DOI:
10.1039/C8LC00684A
(Tutorial Review)
Lab Chip, 2019,
19, 11-34
Cancer diagnosis: from tumor to liquid biopsy and beyond
Received
30th June 2018
, Accepted 11th November 2018
First published on 13th November 2018
Abstract
Technological advancements in research on circulating biomarkers from patient derived blood have enabled a less invasive means of diagnosing non-hematologic cancers. Considered a more practical way of real-time patient monitoring than traditional tumor biopsy, liquid biopsy markers including circulating tumor cells (CTCs), circulating tumor DNA (ctDNA) and extracellular vesicles (EVs) and exosomes certainly have the potential to change the dynamics of cancer management and treatment. Liquid biopsy essentially presents a snapshot of the disease from the primary and/or distant tumor locations and can be utilized for repeated sampling of tumor markers to adjust therapy according to the patient's response to treatment, also known as personalized or precision treatment. In this review, we discuss the research progress in this field with respect to each of the liquid biopsy markers ranging from CTCs, EVs to ctDNA. First, we highlight key CTC technologies that have been commercialized and extensively employed for patient sample analysis. Next, we present some recent developments with regards to exosome and ctDNA research. We then conclude with some future perspectives on the areas of research for these biomarkers. Taken together, we believe these non-invasive capabilities and their potential for diagnostic development can influence treatment selections and aid precision cancer therapies.
1. Introduction
Prognosis of cancer is largely related to the examination of a detectable primary tumor or resulting colonization of disseminated cancer cells that migrate to other sites within the body.1,2 The appearance of clinically detectable metastases can take years from the onset of cancer and underlying mechanism of this observed dormancy remains unknown. Despite emerging targeted therapies changing the course of cancer treatment, the associated tumor heterogeneity and clonal selection or evolution result in tumor resistance towards such treatments. With efficacy of targeted therapies being specific for cancer subtypes, it is imperative that appropriate sub-classification of cancer be done to minimize treatment implications on the patient.3 For instance, in the case of non-small cell lung cancer, folate inhibitor was found to be effective in treating adenocarcinomas and poorly differentiated non-small cell carcinomas, but ineffective for squamous cell carcinomas.4 Thus, specific subtyping of cancer does impact the selection or removal of specific target agents during treatment.
Tumor tissue biopsy, considered the gold standard of cancer subtyping, provides only a snapshot of the disease and is rather difficult to obtain in several cases. Partial sampling and non-specific findings during immunohistochemical tumor analysis show discrepancies in the diagnosis of patients and subsequently result in ineffective therapy.5,6 Further, routine monitoring requires the patient to endure pain during multiple biopsy procedures in the course of treatment. To this end, research advances made in cancer research and biomedical technology have enabled development of new diagnostic tools to complement conventional biopsies through non-invasive sampling of bodily fluids known as liquid biopsy. This ability to obtain information on non-hematologic cancers has been one of the most exciting breakthroughs that has driven major advances in cancer diagnostics. With liquid biopsy promising to provide a real-time feedback on patient condition, it has certainly increased the applicability of cellular and molecular therapeutics that rely on systematic and routine measurements of critical biomarkers.7 These biomarkers include tumor-derived entities such as circulating tumor cells (CTCs), circulating tumor DNA (ctDNA) and extracellular vesicles (EVs), regarded to be breakaway products or entities released from cells within the primary tumor and believed to play major roles in metastasis. While CTCs and ctDNA present in blood have already demonstrated potential to present corresponding information on possible therapeutic targets and resistance mechanisms,8,9 EVs on the contrary present in several bodily fluids (urine, saliva, breast milk, cerebrospinal fluid etc.) including blood are believed to be genetic messengers and present an alternative mode of cancer progression.
1.1 Liquid biopsy markers: discovery and progress
Each of these representative biomarkers discovered at different time periods offer immense potential together or as standalone liquid biopsy biomarkers for cancer. Fig. 1 represents key landmark events that demonstrated the potential of CTCs (red), ctDNA (blue) and EVs (orange) as cancer biomarkers, from discovery through to recent technological developments in isolation and analysis of these biomarkers. CTCs are believed to be cells that detach from the primary and metastatic tumor masses into the bloodstream. CTCs are believed to comprise metastatic cell population and represent tumor traits including intratumor heterogeneity and clonal evolution.10,11 With CTCs and their prognostic potential being explored extensively in the last few decades, their earliest observations date back to early 19th century when initial observations of breakaway tumor cells were reported by Récamier in 1829.12 Following this, in 1869, Thomas Ashworth13 reported the presence of cells identical to that of cancer and postulated that these observations could help understand the origin of multiple tumors within the same individual. He also added that these cells potentially originating from the tumor mass possess the ability to traverse into the circulatory system. These findings drove cancer research for the next hundred years in terms of investigating the role of these breakaway cancer cells in metastasis. However, the diagnostic and prognostic potential of these rare cells were realized only upon the development of technologies with the required sensitivity and selectivity. In this regard, earlier approaches of CTC isolation involved the use of simple filtration14 and sedimentation techniques.15 Post this, it took over three decades to establish the presence and correlation of CTCs in cancer progression during which Racila et al., came up with an epithelial cell adhesion molecule (EpCAM) based immunomagnetic separation approach.16 This led to the first technique being approved by the Food and Drug Administration (FDA) and used for clinical detection of CTC.17 Simultaneously, microfluidics was being viewed as an alternative platform approach due to the low shear and controlled fluid flow that can maximize cell–antibody interactions thereby maximizing the throughput. In this regard, Nagrath et al., developed a microfluidic approach popularly known as CTC-chip containing antibody coated microposts for the isolation of CTCs from blood samples.18 Despite persistent efforts in developing new isolation technologies, multiple investigations revealed poor prognosis related to elevated CTC counts suggesting the need for improved cancer cell analysis to establish correlation with patient survival rates.19,20 Thus, the landscape of cancer therapy shifted focus from enumeration of CTC counts towards characterization of retrieved cells to establish their potential clinical utility. This meant establishment of new enumeration strategies apart from just EpCAM based isolation and also accounting for substantial heterogeneity of CTCs at both morphological and molecular level.21 In this regard, CTC enrichment technologies based on unbiased physical characteristics have outperformed antibody-dependent capture systems in terms of sensitivity. In particular, microfluidics-based separation technique for CTCs isolation from blood using inertial microfluidics garnered widespread attention for its simpler operation and feasibility for downstream analysis.22 This technology exploits the inherent size difference between CTCs of certain types of cancer and hematologic cells (CTCs, 10–20 μm; red blood cells (RBCs), 8 μm discoid; leukocytes, 7–12 μm), to achieve efficient CTCs separation from blood. Further, to transition from CTC enumeration to molecular characterization, technology development focused on obtaining more highly enriched CTC population (i.e., with minimal blood cell background) with greater recovery rates for amenable downstream processing.23 A vast array of technologies were developed that involved integration of multiple physical, biomechanical and immunoaffinity approaches to retrieve cells whilst also being able to profile cells based on tumor marker expression. Despite the ingenuity of these techniques, heterogeneity among CTCs at the single cell level with progressive loss of marker expression and genotypic information suggest the need for more sensitive isolation techniques.24 This deeper understanding of intra-tumor and inter-cellular heterogeneity is the current need of CTC research, with technology development being driven towards single cell profiling.
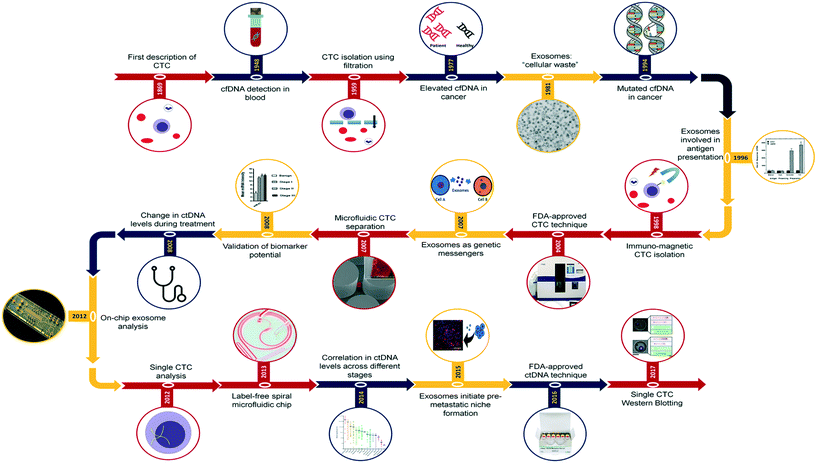 |
| Fig. 1 Research milestones in liquid biopsy research. Schematic representation of key milestones in liquid biopsy research that covers biomarkers including CTCs, ctDNA and EVs. Images were adapted and reproduced with permission from ref. 42. Copyright (1996) Rockefeller University Press, ref. 18. Copyright (2007) Nature Publishing Group, ref. 163 Copyright (2012) Nature Publishing Group, ref. 22 Copyright (2013) Nature Publishing Group, ref. 84 Copyright (2017) Nature Publishing Group. | |
Similar to CTCs, some fragments of DNA known as ctDNA, shed into the bloodstream are believed to be derived from apoptotic or necrotic cells. Since a fraction of cell-free DNA (cfDNA) is also contributed by normal host cells, they increase the background noise for ctDNA analysis, particularly during therapy or analysis during surgeries.25 Despite the mechanism of ctDNA release being poorly understood, they hold tremendous potential as tumor biomarkers. Widespread interest in cfDNA research occurred as early as 1948, when Mandel and Metais26 described the presence of DNA outside the cell, circulating freely in plasma. However, the release of cfDNA by tumor cells was first identified by Kunkel in 1973, when a decrease in cfDNA levels was observed in patients undergoing chemotherapy.27 Over the years, many studies have consistently detected higher levels of ctDNA in cancer patients, especially if the sample was taken close to a tumor site.28,29 Several subsequent studies demonstrated a quantitative correlation between ctDNA levels and tumor burden, with ctDNA also believed to comprise hallmark mutations associated with cancer.30–33 However, the clinical potential of ctDNA was first established through prenatal investigations on women with male foetuses comprising Y chromosomal DNA from cfDNA in their plasma.34 This research instigated the use of blood test during pregnancy to determine fetal gender and any chromosomal abnormalities.35 Further, it also led to several investigations on the use of cfDNA as a diagnostic tool with results suggesting the potential of ctDNA to outperform other more extensively researched biomarkers such as CTCs as a diagnostic marker. While increasing levels of both CTCs and ctDNA indicate poor prognosis in patients, ctDNA had a marked increase in sensitivity over CTC in determining the tumor burden.36 With the viability of cfDNA in blood found to range between 16 minutes to 2.5 hours,31 these findings have led to the belief that ctDNA could be used as a real-time biomarker for cancer diagnosis. As with any biomarker, the first step would be first to isolate it before any analysis can take place. Particularly for ctDNA, the quantities that are available per ml are minute. This is because cfDNA concentrations have been found to range between 1 to 10 ng per ml of plasma37 and between 1 to hundreds of ng per ml of plasma, depending on the type of cancer, in healthy individuals and cancer patients, respectively. Recent advances in high-throughput sequencing and sophisticated computational methods has improved the ability to detect and characterize ctDNA. Further, recent techniques have also been able to identify single-point mutations and track multiple genes of interest with increasing sensitivity. Recently, Roche's ctDNA-based detection of epidermal growth factor receptor (EGFR) mutations, a companion diagnostic for erlotinib in lung cancer patients, was the first liquid biopsy to obtain FDA approval.38 These companion diagnostic liquid biopsies can significantly increase the feasibility of targeted therapy for patients where tumors are difficult to access safely.
In addition to CTCs and ctDNA, there is mounting evidence on nano-sized vesicles released by most cells into bodily fluids (e.g., blood, saliva, urine etc.) and believed to comprise vital information (e.g., mRNAs, microRNAs and proteins) representative of the parent cell or tumor.39 Generally recognized as exosomes, these vesicles derived from tumor cells contribute to cancer progression by facilitating local and systemic cell communication, e.g., between the primary tumor and bone marrow cells.40 Early observations were made in 1970s when endosome like structures carried certain materials into cells and simultaneously release vesicle shaped structures.41 Considered as ‘cellular waste’ for several years, these mysterious molecules were believed to have no specific function. However, certain key observations on their role in mediating immune responses42 rekindled interest in understanding their role in several biological mechanisms. Since then, research into these nano-sized extracellular vesicles (40–200 nm) of endocytic origin has gained widespread interest. Although the biogenesis of these EVs still remains elusive, they are believed to originate from the intraluminal budding of multivesicular bodies (MVBs) with late endosomal membrane. The release of EVs is associated with membrane inversion events that occur upon fusion with the plasma membrane and the membrane budding results in encapsulation of cytoplasmic RNA, protein and even DNA.39 These vesicles carrying a cargo of biomolecules possibly derived from the parent cell possess the ability to alter the biochemical composition, signalling pathways, and genomic state of any recipient cell. Similarly, EVs released from tumor cells are believed to mediate communication between the primary tumor and bone marrow cells via transfer of molecular contents derived from the parent cell.43 The biggest advantage of EV analysis over other blood-based markers such as CTCs (1–100 cells per mL of blood) is the access to a larger population of biomarkers in EVs (8.0 × 103 to 5.0 × 105 EVs per μL in biological fluids such as serum, blood, plasma etc.).44 Thus, they can be regarded as novel disease biomarkers that represent a simple and non-invasive source of profiling the parent tumor or cell type.
Several reviews about CTC and ctDNA published over the last few years.2,45–48 In this review, we will focus on key CTC technologies that have been involved in clinical validation or utility including several commercialized techniques. Further, we also describe some of the latest techniques for ctDNA and exosome isolation thereby suggesting a promising perspective on liquid biopsy research.
2. Challenges in liquid biopsy research
The peripheral blood is the most common liquid biopsy source containing a pool of cells and/or DNA fragments derived from the primary tumor and different metastatic sites.49 Analysis of these cellular and molecular materials are the cornerstones of liquid biopsy diagnostics. Although the extent of accuracy on the information obtained from liquid biopsy remains arguable, they are still the most potent form of obtaining tumor-related information. Furthermore, liquid biopsy diagnostics may enable focused screening of populations at higher risk, which will drastically reduce healthcare costs. However, despite promising results, early disease diagnosis requires diagnostic tools with high sensitivity and specificity. For instance, conditions for ctDNA analysis must be standardised despite the ease of being able to obtain ctDNA from serum samples compared to CTCs from whole blood.50 ctDNA levels in these samples are generally affected by dilution by normal DNAs from dying blood cells. On the other hand, recent developments in CTC biology has shifted focus towards new technological developments for CTC detection rather than the introduction of CTCs into clinical diagnostics.
Over the past decade, a plethora of CTC technologies has been developed focusing on CTC retrieval from patient blood sample non-differentially with limited or no blood cell contamination within a reasonable time period. However, extremely high number of blood cells and viscosity of blood limit the throughput of separation in these devices, which also makes it hard to derive CTCs with high purity. Most methods developed earlier isolated CTCs using epithelial markers such as EpCAM and identified candidate CTCs using cytokeratin markers, which are absent in cells of mesenchymal phenotype. With CTCs undergoing an epithelial-to-mesenchymal transition (EMT), the reduced expression of epithelial markers, increased plasticity and capacity for migration make the isolation of CTCs even more challenging. Besides, traditional enumeration methods based on epithelial markers can only access limited subtypes of CTCs, making it hard to establish a standard evaluation procedure for all cancer patients. For instance, CellSearch®51 as the first FDA approved CTC isolation technique, provides CTC counts in peripheral blood as a reference for cancer prognosis and is a commonly used approach to establish clinical relationship of CTCs with cancer progression. CTC enumeration in metastatic cancer patients is directly related with patient survival in breast, prostate, and lung cancers and it is a reliable indicator of prognosis of surgery and cancer treatment. However, CTC enumeration gives limited information and the reading can vary due to individual difference and cancer cell heterogeneity.
In the past decade, studies on CTCs have gone far further than simple cell counts. Current techniques and platforms have allowed researchers to characterize CTCs from a single cell gene level. DNA and RNA expression level in CTCs can be used to facilitate the assessment and understanding of cancer therapy, especially their failure. CTC counts have been investigated for this specific purpose, and with lack of understanding of the relationship of CTCs in different cancer conditions, it is possible to use other information, e.g. RNA and DNA expression, as new reference for patient condition monitoring. Research in castration-resistant prostate cancer (CRPC) showed that the expression of AR-V7 mRNA in CTCs is associated with resistance to enzalutamide and abiraterone therapies, demonstrating that the failure of these treatments is related to androgen signaling.52 A follow-up study on the RNA-seq of clinical CTC samples showed that the detection of AR-V7 is associated with androgen-receptor targeting therapies because of the lack of ligand-binding domain of protein encoded by AR-V7.53 The result provides clinicians with a new perspective that RNA levels of CTCs from cancer patients can potentially be used for treatment stratification. Besides, the levels of DNA/RNA/protein expression are good quantitative parameters for dynamic monitoring of cancer therapy. The separation of CTCs is only a starting point of CTC related liquid biopsy, as the ultimate goal of current research is to obtain information on the physiological status of a patient from the CTCs and use the knowledge to enable a more effective treatment for the patient.
Besides these considerations, several key questions about the biology and clinical relevance of ctDNA or EVs still need better understanding. Several hypotheses require further investigation including the potential release of ctDNA by tumor cells or cells undergoing apoptosis.46 Earlier approaches for ctDNA focused only on alterations in commonly altered genes, thereby limiting the number of mutations analysed.54 However, recent studies focus on de novo mutations through unbiased analyses in a large number of exons or genome-wide analyses. Further, it is expected that with improvements in sensitivity of Next-Gen Sequencing (NGS) will only enhance our capabilities in detecting single alterations among wild-type molecules. This can potentially extend the applications of ctDNA from disease monitoring to detection of residual disease after surgery and early detection.
On the other hand, EV research has made significant progress in less than half a decade with their role in disease diagnosis slowly starting to evolve from being considered as cellular waste to being recognized as biological footprint of the parent tumor. Of particular interest is the surface protein expression that can provide invaluable information associated with the physiological states of the parental cells of EVs.55 Although EVs largely share several protein markers regardless of the cell type, they also carry a small fraction of proteins that are cell-specific and reflect on the type and pathophysiological conditions of those secreting cells. This overlap of surface protein markers and associated heterogeneity among exosomes derived from multiple cell types renders their isolation rather more difficult. Thus, there is still a need to establish better exosomal markers for the isolation of desired EV populations with high purity.56 Despite impressive strides made in the field, most of the microfluidic devices are still not compatible for clinical analysis due to scalability, standardization and validation.57 Further, several approaches are time-consuming, require extensive pre-treatment steps and do not recover enough EVs for protein or nucleic acid analysis. Thus, there is a need for isolation techniques that retrieve EVs selectively in a cost-effective and rapid manner. It is also important that the technique is susceptible to integration with downstream proteomic or sequencing analysis.
3. Technological advancements in liquid biopsy
3.1 Circulating tumor cells (CTCs)
The unique phenotypic and molecular characteristics of CTCs are believed to increase their metastatic potential and has in turn aided their utility as liquid biopsy. With tumor cell survival within the bloodstream being difficult it is believed that CTCs utilize a unique process for survival and initiation of metastasis that is still poorly understood.58 The cells that survive this stringent process extravasate into secondary organs, fostered by chemokines and interactions with activated platelets or macrophages. The presence of CTCs post several months of primary tumor resection is a clear indication of tumor cell recirculation into the bloodstream.59 Despite very little evidence available on tumor cell recirculation, studies on mouse model suggest the correlation of the presence of disseminated tumor cells (DTCs) in the bone marrow with metastatic and regional relapse with more aggressive tumor cell variants.60 Thus, CTC as a blood borne marker is considered a useful choice for the development of effective therapies with new targeted drug regimens. Although earlier studies on CTCs indicate negative prognostic significance in breast, colon and prostate cancers, recent studies focused on specific genetic mutations identified in CTCs are believed to be predictive of response to targeted inhibitors. Thus, CTC-based genotyping can provide a simple non-invasive strategy in obtaining clinically relevant genotypes needed for treatment selection.
In fact, as CTC separation has been extensively investigated, there are detailed reviews on the comparison of the most recent technologies with detailed discussion on different evaluating parameters.61,62 In addition, as the idea of liquid biopsy becomes increasingly popular, there are a few good recent reviews that also discuss CTCs as a biomarker for liquid biopsy.47,63,64 In this section, we plan to discuss some representative techniques based on their methodology of CTC isolation with particular emphasis on commercialized technologies and the clinical studies performed using these technologies and limitations of such technologies in clinical settings. Table 1 summarizes some of the key commercially available technologies for CTC isolation highlighting their key limitations and clinical implications.
Table 1 Commercial technologies for CTC isolation and enrichment
Technology |
Principle |
Specifications and performance |
Limitations |
Implications from clinical studies |
Ref. |
CellSearch® |
Immunoaffinity |
EpCAM labeling, CD45 |
Loss of CTCs due to EpCAM dependent strategy |
Relation between CTC count and cancer prognosis |
51
|
Magnetic force |
CK, DAPI staining |
Recovery: 80% |
Throughput: 7.5 ml/90 min |
OncoQuick® |
Density |
Recovery: 87% |
Loss of tumor cells that migrate either to the plasma fraction or to the bottom of the gradient due to the formation of aggregates |
Evaluation of treatment/metastasis. RT-PCR: 30% (11/37) of gastrointestinal carcinoma patients |
75
|
Size |
Throughput: 7.5 ml/40 min |
ISET® |
Size |
Sensitivity: 1 CTCs per 10 mL of blood |
Loss of CTC through the pores of 8 μm and contamination from WBCs |
Early diagnosis of lung cancer |
76
|
Filtration |
Throughput: 7.5 ml/40 min |
CTC-iChip |
Size |
EpCAM/CD45, CD15, CD66b |
Complicated fabrication and potential RBC contamination |
Improved sensitivity to CTC subtypes |
68
|
Immunoaffinity |
Magnetic force |
Recovery: 98.6% |
Throughput: 1–2 ml h−1 |
ClearCell® FX |
Size |
Sensitivity: 10 000× enrichment of CTCs |
Some loss of CTCs (due to different size range) through the white/red cell outlet channel |
Sequencing drug testing |
85
|
Inertial focusing |
Throughput: 7.5 ml/30 min |
OncoCEE™ |
Antibody cocktail |
EpCAM, HER2, MUC-1, EGFR, TROP-2, pre-enrichment |
Competitive antibody dependent capture and potential contamination of WBC/RBCs |
ER/PR receptor for prognosis |
67
|
Hydrodynamic |
Throughput: 16 μl min−1 |
ApoStream® |
Dielectrophoretic-FFF |
Recovery: 70% |
Pre-enrichment required which may result in low recovery |
Separation of CTC from colon tumor patients |
86
|
Collection volume: ∼1.5 mL (starting blood volume: 50 μl–10 ml) |
Throughput: 10 ml/60 min |
Parsortix™ |
Size and resistance based-system |
Recovery: >80% CTCs |
Buffy-coat enrichment and CTC loss due to different size ranges |
Harvesting recovered CTCs from patients and identification of gene alteration in prostate cancer patients |
87, 88 |
Throughput: 5 ml h−1 |
3.1.1 Immunoaffinity-based CTC enrichment.
Immunoaffinity-based CTC enrichment techniques target specific surface markers of CTC with antibodies and either pull them out using external force field or capture them in a designed structure. The first and only FDA approved CellSearch® system targets EpCAM for positive selection of CTCs from patient blood samples.65 They established a standard to identify CTCs as EpCAM+/CD45-/CK+ nucleated cells. Meanwhile, CTCs undergoing epithelial–mesenchymal-transition (EMT) can gradually lose their epithelial characteristics and thus exhibit no or very limited EpCAM expression.66 These clinical variations and the discovered EMT process happening in metastasis cancer cells cast doubts on the effectiveness of the gold standard system. To tackle this problem, immunomagnetic labeling methods stemmed from the CellSearch® system later made use of other surface markers that have been shown to present in both EMT and non-EMT CTCs together with the conventional EpCAM labeling and CD45, CK staining.67 Another strategy developed to address this issue is concentrating CTCs through negative selection. Leukocyte markers CD45 and CD15 are used to remove other nucleated cells in peripheral blood and keep only CTCs in the collection tube.68 In addition to these, the advent of microfluidics in CTC isolation has immensely improved standards of CTC research with regards to isolation efficiency, purity and ability to integrate multiple physical phenomenon that can result simultaneous isolation of EpCAM (+) and EpCAM (−) CTCs. These include separation technologies that build specific microstructures or surface for antibody-based cell capturing or concentrating in order to use antibodies for capturing CTCs on microfluidic devices, which can achieve a higher throughput with reduced volume and cost and make operation easier for users. Herringbone (HB), graphene oxide (GO) and geometrically enhanced mixing (GEM) chips all belong to this category.69–71 Micropost array labeled with antibody cocktails has also been shown as an effective way to capture CTCs with greater heterogeneity.67 Some of the key immunoaffinity based technologies include:
CTC and herringbone-chip.
One of the earliest developed microfluidic based approaches for CTC isolation from whole blood involving the use of antibody-coated (EpCAM) microposts to disrupt fluid streamlines, thereby enhancing antibody–cell interactions.18 CTC-chip with improved yield and purity as compared to CellSearch® enabled serial genotyping of lung cancers carrying EGFR mutations. The use of complex micropost structures presented scale up challenges for high-throughput operation and use of laminar flow based cell capture also decreased the purity of obtained CTC fraction. Despite these limitations, CTC-chip is a landmark technology in immunoaffinity based CTC capture. In this regard, to improve upon the performance of CTC isolation, an alternative approach-using antibody coated surface ridges or herringbones (HB-chip)72 in the wall of the device successfully generated microvortices to maximize collisions between CTCs and antibody-coated walls. With a much simpler geometry compared to CTC-chip, the HB-chip provided an improved CTC capture platform. The clinical utility of this chip was demonstrated with prostate cancer samples and CTCs were detected from 14 out of 15 patients and the tumor-specific TMPRSS2-ERG translocation was readily identified following RNA isolation and RT-PCR analysis. More importantly, it was one of the first approaches to report the presence of CTC clusters from patient samples.
CTC-iChip.
CTC-iChip developed by the same research group, CTC-iChip uses two components to enable negative and positive selection of CTCs. This is a unique capture platform with three distinct microfluidic principles integrated into a single device including, (i) debulking blood sample using deterministic lateral displacement, (ii) inertial focusing of debulked nucleated cells under asymmetric curved channels that traverse the laminar flow path, and (iii) positive or negative selection of CTCs using immunomagnetic separation (posCTC-iChip; anti-EpCAM functionalized beads) or WBC depletion (negCTC-iChip; anti-CD45 and anti-CD15 functionalized beads) post focusing of nucleated cell population. This system overcomes the shortcomings of CellSearch® system, which is limited by the ability to retrieve viable CTCs for downstream analysis.68 To verify the performance of the CTC-iChip, in a clinical study, patient samples from a broad category including breast cancer, melanoma, lung cancer, colorectal cancer and pancreatic cancer were tested using the CTC-iChip.68 The CTC-iChip shows superior sensitivity in comparison to CellSearch® especially in detecting low CTC samples (90% above threshold in CTC-iChip and 57% for CellSearch®) and also a higher number detected (3.2 cells per ml in CTC-iChip and 1.7 cells per ml for CellSearch®) from different subtypes. Besides it's operating mechanism, CTCs isolated from CTC-iChip are easy to retrieve for subsequent molecular analysis. This was also demonstrated in a clinical study where RNA expression analysis was performed on candidate CTCs. In this regard, RNA expression of 15 single CTCs from prostate cancer patient regarding 43 genes that were related with AR signaling and epithelial, mesenchymal, stem cell proliferation and WBC factors were studied. The expression pattern shows heterogeneity among different individual cells. The drawbacks of the CTC-iChip might be related to the chip fabrication process. The design is relatively complicated and the requirement for manufacturing is thus higher than several other microfluidic devices.
OncoCEE™.
OncoCEE™ is a product based on antibody labeling and capture. It addresses the issue of CTC heterogeneity by coating CTCs with a cocktail of antibodies related to cancer cells including human epidermal growth factor receptor-2 (HER2) and EGFR.67 Whole blood is pre-enriched through density gradient centrifugation followed by labeling with aforementioned antibody cocktail. The stock solution is flowed into a coated micropost array for CTC capture and captured CTCs are retained inside the channel. Clinical studies from the same group shows that the microarray can capture EpCAM negative and CK negative CTCs in breast, lung, prostate and colorectal cancer patients and the number captured is also higher compared to just anti-EpCAM based capture. However, as the cells are captured and evaluated inside the channel, it is difficult to release the CTCs from the posts, which impedes the downstream analysis of the cells. Nevertheless, this technique managed to address the heterogeneity issue of CTCs and brought a wider population of CTCs for clinical analysis. Clinical studies also demonstrated that OncoCEE™ platform is feasible to be used for protein characterization of CTCs derived from patient sample. Kalinsky et al., used OncoCEE™ to investigate the correlation between estrogen receptor (ER) and progesterone receptor (PR) status of CTC and the primary tumor, as ER and PR have been shown to have prognostic and predictive value in breast cancer. The results demonstrated high concordance between the ER/PR status and primary breast tumor (15/22, 68%) and between CTCs and metastatic tumor (19/24, 83%). This result revealed that CTCs could effectively reflect the same information as in primary tumor and they are more alike to metastatic tumors.73
3.1.2 Biophysical property-based CTC enrichment.
Besides using molecular labeling for CTCs purification, techniques probing the physical properties of CTCs also achieved great success in recent years. Fundamental studies on CTCs show their altered physical properties including density, size, deformability and dielectrophoretic properties from other nucleated blood cells or normal epithelial cells. Deterministic flow is developed to make use of the flow profile produced by the fluid flowing through the posts and separate particles with different size to different layers of the device.74 Centrifuge techniques are developed to separate CTCs through density gradient. The preparation and processing is simple and this technique is a good choice for pre-enrichment.75 Polymer porous membrane and microfiltration systems have also been developed as a high throughput, easy to operate method for CTC capture.76 However, these techniques suffer from the large number of background cells with similar biophysical property (e.g. similar size of some leukocytes with CTCs) and the extremely large cell density of whole blood has greatly compromised their effectiveness and efficiency. Deformability difference has also been considered as an important biophysical marker for both primary tumor cells and CTCs.77 Thus, it is theoretically viable to find a threshold and separate blood cells according to their deformability range by designing microstructures. A microfluidic device with ratchets structure has proved this concept, and has exhibited competence in potentially fractionating blood into different components in a single run.78 However, the biggest limitation encountered by the deformability device is the throughput of the chip, or to say throughput is the bottle neck for every technology based on physical properties for CTC isolation. To achieve a high recovery rate of CTCs, deformability based devices usually take more than one hour to process 1 mL of blood, which impedes their usage as suitable clinical setups. Dielectrophoresis (DEP) captures CTCs through the conductivity difference of cells suffer from composition and morphology factors.79 There are two strategies for isolating CTCs with DEP: 1) direct target cells to different direction of electric field by controlling the electrical frequency; 2) trap the targeted cells in a DEP cage designed based on the dielectric property of CTC.80,81
The biggest advantage of non-labeling techniques is the absence of antibodies and any biomolecules that may potentially influence the downstream analysis of the targeted cells. However, the tradeoff here is that the targeted physical parameters are not always exclusive for CTCs. Additionally, cells exhibit more versatile properties in even similar physical or physiological conditions, so it is still difficult to purely derive one specific population of CTCs from blood by only using biophysical markers. Although these approached to concentrate cells opens the door for single CTC analysis, the large number of background cells is still impeding technological developments toward single CTC analysis. Some of the key technologies in this space include:
Vortex chip.
Vortex chip is designed to trap CTCs under inertial forces. The inertial microfluidic chambers protrude from the rectangular straight channel and can effectively capture CTCs above a pre-designed size cutoff through inertial force.82 The latest version of the chip also allows detection and enumeration from both size-based and deformability based parameters by integrating a deformability cytometer component, thereby giving a higher accuracy in capturing the CTCs with minimized background leukocytes.83 As compared to the CTC-iChip, Vortex technology is simpler in manufacturing and there are even fewer steps in sample preparation. The limitation of this technology is the additional flushing step needed for CTC release and smaller cell washout, which added to the complexity of the chip. From their clinical result, the Vortex chip successfully demonstrated the separation of CTCs from breast, prostate and lung cancer patient samples with minimal white cell contamination. In another study on lung cancer, they characterized the expression of PD-L1, which is related with immune-evasion of cancer cell, in CTCs from metastatic lung cancer patients and found greater heterogeneity in CTCs from individual patients. These downstream studies demonstrated the suitability for the Vortex chip to be used in single cell related clinical studies. Recently, this chip was utilized to develop an integrated microfluidic assay to measure multiple protein targets in single CTCs.84 This chip involving single cell-resolution Western Blotting (scWB) combines polyacrylamide gel electrophoresis (PAGE) of single-CTC lysate with subsequent antibody probing of PAGE-resolved protein targets. This approach for CTC and protein separation (seconds) of isolated cell lysate maximized local protein concentrations for detection, even with low protein copy numbers (Fig. 2).
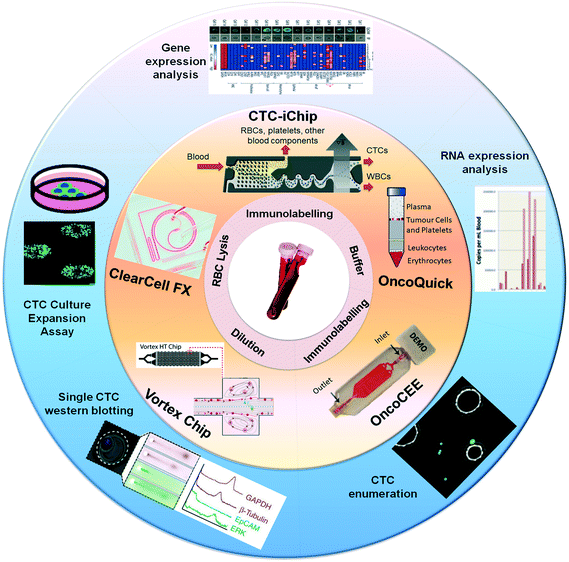 |
| Fig. 2 Technologies for CTC isolation, enumeration and profiling. Overview of commercially available technologies for CTC analysis including (a) Vortex chip, (b) ClearCell® FX, (c) CTC-iChip, (d) OncoQuick® and (e) OncoCEE™, from their initial prototype (orange section) through to current advancements that have resulted from these technologies (blue section). Images were adapted and reproduced with permission from (Vortex chip) ref. 82. Copyright (2014) Royal Society of Chemistry, (ClearCell® FX) ref. 22. Copyright (2013) Nature Publishing Group, (CTC-iChip) ref. 68 Copyright (2013) American Association for the Advancement of Science, (OncoQuick®) ref. 75 Copyright (2002) Wiley-Online library, (OncoCEE™) ref. 67 Copyright (2011) Hindawi Publishing Group. | |
ClearCell® FX.
ClearCell® FX employs a simple spiral microfluidics chip for CTC enrichment. The current version of the system requires red blood cell lysis as a pre-treatment step.22,85 In curvilinear spiral channels, an additional force component called “Dean drag force” (FD) acts on cells, with cells moving along “Dean vortices” migrate towards inner wall, strong inertial lift forces confine CTCs to inner wall while smaller blood cells continue to be entrained along dean vortex flow. This enables focusing CTCs and blood cells along distinct streams within the microchannel and are retrieved through two separate outlets. This chip is capable of concentrating rare cells with a relatively high throughput (7.5 mL whole blood in 45 min) and in an easy operating manner. From a manufacturing perspective the spiral chip involves no complex microstructures so the fabrication process is relatively smooth allowing it to be affordable. The study has also demonstrated several advances including integration for single CTC analysis. The output from the system, which is CTCs with WBCs (1 CTCs with ∼1000 nucleated blood cells), can be used for single cell molecular analysis. This group reported a single cell capturing system as an accessory for single CTC analysis.89 The device can be used to isolate single viable CTCs and gene expression study were performed on CTCs from lung cancer patients from which they pinpoint the level of T790M in EGFR mutant lung cancer. Besides, the same group also developed a CTC expansion assay based on microwells that can establish clusters of CTCs for downstream drug screening.90,91 This expansion assay revealed that the formation of CTC clusters inversely correlates with drug concentration in the medium, verifying the feasibility of the device as a CTC-based drug screen platform. Further, the technology shows great compatibility for downstream molecular analysis of the enriched CTCs.
OncoQuick®.
OncoQuick® integrates density-based and size-based filtration on one device. It is a proven tool in detecting CTCs, however, the sensitivity is relatively low compared to CellSearch® system.75 In clinical studies, researchers have been using this chip as a pre-enrichment step for downstream processing or analysis. It has been used in a clinical study on melanoma patients, in which they did qPCR on 4 melanocyte target RNAs (TYR, MLANA, MITF and MIF). They found that melanoma patients had higher expression of MLANA and MIF while healthy controls showed elevated expression in TYR and MITF expression.92 Another study investigated the relationship of metastatic breast cancer, bone marrow metastasis, systematic therapies and CTCs. They monitored CTC occurrence and number in patient's peripheral blood during systematic therapies. It was observed that CTCs tend to be non-proliferative and can survive after chemotherapy.93
ISET®.
ISET® uses size-based filtration for CTC isolation, and there are quite a few clinical studies using this chip as the processing steps are relatively simple.76,94 The CTCs are captured inside the porous membrane and can be immunostained for detection. One clinical study used ISET® to investigate CTCs as an early diagnostic marker for non-small cell lung cancer. This study investigated patients with chronic obstructive pulmonary disease which is considered of high risk for lung cancer. 5 out of 168 COPD patients studied tested positive for CTCs and developed lung nodules in 1 to 4 years after CTC detection with the follow up tumor biopsy confirming early stage lung cancer.95 The result has shown CTCs possess a great potential to be a biomarker for early diagnosis of cancer. Similarly, another clinical study compared ISET® with CellSearch® in more than 200 patients. They found these two technologies had similar sensitivities: 104/210 detected using ISET® and 82/210 detected using CellSearch®. It is noticeable that CTCs was detected in 62/210 patients using ISET® that was not detected by CellSearch®. Meanwhile, CTCs were detected in 40/210 patients using CellSearch® but not detected with ISET®. The CTCs that were detected both by ISET® and CellSearch® were in only 42/210 patients.94 This research indicates that size-based technology might be suitable for targeting different subtypes of CTCs in comparison to immunoaffinity based technology, which is something we need to pay attention while selecting techniques for liquid biopsy analysis. Missing a population of CTCs could mean a great loss of information that we could have harnessed from the cells. A limitation of size-based filtration technique is they usually cannot release the captured cells for downstream applications, which limits their application in clinics. Additionally, the flow of blood into the device cannot be high enough to avoid clotting and guarantee effective capture, which result in a relatively low overall throughput of the device.
Parsortix™ (ANGLE).
Parsortix™ device87,88 sorts cells by both deformability and size. The channels comprise a stair-like architecture that causes a gradual decrease in width until it reaches approximately <10 μm. These steps of varying sizes enable cell separation based on size, following which the direction of flow is reversed to harvest the lodged cells. The device consists of a looped cassette design that maximizes length of the separating steps whilst providing fluid paths with minimal resistance to flow. Xu et al.,96 utilized this technology to harvest both CK+ CTCs and CK−/vimentin+/CD45-cells. A proportion of vimentin+/CD45- circulating cells showed genetic alterations that were indicative of epithelial-to-mesenchymal transition. Furthermore, varied response to chemotherapy was observed in CK+ CTCs. This system is currently evaluated for its suitability in in a multicenter clinical study on CTC capture from metastatic breast cancer patients and use of multiple downstream analysis techniques (e.g., cytopathology, qPCR, FISH, and RNAseq) on the harvested CTCs.97
In addition to the above-mentioned approaches, the last few years has witnessed advancements in most of these technologies with several second or third generation platforms being developed to increase their suitability towards CTC analysis in clinical settings. Some of these key technologies developed in the last 3 to 4 years is summarized in Table 2.
Table 2 Recent CTC Technologies developed based on various antibody-dependent and independent approaches
Technology |
Principle |
Features |
Merit(s) |
Demerit(s) |
Comments |
Ref. |
Single CTC chip |
Pinch flow/inertia focusing |
Single CTCs from enriched blood samples |
Enable single cell analysis on CTCs |
Labor intensive |
This chip is an accessory for CTC enrichment chip |
98
|
NanoVelcro |
Coated nanostructure substrates |
CTCs with well-preserved RNA transcripts |
Recover intact CTCs for downstream analysis |
Throughput and specificity |
Currently this is the 4th generation chip |
99–102
|
CaTCh FISH |
Magnetic separation/fluorescence in situ hybridization |
Capture CTCs for in situ RNA analysis |
High throughput, high sensitivity |
Multi-step, specificity |
Provide a solution for CTC isolation and FISH |
103
|
Capiocyte |
Immunocapture |
Reliable monitoring of CTC count in treatment |
Specificity up to 38% |
Only demonstrated ability for cell counting |
Cell rolling is employed to enhance capture specificity |
104
|
Two-stage cluster chip |
Size and asymmetry based capturing |
99% CTC clusters recovery with 87% viability |
High recovery rate and simple principle |
Specificity in high cell background |
This is an improved version of the previous cluster chip |
105, 106 |
Nanofiber |
Thermo-responsive chitosan nanofiber substrate |
Capture and release CTCs through temperature-mediated transition |
Relative high purity in bio-interface methods |
Throughput and sensitivity |
There is no relevant clinical study yet |
107
|
Thermal sensitive graphene oxide |
Antibody conjugated GO-PEG in thermo-responsive polymer |
88.2% efficiency with 1 ml per hour |
Easy and scalable |
Throughput and sensitivity |
Validated capture performance with clinical samples |
108, 109 |
Acoustic separation |
Standing surface acoustic waves |
Throughput is 20 times higher than previous devices using the same principle |
High throughput and non-labelling |
High background blood cell |
Validated with breast cancer patient blood |
110
|
CTC culture chip |
Microwell for cell culture |
Derived spheroids from patient blood sample |
Can establish tumor-on-chip for clinical study |
Low yield |
Demonstrated drug testing with patient samples |
90, 111 |
Single CTC immunoblotting |
Vortex HT chip and single cell protein-PAGE |
Single CTC western blotting in microwell |
Analyze protein profile of single CTC |
Low efficiency |
First work doing immunoblotting on CTCs |
84
|
Magnetic ranking cytometer |
Magnetic sorting based on protein level |
Characterized phenotypic change of CTCs during chemotherapeutic in xenograft model |
Counting of cells of different phenotype |
Clinical validation needed |
First work attempting to monitor change of protein level during treatment |
112
|
3.2 Circulating tumor DNA (ctDNA)
ctDNA demonstrates a strong concordance with tumor biopsy profiles and provides real-time genetic information on the disease.113 With ctDNA being derived from multiple cancer sites, it provides an improved representation of the malignancy in comparison to a localized tumor biopsy. Further, the ease of access to ctDNA enables repeated and longitudinal profiling of the tumor, thereby providing a simple tool for monitoring tumor burden and response to treatment.114,115 The main challenges in the analysis of ctDNA include the minimal amount of accessible DNA per sample and dilution of ctDNA levels due to DNA from other nucleated cells. Coupled with their shorter read lengths, the wide variation in ctDNA levels from the same patient retrieved at different intervals require extremely sensitive and efficient isolation techniques. In this regard, typical methods for ctDNA analysis involve an initial extraction or isolation procedure followed by molecular analysis techniques to distinguish between cfDNA released from healthy cells and ctDNA released by the tumor. Despite being accessible through a number of bodily fluids, blood is predominantly used for ctDNA extraction owing to its proximity of the tumor. Further, there is very little evidence on the presence of ctDNA in other bodily fluids such as urine114 or saliva116 if the cancer occurred internally or at a distal location such as in lung or liver.
3.2.1 Isolation of ctDNA from plasma and whole blood.
Plasma based ctDNA extraction approaches have also garnered widespread interest owing to the absence of any blood cell contamination that can dilute the ctDNA levels. This is of particular importance as white blood cells117 begin to lyse within hours of blood being drawn from the patient and in turn dilute ctDNA level in the sample. As such, blood drawn from the patient needs to be centrifuged within 4–5 hours to prevent this contamination. Serum, on the other hand, is also a potentially useful bodily fluid for ctDNA extraction and can result in higher yields for analysis.118 However, the complexity of coagulation might result in the lysis of cells and would imply that ctDNA levels from tumor would be diluted. Further, extraction of ctDNA from plasma has been demonstrated to have a marginally higher sensitivity in detection as compared to serum.119
Of several methods used for ctDNA extraction from patients, one of the most popular techniques is the affinity based technique. In this method, DNA binding proteins coated to micro-pillars are woven to form a membrane and ctDNA from plasma was isolated under flow conditions. Similar membrane-affinity based techniques have extensively been utilised for ctDNA isolation. Fong et al., evaluated seven isolation approaches in parallel for the extraction of ctDNA from 12 pooled sera obtained from 67 colorectal cancer patients.120 Among several commercial protocols tested, QIAamp DNA blood kit was able to consistently retrieve a larger mass of ctDNA in comparison to rest of the approaches. Similar affinity based approaches have been demonstrated with the use of magnetic bead coated oligonucleotide probes (Fig. 3a) to selectively isolate ctDNA from human plasma.121 The accuracy of the DNA captured is also preserved as studies have shown that magnetism has a negligible effect on the methylation of DNA. Commercially available kits such as Dynabeads or GeneCatcher from Thermofisher have been developed based on similar principles. Recently, Dias et al., developed a portable microfluidic platform containing magnetoresistive sensors that offer higher sensitivity and increased portability in comparison to standard fluorescence techniques.122 Despite its greater autonomy compared to conventional methods, more studies on clinical samples are required to establish the applicability of this method with respect to throughput and reliability. Similarly, Guha et al.,123 adopted a novel magnetic probe based approach known as differential strand separation at critical temperature (DISSECT) wherein DNA is first attached to a streptavidin-coated magnetic bead that targets exon 8 of TP53. Whenever mutations occur in the exon, this results in a mismatch of bonding between the probe and the targeted region and as such, the melting temperature of the duplex is reduced. Subsequently when heat is applied, these mutations containing cfDNA or ctDNA will be preferentially discharged into the solution while those without the mutations remain unbounded. This facilitates distinction between ctDNA and cfDNA under an applied magnetic field. Patient sample analysis demonstrated higher sensitivity than conventional methods as this method can be further repeated to enrich the sample. Further, the approach was used to detect multiple mutations within a sample using a single denaturation temperature.
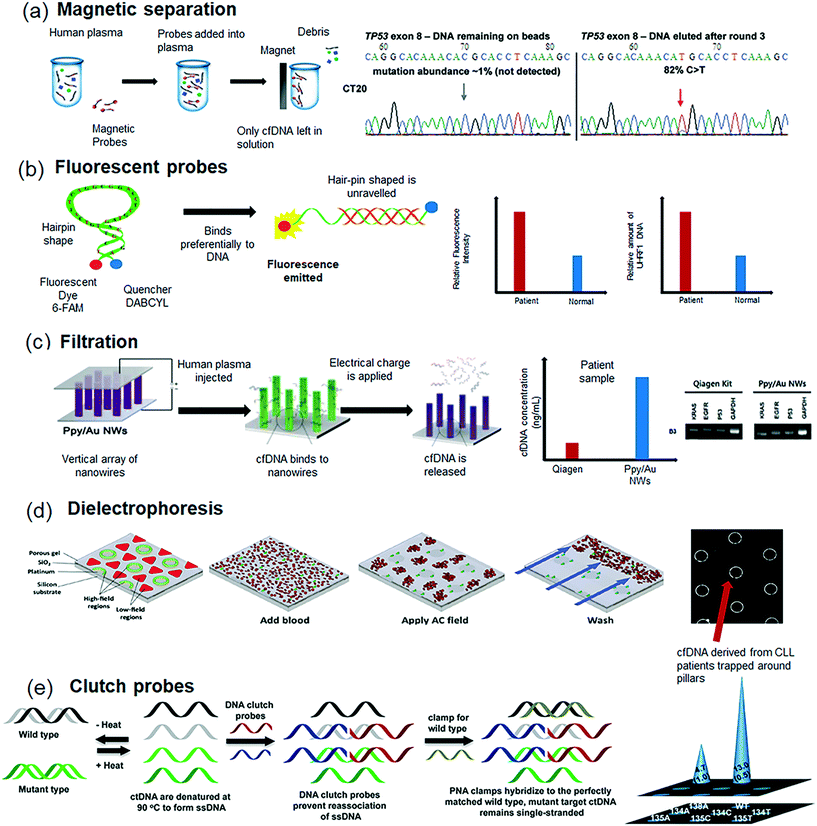 |
| Fig. 3 Methodologies for ctDNA isolation from plasma. (a) Overview of the magnetic capture hybridization technology for the isolation and analysis of cfDNA from plasma samples. (b) Molecular beacon based ctDNA analysis based on proximity based fluorescence emission, wherein close proximity of the hairpin ends quenches the fluorescence whilst unravelling the ends of the hairpin binds to a single stranded cfDNA thereby emitting a fluorescence signal. (c) Schematic representation of Ppy-coated Au nanowires (Ppy/Au NWs) for ctDNA analysis based on spontaneous redox behaviour of Ppy in response to the applied electric fields. (d) Dielectrophoretic trapping of ctDNA in a device containing micropillars, and (e) DNA clutch probe assay that render specific ctDNA sequences accessible to identify desired mutations. Images were adapted and reproduced with permission from (a) ref. 121 (b) ref. 124 Copyright (2010, 2016) Elsevier Publishing Group, (c) ref. 126 Copyright (2016) Ivyspring Publishing Group, (d) ref. 127 Copyright (2014) the American Association for Clinical Chemistry and (e) ref. 128 Copyright (2016) American Chemical Society. | |
Similar affinity based fluorescence probes such as molecular beacons124 or fluorescent gold nanoclusters125 have been used to isolate cfDNA. While traditional molecular beacon assay works based on proximity based emission wherein at close proximity and at the ends of the hairpin, few photons are effectively emitted and the probe does not emit fluorescence (Fig. 3b). However, when the ends of the hairpin unravel as the ring binds to a single stranded cfDNA in the sample, the distance between the fluorophore and quencher increases thereby emitting a fluorescence signal. To this end, gold nanoclusters work on the principle of electrostatic attraction wherein nanoparticles do not emit fluorescence due to quenching when the electrostatic forces of attraction hold them closely together. However, when cfDNA is introduced, the bonding on the gold nanoparticle is disrupted when it embeds itself in the negatively charged double helix cfDNA and emits a fluorescence signal. Recently, Gui et al. demonstrated the use of molecular beacons for the in situ monitoring of cfDNA levels based on the quantification of fluorescence intensity.124 The method is rapid and was shown to work well even in serum for the detection of the UHRF1 mutation in breast cancer patients. Jeon et al., demonstrated the use of vertical arrays of polymer coated Au nanowires as physical traps to isolate cfDNA.126 Upon the application of an electrical potential, the polymer film started to breakdown and shrink, thereby releasing the cfDNA that was trapped between the nanowires (Fig. 3c). The device was reported to successfully extract DNA at a concentration of 10 pg mL−1 with an efficiency of 50%. Despite plasma based methods demonstrating acceptable isolation efficiency, the need for pre-processing blood might still impact the recovery of cfDNA. In this regard, Sonnenberg et al., demonstrated the retrieval of ctDNA directly from whole blood (Fig. 3d) using an AC electrokinetic device containing circular microarray electrodes.127 At the optimized AC frequency and voltage, cfDNA, which is more polarizable than the surrounding media, experience positive DEP (p-DEP) that concentrates DNA into the DEP high-field regions over the circular microelectrode. On the other hand, less polarizable blood cells experience negative DEP (n-DEP), causing them to move into the DEP low-field regions between the microelectrodes. The study demonstrated rapid isolation of ctDNA from patients with chronic lymphocytic leukemia (CLL) and the performance was comparable with commercial Qiagen protocol. However, it must be mentioned that there is a physical limit to the size of particles that can be separated. As the volume of the particle decreases, forces that were previously negligible such as diffusion and convection now become dominant. Recently, Das et al.,128 developed a novel approach for the detection of mutated ctDNA using a DNA clutch probe (DCPs) based approach (Fig. 3e). The use of DCPs rendered only specific ctDNA sequences accessible for hybridization with the target probe whilst simultaneously deactivating any closely related sequences. The presence of mutated DNA was detected using a three-dimensional electrochemical sensor fabricated using electrodeposited gold. The sensor demonstrated highly sensitive detection of mutated ctDNA to as low as 1 fg μL−1 of target mutations from melanoma and lung cancer patients.
While these methods for ctDNA extraction have demonstrated their potential for disease diagnosis, prognosis and patient monitoring, the main challenge still remains with regards to variations in the amount of cfDNA extracted from patient to patient. In an experiment conducted by Fleischhacker et al., to demonstrate how severe this deviation may be, even cfDNA extracted from the same plasma using identical extraction kit and protocols but conducted in different labs had produced very different readings.129 Nevertheless over the years, efforts have gone into standardising protocols, the results still remain different.
3.2.2 Analysis of ctDNA.
It is important that techniques used for cfDNA isolation be able to distinguish between ctDNA and cfDNA derived from tumor and other cell types respectively. While an in-depth discussion of the methods available for the analysis of ctDNA is beyond the scope of this review, this section aims to provide the reader with a brief overview of the methods. Table 3 summarises some of the key ctDNA detection approaches and their potential application in clinical studies performed using them. Methods to distinguish between ctDNA from cfDNA utilises the advances that have been made in identifying gene mutations which have been demonstrated to have an association with cancer. Examples of these mutations include the nucleotide mutations in cordon 12 and cordon 13 in the Kirsten rat sarcoma viral oncogene homologue (KRAS) gene,130 which has been demonstrated to be present in nearly a quarter of all cancers and EGFR mutations which were shown to be associated with the overactive cell growth and division mechanism that is present in many cancers including lung cancer.131,132 In addition to seeking out point mutations, the frequency of mutations and chromosomal rearrangements can also be used as a method to distinguish ctDNA from cfDNA.
Table 3 Summary of ctDNA detection approaches
Principle |
Method |
Merit(s) |
Demerit(s) |
Clinical studies |
Ref. |
PCR |
Droplet based PCR |
High sensitivity, high throughput |
Small region of interrogation implies that point mutations must be known |
Colorectal cancer |
134, 135 |
Digital PCR |
High sensitivity, high throughput |
Non-small cell lung cancer, breast cancer |
136, 137 |
BEAMing |
High sensitivity, cost effective |
Colorectal cancer |
138, 139 |
Mass spectrometry |
Ease of use |
Late stage advanced solid tumors |
140
|
ARMS-PCR |
Commercially available (with regulatory approval), covers variants |
Breast cancer |
141
|
Bi-PAP amplification |
Rapid detection of ultra-rare mutation |
NA |
142
|
Gene sequencing |
TAm-Seq |
High sensitivity, cost effective, high throughput |
Higher read depth or fidelity required for enhanced mutation detection |
Ovarian cancer |
143
|
Safe-SeqS |
Automatic querying of multiple bases sequentially |
NA |
144
|
Ion-AmpliSeq |
50-Cancer gene panel allows for rapid screening (7 days) of cancer genes in cfDNA |
Breast cancer |
145
|
CAPP-Seq |
Eliminate patient-specific optimization, coverage of nearly all patients |
Non-small cell lung cancer |
146
|
Digital karyotyping |
Perform relatively well and quickly without the need for whole genome sequencing |
Childhood neuroblastoma |
147
|
OnTarget |
Able to select for sequence content |
NA |
148
|
Whole genome sequencing |
Digital karyotyping |
Extensive application |
Slow, expensive |
Breast cancer, ovarian cancer |
149, 150 |
PARE |
Robust, sensitive, extensive application |
Leukaemia, lymphoma, breast cancer, colorectal cancer |
151–153
|
Plasma-Seq |
Robust, sensitive |
Prostate cancer |
154
|
Analysis of point mutations can be achieved using traditional polymerase chain reaction (PCR) that amplifies the retrieved DNA and subsequent sequence data analysis obtained from the patient sample is then compared with a genomic library. While the addition of various forms of PCR such as the digital PCR and qPCR has made this method relatively easier to implement with higher sensitivities, PCR methods are still qualitative since one will not be able to obtain precise measurements of levels of ctDNA present.133 More recently, a number of digital genomic methods have been developed to quantify mutations by analyzing individual template molecules. This is achieved by running PCR on serially diluting DNA down to single or fewer templates per reaction. Quantification is done based on the number of reactions containing wild-type or mutant PCR product.
In an effort to quantify the levels of ctDNA present, a technique combining flow cytometry and PCR, also known as BEAMing (beads, emulsions, amplification and magnetics) has been developed.155 This sensitive and cost-effective approach facilitates single-molecule PCR reactions to be performed on magnetic beads in water-in-oil emulsions. Distinction between mutant and wild-type coated beads is achieved using allele-specific fluorescence probes complementary to the known wild-type or mutant sequences. With a high signal-to-noise ratio obtained by hybridization or enzymatic assays, beads were analyzed rapidly using flow cytometry.
One of the inherent limitations of the above mentioned method is that the precision in identifying point mutations in the DNA will limit the size of the gene interrogation region. Consequently, it will be unable to provide information for cancers if the clinicians have no prior indication of the location and the type of cancer since they will not know the mutations to look out for. As different cancers may have different point mutations, the highly specific nature of looking for point mutations also work against it since different mutations will have to be sought for different types of cancer. In order to build a more generalised technique and build a more comprehensive picture of the mutations, given the heterogeneity of cancer, a technique analysing a larger gene interrogation region was developed – the analysis of the whole genome. Two genome-wide analysis methods to identify alterations in ctDNA include personalized analysis of rearranged ends (PARE)156 and digital karyotyping.157 PARE utilizes genome rearrangements associated with tumors to identify and detect biomarkers in circulation. Alternatively, digital karyotyping is used to analyze copy-number alterations and any set of sequences that can detect uncharacterized chromosomal changes and exogenous sequences in cancer. Recently, Newman and his team performed whole genome analysis by looking for a common set of genomic mutations or panel across lung cancers.32 By amplifying only these regions of interest where mutations are inclined to occur, this method is a combination of both breadth and depth. Breadth here refers to sampling a larger region compared to PCR based approaches and depth indicates interrogation of known regions of interest. Despite primarily being a statistical method, the panel detected ctDNA in 100% of patients with stage II–IV non-small cell lung cancer (NSCLC). However, as the results are only based on 17 patients, clinical trials conducted with a larger cohort of patients will have to be conducted to establish credibility of this approach.
3.3 Extracellular vesicles (EVs)
Exosome research is a rapidly growing field both in biology and medicine. With several studies exploring the clinical utility and diagnostic potential of EVs, there is a growing need for better isolation and characterization techniques. Although several methods have been developed to isolate EVs from bodily fluids, only a few of these can clearly discriminate between EVs and other cellular material lipid-like structures or retrovirus particles, similar in terms of size and density. Several commonly used methods to isolate EVs from plasma or other sample include ultracentrifugation (UC), filtration, polymer-based precipitation, size exclusion chromatography (SEC) and magnetic associated cell sorting (MACS). Evaluation and comparison of these common exosome isolation methods have been performed by some researchers.158 For instance, Tauro et al.159 employed three different methods including UC, density-gradient centrifuge (DG) and EpCAM immunoaffinity capture (IAC), to isolate exosome from cell culture medium. It was observed that IAC isolated EVs demonstrated significantly higher level of quantitative protein spectrum in comparison to UC and DG isolated EVs. However, these results might be limited to specific sample used for analysis. Similarly, Kalra et al.,160 tested various exosome isolation methods using human blood plasma. The results indicated that DG outperformed UC and IAC, which was contradictory to results obtained with cancer cell culture medium. These isolation methodologies and their impact on downstream analysis of EVs are reviewed in detail by Taylor et al.161 To sum up, the performance of common exosome isolation methods significantly vary due to contamination, poor yield, intensive labor or requirements on equipment being some of the major factors influencing the yield.
The integration of microfluidics and various sensing components or sensors has significantly improved the accuracy of exosome isolation from biological samples. Several notable approaches improved the feasibility of immuno-affinity based isolation162–164 through incorporation of functionalized nanomaterials or nano-sized membrane that can specifically select for EVs from biological fluids. Similarly, the use of periodic plasmonic nanoholes similar to vesicle size (30–200 nm) or electric field induced isolation techniques has improved sensitivity.165 These approaches are rapid and efficient in comparison to standard techniques notably, ultracentrifugation, sedimentation or density-gradient separation. However, there is a need to optimize these microfluidic platforms to meet the demands of clinical settings. In this regard, this section will highlight some recent exosome isolation and detection platforms that demonstrate potential for integration with standard bio-analytical systems for molecular profiling and quantification of EVs.
3.3.1 Microfluidic isolation of EVs.
A very recent and representative work that can separate EVs from other components such as MVs based on size is a viscoelastic flow device (Fig. 4a) developed by Liu et al.166 The addition of poly(oxyethylene) (PEO) to the exosome sample would influence the particle position within the channel due to elastic lift force, inertial lift force and viscous drag force acting on the vesicles. Using this approach, vesicles with diameter smaller than 200 nm were separated from MVs with diameter larger than 200 nm from fetal bovine serum. However, the compatibility of PEO with every biological sample and its effect on the downstream proteomics or gene analysis remain unknown. Similarly, Liang et al.,167 demonstrated a size based separation approach where 200 nm and 30 nm filter membranes were assembled in the sample flow path within a PMMA channel. The chip was demonstrated to be able to recover exosome from cell culture medium and urine sample. Isolation of EVs using urine samples obtained from both bladder cancer patients and healthy controls suggested the possibility of EVs concentration to be a potential biomarker. Despite the design and operation of the filtration device being simple, a common problem in filtration system is clogging especially when the pore size is as small as 200 nm and 30 nm. Deterministic lateral displacement168 was also adapted for exosome isolation by Wunsch et al. The gap size between pillars was designed to range between 25 to 235 nm to separate particles of size between 20 to 110 nm with high resolution. Although the manipulation of particle motion was precise, the throughput was slow and fabrication process was complicated due to the smaller separations. Yeo et al.,169 demonstrated a simple approach for the rapid separation of EVs using centrifugal microfluidics known as microfluidic CEntrifugal nanoparticles separation and extraction (μCENSE) platform. This platform comprises a simple centrifugal chip containing a serpentine channel with single inlet and two bifurcating outlets. This chip is mounted onto a tabletop centrifuge and when subjected to centrifugal forces, this chip enables nanovesicle separation based on size along the short curved microchannel. With centrifugal force requirement being several folds lower than conventional methods, the nanovesicles migrate within the microchannel to form distinct streams of different sized vesicles. The smaller nanovesicles and exosomes can then be extracted. Lee et al.,170 explored the possibility of using acoustic forces to separate EVs from MVs on chip. Under the applied field, a cutoff diameter of 300 nm was set so that MVs were deviated whilst EVs remained unperturbed during flow through the microchannel.
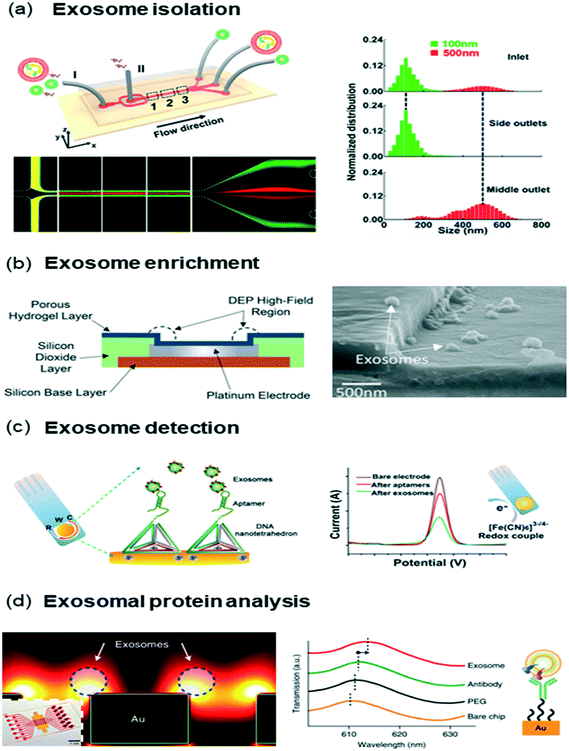 |
| Fig. 4 Exosome isolation and analysis (a) size-based exosome isolation chip that utilized viscoelastic force to push MVs into sheath flow while exosomes stayed at sample flow. (b) DEP based enrichment of exosomes, (c) electrochemical exosome detection using a DNA tetrahedron-aptamer capture probe. The aptamer orientation enhanced capture performance via improved sensor–target interactions. (d) Exosomal protein analysis chip based on nanoplasmonic sensing. Each antibody functionalized nano-plasmonic nanohole enabled protein sensing on the isolated exosomes. Images were adapted and reproduced with permission from (a) ref. 166. (b) Ref. 176 (c) ref. 177 Copyright (2017, 2016, 2017) American Chemical Society, (c) ref. 165 Copyright (2013) Nature Publishing Group. | |
Immunoaffinity approaches with the use of vesicular or tumor specific markers are also being viewed upon as sustainable technologies for exosome isolation. The goal of immuno-capture on-chip is usually to achieve higher sensitivity compared to MACS and subsequent parallel profiling of exosomes using multiple markers. Chen et al.,171 utilized a chip containing wide but shallow channel with herringbone groves features functionalized with anti-CD63 or anti-CD4 for exosome isolation. The device was able to capture exosome from sera, and captured EVs were retrieved for downstream RNA analysis. Operation of this device was relatively easier than MACS. Herringbone structure mediated fluid mixing has previously been demonstrated for improved target–antibody interaction. Similarly, Kanwar et al.,172 developed an integrated microfluidic chip with an array of circular chambers functionalized with anti-CD63 for the isolation and detection of EVs from serum samples. Captured EVs were stained using carbocyanine dye and measured using standard analytical tools such as a plate reader. The major advantage of using this device is the convenience of on-chip signal measurement, providing the potential of clinical diagnosis. He et al.,173 developed an alternative integrated platform for immunomagnetic isolation, exosome lysis and on-chip protein analysis. The approach also demonstrated phenotyping of exosome subpopulations by targeting a panel of common exosomal and tumor-specific markers.
Electric fields have also been utilized to modulate fluid flow and molecules around on surfaces that can significantly improve exosome isolation by accentuating capture performance. Ibsen et al.,174 developed a DEP-based exosome isolation device ExoVertia™ (Biological Dynamics, San Diego, CA, USA) to rapidly isolate and recover glioblastoma EVs from human plasma samples. Requiring minimal sample volumes, the microelectrodes were able to concentrate EVs into high-field regions around the microelectrodes within 15 min. However, the device is not characterized to precisely distinguish between EVs, MVs and soluble proteins in sample. Additionally, the electric field parameters such as permittivity of exosome and conductivity of media are not discussed, which is important in determining the electric field condition. Similarly, Vaidyanathan et al.,175 utilized alternating current electrohydrodynamics based fluid flow phenomenon that significantly improved sensor–target interactions. This fluid flow is characteristic of fluid mixing enabled by forces generated within nanometers from the surface. Tumor-specific exosome capture was demonstrated using EVs derived from cell lines and patient serum samples whilst being detected using a colorimetric approach. Despite being highly sensitive, this approach is limited to isolating EVs based on specific capture agents and cannot retrieve isolated EVs for downstream analysis.
3.3.2 On-chip detection and analysis of EVs.
The basic idea of on-chip detection is to harbor EVs onto any microdevice in a way that can produce a detectable signal using several read-out approaches. Doldán et al.,176 designed an electrochemical chip (Fig. 4b) that was able to sense the level of exosome in two different strategies called signal-off and signal-on. In signal-off mode, electrode was functionalized with mouse α-CD9 antibodies to capture CD9 positive EVs. Following this, horseradish peroxidase (HRP)-conjugated anti-IgG antibodies was added and attached to the unbound mouse α-CD9 antibodies. Finally, 3,3′,5,5′-tetramethyl benzidine (TMB)/H2O2 was introduced and electrochemical signal as a result of TMB reduction was recorded. In signal-on mode, the principle was similar, but adjustments in the use of antibodies lead to a positive correlation between exosome concentration and current signal intensity. The limit of detection (LOD) of this electrochemical sensor was 200 EVs per μL. More recently, Wang et al.,177 reported an aptamer-based exosome sensor to specifically capture target EVs. To address the general problems with aptamer functionalization of self-assembly aggregation and random orientation, DNA-nanotetrahedron was designed (Fig. 4c) to ensure stable and oriented layer of aptamer on the surface of electrode, which significantly enhanced the sensitivity of the sensor. The LOD of this sensor was tested to be 2.09 × 104 EVs per mL. Ko et al.,178 developed a phone compatible exosome detection method. In their design, exosome sample was driven through a channel by gravity. Near the inlet, anti-CD45 and anti-CD61 coated large microbeads were introduced to bind unwanted EVs from leukocytes and platelets, and anti-CD81 coated small microbeads were used to attach remaining target EVs. A filtration was set along the channel to block large beads and allow small beads to go through. A phone compatible holder was designed to guide the filtered phone flash light toward the outlet region, and the ELISA lights were filtered and received by the phone camera so that a fluorescence photo could be taken. The system was applied to detect the exosome level of cortical neurons with and without injury, and proved to successfully reflect the different status.
Similarly, Olcum et al.,179 exploited the use of suspended nanochannel resonators (SNRs) system to achieve extreme nanoparticle mass sensitivity in solution. In the SNR system, a suspended microcantilever oscillated under the control of a piezoelectric actuator, with the vibration being read out using a laser component. Feedback between laser photodetector and piezoelectric actuator was built up so that the oscillation of microcantilever was always at resonant frequency. When a nanoparticle approaches the cantilever, the resonant frequency of cantilever would be shifted to an extent decided by the mass of the nanoparticle. The SNR system was used to validate exosome sample from 3T3-J2 fibroblasts or primary hepatocytes culture medium. Mass/size distribution of fibroblast exosome was found to be broader than hepatocyte EVs.
EVs and MVs have been reported to contain important proteins and genetic molecules. The level of some exosomal content is usually related to potential of biomarker. Therefore, proteomics and gene sequencing or detection is sometimes performed with exosome sample. Besides the conventional techniques such as western blotting, mass spectrometry, PCR and microarray, more portable techniques for protein and RNA analysis are highly expected to meet the needs of clinical diagnosis of cancer and other diseases. Most of the protein analyses on chip utilize immunoaffinity binding to evaluate the level of interested proteins. Im et al.,165 produced a surface plasmon resonance (SPR) based exosome protein profiling device, called nPLEX platform (Fig. 4d). Plasmonic nanoholes were patterned in gold film on glass and functionalized with affinity ligands. The size of nanoholes was 200 nm in diameter so that enhanced electromagnetic field region was comparable to exosome in size, resulting in a higher sensitivity for exosome detection. By principle, when target EVs bind to the ligand, spectral shift or intensity changes would be observed, enabling protein level estimation. This chip successfully identified higher level of EpCAM and CD24 in ovarian cancer ascites without pre-treatment such as isolation or enrichment of exosomes. Yoshioka et al.,180 developed a rapid and high-throughput exosome surface protein analysis system with the help of photosensitizer-beads. Donor beads were coated with antibody using biotin–streptavidin chemistry. Acceptor beads were conjugated to a second antibody. When the donor beads were excited by 680 nm laser, singlet oxygen would be released and the acceptor beads, which were within 200 nm distance of the donor beads, would be excited to emit 615 nm light. This protocol could be operated in a 96-well plate to achieve a high-throughput analysis. It was validated to be able to indicate the level of CD147/CD9 double positive EVs from different cell lines. Similarly, Jeong et al., employed an electrochemical method to monitor the level of exosome surface proteins. EVs were immunomagnetically isolated and subsequently, magnetic beads aggregated captured exosomes onto the electrode under magnetic field. Loading of TMB would then induce current of intensity proportional to the level of HRP, which indicates the level of target proteins. The system was packaged in a handhold box with eight parallel analysis channels. Clinical feasibility of this device was demonstrated with ovarian cancer patient plasma showing a higher level of CD24. On the other hand, Shao et al.,181 reported an integrated microfluidic platform for immuno-magnetic capture of exosomes for RNA analysis. EVs were captured on-chip followed by lysis to release the exosomal RNA. Released RNA was attracted by electrostatic force onto glass-beads. Post extraction, RNA was reverse-transcribed to prepare for next real-time PCR on chip. When the amplification was completed, chamber fluorescence was measured as RNA level indicator. The device was applied in glioblastoma treatment efficacy monitoring with the profiling of selected exosomal mRNA. In addition to the above-mentioned approaches, several platforms have been developed in the last 3–4 years focusing on improved isolation and detection of exosomes in terms of sensitivity, portability and ease of analysis. Some of these key technologies are listed in Table 4. Despite these successful demonstrations on exosome isolation, more detailed analyses of EVs and their biological significance is warranted to further understand their potential for disease diagnosis.
Table 4 EV isolation and detection technologies
Technology |
Principle |
Performance |
Merit(s) |
Demerit(s) |
Ref. |
Viscoelastic flow chip |
Size-dependent; viscoelasticity of vesicles |
Separation efficiency- ∼80% |
Label-free continuous separation |
Polymer residue might be present in retrieved EV samples |
166
|
Double-filtration chip |
Size-dependent filtration |
Separation efficiency- ∼81.3% |
High purity |
May be prone to clogging; fabrication of 30 nm pore membrane may be challenging |
167
|
Exosome total analysis chip (ExoTIC) |
Size-dependent; membrane based filtration |
∼4–1000 fold higher yield than ultracentrifugation |
High purity and low-cost assembly |
Fabrication of uniform multiple nano-sized pores may be challenging |
182
|
Exodisc |
Size-dependent filtration using centrifugation |
Separation efficiency- ∼95% |
High purity and dual-membrane filtration |
May be prone to clogging; uniform membrane pore fabrication might be difficult |
183
|
CEntrifugal nanoparticles separation and extraction (μCENSE) platform |
Centrifugal microfluidics |
Separation efficiency- ∼90% |
High purity and rapid isolation |
Can handle only small sample volume (10 μL) |
169
|
Acoustic nanofilter chip |
Acoustophoresis; size-selective separation |
Separation efficiency- ∼90% |
High efficiency and continuous operation |
Loss of EV population due to different sizes |
170
|
EXO-electrochemical biosensor |
Immuno-affinity based capture; electrochemical detection |
Sensitivity: 2 × 105 mL−1 |
High sensitivity |
Loss of EVs due to single antibody based capture |
176
|
Aptasensor |
DNA tetrahedron and aptamer based capture |
Sensitivity: 2.09 × 104 mL−1 |
Highly sensitive and portable sensor |
Aptamer selectivity and complex sensor fabrication |
177
|
Magneto-electrochemical sensor |
Magnetic enrichment and enzymatic amplification |
Sensitivity: 3 × 104 mL−1 |
Multiplexed and portable sensor |
Loss of EVs due to single antibody based capture |
184
|
AC-EHD chip |
Electrohydrodynamic fluid flow |
Sensitivity: 2.76 × 106 mL−1 |
Multiplexed on-chip capture and analysis |
Cannot retrieve EVs for downstream analysis |
175
|
Mobile exosome detector (μMED) |
Microbead-based immunocapture |
Sensitivity: 1 × 107 mL−1 |
Simple operation |
Limited sensitivity and accuracy |
178
|
ExoScreen |
Photosensitizer-bead based capture and detection |
Sensitivity: 15.6 ng of purified EVs |
Good sensitivity |
Accuracy of fluorescence may be moderate |
180
|
Immuno-magnetic exosomal RNA (iMER) analysis chip |
Immuno-magnetic selection |
Capture efficiency- ∼93% |
High efficiency and integrated exosomal RNA analysis |
Enrichment is dependent on surface marker |
181
|
4. Conclusions and future directions
With strategies in cancer management continuing to evolve, the challenges and demands associated with diagnosis, prognosis, and prediction of treatment resistance continue to grow in tandem. However, tumor heterogeneity remains a major roadblock in devising therapy regimens based on individual genome analysis despite the availability of molecular agents to target specific genomic changes. In this regard, CTCs and ctDNA form the core of liquid biopsy diagnostics and paved way to new avenues in cancer therapy and management. Liquid biopsy could serve as effective alternatives in cases where primary tumors are difficult to biopsy. Moreover, liquid biopsy could help stratify patients and focus screening modalities to the population with higher risk, thereby reducing side effects (e.g., radiation in mammography) and health care costs. However, it might be more relevant to utilize CTCs and ctDNA during systemic therapy rather than as early diagnosis markers where the impediments are sensitivity and selectivity. In this regard, analyses of ctDNA and CTCs response to targeted therapies might increase feasibility towards clinical practice. Additional directions can include tests for mutations in genes encoding therapeutic targets and/or the corresponding resistant genes that can be tested easily with a small cohort of patients to begin with. Earlier studies observed inconsistencies in the detection of ctDNA mutations and can be improved by approaches that can identify rare mutations with high sensitivity in matched ctDNA and tumor tissue samples.
These critical concerns mentioned above have caused a paradigm shift in liquid biopsy research with EVs or ctDNA being preferred markers over CTCs. However, eventual clinical utility of all these markers still needs more validation. Although EVs and ctDNAs are more abundant and seem more convenient to analyze than CTCs, they are also technical challenges and concerns. It is important to note that the field of EVs and their potential for liquid biopsy is still in its budding stages whilst requiring further investigations to gain further insights. However, inherent characteristics of EVs certainly make them ideal biomarkers for research, disease diagnosis and therapy. While significant advances have been made in terms of EVs isolation and detection strategies, it is imperative to address key challenges related to isolation of sub-population of EVs or disease-specific nanovesicles under a single platform to facilitate clinical integration of EV technologies. Furthermore, greater clinical validation may be required with extensive analysis of disease specific EVs to understand their clinical potential and role in disease progression. With regards to realizing the tumor-modulating potential of EVs, it is important to understand the role of EVs in oncogenic exosome signaling.185 A possible approach would be to test models devoid of such nanovesicles that might prevent metastasis and tumorigenesis. However, implementation of this approach is limited by the associated technical challenges involved in removing EVs.
In addition to these, several challenges in the field however need to be addressed before realizing the full potential of liquid biopsies as a true diagnostics for disease management. In particular, deeper insights into the biology behind EVs, ctDNA and CTCs can establish correlation between molecular profiles and physiological disease state of the patient. While several cases have indicated a mutual correlation of CTC numbers and ctDNA levels, the fundamental biology associated with this correlation is still poorly understood.186 Several other challenges associated with the implementation of liquid biopsy in clinical settings include (i) the potential of ctDNA to be considered a full representation of the cancer stage, and (ii) considering ctDNA, CTCs, and EVs are widely known circulating biomarkers, it is intriguing to know if all metastases contribute to the release of these biomarkers in the bloodstream. In addition to these challenges, the field has also witnessed the rise of several new DNA and CTC based approaches as liquid biopsy diagnostics. This includes plasma DNA tissue mapping that involves a genome-wide bisulfite sequencing of plasma DNA and methylation deconvolution of the sequencing data to trace the tissue of origin of plasma DNA. Since plasma DNA is nucleosome-protected, nucleosome mapping that involves the analysis of the genomic sequencing coverage of plasma DNA fragments can reveal the position of nucleosomes. This can easily help distinguish the high and low expressed genes due to the shorter read depth in the nucleosome depleted region. Also, the development of CTC culture has shown promising prospects.111,187 Patient derived CTCs grown in culture and injected into mice confirmed that characteristics of the original tumor were maintained.188 Furthermore, CTC derived cell lines are believed to support therapy selections and potentially aid drug testing or development.
From this review, we can conclude that each of these three liquid biopsy biomarkers has its advantages as well as limitations. Moving forward, effective cancer diagnosis and management may move towards simultaneous analysis of all these liquid biopsy biomarkers in tandem and from a single patient sample, rather than just relying on single biomarker based analysis which is proving to be inconclusive due to heterogeneity and variation in biomarker levels within and among different individuals.
Conflicts of interest
C. T. L. is the co-founder of Clearbridge Biomedics Pte Ltd, which is commercializing the ClearCell® FX spiral biochips for CTC enrichment.
Acknowledgements
P. Z. acknowledges scholarship and support from NUS Graduate School for Integrative Sciences and Engineering (NGS). K. J. acknowledges scholarship and support from the Mechanobiology Institute (MBI) at the National University of Singapore (NUS). Support provided by the National Research Foundation, Prime Minister's Office, Singapore under its Research Centre of Excellence, MBI at NUS is also acknowledged.
References
- S. A. Eccles and D. R. Welch, Lancet, 2007, 369, 1742–1757 CrossRef CAS.
- D. A. Haber and V. E. Velculescu, Cancer Discovery, 2014, 4, 650–661 CrossRef CAS PubMed.
- S. Mukhopadhyay, Mod. Pathol., 2012, 25, 1054 CrossRef.
- G. V. Scagliotti, P. Parikh, J. v. Pawel, B. Biesma, J. Vansteenkiste, C. Manegold, P. Serwatowski, U. Gatzemeier, R. Digumarti, M. Zukin, J. S. Lee, A. Mellemgaard, K. Park, S. Patil, J. Rolski, T. Goksel, F. d. Marinis, L. Simms, K. P. Sugarman and D. Gandara, J. Clin. Oncol., 2008, 26, 3543–3551 CrossRef CAS PubMed.
- K. Pantel and C. Alix-Panabières, Trends Mol. Med., 2010, 16, 398–406 CrossRef PubMed.
- L. B. Rorke, Cancer, 1997, 79, 665–667 CrossRef CAS PubMed.
- F. Riva, O. I. Dronov, D. I. Khomenko, F. Huguet, C. Louvet, P. Mariani, M.-H. Stern, O. Lantz, C. Proudhon, J.-Y. Pierga and F.-C. Bidard, Mol. Oncol., 2016, 10, 481–493 CrossRef CAS PubMed.
- M. Cristofanilli, G. T. Budd, M. J. Ellis, A. Stopeck, J. Matera, M. C. Miller, J. M. Reuben, G. V. Doyle, W. J. Allard, L. W. M. M. Terstappen and D. F. Hayes, N. Engl. J. Med., 2004, 351, 781–791 CrossRef CAS PubMed.
- S. J. Dawson, N. Rosenfeld and C. Caldas, N. Engl. J. Med., 2013, 369, 93–94 CrossRef CAS PubMed.
- M. Yu, S. Stott, M. Toner, S. Maheswaran and D. A. Haber, J. Cell Biol., 2011, 192, 373–382 CrossRef CAS PubMed.
- C. Alix-Panabières and K. Pantel, Clin. Chem., 2013, 59, 110–118 CrossRef PubMed.
-
J. C. Récamier, Recherches sur le traitement du cancer sur la compression methodique simple ou combinee et sur l'histoire generale de la meme maladie, Gabon, Paris, 2nd edn, 1829 Search PubMed.
- T. R. Ashworth, Med. J. Aust., 1869, 14, 146–149 Search PubMed.
- I. Salgado, J. F. Hopkirk, R. C. Long, A. C. Ritchie, S. Ritchie and D. R. Webster, Can. Med. Assoc. J., 1959, 81, 619–622 CAS.
- R. F. Alexander and A. I. Spriggs, J. Clin. Pathol., 1960, 13, 414–424 CrossRef CAS PubMed.
- E. Racila, D. Euhus, A. J. Weiss, C. Rao, J. McConnell, L. W. M. M. Terstappen and J. W. Uhr, Proc. Natl. Acad. Sci. U. S. A., 1998, 95, 4589–4594 CrossRef CAS.
- W. J. Allard, J. Matera, M. C. Miller, M. Repollet, M. C. Connelly, C. Rao, A. G. J. Tibbe, J. W. Uhr and L. W. M. M. Terstappen, Clin. Cancer Res., 2004, 10, 6897–6904 CrossRef PubMed.
- S. Nagrath, L. V. Sequist, S. Maheswaran, D. W. Bell, D. Irimia, L. Ulkus, M. R. Smith, E. L. Kwak, S. Digumarthy, A. Muzikansky, P. Ryan, U. J. Balis, R. G. Tompkins, D. A. Haber and M. Toner, Nature, 2007, 450, 1235–1239 CrossRef CAS PubMed.
- D. C. Danila, G. Heller, G. A. Gignac, R. Gonzalez-Espinoza, A. Anand, E. Tanaka, H. Lilja, L. Schwartz, S. Larson, M. Fleisher and H. I. Scher, Clin. Cancer Res., 2007, 13, 7053–7058 CrossRef CAS PubMed.
- M. Cristofanilli, D. F. Hayes, G. T. Budd, M. J. Ellis, A. Stopeck, J. M. Reuben, G. V. Doyle, J. Matera, W. J. Allard, M. C. Miller, H. A. Fritsche, G. N. Hortobagyi and L. W. M. M. Terstappen, J. Clin. Oncol., 2005, 23, 1420–1430 CrossRef PubMed.
- N. J. Nelson, J. Natl. Cancer Inst., 2010, 102, 146–148 CrossRef PubMed.
- H. W. Hou, M. E. Warkiani, B. L. Khoo, Z. R. Li, R. A. Soo, D. S. Tan, W. T. Lim, J. Han, A. A. Bhagat and C. T. Lim, Sci. Rep., 2013, 3, 1259 CrossRef PubMed.
- M. Dena, B. Kelly, K. Anand, S. L. Madelyn, M. Michael, B. Franziska, V. Katharina, L. Daniel, N. Jorge, B. Lyudmila, H. K. Andrew, W. M. Korn, S. Ethan, C. Michael, Y. Xing, M. Thomas, L. Rachelle, H. Meghana, Y. Craig, K. Joshua, P. Yelena, S. Devin, N. David and K. Peter, Phys. Biol., 2012, 9, 016003 CrossRef PubMed.
- X.-X. Chen and F. Bai, Cancer Biol. Med., 2015, 12, 184–192 CAS.
- C. Bettegowda, M. Sausen, R. J. Leary, I. Kinde, Y. Wang, N. Agrawal, B. R. Bartlett, H. Wang, B. Luber, R. M. Alani, E. S. Antonarakis, N. S. Azad, A. Bardelli, H. Brem, J. L. Cameron, C. C. Lee, L. A. Fecher, G. L. Gallia, P. Gibbs, D. Le, R. L. Giuntoli, M. Goggins, M. D. Hogarty, M. Holdhoff, S.-M. Hong, Y. Jiao, H. H. Juhl, J. J. Kim, G. Siravegna, D. A. Laheru, C. Lauricella, M. Lim, E. J. Lipson, S. K. N. Marie, G. J. Netto, K. S. Oliner, A. Olivi, L. Olsson, G. J. Riggins, A. Sartore-Bianchi, K. Schmidt, L.-M. Shih, S. M. Oba-Shinjo, S. Siena, D. Theodorescu, J. Tie, T. T. Harkins, S. Veronese, T.-L. Wang, J. D. Weingart, C. L. Wolfgang, L. D. Wood, D. Xing, R. H. Hruban, J. Wu, P. J. Allen, C. M. Schmidt, M. A. Choti, V. E. Velculescu, K. W. Kinzler, B. Vogelstein, N. Papadopoulos and L. A. Diaz, Sci. Transl. Med., 2014, 6, 224ra224 Search PubMed.
- P. Mandel and P. Metais, C. R. Seances Soc. Biol. Fil., 1948, 142, 241–243 CAS.
- D. Koffler, V. Agnello, R. Winchester and H. G. Kunkel, J. Clin. Invest., 1973, 52, 198–204 CrossRef CAS PubMed.
- P. Anker, M. Stroun and P. A. Maurice, Cancer Res., 1975, 35, 2375–2382 CAS.
- P. Chomczynski and N. Sacchi, Anal. Biochem., 1987, 162, 156–159 CrossRef CAS PubMed.
- H. Cheng, C. Liu, J. Jiang, G. Luo, Y. Lu, K. Jin, M. Guo, Z. Zhang, J. Xu, L. Liu, Q. Ni and X. Yu, Int. J. Cancer, 2017, 140, 2344–2350 CrossRef CAS PubMed.
- F. Diehl, K. Schmidt, M. A. Choti, K. Romans, S. Goodman, M. Li, K. Thornton, N. Agrawal, L. Sokoll, S. A. Szabo, K. W. Kinzler, B. Vogelstein and L. A. Diaz, Nat. Med., 2008, 14, 985–990 CrossRef CAS PubMed.
- A. M. Newman, S. V. Bratman, J. To, J. F. Wynne, N. C. W. Eclov, L. A. Modlin, C. L. Liu, J. W. Neal, H. A. Wakelee, R. E. Merritt, J. B. Shrager, B. W. Loo, A. A. Alizadeh and M. Diehn, Nat. Med., 2014, 20, 548–554 CrossRef CAS PubMed.
- M. Roschewski, K. Dunleavy, S. Pittaluga, M. Moorhead, F. Pepin, K. Kong, M. Shovlin, E. S. Jaffe, L. M. Staudt, C. Lai, S. M. Steinberg, C. C. Chen, J. Zheng, T. D. Willis, M. Faham and W. H. Wilson, Lancet Oncol., 2015, 16, 541–549 CrossRef PubMed.
- Y. M. D. Lo, N. Corbetta, P. F. Chamberlain, V. Rai, I. L. Sargent, C. W. G. Redman and J. S. Wainscoat, Lancet, 1997, 350, 485–487 CrossRef CAS.
- R. W. K. Chiu and Y. M. D. Lo, Prenatal Diagn., 2012, 32, 401–406 CrossRef CAS PubMed.
- M. B. Freidin, D. V. Freydina, M. Leung, A. M. Fernandez, A. G. Nicholson and E. Lim, Clin. Chem., 2015, 61, 1299–1304 CrossRef CAS PubMed.
- E. Gormally, P. Hainaut, E. Caboux, L. Airoldi, H. Autrup, C. Malaveille, A. Dunning, S. Garte, G. Matullo, K. Overvad, A. Tjonneland, F. Clavel-Chapelon, P. Boffetta, H. Boeing, A. Trichopoulou, D. Palli, V. Krogh, R. Tumino, S. Panico, H. B. Bueno-De-Mesquita, P. H. Peeters, E. Lund, C. A. Gonzalez, C. Martinez, M. Dorronsoro, A. Barricarte, M. J. Tormo, J. R. Quiros, G. Berglund, G. Hallmans, N. E. Day, T. J. Key, F. Veglia, M. Peluso, T. Norat, R. Saracci, R. Kaaks, E. Riboli and P. Vineis, Int. J. Cancer, 2004, 111, 746–749 CrossRef CAS PubMed.
- J. Uchida, K. Kato, Y. Kukita, T. Kumagai, K. Nishino, H. Daga, I. Nagatomo, T. Inoue, M. Kimura, S. Oba, Y. Ito, K. Takeda and F. Imamura, Clin. Chem., 2015, 61, 1191–1196 CrossRef CAS PubMed.
- C. Thery, M. Ostrowski and E. Segura, Nat. Rev. Immunol., 2009, 9, 581–593 CrossRef CAS PubMed.
- H. Valadi, K. Ekstrom, A. Bossios, M. Sjostrand, J. J. Lee and J. O. Lotvall, Nat. Cell Biol., 2007, 9, 654–659 CrossRef CAS PubMed.
- J. Couzin, Science, 2005, 308, 1862–1863 CrossRef CAS PubMed.
- G. Raposo, H. W. Nijman, W. Stoorvogel, R. Liejendekker, C. V. Harding, C. J. Melief and H. J. Geuze, J. Exp. Med., 1996, 183, 1161–1172 CrossRef CAS PubMed.
- D. D. Taylor and C. Gercel-Taylor, Gynecol. Oncol., 2008, 110, 13–21 CrossRef CAS PubMed.
- J. de Vrij, S. L. N. Maas, M. van Nispen, M. Sena-Esteves, R. W. A. Limpens, A. J. Koster, S. Leenstra, M. L. Lamfers and M. L. D. Broekman, Nanomedicine, 2013, 8, 1443–1458 CrossRef CAS PubMed.
- M. M. Ferreira, V. C. Ramani and S. S. Jeffrey, Mol. Oncol., 2016, 10, 374–394 CrossRef CAS PubMed.
- K. Pantel and C. Alix-Panabières, Cancer Res., 2013, 73, 6384–6388 CrossRef CAS PubMed.
- C. Alix-Panabieres and K. Pantel, Cancer Discovery, 2016, 6, 479–491 CrossRef CAS PubMed.
- M. Umer, R. Vaidyanathan, N.-T. Nguyen and M. J. A. Shiddiky, Biotechnol. Adv., 2018, 36, 1367–1389 CrossRef CAS PubMed.
- K. Pantel and C. Alix-Panabières, Cancer Res., 2013, 73, 6384 CrossRef CAS PubMed.
- H. Schwarzenbach, D. S. B. Hoon and K. Pantel, Nat. Rev. Cancer, 2011, 11, 426 CrossRef CAS PubMed.
- M. Cristofanilli, G. T. Budd, M. J. Ellis, A. Stopeck, J. Matera, M. C. Miller, J. M. Reuben, G. V. Doyle, W. J. Allard, L. W. Terstappen and D. F. Hayes, N. Engl. J. Med., 2004, 351, 781–791 CrossRef CAS PubMed.
- E. S. Antonarakis, C. Lu, H. Wang, B. Luber, M. Nakazawa, J. C. Roeser, Y. Chen, T. A. Mohammad, Y. Chen, H. L. Fedor, T. L. Lotan, Q. Zheng, A. M. De Marzo, J. T. Isaacs, W. B. Isaacs, R. Nadal, C. J. Paller, S. R. Denmeade, M. A. Carducci, M. A. Eisenberger and J. Luo, N. Engl. J. Med., 2014, 371, 1028–1038 CrossRef PubMed.
- D. T. Miyamoto, Y. Zheng, B. S. Wittner, R. J. Lee, H. Zhu, K. T. Broderick, R. Desai, D. B. Fox, B. W. Brannigan, J. Trautwein, K. S. Arora, N. Desai, D. M. Dahl, L. V. Sequist, M. R. Smith, R. Kapur, C. L. Wu, T. Shioda, S. Ramaswamy, D. T. Ting, M. Toner, S. Maheswaran and D. A. Haber, Science, 2015, 349, 1351–1356 CrossRef CAS PubMed.
- H. Schwarzenbach, D. S. B. Hoon, K. Pantel, I. Kinde, J. R. Hecht, J. Berlin and B. Brandt, Nat. Rev. Cancer, 2011, 11, 426–437 CrossRef CAS PubMed.
- S. Keller, M. P. Sanderson, A. Stoeck and P. Altevogt, Immunol. Lett., 2006, 107, 102–108 CrossRef CAS PubMed.
- E. Zeringer, T. Barta, M. Li and A. V. Vlassov, Cold Spring Harb. Protoc., 2015 DOI:10.1101/pdb.top074476.
- P. Li, M. Kaslan, S. H. Lee, J. Yao and Z. Gao, Theranostics, 2017, 7, 789–804 CrossRef CAS PubMed.
- Y. Kang and K. Pantel, Cancer Cell, 2013, 23, 573–581 CrossRef CAS PubMed.
- V. Müller, N. Stahmann, S. Riethdorf, T. Rau, T. Zabel, A. Goetz, F. Jänicke and K. Pantel, Clin. Cancer Res., 2005, 11, 3678–3685 CrossRef PubMed.
- S. Braun, F. D. Vogl, B. Naume, W. Janni, M. P. Osborne, R. C. Coombes, G. Schlimok, I. J. Diel, B. Gerber, G. Gebauer, J.-Y. Pierga, C. Marth, D. Oruzio, G. Wiedswang, E.-F. Solomayer, G. Kundt, B. Strobl, T. Fehm, G. Y. C. Wong, J. Bliss, A. Vincent-Salomon and K. Pantel, N. Engl. J. Med., 2005, 353, 793–802 CrossRef CAS PubMed.
- M. M. Ferreira, V. C. Ramani and S. S. Jeffrey, Mol. Oncol., 2016, 10, 374–394 CrossRef CAS PubMed.
- T. W. Friedlander, G. Premasekharan and P. L. Paris, Pharmacol. Ther., 2014, 142, 271–280 CrossRef CAS PubMed.
- C. Alix-Panabieres and K. Pantel, Clin. Chem., 2013, 59, 110–118 CrossRef CAS PubMed.
- S. A. Joosse, T. M. Gorges and K. Pantel, EMBO Mol. Med., 2015, 7, 1–11 CrossRef CAS PubMed.
- E. Racila, D. Euhus, A. J. Weiss, C. Rao, J. McConnell, L. W. Terstappen and J. W. Uhr, Proc. Natl. Acad. Sci. U. S. A., 1998, 95, 4589–4594 CrossRef CAS.
- R. Kalluri and R. A. Weinberg, J. Clin. Invest., 2009, 119, 1420–1428 CrossRef CAS PubMed.
- S. D. Mikolajczyk, L. S. Millar, P. Tsinberg, S. M. Coutts, M. Zomorrodi, T. Pham, F. Z. Bischoff and T. J. Pircher, J. Oncol., 2011, 2011, 252361 Search PubMed.
- E. Ozkumur, A. M. Shah, J. C. Ciciliano, B. L. Emmink, D. T. Miyamoto, E. Brachtel, M. Yu, P. I. Chen, B. Morgan, J. Trautwein, A. Kimura, S. Sengupta, S. L. Stott, N. M. Karabacak, T. A. Barber, J. R. Walsh, K. Smith, P. S. Spuhler, J. P. Sullivan, R. J. Lee, D. T. Ting, X. Luo, A. T. Shaw, A. Bardia, L. V. Sequist, D. N. Louis, S. Maheswaran, R. Kapur, D. A. Haber and M. Toner, Sci. Transl. Med., 2013, 5, 179ra147 Search PubMed.
- W. Sheng, O. O. Ogunwobi, T. Chen, J. Zhang, T. J. George, C. Liu and Z. H. Fan, Lab Chip, 2014, 14, 89–98 RSC.
- H. J. Yoon, T. H. Kim, Z. Zhang, E. Azizi, T. M. Pham, C. Paoletti, J. Lin, N. Ramnath, M. S. Wicha, D. F. Hayes, D. M. Simeone and S. Nagrath, Nat. Nanotechnol., 2013, 8, 735–741 CrossRef CAS PubMed.
- S. L. Stott, C. H. Hsu, D. I. Tsukrov, M. Yu, D. T. Miyamoto, B. A. Waltman, S. M. Rothenberg, A. M. Shah, M. E. Smas, G. K. Korir, F. P. Floyd, Jr., A. J. Gilman, J. B. Lord, D. Winokur, S. Springer, D. Irimia, S. Nagrath, L. V. Sequist, R. J. Lee, K. J. Isselbacher, S. Maheswaran, D. A. Haber and M. Toner, Proc. Natl. Acad. Sci. U. S. A., 2010, 107, 18392–18397 CrossRef CAS PubMed.
- S. L. Stott, C. H. Hsu, D. I. Tsukrov, M. Yu, D. T. Miyamoto, B. A. Waltman, S. M. Rothenberg, A. M. Shah, M. E. Smas, G. K. Korir, F. P. Floyd Jr, A. J. Gilman, J. B. Lord, D. Winokur, S. Springer, D. Irimia, S. Nagrath, L. V. Sequist, R. J. Lee, K. J. Isselbacher, S. Maheswaran, D. A. Haber and M. Toner, Proc. Natl. Acad. Sci. U. S. A., 2010, 107, 18392–18397 CrossRef CAS PubMed.
- K. Kalinsky, J. A. Mayer, X. Xu, T. Pham, K. L. Wong, E. Villarin, T. J. Pircher, M. Brown, M. A. Maurer and F. Z. Bischoff, Clin. Transl. Oncol., 2015, 17, 539–546 CrossRef CAS PubMed.
- L. R. Huang, E. C. Cox, R. H. Austin and J. C. Sturm, Science, 2004, 304, 987–990 CrossRef CAS PubMed.
- R. Rosenberg, R. Gertler, J. Friederichs, K. Fuehrer, M. Dahm, R. Phelps, S. Thorban, H. Nekarda and J. R. Siewert, Cytometry, 2002, 49, 150–158 CrossRef CAS PubMed.
- G. Vona, A. Sabile, M. Louha, V. Sitruk, S. Romana, K. Schutze, F. Capron, D. Franco, M. Pazzagli, M. Vekemans, B. Lacour, C. Brechot and P. Paterlini-Brechot, Am. J. Pathol., 2000, 156, 57–63 CrossRef CAS PubMed.
- F. Guilak, J. R. Tedrow and R. Burgkart, Biochem. Biophys. Res. Commun., 2000, 269, 781–786 CrossRef CAS PubMed.
- E. S. Park, C. Jin, Q. Guo, R. R. Ang, S. P. Duffy, K. Matthews, A. Azad, H. Abdi, T. Todenhofer, J. Bazov, K. N. Chi, P. C. Black and H. Ma, Small, 2016, 12, 1909–1919 CrossRef CAS PubMed.
- R. D. Stoy, K. R. Foster and H. P. Schwan, Phys. Med. Biol., 1982, 27, 501–513 CrossRef CAS PubMed.
- N. Manaresi, A. Romani, G. Medoro, L. Altomare, A. Leonardi, M. Tartagni and R. Guerrieri, IEEE J. Solid-State Circuits, 2003, 38, 2297–2305 CrossRef.
- V. Gupta, I. Jafferji, M. Garza, V. O. Melnikova, D. K. Hasegawa, R. Pethig and D. W. Davis, Biomicrofluidics, 2012, 6, 24133 CrossRef PubMed.
- E. Sollier, D. E. Go, J. Che, D. R. Gossett, S. O'Byrne, W. M. Weaver, N. Kummer, M. Rettig, J. Goldman, N. Nickols, S. McCloskey, R. P. Kulkarni and D. Di Carlo, Lab Chip, 2014, 14, 63–77 RSC.
- J. Che, V. Yu, E. B. Garon, J. W. Goldman and D. Di Carlo, Lab Chip, 2017, 17, 1452–1461 RSC.
- E. Sinkala, E. Sollier-Christen, C. Renier, E. Rosàs-Canyelles, J. Che, K. Heirich, T. A. Duncombe, J. Vlassakis, K. A. Yamauchi, H. Huang, S. S. Jeffrey and A. E. Herr, Nat. Commun., 2017, 8, 14622 CrossRef CAS PubMed.
- M. E. Warkiani, B. L. Khoo, L. Wu, A. K. P. Tay, A. A. S. Bhagat, J. Han and C. T. Lim, Nat. Protoc., 2015, 11, 134 CrossRef PubMed.
- V. Gupta, I. Jafferji, M. Garza, V. O. Melnikova, D. K. Hasegawa, R. Pethig and D. W. Davis, Biomicrofluidics, 2012, 6, 024133 CrossRef PubMed.
- G. E. Hvichia, Z. Parveen, C. Wagner, M. Janning, J. Quidde, A. Stein, V. Müller, S. Loges, R. P. L. Neves, N. H. Stoecklein, H. Wikman, S. Riethdorf, K. Pantel and T. M. Gorges, Int. J. Cancer, 2016, 138, 2894–2904 CrossRef CAS PubMed.
- L. Xu, X. Mao, A. Imrali, F. Syed, K. Mutsvangwa, D. Berney, P. Cathcart, J. Hines, J. Shamash and Y.-J. Lu, PLoS One, 2015, 10, e0138032 CrossRef PubMed.
- T. Yeo, S. J. Tan, C. L. Lim, D. P. Lau, Y. W. Chua, S. S. Krisna, G. Iyer, G. S. Tan, T. K. Lim, D. S. Tan, W. T. Lim and C. T. Lim, Sci. Rep., 2016, 6, 22076 CrossRef CAS PubMed.
- B. L. Khoo, S. C. Lee, P. Kumar, T. Z. Tan, M. E. Warkiani, S. G. Ow, S. Nandi, C. T. Lim and J. P. Thiery, Oncotarget, 2015, 6, 15578–15593 CrossRef PubMed.
- B. L. Khoo, G. Grenci, T. Jing, Y. B. Lim, S. C. Lee, J. P. Thiery, J. Han and C. T. Lim, Sci. Adv., 2016, 2, e1600274 CrossRef PubMed.
- G. A. Clawson, E. Kimchi, S. D. Patrick, P. Xin, R. Harouaka, S. Zheng, A. Berg, T. Schell, K. F. Staveley-O'Carroll, R. I. Neves, P. J. Mosca and D. Thiboutot, PLoS One, 2012, 7, e41052 CrossRef CAS PubMed.
- V. Muller, N. Stahmann, S. Riethdorf, T. Rau, T. Zabel, A. Goetz, F. Janicke and K. Pantel, Clin. Cancer Res., 2005, 11, 3678–3685 CrossRef PubMed.
- V. Hofman, M. I. Ilie, E. Long, E. Selva, C. Bonnetaud, T. Molina, N. Venissac, J. Mouroux, P. Vielh and P. Hofman, Int. J. Cancer, 2011, 129, 1651–1660 CrossRef CAS PubMed.
- M. Ilie, V. Hofman, E. Long-Mira, E. Selva, J. M. Vignaud, B. Padovani, J. Mouroux, C. H. Marquette and P. Hofman, PLoS One, 2014, 9, e111597 CrossRef PubMed.
- L. Xu, X. Mao, P. Y. Chan, J. Shamash and Y.-J. Lu, Lancet, 2016, 387, S25 CrossRef.
- M. C. Miller, P. S. Robinson, C. Wagner and D. J. O'Shannessy, Cytometry, Part A, 2018, 0, 1–6 Search PubMed.
- T. Yeo, S. J. Tan, C. L. Lim, D. P. X. Lau, Y. W. Chua, S. S. Krisna, G. Iyer, G. S. Tan, T. K. H. Lim, D. S. W. Tan, W.-T. Lim and C. T. Lim, Sci. Rep., 2016, 6, 22076 CrossRef CAS PubMed.
- Y. J. Jan, J.-F. Chen, Y. Zhu, Y.-T. Lu, S. H. Chen, H. Chung, M. Smalley, Y.-W. Huang, J. Dong, L.-C. Chen, H.-H. Yu, J. S. Tomlinson, S. Hou, V. G. Agopian, E. M. Posadas and H.-R. Tseng, Adv. Drug Delivery Rev., 2018, 125, 78–93 CrossRef CAS PubMed.
- Z. Ke, M. Lin, J.-F. Chen, J.-S. Choi, Y. Zhang, A. Fong, A.-J. Liang, S.-F. Chen, Q. Li, W. Fang, P. Zhang, M. A. Garcia, T. Lee, M. Song, H.-A. Lin, H. Zhao, S.-C. Luo, S. Hou, H.-H. Yu and H.-R. Tseng, ACS Nano, 2015, 9, 62–70 CrossRef CAS PubMed.
- S. Wang, K. Liu, J. Liu, Z. T. F. Yu, X. Xu, L. Zhao, T. Lee, E. K. Lee, J. Reiss, Y.-K. Lee, L. W. K. Chung, J. Huang, M. Rettig, D. Seligson, K. N. Duraiswamy, C. K. F. Shen and H.-R. Tseng, Angew. Chem., Int. Ed., 2011, 50, 3084–3088 CrossRef CAS PubMed.
- S. Wang, H. Wang, J. Jiao, K.-J. Chen, G. E. Owens, K.-I. Kamei, J. Sun, D. J. Sherman, C. P. Behrenbruch, H. Wu and H.-R. Tseng, Angew. Chem., Int. Ed., 2009, 48, 8970–8973 CrossRef CAS PubMed.
- J. Ko, N. Bhagwat, S. S. Yee, T. Black, C. Redlinger, J. Romeo, M. O'Hara, A. Raj, E. L. Carpenter, B. Z. Stanger and D. Issadore, Lab Chip, 2017, 17, 3086–3096 RSC.
- J. H. Myung, M. J. Eblan, J. M. Caster, S.-J. Park, M. J. Poellmann, K. Wang, K. A. Tam, S. M. Miller, C. Shen, R. C. Chen, T. Zhang, J. E. Tepper, B. S. Chera, A. Z. Wang and S. Hong, Clin. Cancer Res., 2018, 24, 2539 CrossRef CAS PubMed.
- S. H. Au, J. Edd, A. E. Stoddard, K. H. K. Wong, F. Fachin, S. Maheswaran, D. A. Haber, S. L. Stott, R. Kapur and M. Toner, Sci. Rep., 2017, 7, 2433 CrossRef PubMed.
- A. F. Sarioglu, N. Aceto, N. Kojic, M. C. Donaldson, M. Zeinali, B. Hamza, A. Engstrom, H. Zhu, T. K. Sundaresan, D. T. Miyamoto, X. Luo, A. Bardia, B. S. Wittner, S. Ramaswamy, T. Shioda, D. T. Ting, S. L. Stott, R. Kapur, S. Maheswaran, D. A. Haber and M. Toner, Nat. Methods, 2015, 12, 685 CrossRef CAS PubMed.
- Z. Wang, N. Sun, M. Liu, Y. Cao, K. Wang, J. Wang and R. Pei, ACS Sens., 2017, 2, 547–552 CrossRef CAS PubMed.
- H. J. Yoon, T. H. Kim, Z. Zhang, E. Azizi, T. M. Pham, C. Paoletti, J. Lin, N. Ramnath, M. S. Wicha, D. F. Hayes, D. M. Simeone and S. Nagrath, Nat. Nanotechnol., 2013, 8, 735 CrossRef CAS PubMed.
- H. J. Yoon, A. Shanker, Y. Wang, M. Kozminsky, Q. Jin, N. Palanisamy, M. L. Burness, E. Azizi, D. M. Simeone, M. S. Wicha, J. Kim and S. Nagrath, Adv. Mater., 2016, 28, 4891–4897 CrossRef CAS PubMed.
- P. Li, Z. Mao, Z. Peng, L. Zhou, Y. Chen, P.-H. Huang, C. I. Truica, J. J. Drabick, W. S. El-Deiry, M. Dao, S. Suresh and T. J. Huang, Proc. Natl. Acad. Sci. U. S. A., 2015, 112, 4970–4975 CrossRef CAS PubMed.
- B. L. Khoo, G. Grenci, Y. B. Lim, S. C. Lee, J. Han and C. T. Lim, Nat. Protoc., 2017, 13, 34 CrossRef PubMed.
- L. Kermanshah, M. Poudineh, S. Ahmed, L. N. M. Nguyen, S. Srikant, R. Makonnen, F. Pena Cantu, M. Corrigan and S. O. Kelley, Lab Chip, 2018, 18, 2055–2064 RSC.
- B. Shapiro, M. Chakrabarty, E. M. Cohn and S. A. Leon, Cancer, 1983, 51, 2116–2120 CrossRef CAS PubMed.
- K. Birkenkamp-Demtröder, I. Nordentoft, E. Christensen, S. Høyer, T. Reinert, S. Vang, M. Borre, M. Agerbæk, J. B. Jensen, T. F. Ørntoft and L. Dyrskjøt, Eur. Urol., 2016, 70, 75–82 CrossRef PubMed.
- D. Sidransky, T. Tokino, S. R. Hamilton, K. W. Kinzler, D. Sidransky, T. Tokino, S. R. Hamilton, K. W. Kinzler, B. Levin, P. Frost and B. Vogelstein, Science, 2016, 256, 102–105 CrossRef.
- S. K. Mithani, I. M. Smith, S. Zhou, A. Gray, W. M. Koch, A. Maitra and J. A. Califano, Clin. Cancer Res., 2007, 13, 7335–7340 CrossRef CAS PubMed.
- S. A. Vogelaar, D. Posthuma, D. Boomsma and C. Kluft, Vasc. Pharmacol., 2002, 39, 123–125 CrossRef CAS PubMed.
- A. Zinkova, I. Brynychova, A. Svacina, M. Jirkovska and M. Korabecna, Sci. Rep., 2017, 7, 2591 CrossRef PubMed.
- S. R. Morgan, J. Morgan, E. Jessica Whiteley, J. Emma Donald, M. T. John Smith, E. M. T. Eisenberg, L. E. Kallam and K.-M. Lauren, Clin. Med. Insights: Pathol., 2012, 5, 15 CAS.
- S. L. Fong, J. T. Zhang, C. K. Lim, K. W. Eu and Y. Liu, Clin. Chem., 2009, 55(3), 587–589 CrossRef CAS PubMed.
- E. Omiccioli, P. Maltese, M. Menotta, G. Amagliani and M. Magnani, J. Biotechnol., 2010, 150, 461–462 CrossRef.
- T. M. Dias, F. A. Cardoso, S. A. M. Martins, V. C. Martins, S. Cardoso, J. F. Gaspar, G. Monteiro and P. P. Freitas, Anal. Methods, 2016, 8, 119–128 RSC.
- M. Guha, E. Castellanos-Rizaldos, P. Liu, H. Mamon and G. M. Makrigiorgos, Nucleic Acids Res., 2013, 41, 1–9 CrossRef PubMed.
- Z. Gui, Q. Wang, J. Li, M. Zhu, L. Yu, T. Xun, F. Yan and H. Ju, Talanta, 2016, 154, 520–525 CrossRef CAS PubMed.
- Z. Chen, G. Liu, M. Chen, X. Chen, M. Wu and X. Chen, Comb. Chem. High Throughput Screening, 2010, 13, 383–392 CrossRef CAS PubMed.
- S. H. Jeon, H. J. Lee, K. Bae, K. A. Yoon, E. S. Lee and Y. Cho, Theranostics, 2016, 6(6), 828–836 CrossRef CAS PubMed.
- A. Sonnenberg, J. Y. Marciniak, L. Rassenti, E. M. Ghia, E. A. Skowronski, S. Manouchehri, J. McCanna, G. F. Widhopf, T. J. Kipps and M. J. Heller, Clin. Chem., 2014, 60(3), 500–509 CrossRef CAS PubMed.
- J. Das, I. Ivanov, E. H. Sargent and S. O. Kelley, J. Am. Chem. Soc., 2016, 138, 11009–11016 CrossRef CAS PubMed.
- M. Fleischhacker, B. Schmidt, S. Weickmann, D. M. I. Fersching, G. S. Leszinski, B. Siegele, O. J. Stötzer, D. Nagel and S. Holdenrieder, Clin. Chim. Acta, 2011, 412, 2085–2088 CrossRef CAS PubMed.
- A. K. Arrington, E. L. Heinrich, W. Lee, M. Duldulao, S. Patel, J. Sanchez, J. Garcia-Aguilar and J. Kim, Int. J. Mol. Sci., 2012, 13, 12153–12168 CrossRef CAS PubMed.
- W. Pao, V. Miller, M. Zakowski, J. Doherty, K. Politi, I. Sarkaria, B. Singh, R. Heelan, V. Rusch, L. Fulton, E. Mardis, D. Kupfer, R. Wilson, M. Kris and H. Varmus, Proc. Natl. Acad. Sci. U. S. A., 2004, 101, 13306–13311 CrossRef CAS PubMed.
- L. V. Sequist, J.-C. Soria, J. W. Goldman, H. A. Wakelee, S. M. Gadgeel, A. Varga, V. Papadimitrakopoulou, B. J. Solomon, G. R. Oxnard, R. Dziadziuszko, D. L. Aisner, R. C. Doebele, C. Galasso, E. B. Garon, R. S. Heist, J. Logan, J. W. Neal, M. A. Mendenhall, S. Nichols, Z. Piotrowska, A. J. Wozniak, M. Raponi, C. A. Karlovich, S. Jaw-Tsai, J. Isaacson, D. Despain, S. L. Matheny, L. Rolfe, A. R. Allen and D. R. Camidge, N. Engl. J. Med., 2015, 372, 1700–1709 CrossRef PubMed.
- B. Vogelstein and K. W. Kinzler, Proc. Natl. Acad. Sci. U. S. A., 1999, 96, 9236–9241 CrossRef CAS.
- D. Pekin, Y. Skhiri, J.-C. Baret, D. Le Corre, L. Mazutis, C. Ben Salem, F. Millot, A. El Harrak, J. B. Hutchison, J. W. Larson, D. R. Link, P. Laurent-Puig, A. D. Griffiths and V. Taly, Lab Chip, 2011, 11, 2156–2166 RSC.
- V. Taly, D. Pekin, L. Benhaim, S. K. Kotsopoulos, D. Le Corre, X. Li, I. Atochin, D. R. Link, A. D. Griffiths, K. Pallier, H. Blons, O. Bouché, B. Landi, J. B. Hutchison and P. Laurent-Puig, Clin. Chem., 2013, 59, 1722 CrossRef CAS PubMed.
- I. Garcia-Murillas, G. Schiavon, B. Weigelt, C. Ng, S. Hrebien, R. J. Cutts, M. Cheang, P. Osin, A. Nerurkar, I. Kozarewa, J. A. Garrido, M. Dowsett, J. S. Reis-Filho, I. E. Smith and N. C. Turner, Sci. Transl. Med., 2015, 7, 302ra133 CrossRef PubMed.
- T. K. F. Yung, K. C. A. Chan, T. S. K. Mok, J. Tong, K.-F. To and Y. M. D. Lo, Clin. Cancer Res., 2009, 15, 2076 CrossRef CAS PubMed.
- F. Diehl, M. Li, D. Dressman, Y. He, D. Shen, S. Szabo, L. A. Diaz, S. N. Goodman, K. A. David, H. Juhl, K. W. Kinzler and B. Vogelstein, Proc. Natl. Acad. Sci. U. S. A., 2005, 102, 16368 CrossRef CAS PubMed.
- D. Dressman, H. Yan, G. Traverso, K. W. Kinzler and B. Vogelstein, Proc. Natl. Acad. Sci. U. S. A., 2003, 100, 8817 CrossRef CAS PubMed.
- G. Perkins, T. A. Yap, L. Pope, A. M. Cassidy, J. P. Dukes, R. Riisnaes, C. Massard, P. A. Cassier, S. Miranda, J. Clark, K. A. Denholm, K. Thway, D. Gonzalez De Castro, G. Attard, L. R. Molife, S. B. Kaye, U. Banerji and J. S. de Bono, PLoS One, 2012, 7, e47020 CrossRef CAS PubMed.
- R. E. Board, A. M. Wardley, J. M. Dixon, A. C. Armstrong, S. Howell, L. Renshaw, E. Donald, A. Greystoke, M. Ranson, A. Hughes and C. Dive, Breast Cancer Res. Treat., 2010, 120, 461–467 CrossRef CAS PubMed.
- J. Shi, Q. Liu and S. S. Sommer, Hum. Mutat., 2006, 28, 131–136 CrossRef PubMed.
- T. Forshew, M. Murtaza, C. Parkinson, D. Gale, D. W. Y. Tsui, F. Kaper, S.-J. Dawson, A. M. Piskorz, M. Jimenez-Linan, D. Bentley, J. Hadfield, A. P. May, C. Caldas, J. D. Brenton and N. Rosenfeld, Sci. Transl. Med., 2012, 4, 136ra168 Search PubMed.
- I. Kinde, J. Wu, N. Papadopoulos, K. W. Kinzler and B. Vogelstein, Proc. Natl. Acad. Sci. U. S. A., 2011, 108, 9530 CrossRef PubMed.
- F. Rothé, J. F. Laes, D. Lambrechts, D. Smeets, D. Vincent, M. Maetens, D. Fumagalli, S. Michiels, S. Drisis, C. Moerman, J. P. Detiffe, D. Larsimont, A. Awada, M. Piccart, C. Sotiriou and M. Ignatiadis, Ann. Oncol., 2014, 25, 1959–1965 CrossRef PubMed.
- A. M. Newman, S. V. Bratman, J. To, J. F. Wynne, N. C. W. Eclov, L. A. Modlin, C. L. Liu, J. W. Neal, H. A. Wakelee, R. E. Merritt, J. B. Shrager, B. W. Loo Jr, A. A. Alizadeh and M. Diehn, Nat. Med., 2014, 20, 548 CrossRef CAS PubMed.
- M. Sausen, R. J. Leary, S. Jones, J. Wu, C. P. Reynolds, X. Liu, A. Blackford, G. Parmigiani, L. A. Diaz Jr, N. Papadopoulos, B. Vogelstein, K. W. Kinzler, V. E. Velculescu and M. D. Hogarty, Nat. Genet., 2012, 45, 12 CrossRef PubMed.
- J. D. Thompson, G. Shibahara, S. Rajan, J. Pel and A. Marziali, PLoS One, 2012, 7, e31597 CrossRef CAS PubMed.
- K. C. A. Chan, P. Jiang, Y. W. L. Zheng, G. J. W. Liao, H. Sun, J. Wong, S. S. N. Siu, W. C. Chan, S. L. Chan, A. T. C. Chan, P. B. S. Lai, R. W. K. Chiu and Y. M. D. Lo, Clin. Chem., 2013, 59, 211 CrossRef CAS PubMed.
- T.-L. Wang, C. Maierhofer, M. R. Speicher, C. Lengauer, B. Vogelstein, K. W. Kinzler and V. E. Velculescu, Proc. Natl. Acad. Sci. U. S. A., 2002, 99, 16156 CrossRef CAS PubMed.
- R. J. Leary, I. Kinde, F. Diehl, K. Schmidt, C. Clouser, C. Duncan, A. Antipova, C. Lee, K. McKernan, F. M. De La Vega, K. W. Kinzler, B. Vogelstein, L. A. Diaz and V. E. Velculescu, Sci. Transl. Med., 2010, 2, 20ra14 Search PubMed.
- R. J. Leary, M. Sausen, I. Kinde, N. Papadopoulos, J. D. Carpten, D. Craig, J. O'Shaughnessy, K. W. Kinzler, G. Parmigiani, B. Vogelstein, L. A. Diaz and V. E. Velculescu, Sci. Transl. Med., 2012, 4, 162ra154 Search PubMed.
- D. J. McBride, A. K. Orpana, C. Sotiriou, H. Joensuu, P. J. Stephens, L. J. Mudie, E. Hämäläinen, L. A. Stebbings, L. C. Andersson, A. M. Flanagan, V. Durbecq, M. Ignatiadis, O. Kallioniemi, C. A. Heckman, K. Alitalo, H. Edgren, P. A. Futreal, M. R. Stratton and P. J. Campbell, Genes, Chromosomes Cancer, 2010, 49, 1062–1069 CrossRef CAS PubMed.
- E. Heitzer, P. Ulz, J. Belic, S. Gutschi, F. Quehenberger, K. Fischereder, T. Benezeder, M. Auer, C. Pischler, S. Mannweiler, M. Pichler, F. Eisner, M. Haeusler, S. Riethdorf, K. Pantel, H. Samonigg, G. Hoefler, H. Augustin, J. B. Geigl and M. R. Speicher, Genome Med., 2013, 5, 30 CrossRef CAS PubMed.
- D. Dressman, H. Yan, G. Traverso, K. W. Kinzler and B. Vogelstein, Proc. Natl. Acad. Sci. U. S. A., 2003, 100, 8817–8822 CrossRef CAS PubMed.
- R. J. Leary, I. Kinde, F. Diehl, K. Schmidt, C. Clouser, C. Duncan, A. Antipova, C. Lee, K. McKernan, F. M. De La Vega, K. W. Kinzler, B. Vogelstein, L. A. Diaz and V. E. Velculescu, Sci. Transl. Med., 2010, 2, 20ra14 Search PubMed.
- T.-L. Wang, C. Maierhofer, M. R. Speicher, C. Lengauer, B. Vogelstein, K. W. Kinzler and V. E. Velculescu, Proc. Natl. Acad. Sci. U. S. A., 2002, 99, 16156–16161 CrossRef CAS PubMed.
- R. E. Lane, D. Korbie, W. Anderson, R. Vaidyanathan and M. Trau, Sci. Rep., 2015, 5, 7639 CrossRef PubMed.
- B. J. Tauro, D. W. Greening, R. A. Mathias, S. Mathivanan, H. Ji and R. J. Simpson, Mol. Cell. Proteomics, 2013, 12, 587–598 CrossRef CAS PubMed.
- H. Kalra, C. G. Adda, M. Liem, C. S. Ang, A. Mechler, R. J. Simpson, M. D. Hulett and S. Mathivanan, Proteomics, 2013, 13, 3354–3364 CrossRef CAS PubMed.
- D. D. Taylor and S. Shah, Methods, 2015, 87, 3–10 CrossRef CAS PubMed.
- C. Chen, J. Skog, C. H. Hsu, R. T. Lessard, L. Balaj, T. Wurdinger, B. S. Carter, X. O. Breakefield, M. Toner and D. Irimia, Lab Chip, 2010, 10, 505–511 RSC.
- S. S. Kanwar, C. J. Dunlay, D. M. Simeone and S. Nagrath, Lab Chip, 2014, 14, 1891–1900 RSC.
- H. Shao, J. Chung, L. Balaj, A. Charest, D. D. Bigner, B. S. Carter, F. H. Hochberg, X. O. Breakefield, R. Weissleder and H. Lee, Nat. Med., 2012, 18, 1835–1840 CrossRef CAS PubMed.
- H. Im, H. Shao, Y. I. Park, V. M. Peterson, C. M. Castro, R. Weissleder and H. Lee, Nat. Biotechnol., 2014, 32, 490–495 CrossRef CAS PubMed.
- C. Liu, J. Guo, F. Tian, N. Yang, F. Yan, Y. Ding, J. Wei, G. Hu, G. Nie and J. Sun, ACS Nano, 2017, 11, 6968–6976 CrossRef CAS PubMed.
- L.-G. Liang, M.-Q. Kong, S. Zhou, Y.-F. Sheng, P. Wang, T. Yu, F. Inci, W. P. Kuo, L.-J. Li, U. Demirci and S. Wang, Sci. Rep., 2017, 7, 46224 CrossRef PubMed.
- B. H. Wunsch, J. T. Smith, S. M. Gifford, C. Wang, M. Brink, R. L. Bruce, R. H. Austin, G. Stolovitzky and Y. Astier, Nat. Nanotechnol., 2016, 11, 936–940 CrossRef CAS PubMed.
- J. C. Yeo, Kenry, Z. Zhao, P. Zhang, Z. Wang and C. T. Lim, Biomicrofluidics, 2018, 12, 024103 CrossRef.
- K. Lee, H. Shao, R. Weissleder and H. Lee, ACS Nano, 2015, 9, 2321–2327 CrossRef CAS PubMed.
- C. Chen, J. Skog, C.-H. Hsu, R. T. Lessard, L. Balaj, T. Wurdinger, B. S. Carter, X. O. Breakefield, M. Toner and D. Irimia, Lab Chip, 2010, 10, 505–511 RSC.
- S. S. Kanwar, C. J. Dunlay, D. M. Simeone and S. Nagrath, Lab Chip, 2014, 14, 1891–1900 RSC.
- M. He, J. Crow, M. Roth, Y. Zeng and A. K. Godwin, Lab Chip, 2014, 14, 3773–3780 RSC.
- S. D. Ibsen, J. Wright, J. M. Lewis, S. Kim, S.-Y. Ko, J. Ong, S. Manouchehri, A. Vyas, J. Akers, C. C. Chen, B. S. Carter, S. C. Esener and M. J. Heller, ACS Nano, 2017, 11, 6641–6651 CrossRef CAS PubMed.
- R. Vaidyanathan, M. Naghibosadat, S. Rauf, D. Korbie, L. G. Carrascosa, M. J. A. Shiddiky and M. Trau, Anal. Chem., 2014, 86, 11125–11132 CrossRef CAS PubMed.
- X. Doldán, P. Fagúndez, A. Cayota, J. Laíz and J. P. Tosar, Anal. Chem., 2016, 88, 10466–10473 CrossRef PubMed.
- S. Wang, L. Zhang, S. Wan, S. Cansiz, C. Cui, Y. Liu, R. Cai, C. Hong, I. T. Teng, M. Shi, Y. Wu, Y. Dong and W. Tan, ACS Nano, 2017, 11(4), 3943–3949 CrossRef CAS PubMed.
- J. Ko, M. A. Hemphill, D. Gabrieli, L. Wu, V. Yelleswarapu, G. Lawrence, W. Pennycooke, A. Singh, D. F. Meaney and D. Issadore, Sci. Rep., 2016, 6, 31215 CrossRef CAS PubMed.
- S. Olcum, N. Cermak, S. C. Wasserman, K. S. Christine, H. Atsumi, K. R. Payer, W. Shen, J. Lee, A. M. Belcher, S. N. Bhatia and S. R. Manalis, Proc. Natl. Acad. Sci. U. S. A., 2014, 111, 1310–1315 CrossRef CAS PubMed.
- Y. Yoshioka, N. Kosaka, Y. Konishi, H. Ohta, H. Okamoto, H. Sonoda, R. Nonaka, H. Yamamoto, H. Ishii, M. Mori, K. Furuta, T. Nakajima, H. Hayashi, H. Sugisaki, H. Higashimoto, T. Kato, F. Takeshita and T. Ochiya, Nat. Commun., 2014, 5, 3591 CrossRef PubMed.
- H. Shao, J. Chung, K. Lee, L. Balaj, C. Min, B. S. Carter, F. H. Hochberg, X. O. Breakefield, H. Lee and R. Weissleder, Nat. Commun., 2015, 6, 6999 CrossRef CAS PubMed.
- F. Liu, O. Vermesh, V. Mani, T. J. Ge, S. J. Madsen, A. Sabour, E.-C. Hsu, G. Gowrishankar, M. Kanada, J. V. Jokerst, R. G. Sierra, E. Chang, K. Lau, K. Sridhar, A. Bermudez, S. J. Pitteri, T. Stoyanova, R. Sinclair, V. S. Nair, S. S. Gambhir and U. Demirci, ACS Nano, 2017, 11, 10712–10723 CrossRef CAS PubMed.
- H.-K. Woo, V. Sunkara, J. Park, T.-H. Kim, J.-R. Han, C.-J. Kim, H.-I. Choi, Y.-K. Kim and Y.-K. Cho, ACS Nano, 2017, 11, 1360–1370 CrossRef CAS PubMed.
- S. Jeong, J. Park, D. Pathania, C. M. Castro, R. Weissleder and H. Lee, ACS Nano, 2016, 10, 1802–1809 CrossRef CAS PubMed.
- N. L. Syn, L. Wang, E. K.-H. Chow, C. T. Lim and B.-C. Goh, Trends Biotechnol., 2017, 35, 665–676 CrossRef CAS PubMed.
- M. Heidary, M. Auer, P. Ulz, E. Heitzer, E. Petru, C. Gasch, S. Riethdorf, O. Mauermann, I. Lafer, G. Pristauz, S. Lax, K. Pantel, J. B. Geigl and M. R. Speicher, Breast Cancer Res., 2014, 16, 421 CrossRef PubMed.
- B. L. Khoo, G. Grenci, T. Jing, Y. B. Lim, S. C. Lee, J. P. Thiery, J. Han and C. T. Lim, Sci. Adv., 2016, 2, e1600274 CrossRef PubMed.
- C. L. Hodgkinson, C. J. Morrow, Y. Li, R. L. Metcalf, D. G. Rothwell, F. Trapani, R. Polanski, D. J. Burt, K. L. Simpson, K. Morris, S. D. Pepper, D. Nonaka, A. Greystoke, P. Kelly, B. Bola, M. G. Krebs, J. Antonello, M. Ayub, S. Faulkner, L. Priest, L. Carter, C. Tate, C. J. Miller, F. Blackhall, G. Brady and C. Dive, Nat. Med., 2014, 20, 897 CrossRef CAS PubMed.
|
This journal is © The Royal Society of Chemistry 2019 |
Click here to see how this site uses Cookies. View our privacy policy here.