., 2016, 17, 242–248 CrossRef PubMed.
- A. J. Tavares, W. Poon, Y.-N. Zhang, Q. Dai, R. Besla, D. Ding, B. Ouyang, A. Li, J. Chen and G. Zheng, Proc. Natl. Acad. Sci. U. S. A., 2017, 114, E10871–E10880 CrossRef CAS PubMed.
- Y. Zhang, J. Yu, L. Qiang and Z. Gu, Sci. China: Life Sci., 2018, 1–7 Search PubMed.
- A. Dolor and F. C. Szoka Jr, Mol. Pharmaceutics, 2018, 15, 2069–2083 CrossRef CAS PubMed.
- C. Rejeeth, R. Vivek, V. NipunBabu, A. Sharma, X. Ding and K. Qian, MedChemComm, 2017, 8, 2055–2059 RSC.
- Y. Min, K. C. Roche, S. Tian, M. J. Eblan, K. P. McKinnon, J. M. Caster, S. Chai, L. E. Herring, L. Zhang and T. Zhang, Nat. Nanotechnol., 2017, 12, 877 CrossRef CAS PubMed.
- L. Tang, Y. Zheng, M. B. Melo, L. Mabardi, A. P. Castaño, Y.-Q. Xie, N. Li, S. B. Kudchodkar, H. C. Wong and E. K. Jeng, Nat. Biotechnol., 2018, 36, 707 CAS.
- F. C. Lam, S. W. Morton, J. Wyckoff, T.-L. Han, M. K. Hwang, A. Maffa, E. Balkanska-Sinclair, M. B. Yaffe, S. R. Floyd and P. T. Hammond, Nat. Commun., 2018, 9, 1991 CrossRef PubMed.
- R. Peng, X. Zheng, Y. Lyu, L. Xu, X.-B. Zhang, G. Ke, Q. Liu, C. You, S. Huan and W. Tan, J. Am. Chem. Soc., 2018, 140, 9793–9796 CrossRef CAS PubMed.
- E. J. Chung, Exp. Biol. Med., 2016, 241, 891–898 CrossRef CAS PubMed.
- W. Chen, Y. Wang, M. Qin, X. Zhang, Z. Zhang, X. Sun and Z. Gu, ACS Nano, 2018, 12, 5995–6005 CrossRef CAS PubMed.
- G. Feng, J. Liu, C.-J. Zhang and B. Liu, ACS Appl. Mater. Interfaces, 2018, 10, 11546–11553 CrossRef CAS PubMed.
- Y. Xie, Y. Liu, J. Yang, Y. Liu, F. Hu, K. Zhu and X. Jiang, Angew. Chem., Int. Ed., 2018, 57, 3958–3962 CrossRef CAS PubMed.
- M. Tanzid, N. J. Hogan, H. Robatjazi, A. Veeraraghavan and N. J. Halas, J. Opt., 2018, 20, 054001 CrossRef.
- W. Yin, T. Bao, X. Zhang, Q. Gao, J. Yu, X. Dong, L. Yan, Z. Gu and Y. Zhao, Nanoscale, 2018, 10, 1517–1531 RSC.
- H. Meng and A. E. Nel, Adv. Drug Delivery Rev., 2018, 130, 50–57 CrossRef CAS PubMed.
- C. E. Wang, R. C. Yumul, J. Lin, Y. Cheng, A. Lieber and S. H. Pun, J. Controlled Release, 2018, 272, 9–16 CrossRef CAS PubMed.
- C. H. Kapadia, J. R. Melamed and E. S. Day, BioDrugs, 2018, 32, 297–309 CrossRef CAS PubMed.
- S. L. Chang, A. S. Barnard, C. Dwyer, C. Boothroyd, E. Osawa and R. Nicholls, Microsc. Microanal., 2016, 22, 1392–1393 CrossRef.
- H. Jo and C. Ban, Exp. Mol. Med., 2016, 48, e230 CrossRef CAS PubMed.
- J. Liu, T. Wei, J. Zhao, Y. Huang, H. Deng, A. Kumar, C. Wang, Z. Liang, X. Ma and X.-J. Liang, Biomaterials, 2016, 91, 44–56 CrossRef CAS PubMed.
- Q. Zhou, Y. Li, Y. Zhu, C. Yu, H. Jia, B. Bao, H. Hu, C. Xiao, J. Zhang and X. Zeng, J. Controlled Release, 2018, 275, 67–77 CrossRef CAS PubMed.
- D. Zhang, Y. Y. Kong, J. H. Sun, S. J. Huo, M. Zhou, Y. L. Gui, X. Mu, H. Chen, S. Q. Yu and Q. Xu, Int. J. Nanomed., 2017, 12, 2081 CrossRef CAS PubMed.
- S.-S. Qi, J.-H. Sun, H.-H. Yu and S.-Q. Yu, Drug Delivery, 2017, 24, 1909–1926 CrossRef CAS PubMed.
- J. M. Karp and D. Peer, Nanotechnology, 2018, 29, 4 CrossRef PubMed.
- Q.-Y. Lin, E. Palacios, W. Zhou, Z. Li, J. A. Mason, Z. Liu, H. Lin, P.-C. Chen, V. P. Dravid and K. Aydin, Nano Lett., 2018, 18, 2645–2649 CrossRef CAS PubMed.
- N. DiStasio, S. Lehoux, A. Khademhosseini and M. Tabrizian, Materials, 2018, 11, 754 CrossRef PubMed.
- G. L. Drusano, M. Neely, M. Van Guilder, A. Schumitzky, D. Brown, S. Fikes, C. Peloquin and A. Louie, PLoS One, 2014, 9, e101311 CrossRef PubMed.
- A. H. Gradman, J. N. Basile, B. L. Carter, G. L. Bakris and A. S. o. H. W. Group, J. Am. Soc. Hypertens., 2010, 4, 90–98 CrossRef CAS PubMed.
- T. Tängdén, Upsala J. Med. Sci., 2014, 119, 149–153 CrossRef PubMed.
- J. R. Pritchard, P. M. Bruno, L. A. Gilbert, K. L. Capron, D. A. Lauffenburger and M. T. Hemann, Proc. Natl. Acad. Sci. U. S. A., 2013, 110, E170–E179 CrossRef CAS PubMed.
- S. Teeraananchai, S. Kerr, J. Amin, K. Ruxrungtham and M. Law, HIV Med., 2017, 18, 256–266 CrossRef CAS PubMed.
- A. M. Anderson, J. Harezlak, A. Bharti, D. Mi, M. J. Taylor, E. S. Daar, G. Schifitto, J. Zhong, J. R. Alger and M. S. Brown, J. Acquired Immune Defic. Syndr., 2015, 69, 29 CrossRef CAS PubMed.
- T. Gumbo, J. G. Pasipanodya, P. Wash, A. Burger and H. McIlleron, Antimicrob. Agents Chemother., 2014, 58, 6111–6115 CrossRef PubMed.
- Y. Hu, H. Cheng, X. Zhao, J. Wu, F. Muhammad, S. Lin, J. He, L. Zhou, C. Zhang and Y. Deng, ACS Nano, 2017, 11, 5558–5566 CrossRef CAS PubMed.
- Y. Li, J. Jin, D. Wang, J. Lv, K. Hou, Y. Liu, C. Chen and Z. Tang, Nano Res., 2018, 11, 3294–3305 CrossRef CAS.
- R. García-Álvarez, M. Hadjidemetriou, A. Sánchez-Iglesias, L. M. Liz-Marzán and K. Kostarelos, Nanoscale, 2018, 10, 1256–1264 RSC.
- S. A. Jensen, E. S. Day, C. H. Ko, L. A. Hurley, J. P. Luciano, F. M. Kouri, T. J. Merkel, A. J. Luthi, P. C. Patel and J. I. Cutler, Sci. Transl. Med., 2013, 5, 209ra152 RSC.
- D.-K. Lee, T. Kee, Z. Liang, D. Hsiou, D. Miya, B. Wu, E. Osawa, E. K.-H. Chow, E. C. Sung and M. K. Kang, Proc. Natl. Acad. Sci. U. S. A., 2017, 114, 9445–9454 CrossRef PubMed.
- D.-K. Lee, S. V. Kim, A. N. Limansubroto, A. Yen, A. Soundia, C.-Y. Wang, W. Shi, C. Hong, S. Tetradis, Y. Kim, N.-H. Park, M. K. Kang and D. Ho, ACS Nano, 2015, 9, 11490–11501 CrossRef CAS PubMed.
- C. Hong, D. Song, D.-K. Lee, L. Lin, H. C. Pan, D. Lee, P. Deng, Z. Liu, D. Hadaya and H.-L. Lee, Proc. Natl. Acad. Sci. U. S. A., 2017, 114, E7218–E7225 CrossRef CAS PubMed.
- U. Bulbake, S. Doppalapudi, N. Kommineni and W. Khan, Pharmaceutics, 2017, 9, 12 CrossRef PubMed.
- E. Kipps, K. Young and N. Starling, Ther. Adv. Med. Oncol., 2017, 9, 159–170 CrossRef CAS PubMed.
- E. C. Chen, A. T. Fathi and A. M. Brunner, OncoTargets Ther., 2018, 11, 3425 CrossRef PubMed.
- A. Wang-Gillam, C.-P. Li, G. Bodoky, A. Dean, Y.-S. Shan, G. Jameson, T. Macarulla, K.-H. Lee, D. Cunningham and J. F. Blanc, Lancet, 2016, 387, 545–557 CrossRef CAS.
- L. Huang, Y. Jiang and Y. Chen, Sci. Rep., 2017, 7, 40752 CrossRef CAS PubMed.
- R. J. Tallarida, Genes Cancer, 2011, 2, 1003–1008 CrossRef PubMed.
- D. Yu, E. Kahen, C. L. Cubitt, J. McGuire, J. Kreahling, J. Lee, S. Altiok, C. C. Lynch, D. M. Sullivan and D. R. Reed, Sci. Rep., 2015, 5, 16991 CrossRef CAS PubMed.
- W. Fan, B. Shen, W. Bu, F. Chen, K. Zhao, S. Zhang, L. Zhou, W. Peng, Q. Xiao and H. Xing, J. Am. Chem. Soc., 2013, 135, 6494–6503 CrossRef CAS PubMed.
- Y. Liu, Y. Liu, W. Bu, C. Cheng, C. Zuo, Q. Xiao, Y. Sun, D. Ni, C. Zhang and J. Liu, Angew. Chem., 2015, 127, 8223–8227 CrossRef.
- J. Liu, Y. Liu, W. Bu, J. Bu, Y. Sun, J. Du and J. Shi, J. Am. Chem. Soc., 2014, 136, 9701–9709 CrossRef CAS PubMed.
- J.-H. Park, L. Gu, G. Von Maltzahn, E. Ruoslahti, S. N. Bhatia and M. J. Sailor, Nat. Mater., 2009, 8, 331 CrossRef CAS PubMed.
- X. Wang, J. H. Lee, R. Li, Y. P. Liao, J. Kang, C. H. Chang, L. M. Guiney, V. Mirshafiee, L. Li and J. Lu, Small, 2018, 14, 1703915 CrossRef PubMed.
- Y. Li, J. Wang, F. Zhao, B. Bai, G. Nie, A. E. Nel and Y. Zhao, Natl. Sci. Rev., 2017, 5, 365–388 CrossRef.
- X. Wang, Y. P. Liao, D. Telesca, C. H. Chang, T. Xia and A. E. Nel, Small, 2017, 13, 1700776 CrossRef PubMed.
- B. Pelaz, C. Alexiou, R. A. Alvarez-Puebla, F. Alves, A. M. Andrews, S. Ashraf, L. P. Balogh, L. Ballerini, A. Bestetti and C. Brendel, ACS Nano, 2017, 11, 2313–2381 CrossRef CAS PubMed.
- A. Khademhosseini, W. W. Chan, M. Chhowalla, S. C. Glotzer, Y. Gogotsi, J. H. Hafner, P. T. Hammond, M. C. Hersam, A. Javey and C. R. Kagan, ACS Nano, 2017, 11, 1123–1126 CrossRef CAS PubMed.
- W. W. Chan, M. Chhowalla, S. Glotzer, Y. Gogotsi, J. H. Hafner, P. T. Hammond, M. C. Hersam, A. Javey, C. R. Kagan and A. Khademhosseini, ACS Nano, 2016, 10, 10615–10617 CrossRef CAS PubMed.
- X. Liu, A. Situ, Y. Kang, K. R. Villabroza, Y. Liao, C. H. Chang, T. Donahue, A. E. Nel and H. Meng, ACS Nano, 2016, 10, 2702–2715 CrossRef CAS PubMed.
- W. J. Parak, A. E. Nel and P. S. Weiss, ACS Nano, 2015, 9, 6637–6640 CrossRef CAS PubMed.
- Q. He, Z. Zhang, Y. Gao, J. Shi and Y. Li, Small, 2009, 5, 2722–2729 CrossRef CAS PubMed.
- L. Pan, Q. He, J. Liu, Y. Chen, M. Ma, L. Zhang and J. Shi, J. Am. Chem. Soc., 2012, 134, 5722–5725 CrossRef CAS PubMed.
- Q. He and J. Shi, J. Mater. Chem., 2011, 21, 5845–5855 RSC.
- J. Liu, W. Bu, L. Pan and J. Shi, Angew. Chem., 2013, 125, 4471–4475 CrossRef.
- Y. Gao, Y. Chen, X. Ji, X. He, Q. Yin, Z. Zhang, J. Shi and Y. Li, ACS Nano, 2011, 5, 9788–9798 CrossRef CAS PubMed.
- M. Yan, J. Du, Z. Gu, M. Liang, Y. Hu, W. Zhang, S. Priceman, L. Wu, Z. H. Zhou and Z. Liu, Nat. Nanotechnol., 2010, 5, 48 CrossRef CAS PubMed.
- Y.-L. Luo, C.-F. Xu, H.-J. Li, Z.-T. Cao, J. Liu, J.-L. Wang, X.-J. Du, X.-Z. Yang, Z. Gu and J. Wang, ACS Nano, 2018, 12, 994–1005 CrossRef CAS PubMed.
- C.-g. Qian, Y.-l. Chen, P.-j. Feng, X.-z. Xiao, M. Dong, J.-c. Yu, Q.-y. Hu, Q.-d. Shen and Z. Gu, Acta Pharmacol. Sin., 2017, 38, 764 CrossRef CAS PubMed.
-
D. B. Pacardo, F. S. Ligler and Z. Gu, Biomedical Nanotechnology, Springer, 2017, pp. 195–208 Search PubMed.
- J. Di, J. Yu, Q. Wang, S. Yao, D. Suo, Y. Ye, M. Pless, Y. Zhu, Y. Jing and Z. Gu, Nano Res., 2017, 10, 1393–1402 CrossRef CAS.
- D. Shi, X. Xu, Y. Ye, K. Song, Y. Cheng, J. Di, Q. Hu, J. Li, H. Ju and Q. Jiang, ACS Nano, 2016, 10, 1292–1299 CrossRef CAS PubMed.
- Q. Hu, W. Sun, Y. Lu, H. N. Bomba, Y. Ye, T. Jiang, A. J. Isaacson and Z. Gu, Nano Lett., 2016, 16, 1118–1126 CrossRef CAS PubMed.
- Q. Hu, C. Qian, W. Sun, J. Wang, Z. Chen, H. N. Bomba, H. Xin, Q. Shen and Z. Gu, Adv. Mater., 2016, 28, 9573–9580 CrossRef CAS PubMed.
- Z. J. Deng, S. W. Morton, E. Ben-Akiva, E. C. Dreaden, K. E. Shopsowitz and P. T. Hammond, ACS Nano, 2013, 7, 9571–9584 CrossRef CAS PubMed.
- Y. H. Roh, J. B. Lee, K. E. Shopsowitz, E. C. Dreaden, S. W. Morton, Z. Poon, J. Hong, I. Yamin, D. K. Bonner and P. T. Hammond, ACS Nano, 2014, 8, 9767–9780 CrossRef CAS PubMed.
- J. R. Kovacs, C. Liu and P. T. Hammond, ACS Appl. Mater. Interfaces, 2015, 7, 13375–13383 CrossRef CAS PubMed.
- D. J. Schmidt, E. M. Pridgen, P. T. Hammond and J. C. Love, J. Chem. Educ., 2010, 87, 208–211 CrossRef CAS.
- J. Kim, S. W. Lee, P. T. Hammond and Y. Shao-Horn, Chem. Mater., 2009, 21, 2993–3001 CrossRef CAS.
- O. F. Khan, P. S. Kowalski, J. C. Doloff, J. K. Tsosie, V. Bakthavatchalu, C. B. Winn, J. Haupt, M. Jamiel, R. Langer and D. G. Anderson, Sci. Adv., 2018, 4, eaar8409 CrossRef PubMed.
- D. C. van der Valk, C. F. van der Ven, M. C. Blaser, J. M. Grolman, P.-J. Wu, O. S. Fenton, L. H. Lee, M. W. Tibbitt, J. L. Andresen and J. R. Wen, Nanomaterials, 2018, 8, 296 CrossRef PubMed.
- P. P. Guimarães, S. Gaglione, T. Sewastianik, R. D. Carrasco, R. Langer and M. J. Mitchell, ACS Nano, 2018, 12, 912–931 CrossRef PubMed.
- P. W. Bisso, M. Tai, H. Katepalli, N. Bertrand, D. Blankschtein and R. Langer, Nano Lett., 2017, 18, 618–628 CrossRef PubMed.
- S. Zhang, R. Langer and G. Traverso, Nano Today, 2017, 16, 82–96 CrossRef CAS.
- S. P. Nukavarapu, S. G. Kumbar, J. L. Brown, N. R. Krogman, A. L. Weikel, M. D. Hindenlang, L. S. Nair, H. R. Allcock and C. T. Laurencin, Biomacromolecules, 2008, 9, 1818–1825 CrossRef CAS PubMed.
- J. X. Zhong, J. R. Clegg, E. W. Ander and N. A. Peppas, J. Biomed. Mater. Res., Part A, 2018, 106, 1677–1686 CrossRef CAS PubMed.
- J. R. Ashton, K. D. Castle, Y. Qi, D. G. Kirsch, J. L. West and C. T. Badea, Theranostics, 2018, 8, 1782 CrossRef PubMed.
- E. J. Chung and M. Tirrell, Adv. Healthcare Mater., 2015, 4, 2408–2422 CrossRef CAS PubMed.
- L. M. Moreau, C. A. Schurman, S. Kewalramani, M. M. Shahjamali, C. A. Mirkin and M. J. Bedzyk, J. Am. Chem. Soc., 2017, 139, 12291–12298 CrossRef CAS PubMed.
- M. R. Jones, K. L. Kohlstedt, M. N. O’Brien, J. Wu, G. C. Schatz and C. A. Mirkin, Nano Lett., 2017, 17, 5830–5835 CrossRef CAS PubMed.
- M. B. Ross, J. C. Ku, M. G. Blaber, C. A. Mirkin and G. C. Schatz, Proc. Natl. Acad. Sci. U. S. A., 2015, 112, 10292–10297 CrossRef CAS PubMed.
- A. B. Chinen, C. M. Guan, J. R. Ferrer, S. N. Barnaby, T. J. Merkel and C. A. Mirkin, Chem. Rev., 2015, 115, 10530–10574 CrossRef CAS PubMed.
- R. S. Riley and E. S. Day, Small, 2017, 13, 1700544 CrossRef PubMed.
- P. S. Kowalski, U. Capasso Palmiero, Y. Huang, A. Rudra, R. Langer and D. G. Anderson, Adv. Mater., 2018, 30, 1801151 CrossRef PubMed.
- W. Tao, X. Ji, X. Zhu, L. Li, J. Wang, Y. Zhang, P. E. Saw, W. Li, N. Kong and M. A. Islam, Adv. Mater., 2018, 1802061 CrossRef PubMed.
- X. Lin, X. Chen, I. A. Riddell, W. Tao, J. Wang, G. Hollett, S. J. Lippard, O. C. Farokhzad, J. Shi and J. Wu, Nano Lett., 2018, 18, 4618–4625 CrossRef PubMed.
- X. Zhu, X. Ji, N. Kong, Y. Chen, M. Mahmoudi, X. Xu, L. Ding, W. Tao, T. Cai and Y. Li, ACS Nano, 2018, 12, 2922–2938 CrossRef CAS PubMed.
- Y. Hao, Y. Chen, M. Lei, T. Zhang, Y. Cao, J. Peng, L. Chen and Z. Qian, Adv. Ther., 2018, 1, 1800008 CrossRef.
- H. Y. Yang, Y. Li and D. S. Lee, Adv. Ther., 2018, 1, 1800011 CrossRef.
- P. Angsantikul, S. Thamphiwatana, Q. Zhang, K. Spiekermann, J. Zhuang, R. H. Fang, W. Gao, M. Obonyo and L. Zhang, Adv. Ther., 2018, 1, 1800016 CrossRef PubMed.
- V. Krishnan, A. Sarode, R. Bhatt, J. D. Oliveira, T. D. Brown, Y. Jiang, J. Reddy Junutula and S. Mitragotri, Adv. Ther., 2018, 1, 1800010 CrossRef.
- K. Gold, B. Slay, M. Knackstedt and A. K. Gaharwar, Adv. Ther., 2018, 1700033 CrossRef.
- A. Sahu, W. I. Choi and G. Tae, Adv. Ther., 2018, 1, 1800026 CrossRef.
- R. Liu, L. Zhang, J. Zhao, Z. Luo, Y. Huang and S. Zhao, Adv. Ther., 2018, 1800041, DOI:10.1002/adtp.201800041.
- A. Alford, M. Rich, V. Kozlovskaya, J. Chen, J. Sherwood, M. Bolding, J. Warram, Y. Bao and E. Kharlampieva, Adv. Ther., 2018, 1800051 CrossRef.
- X. He, S. I. Abrams and J. F. Lovell, Adv. Ther., 2018, 1800060, DOI:10.1002/adtp.201800060.
- S. Zhu, Z. Gu and Y. Zhao, Adv. Ther., 2018, 1800050, DOI:10.1002/adtp.201800050.
- S. Wang, F. Li, X. Hu, M. Lv, C. Fan and D. Ling, Adv. Ther., 2018, 1800059, DOI:10.1002/adtp.201800059.
- A. Zarrinpar, D.-K. Lee, A. Silva, N. Datta, T. Kee, C. Eriksen, K. Weigle, V. Agopian, F. Kaldas and D. Farmer, Sci. Transl. Med., 2016, 8, 333ra349 Search PubMed.
- M. B. Mohd Abdul Rashid, T. B. Toh, A. Silva, L. Nurrul Abdullah, C.-M. Ho, D. Ho and E. K.-H. Chow, J. Lab. Autom., 2015, 20, 423–437 CrossRef PubMed.
- X. Ding, Z. Njus, T. Kong, W. Su, C.-M. Ho and S. Pandey, Sci. Adv., 2017, 3, eaao1254 CrossRef PubMed.
- B.-Y. Lee, D. L. Clemens, A. Silva, B. J. Dillon, S. Masleša-Galić, S. Nava, X. Ding, C.-M. Ho and M. A. Horwitz, Nat. Commun., 2017, 8, 14183 CrossRef CAS PubMed.
- Q. Liu, C. Zhang, X. Ding, H. Deng, D. Zhang, W. Cui, H. Xu, Y. Wang, W. Xu and L. Lv, Sci. Rep., 2015, 5, 11464 CrossRef PubMed.
- D.-K. Lee, V. Y. Chang, T. Kee, C.-M. Ho and D. Ho, SLAS Technol., 2017, 22, 276–288 Search PubMed.
- D. Ho, A. Zarrinpar and E. K.-H. Chow, ACS Nano, 2016, 9087–9092, DOI:10.1021/acsnano.6b06174.
- M. B. M. A. Rashid, T. B. Toh, L. Hooi, A. Silva, Y. Zhang, P. F. Tan, A. L. Teh, N. Karnani, S. Jha, C.-M. Ho, W. J. Chng, D. Ho and E. K.-H. Chow, Sci. Transl. Med., 2018, 10 DOI:10.1126/scitranslmed.aan0941.
- H. Wang, D.-K. Lee, K.-Y. Chen, J.-Y. Chen, K. Zhang, A. Silva, C.-M. Ho and D. Ho, ACS Nano, 2015, 9, 3332–3344 CrossRef CAS PubMed.
- S. Chakradhar, Nat. Med., 2017, 23, 1244–1247 CrossRef CAS PubMed.
- P. K. Wong, F. Yu, A. Shahangian, G. Cheng, R. Sun and C.-M. Ho, Proc. Natl. Acad. Sci. U. S. A., 2008, 105, 5105–5110 CrossRef CAS PubMed.
- A. Leber, R. Hontecillas, V. Abedi, N. Tubau-Juni, V. Zoccoli-Rodriguez, C. Stewart and J. Bassaganya-Riera, Artif. Intell. Med., 2017, 78, 1–13 CrossRef PubMed.
- R. Cai, M. Liu, Y. Hu, B. L. Melton, M. E. Matheny, H. Xu, L. Duan and L. R. Waitman, Artif. Intell. Med., 2017, 76, 7–15 CrossRef PubMed.
- P. Nowak-Sliwinska, A. Weiss, X. Ding, P. J. Dyson, H. Van Den Bergh, A. W. Griffioen and C.-M. Ho, Nat. Protoc., 2016, 11, 302 CrossRef CAS PubMed.
- I. Al-Shyoukh, F. Yu, J. Feng, K. Yan, S. Dubinett, C.-M. Ho, J. S. Shamma and R. Sun, BMC Syst. Biol., 2011, 5, 88 CrossRef PubMed.
- T. Zhang, A. Sharma, Y. Li, Y. Zhou and X. Ding, J. Photochem. Photobiol., B, 2018, 178, 568–576 CrossRef CAS PubMed.
- X. Jia, Y. Li, A. Sharma, Y. Li, G. Xie, G. Wang, J. Jiang, Y. Cheng and X. Ding, Comput. Biol. Chem., 2017, 67, 234–243 CrossRef CAS PubMed.
-
C. Lee, Z. Luo, K. Y. Ngiam, M. Zhang, K. Zheng, G. Chen, B. C. Ooi and W. L. J. Yip, in Handbook of Large-Scale Distributed Computing in Smart Healthcare, ed. S. U. Khan, A. Y. Zomaya and A. Abbas, Springer International Publishing, Cham, 2017, pp. 11–41, DOI:10.1007/978-3-319-58280-1_2.
- D. Ho and A. Zarrinpar, SLAS Technol., 2017, 22, 231–232 Search PubMed.
- A. Weiss, X. Ding, J. R. Van Beijnum, I. Wong, T. J. Wong, R. H. Berndsen, O. Dormond, M. Dallinga, L. Shen and R. O. Schlingemann, Angiogenesis, 2015, 18, 233–244 CrossRef CAS PubMed.
- A. Silva, B.-Y. Lee, D. L. Clemens, T. Kee, X. Ding, C.-M. Ho and M. A. Horwitz, Proc. Natl. Acad. Sci. U. S. A., 2016, 113, E2172–E2179 CrossRef CAS PubMed.
- A. Weiss, R. H. Berndsen, X. Ding, C.-M. Ho, P. J. Dyson, H. Van Den Bergh, A. W. Griffioen and P. Nowak-Sliwinska, Sci. Rep., 2015, 5, 14508 CrossRef CAS PubMed.
- A. Weiss and P. Nowak-Sliwinska, SLAS Technol., 2017, 22, 254–275 Search PubMed.
- A. Zimmer, I. Katzir, E. Dekel, A. E. Mayo and U. Alon, Proc. Natl. Acad. Sci. U. S. A., 2016, 113, 10442–10447 CrossRef CAS PubMed.
- A. Zimmer, A. Tendler, I. Katzir, A. Mayo and U. Alon, PLoS Biol., 2017, 15, e2002518 CrossRef PubMed.
- S. Jha and E. J. Topol, J. Am. Coll. Radiol., 2018, 15, 509–511 CrossRef PubMed.
- A. J. Pantuck, D. K. Lee, T. Kee, P. Wang, S. Lakhotia, M. H. Silverman, C. Mathis, A. Drakaki, A. S. Belldegrun and C. M. Ho, Adv. Ther., 2018, 1800104, DOI:10.1002/adtp.201800104.
- C. Harrison, Nat. Biotechnol., 2018, 36, 673 CrossRef CAS PubMed.
- L. M. Prevedello, B. S. Erdal, J. L. Ryu, K. J. Little, M. Demirer, S. Qian and R. D. White, Radiology, 2017, 285, 923–931 CrossRef PubMed.
- S. E. Dilsizian and E. L. Siegel, Curr. Cardiol. Rep., 2014, 16, 441 CrossRef PubMed.
- G. Von Maltzahn, J.-H. Park, K. Y. Lin, N. Singh, C. Schwöppe, R. Mesters, W. E. Berdel, E. Ruoslahti, M. J. Sailor and S. N. Bhatia, Nat. Mater., 2011, 10, 545 CrossRef CAS PubMed.
- X. Liu, P. Lin, I. Perrett, J. Lin, Y.-P. Liao, C. H. Chang, J. Jiang, N. Wu, T. Donahue and Z. Wainberg, J. Clin. Invest., 2017, 127, 2007–2018 CrossRef PubMed.
- X. Xu, K. Xie, X.-Q. Zhang, E. M. Pridgen, G. Y. Park, D. S. Cui, J. Shi, J. Wu, P. W. Kantoff and S. J. Lippard, Proc. Natl. Acad. Sci. U. S. A., 2013, 110, 18638–18643 CrossRef CAS PubMed.
- L. Gu, Z. J. Deng, S. Roy and P. T. Hammond, Clin. Cancer Res., 2017, 23, 7312–7323 CrossRef CAS PubMed.
- K. Kawamoto, C. A. Houlihan, E. A. Balas and D. F. Lobach, BMJ, 2005, 330, 765 CrossRef PubMed.
- Y. Ran, B. He, K. Hui, J. Xu and L. Sun, Int. J. Data Min. Bioinform., 2018, 20, 91–108 CrossRef.
- H. S. Goldberg, M. D. Paterno, R. W. Grundmeier, B. H. Rocha, J. M. Hoffman, E. Tham, M. Swietlik, M. H. Schaeffer, D. Pabbathi and S. J. Deakyne, Int. J. Med. Inform., 2016, 87, 101–110 CrossRef PubMed.
- P. Hamet and J. Tremblay, Metabolism, 2017, 69, S36–S40 CrossRef CAS PubMed.
- A. M. Williams, Y. Liu, K. R. Regner, F. Jotterand, P. Liu and M. Liang, Physiol. Genomics, 2018, 50, 237–243 CrossRef PubMed.
- M. J. Zellweger, A. Tsirkin, V. Vasilchenko, M. Failer, A. Dressel, M. E. Kleber, P. Ruff and W. März, EPMA J., 2018, 1–13 Search PubMed.
- A. Guglielmo, N. Staropoli, M. Giancotti and M. Mauro, Cost Eff. Resour. Alloc., 2018, 16, 2 CrossRef PubMed.
- Z. N. Warnken, H. D. Smyth, D. A. Davis, S. Weitman, J. G. Kuhn and R. O. Williams III, Mol. Pharmaceutics, 2018, 15, 1392–1402 CrossRef CAS PubMed.
- D. Shinko, C. I. Diakos, S. J. Clarke and K. A. Charles, Clin. Pharmacol. Ther., 2017, 102, 599–610 CrossRef PubMed.
|
This journal is © The Royal Society of Chemistry 2019 |
Click here to see how this site uses Cookies. View our privacy policy here.