DOI:
10.1039/C8GC02059K
(Critical Review)
Green Chem., 2019,
21, 9-35
Recent progress in theoretical and computational studies on the utilization of lignocellulosic materials
Received
4th July 2018
, Accepted 2nd November 2018
First published on 3rd November 2018
Abstract
Lignocellulosic materials were conventionally treated as wastes for disposal, but have now become very important in modern life due to their great roles in the supply of renewable resources. Recently, significant attention has been paid to the dissolution of cellulose, hemicellulose, and lignin, and the production of chemicals and fuels from these feedstocks. It has been demonstrated that lignocellulose has great potential from a sustainable economy perspective and this field has been developing rapidly. Thus, it is critical to review the recent progress in studies on the utilization of lignocellulosic materials. In particular, this work was designed to highlight recent computation-based studies on the chemistry of lignocellulosic materials, from the structural and energetic properties of individual lignocellulose components to their reaction mechanisms in various systems. Importantly, this review summarizes some major simulation studies on the utilization of cellulose, hemicellulose, and lignin via dissolution, catalytic conversion, and pyrolysis by using density functional theory, first-principles calculation, molecular dynamics simulation, and conductor-like screening models. It has been shown that the theoretical and computational investigations play a key role in the understanding of the efficient utilization of lignocellulose.
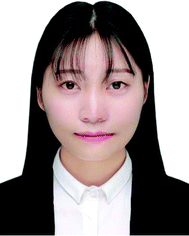 Yaqin Zhang | Yaqin Zhang received her dual master's degree in Chemical Engineering from the Technical University of Denmark (DTU) and University of Chinese Academy of Sciences in 2017, and then she joined the Institute of Process Engineering (IPE), CAS. Her research interests mainly focus on density functional theory calculations and molecular dynamics simulations of the dissolution and conversion of lignocellulosic materials with ionic liquids. |
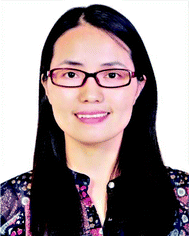 Hongyan He | Dr Hongyan He is an associate professor of applied chemistry at the Institute of Process Engineering (IPE), Chinese Academy of Sciences (CAS). She was a visiting scholar at the University of Wyoming, working with Prof. Maohong Fan. She was elected as a member of the Youth Innovation Promotion Association of CAS in 2016 and received the award of CAS Science and Technology Promotion and Development Prize in 2017. Her current research focuses on the structure–property relationship of ionic liquid systems, CO2 capture and utilization, and the investigation of fundamental reaction processes of biomass and their applications. |
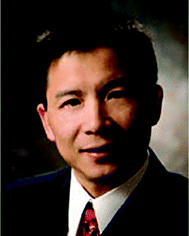 Maohong Fan | Dr Maohong Fan is a SER Professor of Chemical and Petroleum Engineering in the University of Wyoming. He has led and worked on many projects in the areas of chemical production, clean energy production and environmental protection. These projects have been supported by various domestic and international funding agencies such as NSF, DOE, EPA, USGS and USDA in the United States. His refereed books, book chapters, and papers in different chemical and environmental engineering, energy, and chemistry journals are listed in https://scholar.google.com/citations?user=ocl_AloAAAAJ&hl=en. |
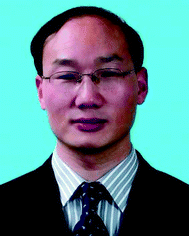 Suojiang Zhang | Prof. Suojiang Zhang is Professor & Director General of the Institute of Process Engineering (IPE), Chinese Academy of Sciences (CAS), an academician of CAS and a Fellow of the RSC. He received his Ph.D. in Physical Chemistry from Zhejiang University in 1994. He currently serves as the Editor-in-Chief of Green Energy & Environment (GEE) and Process Engineering Journal of China. His research focuses on ionic liquids, green process engineering, and large-scale energy storage. |
1. General overview
With the depletion of non-renewable fossil energy sources, such as oil, coal and natural gas, and the deterioration of the ecological environment, the sustainable development and efficient utilization of renewable resources is of great importance in easing the energy shortage and environmental challenges of humankind.1,2 Biomass has been considered as a potential alternative energy resource to fossil fuels because it is abundant, low cost, less polluting, and most importantly, renewable. Currently, China has pledged to increase its non-fossil fuel consumption to 11.4% of the energy mix in the 12th five-year plan, and biomass energy will totally replace about 58 million metric tons of fossil-based energy by 2020 in the 13th five year plan.3,4 In a report by the US Department of Energy, the United States is to increase the volume of renewable fuel to 36 billion gallons by 2022 and about 30% of the total transportation fuels will be produced from biomass by 2030.5 Denmark has also set a goal to cover 35% of its total energy consumption by 2020 using renewable energy (focus on wind and biomass).6 Obviously, biomass resource is a huge treasure for human beings that attracts global attention. The first-generation biofuels such as bioethanol and biodiesel derived from corn and wheat have contributed to the economies of many countries. However, they consume a large quantity of food crops during the production processes, thus competing with the primary needs of humans.7 The second-generation biofuels from lignocellulose would have many advantages in the production of a variety of high-energy fuels, functional materials, and value-added chemicals, as well as in addressing environmental concerns.8,9
Lignocellulosic biomass, such as wheat straw, corn stover, switchgrass, sugarcane bagasse, etc., is the only renewable carbon resource in nature that consists mainly of cellulose, lignin, and hemicellulose.10 Among these, cellulose bears the highest content with a mass fraction of up to 30% to 50%, lignin accounts for 10% to 30%, and hemicellulose ranges from 20% to 30%.7,11 Statistics show that annually 170 million metric tons of lignocellulose are produced,12 while no more than 5% of these lignocellulosic materials are used for diverse applications because of their recalcitrance.13 To carry out innovative valorization of these lignocellulosic materials, many efforts have been devoted to converting them into target chemicals and fuels.14–16 The transformation processes studied include oxidation, hydrogenation, dehydration, pyrolysis, catalyzed depolymerization, dissolution, and biorefining.17–19 For instance, thermal reactions are exploited in the pyrolysis of biomass feedstock to liquid fuels and syngas or charcoal to provide heat or power.20 The dissolution route and catalyzed depolymerization are usually combined to generate sugars and residual lignin.21 The sugars can be subsequently converted into chemical compounds via catalysis or biorefining.7
As a consequence, different high-value-added products have been generated from lignocellulose.1 A series of organic acids (e.g., levulinic acid and lactic acid), alcohols (e.g., ethanol, ethylene glycol, and propylene glycol), and furfural-based compounds are derived from cellulose and hemicellulose.22 Diverse aromatic compounds (e.g., phenols, ferulic acid, and methyl p-hydroxycinnamate) could be obtained by the selective cleavage of C–C and C–O bonds of lignin.23 To the best of our knowledge, some of these compounds can serve as platform chemicals that are further converted, through various catalytic reaction routes, into target bulk chemicals.1,24 Not surprisingly, the production of value-added chemicals and fuels from lignocellulose has become an intensively active field and this concept is in good agreement with the recent sustainable development.10,25
Nevertheless, such processes as dissolution, pyrolysis or catalytic conversion all involve complex reactions and suffer from the recalcitrance of the carbohydrate polymers. However, the experimental results to some extent lack an in-depth recognition of dissolution and conversion mechanisms. Apart from the experimental studies, more and more researchers are studying the intrinsic characteristics by computer modelling and simulation, which are able to provide an insightful view at the atomic level. For example, molecular simulation, including quantum chemical (QC) methods and molecular dynamics (MD) simulation, can offer an opportunity to investigate accurate structures, energies, orbital interactions, and dynamic properties throughout the whole process that may or may not be achieved using conventional experimental techniques, and could even be used to predict experimental phenomena.26–29 In addition, conductor-like screening model for realistic solvation (COSMO-RS) has become rather popular for calculating the thermodynamic properties of pure substances or solutions of mixtures.30,31 The increase in the computational power and the availability of more computational resources has caused an increase in simulation studies of the lignocellulosic system.
After carefully reviewing the literature on this tropic since 2000, we found that there have been many experimental studies related to cellulose, lignin, and hemicellulose. However, most of these studies put an emphasis only on the experimental studies, and the mechanisms from a theoretical viewpoint were less investigated.32,33 Therefore, it is necessary and of great importance to review the progress of the computer modelling and simulation studies on lignocellulose. In this review, the utilization of lignocellulose by dissolution, catalytic conversion, and prevalent pyrolysis was included to offer the theoretical underpinning of the lignocellulosic chemistry. The key topics and methods are presented in Fig. 1. In the following sections, the computational methods, the simulation of interactions between the main components and the mechanisms of dissolution and regeneration, catalytic conversion, and pyrolysis will be discussed. Finally, concluding remarks and developing perspectives on the simulation study of lignocellulosic materials are given. We believe that such a review will provide an understanding of the mechanisms that would promote the development of new technologies to utilize lignocellulosic materials.
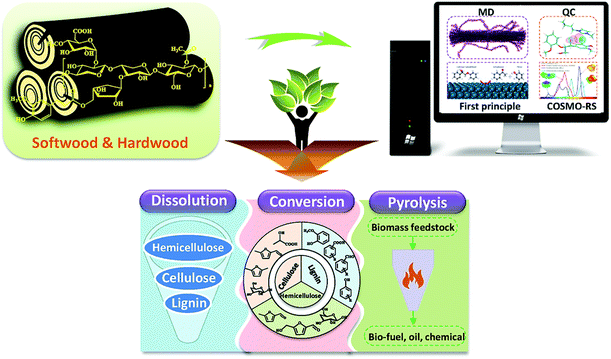 |
| Fig. 1 The dissolution, conversion and pyrolysis processes revealed by computer aided modelling in this review. | |
2. Lignocellulosic materials and their structures
Cellulose, hemicellulose, and lignin constitute the major backbone of the plant cell wall. The proportions of the three components vary in different types of plants (Table 1).34 The hemicellulose and lignin in the cell wall are connected by covalent bonds to form a network structure, in which the cellulose chains are arranged in a highly ordered structure. The complex bundling between cellulose, hemicellulose, and lignin makes these components particularly difficult to separate.
Table 1 Contents of cellulose, hemicellulose, and lignin in common biomass (wt%)34
Types |
Cellulose (%) |
Hemicellulose (%) |
Lignin (%) |
Hardwood |
45–50 |
20–25 |
20–25 |
Softwood |
35–40 |
25–30 |
27–30 |
Wheat straw |
33–40 |
20–25 |
15–20 |
Switch grass |
30–50 |
10–40 |
5–20 |
2.1 Cellulose
Cellulose, as shown in Fig. 2, consists of linear chains with β-1,4 linkages of D-glucopyranose monomers with the molecular formula (C6H10O5)n, where n is the degree of polymerization (DP). Considerable work has been devoted to the detailed structural characterization of such materials. The earliest structural study of cellulose polymers can be traced back to 1920. Hermann Staudinger found that D-glucose polymerizes into long chains, and the top and bottom chains were essentially stacked by hydrogen bonds and van der Waals interactions.35 The structures of cellulose can be divided into three levels: cellulose chains, a supramolecular cellulose layer assembled by crystalline cellulose, and the final fibril layer. The complicated fibril layer is formed by crystalline and amorphous regions, and of course, a variety of pore structures are formed during the cell self-assembly process.36 Since the first X-ray diffraction pattern of bamboo was obtained by Nishikawa,37 more and more scientific researchers have made efforts to reveal the cellulose structure. Nishiyama et al. demonstrated that both Iα and Iβ crystals exist in cellulose structures,38,39 which has made a significant contribution to the further study of cellulose. With in-depth research on cellulose, a variety of crystal morphologies were detected, such as cellulose II.40–42 The dense crystalline structure severely impedes the effective accessibility of enzymes to the cellulose surface,43 which results in the difficulty of degradation of natural cellulose. Therefore, an important step before utilizing cellulose is to reduce its degree of crystallinity by breaking its hydrogen bonds in the crystallization zones. Consequently, cellulose can be hydrolyzed into soluble sugars and further into biofuels (such as bioethanol) or other value-added chemicals.44
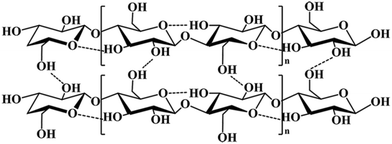 |
| Fig. 2 The inter- and intra-hydrogen bonds of cellulose. | |
2.2 Lignin
Lignin is the most abundant renewable polymer second to cellulose, and the common structure of natural lignin is a complex, non-crystal, and three-dimensional network of phenolic polymers widely found in plants. It is widely accepted that lignin has three primary monomers, which are precursor substances linked by dehydrogenation and radical polymerization.11 The three monomers are p-coumaryl alcohol, coniferyl alcohol, and sinapyl alcohol (Fig. 3), which correspond to the three structural units hydroxyphenyl (H unit), guaiacyl (G unit), and syringyl (S unit), respectively. The content of each unit varies across different plant categories. For example, G units constitute approximately 90% of softwood lignin with a small number of S and H units, while hardwood lignin contains roughly equal proportions of G and S units with a trace amount of H units, and grass lignin contains a mixture of the three units.45,46 As depicted in Fig. 3, the monomers are linked by different types of linkages to form the complex network. The most common linkages in softwood and hardwood are β-O-4, α-O-4, 4-O-5, β-5, β-1, 5–5, and β–β.47 Approximately, up to 75% of the linkages are ether groups (C–O) and 25% of the linkages are carbon–carbon (C–C) bonds.10
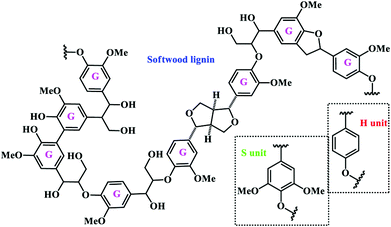 |
| Fig. 3 Representative structure of softwood lignin composed exclusively of G units. The other typical structural units (S and H units) are shown together.47 | |
The lignin structure contains various functional groups, including methoxy, phenolic hydroxyl, aliphatic hydroxyl, carboxyl and carbonyl groups. Among them, the hydroxyl groups can either be free groups or exist in the form of ether bonds coupled with other alkyl and aryl groups. The presence of hydroxyl groups can make lignin form strong inter- and intra-molecular hydrogen bonds. Apart from the hydroxyl groups, methoxy is another typical functional group that is commonly attached to the benzene rings. In all, the existence of functional groups makes lignin highly reactive.10,48 It is also revealed that the oxygen atoms in the methoxy and phenolic hydroxyl groups bear strong electronegativity, and thus a p–π conjugated system with electron-rich benzene rings could be formed.49 Due to the offset of the p electron cloud to the benzene ring, the ortho- and para-sites of benzene rings exhibit significant chemical reactivity, and the addition of electron-donating groups in the ortho- and para-sites can also help to stabilize the intermediate phenoxy radical through resonance stabilization and delocalization.50 Both in softwood and in hardwood, the phenylpropyl groups are linked by β-O-4 bonds in a large proportion of 48–60%. In addition, α-O-4 and 4-O-5 are the other two main linkages with a percentage of 10–15%. The dominant unit in softwood is the guaiacyl group and the fifth carbon in the benzene ring of guaiacyl phenylpropyl is not substituted, resulting in the formation of a 5–5 type linkage with the adjacent phenylpropyl group. On the other hand, hardwood consists of more syringyl groups where the third and fifth carbons of the benzene ring are substituted by methoxy groups, leading to the low probability of forming 5–5 and β-5 type linkages in hardwood. Because of the lack of 5–5 linkage, hardwood tends to have a more linear structure than the multi-branched softwood. Based on the above discussion, destroying various linkages, especially the β-O-4 linkage, will effectively promote the depolymerization of lignin into small aromatic molecules, such as propyl guaiacol, propyl syringol, vanillic or syringic acids, by reductive, oxidative, acid- and base-catalyzed and solvolytic processes.51,52 The platform chemicals from lignin are then reacted in a second step using the existing catalytic technology to produce bulk and fine chemicals.52
2.3 Hemicellulose
Hemicellulose is another abundant polysaccharide in the world, accounting for 20–25% of the total amount of lignocellulose, and it is the main raw material for the preparation of furfural based products.53 Distinct from cellulose, hemicellulose structures do not have a fixed chemical formula; for example, hardwoods mainly contain xyloses while softwoods mainly contain glucosides.54 The backbone of hemicellulose is either a homopolymer or a heteropolymer with short branches linked by β-(1,4)-glycosidic bonds, and occasionally by β-(1,3)-glycosidic bonds. Hemicelluloses have branches with short lateral chains consisting of different monosaccharides. These monosaccharides include pentoses (xylose, rhamnose, and arabinose), hexoses (glucose, mannose, and galactose), and uronic acids (glucuronic and galacturonic acid).55 The most relevant hemicelluloses are xylans and glucomannans and the former is the most abundant component of secondary cell walls of herbaceous plants and hardwood.56 Depending on their biological nature, different types of hemicellulose structures can be found as reported by Gírio et al.54 Here, a typical amorphous structure of hemicellulose substituted by acetate, ferulate, and glucuronate is presented in Fig. 4. In the presence of an acid catalyst, hemicellulose sugars may be broken down into weak acids, furan derivates and phenols.57,58
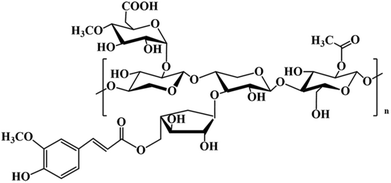 |
| Fig. 4 Typical structure of hemicellulose substituted by acetate, ferulate and glucuronate. | |
2.4 Models
Before introducing the modelling and simulation studies for cellulose, lignin and hemicellulose, the models of lignocellulose studied by molecular simulation and highlights of these simulation studies are summarized in Table 2. The mechanistic studies of the dissolution, conversion and pyrolysis of cellulose, lignin and hemicellulose were highlighted, so that readers can obtain a general understanding of the models and methods before reading specific studies in section 4.
Table 2 Typical studied models and structures
Model types |
Highlights of simulation studies |
Structures |
Ref. |
Cell wall |
Mechanical deformation of the plant cell wall under shear loading |
|
59
|
The mobility of water molecules at the cellulose–xylan and xylan–lignin interfaces |
|
60
|
Hydrophobic and entropy-driven lignin–lignin and lignin–hemicellulose thermodynamic interactions; carbohydrate–π and π–π stacking forces |
|
61
|
Glucuronate strengthening the primary cell wall by coordinating to H-bond donor sites on the cellulose surface |
|
62
|
Core water removal during thermochemical pretreatment is driven by a balance between the entropy and enthalpy of hydration |
|
63
|
|
Cellulose |
Synergistic effect of the cation [Emim]+ and the anion [OAc]− on the cleavage of the cellobiose C–O bond |
|
64
|
Stronger H-bond between [Emim][OAc] and (1,4)-dimethoxy-beta-D-glucose (Glc), as compared to that between Glc–Glc, results in cellulose dissolution |
|
65
|
Conformational changes of cellulose chains in the [Emim][OAc] ionic liquid |
|
66
|
Aggregation of lignin on the surface of cellulose chains in aqueous solution; the distortion of cellulose chains over hydrolysis time |
|
67
|
|
Lignin |
Acid catalyzed cleavage of the β-O-4 linkage of 2-hydroxyethyl phenyl ether in the [Hmim][PF6] solvent model |
|
68
|
H-bonds and π–π stacking effects contribute to lignin dissolution in imidazolium-based ionic liquids |
|
69
|
Hildebrand solubility parameters (δH) of lignin (three monomers) in 1156 ionic liquids |
|
70
|
Mechanism of β-O-4 bond cleavage of 2-phenoxy-1-phenylethanone over the Pd surface by DFT calculations |
|
71
|
Building the special force field parameters for lignin |
|
72
|
Dynamic conformations of lignin polymers under different temperatures |
|
73
|
|
Hemicellulose |
Cluster calculations containing ion pairs of [Bmim][OAc] and xylan. The structure parameters and binding energies affect the solubility of cellulose |
|
74
|
|
Modelling conformational freedom of the φ and ω dihedral angles using MD simulations. The glycosidic linkages affect the conformational flexibility of the backbone in hemicellulose |
|
75
|
|
The formation mechanism of furfural from xylose and the solvent effects on reaction pathways |
|
76
|
|
Pyrolysis mechanism of xylose, O-acetyl xylose and 4-OMeGlcA in hydrogen plasma. The main products, CO, H2, CO2 and C2H2, are identified |
|
77
|
3. Theoretical methods
The general methods, referred to as QC methods, MD simulations, and COSMO-RS, are applied for simulating the lignocellulosic system. Herein, a brief introduction to each method is given in the following subsections.
3.1 Quantum chemistry calculation
Quantum chemistry calculation is an effective method that is widely used to study the molecular structure and to predict the chemical reaction intermediates, reaction barriers, vibrational frequencies, and other thermodynamic parameters via solving the basic Schrödinger equation (eqn (1)).where Ĥ is the Hamiltonian operator, E is the system energy under the steady state, and ψ(x) is the state wave function of the system under the steady state.
The Hartree–Fock (HF) method based on the wave function theory accounts for the kinetic energy of electrons and nuclei and the potential energy of interactions between electron–electron, nuclei–nuclei, and electron–nuclei.78 The density functional theory (DFT) method has become more popular in recent years,79,80 and it has some similarity to the ab initio method in HF theory. However, DFT directly determines the electron density to fully consider the electron correlation effects. At present, the most widely used functionals in DFT include M06-2X,81 hybrid B3LYP,82 PBE0,83 B3PW91,81etc. However, the Generalized Gradient Approximation (GGA)-based DFT functionals have shortcomings in the description of the dispersion forces. To overcome the problem, an empirical dispersion correction is suggested to be added to the Kohn–Sham (KS) energy,84,85 which is referred to as the dispersion corrected DFT functional (DFT-D). After that, the second (D2) and the third (D3) generation corrections proposed by Grimme are shown to cause a significant improvement in the description of noncovalent interactions in a nonpolar moiety or a neutral system.84–86 To consider the behaviour of molecules in different solvent environments, the implicit solvation models, such as the polarizable continuum model (PCM), the solvation model density (SMD),87,88 the reference interaction solvation model (RISM),89 and the 3D-RISM-KH (three-dimensional reference interaction site model with the Kovalenko–Hirata closure approximation) of Kovalenko, were introduced into DFT calculations.90,91 The SMD model of Truhlar et al. has achieved some success in considering both polar and nonpolar parts and is widely used to study chemical reactions,92 predict phase separation,93,94etc. These solvation models are of great scientific and technological importance to predict the chemistry-driven effective interactions and supramolecular cooperative interactions.
3.2 Molecular dynamics simulations
Although DFT accurately describes electron related properties, the scale that it can deal with is limited to 1–10 nm.95 MD simulation is introduced to move from individual molecules to bulk properties of more than thousands of atoms. The trajectory of atoms can be developed using the classical Newtonian equation (eqn (2)). |  | (2) |
where F is the external force on a particle at time t, r is the position of the particle and m is the mass of the particle.
The force field is the basis of the MD simulation, which represents the potential energy of the system. Currently, the four most widely used and most complete all-atom (AA) force fields for biomolecules and biosystems are AMBER (Assisted Model Building with Energy Refinement),96 CHARMM (Chemistry HARvard Macromolecular Mechanics),97 Opls-AA (Optimized Potentials for Liquid Simulations-All Atom),98 and GROMOS (GROningen Molecular Simulation).99 Apart from the AA force field, the UA (United-Atom) and CG (Coarse-Grained) force fields are also widely used because of their abilities to perform large-scale and long-term simulations with limited computational cost.100–102 With the innovation of computer hardware and the development of parallel computing technology, MD simulation is moving toward the mesoscale with a time scale exceeding one millisecond and a spatial scale exceeding 109 atoms.103
3.3 COSMO-RS model
Due to the large quantity of ionic liquids (ILs) synthesized from organic and inorganic cations and anions, it is an enormous challenge to seek the most suitable ILs for biomass dissolution. COSMO-RS is such a model based on statistical thermodynamics for predicting the thermodynamic properties of fluids and liquid mixtures.104,105 It can be applied to process the screening charge density on the surface of molecules to calculate the chemical potential of each species in a solution. The resulting chemical potentials are the basis for the calculation of other thermodynamic equilibrium properties, such as activity coefficients, solubility, partition coefficients, vapor pressure and free energy of solvation. COSMO-RS also integrates the dominant interactions of H-bonds (HB, the most dominant interaction for ILs), misfits (MF), and van der Waals (vdW) forces in IL systems to summarize multiple solvations.106 Therefore, COSMO-RS has been considered to be the most accurate model for predicting the properties of ILs for the currently available tasks.107
COSMO-RS treats a liquid as an ensemble of closely packed and ideally screened molecules. Each piece of the molecular surface is in close contact with another one. It is thus assumed that each molecule is enclosed by a virtual conductor. In COSMO-RS, the σ-profile histogram can be qualitatively divided into three main regions: the H-bond donor region (σ < −0.0082e Å−2), the H-bond acceptor region (σ > +0.0082e Å−2), and the nonpolar region (−0.0082 < σ < +0.0082e Å−2).108 The estimations of excess enthalpy (HE) in the COSMO-RS model arise from summing three contributions associated with interactions, that is, HE = HEHB + HEMF + HEvdW.26 Based on the chemical potential, the activity coefficient can be calculated using eqn (3).109
| 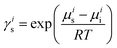 | (3) |
where
μis is the chemical potential of the solvent S, and
μii is the chemical potential of the pure compound
i.
4. Simulation of lignocellulose
4.1 Interactions of lignocellulosic components
Understanding the interactions in the plant cell wall matrix is useful for manipulating the lignocellulose to generate easier deformation of the three components. In this section, the relevant literature is reviewed to focus on cell wall structures and their resistance toward degradation. The deformation mechanism of the cell wall was investigated by MD simulation. Jin et al. reported the mechanical properties of the cell wall material under a shear loading, and it was found that the fibril matrix was sequentially produced and slid along the cellulose surface.59 As indicated in Fig. 5a–c, shear deformation occurs within the lignin matrix and the small deformation in the hemicellulose layer, while the cellulose layers still remain vertical, indicating that the deformation occurs in the lignin layers rather than in the cellulose matrix. In our opinion, it is attributed to the disordered structure of lignin and the well-arranged crystal structure of cellulose. With the breaking and reforming of the H-bond networks at the interfaces, the overall structures become soft and then regain their stiffness, accompanied by the concussion of shear strain. The features allow us to understand the mechanical properties of wood at the molecular level.
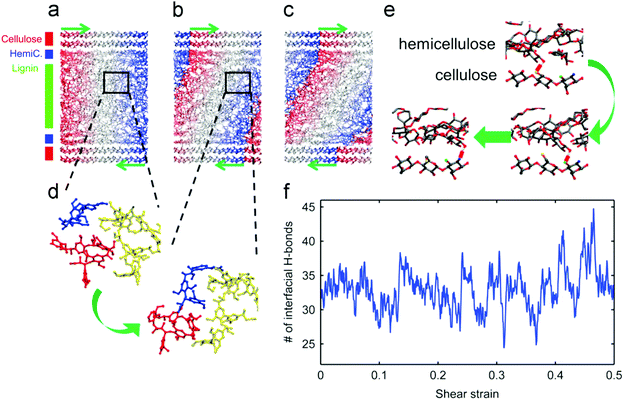 |
| Fig. 5 (a–c) The snapshots taken at the initial state, during the first elastic regime and the second plastic regime, respectively. The color contours correspond to the initial coordinates in the loading direction (indicated by green arrows). (d) Snapshots showing the reorganization of molecules within the matrix after yielding. (e) The dynamic H-bonding (the short red line) between hemicellulose and cellulose. (f) The number of H-bonds at the matrix–cellulose interface with respect to the shear strain on the model.59 | |
Faulon et al. modelled the adsorption of hemicellulose on the elusive (010) surface of cellulose, rather than the dominant (110) and (1−10) surfaces.110,111 The interpretation of the surface and attachment energies of the surfaces has a major influence on the morphology of the cellulose microfibrils. Nevertheless, the lignin structure was not taken into account in their model. Following that work, as shown in Fig. 6, a model representing the secondary plant cell wall was established.60 A combined model that included cellulose chains, lignin models, xylans, and water molecules was simulated to investigate the influence of lignin with different proportions of the basic monomers (S, H, and G units), chain lengths, and linkage types. Despite all these variations, the basic properties resulting from these models do not display apparent diversity. At the cellulose–xylan and xylan–lignin interfaces, xylan tends to penetrate into the lignin structure but there is no interpenetration with the cellulose, which may be due to the faster transport of molecules in lignin than in regular cellulose crystalline. Mazeau studied the dynamic process of adsorption of the β-O-4 lignin dimers on different surfaces of cellulose.36 The van der Waals force was dominant in controlling the association on the (200) surface, and the benzene rings of lignin dimers preferred to be parallel to the glucose rings. The π⋯C–H hydrogen bonding interaction was responsible for the stacking of lignin on the (110), (1−10) and (010) surfaces of cellulose crystals.36 The ordered arrangement was consistent with the experimental detection. The organization of lignin dimers up to the 20-unit oligomer on the cellulose surface was further considered. It has been proved that the branching or some particular inter-molecular linkages were not favourable for ordered arrangement.112
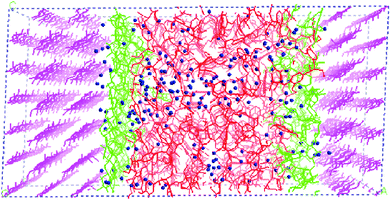 |
| Fig. 6 A model of the secondary plant cell wall. Cellulose is shown in pink, xylan is in green, lignin is in red and water is indicated by blue balls.60 | |
The adsorption or the precipitation of lignin onto the cellulose surfaces hinders the accessibility to cellulose. Not only the structure of lignin but also the crystalline/noncrystalline cellulose affects the preferential association. Petridis et al. found that the crystalline cellulose has a higher tendency to absorb lignin than the noncrystalline regions because of the stronger hydrophilic character of the noncrystalline regions to water.63,67 Therefore, the digestibility and accessibility of cellulose can be increased by maximizing the hydration effects. Moreover, in the processing of lignocellulose (e.g. bioethanol production, enzymatic treatments, pulp and paper processes, etc.), lignin binding with the hydrophobic surfaces of cellulose should be avoided because these hydrophobic sites are expected to associate easily with cellulases.113 After all, cell wall recalcitrance depends on the chemical composition of the lignocellulose matrix. Changing the hemicellulose composition (proportions and branches) can significantly alter the strength and the microstructure of the plant cell wall. The interaction between cellulose nanocrystallites (CN) and hemicellulose hydrogels was revealed by 3D-RISM-KH theory of solvation,62 showing that the cell wall strength was enhanced by the hydrogen bonding interactions between the glucuronate-branched hemicellulose and CN. It was also shown that hemicellulose was localized around the first layer of cellulose with hydrogen bonds and distributed at the second layer by stacking effects.61
4.2 Mechanism of dissolution and regeneration
Cellulose.
As early as 1993, French et al. successfully simulated microcrystalline cellulose containing 24–32 monosaccharide sugar units by computer modelling.114 Since then, computer simulation of the cellulose crystal structure, its solubility, and chemical reactions involving cellulose have developed rapidly.
After the detection of the lactic parameters of cellulose by Nishiyama,115 Matthews successfully simulated the microcrystalline structure of cellulose Iβ and found that the water shell was the most important factor affecting the enzymatic hydrolysis of cellulose crystals.116 Yui et al. reported that cellulose Iα had a higher degree of folding than cellulose Iβ.117 Heiner et al. found that cellulose monoclinic crystals had higher stability than triclinic crystals by MD simulations.118 Bergenstrahle et al. simulated the dissolution behaviour of cellulose crystals in an aqueous solution.119 All these results show that the aliphatic proton in the cellulose crystal structure tends to be in the axial position perpendicular to the glucose ring. The accumulation of hydrophobic groups and the presence of hydrogen bonds in the cellulose chain all inhibit the dissolution of cellulose crystals. To validate the dissolution mechanisms of cellulose in different solvents, Chu et al. simulated the dissolution process of cellulose in four kinds of solvents, i.e., lithium chloride/N,N-dimethylacetamide (LiCl/DMA), water, LiCl/water, and DMA.120 It was found that LiCl/DMA has the best solubility for glucan chains, where the potential of the mean force decreased with the progress of deconstruction. Due to the low solubilities of glucans and LiCl in DMA, the [Li]+ cation and the [Cl]− anion can effectively interact with glucans, thereby deconstructing the intermolecular interactions. The small size of the cation allowed for strong and preferential interactions with cellulose, which were considered as the main driving force responsible for cellulose dissolution. The intermolecular interactions, such as hydrogen bonds, van der Waals interactions, and repulsions, have been identified as the driving forces for dissolving chitobiose in ILs.121
The cellulose solubilization in other solvents was also studied in aqueous sodium hydroxide,122 urea,123 supercritical water,124 and ILs.28,125,126 Miyamoto put forward the dissolution mechanism of cellulose in an aqueous sodium hydroxide solution,122 and it was estimated that water molecules and alkali species permeated into the cellulose crystal from the interlayer space of the (1−10) surface, and the hydrophobic sheets were dissociated into mini crystals and then into separate chains. During the dissolution, the density of sodium ions accumulated around O2 and O3 was two times higher than that in the bulk phase, resulting in approximately 8 wt% solubility of cellulose in the aqueous sodium hydroxide solution. Wernersson found that urea dissolved cellulose by preferentially absorbing on the hydrophobic surfaces of hydroglucose rings and gradually weakening the hydrophobic effect of cellulose chains.123 In Fig. 7, water oxygen was distributed near the hydroxyl groups of the glucose ring, while urea oxygen was mostly located above the glucose rings, and the absorption was accommodated by the interaction between hydroxyl groups of the glucose and urea nitrogen, which was in line with the affinity of water to the hydroxyl group of the glucose ring. It was concluded that these locations resulted in the preferential solvation of cellulose in urea.123 While the treatment of lignocellulose in liquid hot water (LHW) or supercritical water (SCW) is considered to be a green process, the micro-interaction between lignocellulose and water at high temperature presents a practical and scientific challenge.127–129 Wohlert et al. reported the solubility of cellulose in supercritical water over a range of densities by MD simulations.124 At low density, chain dissociation induced the augmentation of water around these dissociated chains, and the partial water molecules were immobilized in the solvation layer. At the same time, new water–cellulose hydrogen bonds were formed. The results revealed that cellulose dissociation was thermodynamically favored in low-density SCW and it was counterbalanced by the entropy gain of cellulose chains and the entropy loss of water.124
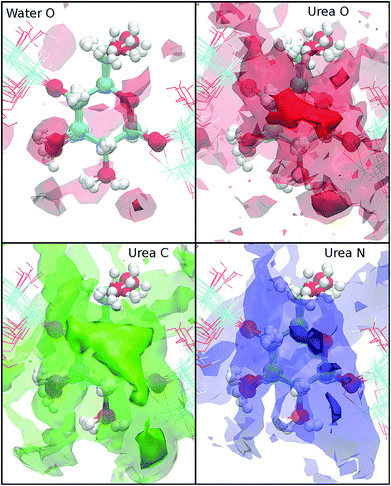 |
| Fig. 7 Isodensity surfaces of g2j(r) for isovalues 3 (transparent surfaces) and 9 (opaque surfaces) for the atom types indicated. The cellulose position is taken as the center of geometry of an arbitrary anhydroglucose unit. The conformations of the cellulose molecules for ten randomly selected configurations are shown in order to give an impression of the conformational flexibility.123 | |
More recently, environment friendly ILs were applied as a green route for dissolving lignocellulose materials.16,44,130 Gross et al. studied the hydrolysis of cellulose in 1-butyl-3-methylimidazolium chloride ([Bmim][Cl]) by MD simulations, and the results showed that the influence of dissolved glucose on the structure of the used solvent is the key factor affecting the solubility of cellulose.131 Both [Bmim]+ cations and [Cl]− anions interacted with the sugar residues. The anion [Cl]− tended to form hydrogen bonds with glucose, while the cation [Bmim]+ was axially closer to the cellulose chains. After that, Wang et al. studied the effect of anionic structures on the dissolution of cellulose in ILs with various kinds of anions.132 It was estimated that anions played a key role in forming hydrogen bonds with hydroxyl protons of cellulose, and the interaction ability of several anions with cellulose decreased in the sequence: [Cl]− > [OAc]− > [(MeO)2PO2]− > [SCN]− > [PF6]−. These results indicated that electron-rich and electronegative hydrogen bond acceptors, anions with a few or no electron-withdrawing groups and shorter alkyl chain cations were expected to be efficient IL solvents for cellulose dissolution. However, the role of cations was still unclear in the dissolution of cellulose. Lu et al. then investigated 13 kinds of ILs with the [OAc]− anion,133 and they found that ILs containing cations without large steric hindrance or with acidic protons were reasonable solvents. The above studies have shown that cations of ILs are found to affect the cellulose solubility, and recent research has proved that cations with unsaturated aromatic rings are more favourable for dissolving cellulose.44,134 Li et al. further revealed the microscopic dissolution process of cellulose bundles in ILs with saturated and unsaturated heterocyclic rings.28 In their results, cellulose bundles remained almost unchanged in saturated 1-butyl-1-methylpyrrolidinium acetate ([Bpyr][OAc]) and 1-butyl-1-methylpiperidinium acetate ([Bpip][OAc]), but the chains separated effectively in unsaturated 1-butyl-3-methylimidazolium acetate ([Bmim][OAc]) and 1-butylpyridinium acetate ([Bpy][OAc]). This phenomenon was attributed to the stronger π-stacking interaction of unsaturated ILs with glucose rings, and the unsaturated ILs also bore smaller steric hindrance, which can be verified by the shorter distances between the glucose and unsaturated cation rings (Fig. 8). Moreover, ILs consisting of unsaturated cations bore smaller viscosities, which remarkably improved the mass diffusion of anions to dissociate cellulose chains.28 Although many studies have shown that ILs were able to dissolve a considerable amount of natural cellulose, these studies focused only on the dissolution of cellulose in pure IL. How does a cosolvent or an anti-solvent behave in cellulose–IL systems? MD simulations were performed for systems containing a cosolvent, e.g., dimethyl sulfoxide (DMSO), and an anti-solvent, e.g., water.135–137 DMSO can decrease the viscosity and facilitate the mass transport of the system, while it did not significantly affect either the structure of ion pairs or the hydrogen bonding interactions between ion pairs and cellulose. On the other hand, water molecules were probably closer to glucose rings and greatly interfered with cellulose–ion pair interactions. Consequently, water was a more proper choice as an anti-solvent for the regeneration of the cellulose component from solutions.65,138 MD simulations further demonstrated that the addition of molecular solvents can significantly lower the viscosity of solutions and lead to better mass and heat transport and thus lower costs and better performance of the IL/co-solvent.66,139
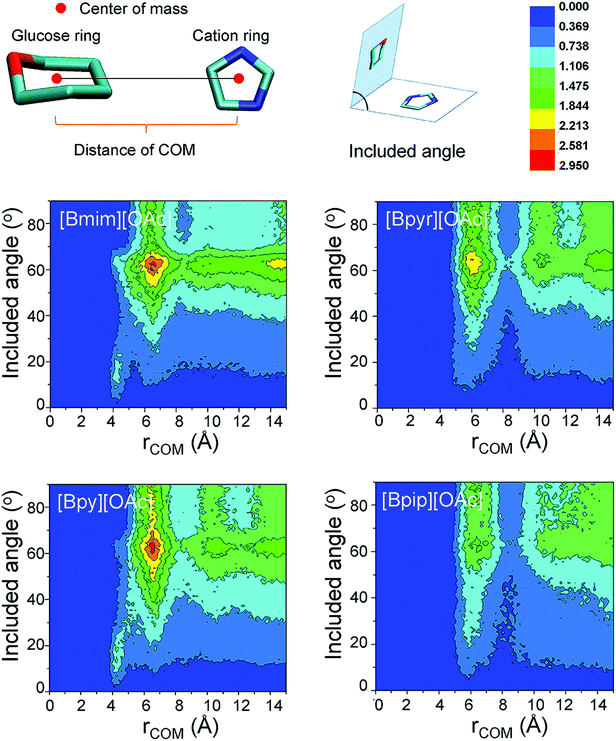 |
| Fig. 8 Colors from dark blue to bright red indicate the probability distribution as shown in the middle. The x-axis represents the distance of the center of mass (COM) and the y-axis represents the angle of the two rings.28 | |
Since ILs are considered as green solvents for biomass dissolution, many excellent research studies on dissolving lignocellulosic materials in ILs have emerged.15,140–142 It is of great importance to investigate the thermodynamic parameters, such as solubility, Henry's law constant, and activity coefficient, of various solutions with or without relying on the experimental data.143–145 Moreover, the parameters are essential for both laboratory studies and pilot plant scale-up. Therefore, the dissolution models can be established by COSMO-RS as described below. Fig. 9 demonstrates the three possible representations of a 1
:
1 IL in COSMO-RS.109 For a meta-file approach, IL is treated as the sum of the σ-profiles, areas, and volumes of the ions. For an ion-pair approach, the ion-pair structure is optimized at the COSMO level, which usually requires a large set of conformations of IL ions because of their weak coordination abilities. For electroneutral mixtures, the molar fractions of the cations and anions of ILs are treated as equal, i.e. ncation = nanion = nIL.146 For an IL and solute mixture with a 1
:
1 ratio (cation + anion + solute), the mole fraction used in experiments depends on the binary system, whereas the mole fraction used in the COSMO-RS calculation depends on the ternary system. Therefore, a parameter such as the activity coefficient of a solute X in the ternary mixture (γternX) of COSMO-RS calculation has to be converted into a binary definition (γbinX). The relationship for the conversion is γbinX = γternX(xternX + xternion), where xternX is the mole fraction of the solute, and xternion is the mole fraction of the ion (=xternanion = xterncation). According to this formula, the activity coefficient at infinite dilution of the solute X is γbinX = 0.5γternX (xternX → 0, xternion → 0.5).109
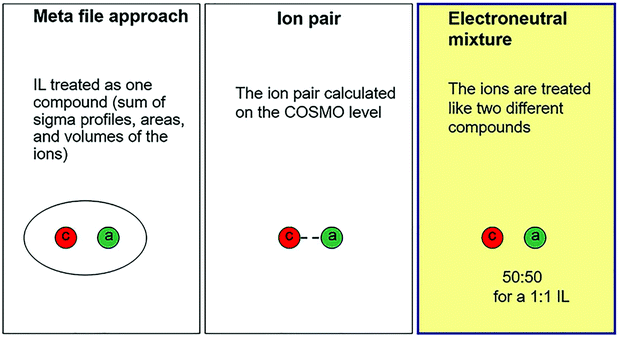 |
| Fig. 9 Three possible representations of a 1 : 1 ionic liquid in COSMO-RS.109 | |
Moreover, activity coefficients are also useful for predicting cellulose solubility in ILs.147 Kahlen et al. applied a mid-monomer glucose unit, cellotriose COSMO file, and predicted the residual contributions to the activity coefficients of cellulose in 2272 different ILs with 32 anions and 71 cations at infinite dilution (Fig. 10).30 The cellotriose cellulose model was first selected from 30 relevant conformers with the lowest energy of 47 kJ mol−1. Then both ends of cellotriose were “cut” to acquire the mid-monomer glucose unit to form the basis of our cellulose model. According to the predicted ln
γres, ILs with high cellulose dissolution capacities were located on the left, while ILs with lower cellulose dissolution capacities were located on the right (Fig. 10). The ln
γres prediction showed that the predicted dissolving power was mostly anion dependent, and cations had a moderate effect on the solubility of cellulose. Kahlen et al. indicated that anions, such as acetate, decanoate, chloride, bromide, and iodide, were predicted to dissolve cellulose very well in their study. The prediction results were finally compared with the cellulose solubilities measured by Vitz et al.,148 and they were in good agreement.
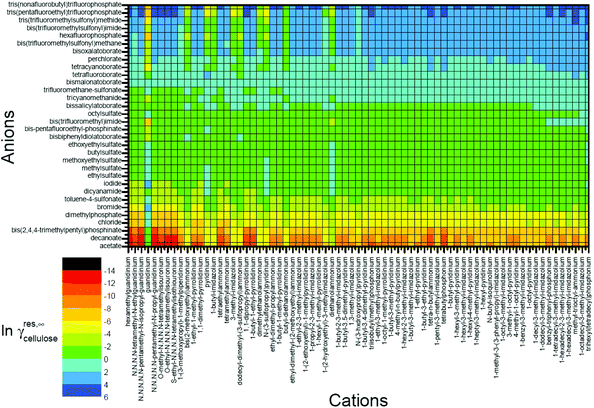 |
| Fig. 10 Residual contributions to the activity coefficients of cellulose in 2272 different ionic liquids at infinite dilution.30 | |
Casas et al. used glucose and pinoresinol to represent cellulose and lignin, respectively.31 The purpose of their work was to study the interactions between IL and lignin/cellulose molecular models by COSMO-RS predictions, and then to obtain the dissolution mechanisms of cellulose and lignin in IL systems. The studied compounds were optimized at the B3LYP/6-31++G(d,p) computational level. Then, the BVP86/TZVP/DGA1 level of theory was used to acquire the COSMO file. The activity and excess enthalpy results evidenced that these two results had similar tendencies, i.e., low activity coefficient values related to low excess enthalpy values (meaning high cellulose solubility), which illustrated that excess enthalpy could also be used for predicting the lignin and cellulose solubility in ILs by COSMO-RS. Apart from the result that anions and cations, respectively, acted in the main and secondary roles in the cellulose dissolution process,31 Casas et al. also showed that hydrogen bonding interactions between IL and cellulose or lignin were the main forces for cellulose or lignin dissolution.
Casas et al.146 subsequently designed a series of 3 × 3 cellulose model structures consisting of nine differently cut monomers, M1 to M9, and 5 lignin structures to be used by COSMO-RS to represent cellulose and lignin. Based on the calculated activity coefficients, excess enthalpies and the experimental results for 12 ILs, the M4 model and p-coumaryl alcohol were finally selected as the best cellulose and lignin models, respectively. Three solubility regions for cellulose and lignin in ILs could be described from the COSMO-RS prediction: (1) when γcellulose and γlignin < 0.85, cellulose and lignin are soluble in IL. (2) When γcellulose ≥ 0.85 and γlignin < 0.97, cellulose is insoluble and lignin is soluble. (3) When γcellulose and γlignin > 0.97, both cellulose and lignin are insoluble. Balaji et al.70 applied the three lignin models of p-coumaryl alcohol, sinapyl alcohol, and coniferyl alcohol to study the lignin solubility from lignocellulose biomass in 1156 ILs by COSMO-RS prediction. The infinite dilution activity coefficient was first predicted by COSMO-RS, and then the predicted infinite dilution activity coefficient was used to calculate the Hildebrand solubility parameter to predict the lignin solubility. The result evidenced that larger cation size was conducive to dissolve lignin due to its larger van der Waals area since the solubility parameters decreased in the order: [13Mmim]+ > [13Emim]+ > [13Hmim]+ > [13BNmim]+ > [13Omim]+, and the parameters of [13Omim]+ were closer to lignin. Five groups of ILs based on the cations of imidazolium, pyridinium, pyrrolidinium, ammonium, and phosphonium with almost all anions ([OAc]−, [B(CN)4]−, [BF4]−, [DBP]−, [HSO4]−, [MeSO4]−, [SCN]−, etc.) had a high solubility for lignin. This is attributed to the fact that their Hildebrand solubility parameter values were basically equal to that of lignin (28.0) at 303.15 K. Liu et al. screened 375 IL candidates by COSMO-RS for three cellulose models, which are glucose (model 1), the mid-monomer part of cellotriose (model 2), and the mid-dimer part of cellotetraose (model 3) (Fig. 11).149 The addressed cellulose models were compared by COSMO-RS, where the mid-monomer in cellotriose was identified as the best model. Furthermore, enthalpy calculations of cellulose dissolution in 7 relevantly selected ILs were accomplished, and the cellulose dissolution was experimentally verified. COSMO-RS excess enthalpy predictions indicated that the cellulose dissolution process was mostly anion dependent and the main forces in the cellulose dissolution in ILs were hydrogen bonds; it was suggested that [OAc]−, [Dec]−, [HCOO]−, [Cl]−, [BEN]−, [DMPO4]−, [DEP]−, [DBP]−, and [Br]− were the most promising anions for cellulose dissolution. Cations with ethyl, allyl, 2-hydroxyethyl, 2-methoxyethyl and acryl-hydroxypropyl functional groups exhibited particularly good properties for cellulose dissolution. The successful application of MD simulations and the COSMO-RS method is a far from trivial task. It is found that cellulose dissolution is accompanied by the cleavage of cellulose chains and a decrease in molecular weight. Therefore, special attention must be paid to choosing an accurate and efficient quantum chemical approach to study the mechanism of cellulose dissolution. In the field of quantum chemistry calculations, current research mainly focuses on a single anion and cation, an ion pair of ILs and glucose, cellulose oligomers (DP = 6–10),150,151 and the interaction of cellobiose and xylans with an implicit solvation model.74 It was believed that anions played an important role due to the formation of hydrogen bonds between anions and hydroxyl groups of cellulose chains, which broke down the inter- and intramolecular hydrogen bonds of cellulose. Afterwards, Du et al. calculated the ring-opening reaction of glucose in the presence of 1-ethyl-3-methylimidazolium acetate ([Emim][OAc]).152 It was estimated that the C2 proton of [Emim]+ was transferred to [OAc]−, and then acetic acid and carbine were formed. The carbine would bind to the terminal carbon of the glucose ring while the proton will be transferred to the terminal oxygen, leading to the cleavage of C–O bonds of glucose rings. Du and Wei demonstrated that the cleavage of C–O bonds of glucose rings was the critical step,152,153 and both the anion and the cation were responsible for cellulose dissolution.64
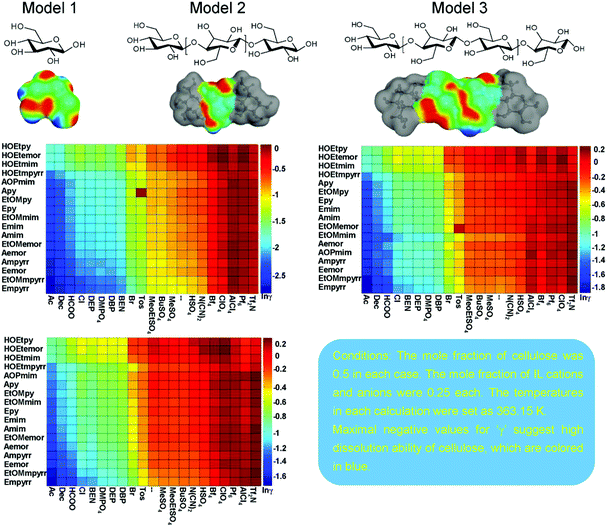 |
| Fig. 11 The designed cellulose models 1–3 and the logarithmic activity coefficient prediction results of models 1–3 in 357 ionic liquids by COSMO-RS.149 | |
In addition, the dissolution or hydrolysis of cellulose was also investigated in mineral acids, Lewis acids, quaternary ammonium perrhenates, transition metal-based ILs, and functional ILs (e.g. ILs containing –COOH and –SO3H groups).154–157 For instance, Loerbroks et al. examined the acid-catalyzed hydrolysis of cellobiose.158 In the presence of hydroxyl groups or intramolecular hydrogen bonds, the basicity of the glycosidic O changed accordingly. In this context, the activation of glycosidic or terminal C–O bonds depended not only on the protonation of oxygen atoms of glycosidic bonds, but also on the conformational changes. To better describe these structures, proper DFT approaches should be adapted in consideration of weak interactions, especially the long-range dispersion effects.84,85,159 Although the above studies have shown that molecular understanding of the chemical structure of cellulose would provide new insights for designing new dissolution approaches and catalysts, developing new methods for the hydrolysis of cellulose is still of great importance for further transformation of cellulose into value-added chemicals.
Lignin.
Lignin is the most abundant natural source of phenolic compounds. It is critically important to separate lignin from other lignocellulosic materials for efficient valorization. However, the complicated three-dimensional network of lignin leads to insolubility of lignin in most common solvents. Some processes, including acidolysis, IL treatment, photochemical degradation, liquid hot water, base-catalyzed hydrolysis and enzyme hydrolysis, have shown advantages in treating lignin.10 Ji et al. investigated the hydrogen bonding interaction in the [Amim][Cl]–LigOH and LigOH–LigOH systems, and found that the stronger hydrogen bonding interaction between [Amim][Cl]–LigOH would replace that between LigOH–LigOH. This process was responsible for lignin dissolution in [Amim][Cl]. At the same time, addition of water to the solutions reduced the hydrogen bonds in [Amim][Cl]–LigOH, leading to the regeneration of lignin from [Amim][Cl].160 Janesko et al. performed a comprehensive study to elucidate the noncovalent interactions between various ILs and cellulose/lignin. They highlighted the importance of IL anions in dissolving cellulose and IL cations in tuning the relative solubilities of lignin. Furthermore, they found that ILs with π-conjugated cations are favourable for enhancing lignin solubility.161 Zhang et al. computed the interactions between different ILs containing a series of cations and anions with the β-O-4 model compound of lignin.69 Their DFT calculations (Fig. 12) indicated that π–π stacking between imidazolium-based cations and aromatic rings is an important factor affecting lignin dissolution, and anions still play a key role in providing hydrogen bonding interactions. These findings have later been confirmed by Wang's work.162 However, much stronger π-stacking interaction or H-bonds will make the dissociation between lignin and ILs difficult, and thus the strength of these interactions should be balanced when choosing proper ILs. Additionally, the acidity, viscosity, and steric effects of ILs also need to be considered in these processes. Zhang et al. investigated the pretreatment mechanism of β-O-4 lignin using DFT and MD simulations.163 The simulation results showed that the adsorption, solubility, and decomposition of lignin in the phosphoric acid–acetone system were mainly due to the interactions between lignin and the species of solvent molecules, i.e., H2O, CH3COCH3 and H3PO4. β-O-4 linkage played a very important role in charge transport, and thus lignin dissolved in acetone at about 273.15 K and flocculated on adding the anti-solvent water.163 Mar et al. also found that the first cleavage of β-O-4 linkage caused the subsequent cleavage of aromatic C–C bonds by destabilizing adjacent bonds.164 Recently, a special molecular force field developed by Petridis et al. enabled the full simulation of lignocellulosic materials.72 They established a softwood lignin model and evaluated the behaviour of structures and dynamics as a function of temperature using the CHARMM force field.73 Nine lignin models with zero to six branch points were simulated at four different temperatures: 300, 360, 420, and 480 K. As a result, the state of lignin transitioned from the compact conformations with glassy dynamics to the extended conformations with enhanced dynamics with the increase of simulated temperature. Fig. 13 shows that lignin models with chain length between 6 and 41 collapse both at ambient (300 K) and high temperatures (500 K). Described by the parameter gyration, Rg, nonpolar lignin has a lower Rg value than that of polar wild-type lignin, indicating that nonpolar lignin reduced its electrostatic interactions with water; thus it was kept stable at a smaller degree of polymerization. However, for the wild-type lignin, the shape was aspherical for short chains due to the interactions between water and hydroxyl groups, and the sphere conformation was favoured by monomer–monomer interactions.165 The accuracy of this force field was further validated by a series of simulation studies.63,67,73,113
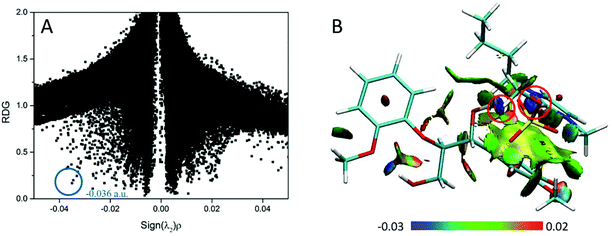 |
| Fig. 12 (A) RDG scatter plots (isovalue = 0.5 a.u.) and (B) isosurfaces (s = 0.7 a.u.) of BmimMeSO4–GG conformer. The isosurfaces are colored on a blue-green-red scale according to the values of sign(λ2)ρ, ranging from −0.03 to 0.02 a.u. Blue indicates strong attractive interactions and green indicates the π-stacking interaction.69 | |
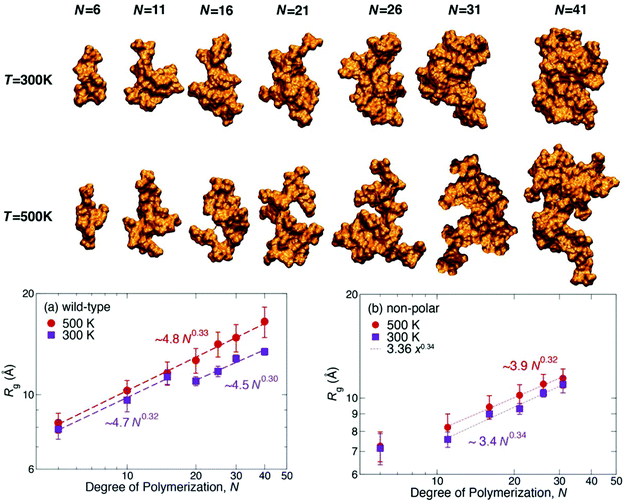 |
| Fig. 13 Representative structures of the seven lignin polymers of degree of polymerization N at two temperatures. To help visualize asphericity, the first and second principal axes of the molecules lie in the plane of the paper. Structures were selected to have Rg and asphericity consistent with the bottom figures: (a) wild-type and (b) nonpolar models at 300 and 500 K, respectively. Each data point is the ensemble average of the last 100 ns of three independent trajectories. The dashed lines are power-law fits to the data.165 | |
Hemicellulose.
The dissolution of hemicellulose is not yet fully understood. Payal et al. calculated the dissolution mechanisms of hemicellulose and cellulose in solvents, including the IL 1,3-dimethylimidazolium acetate, water, and methanol.74 It was found that the binding energy between xylan and IL was 20 kcal mol−1 stronger than that between cellobiose and IL, and the rotation of pentose rings led to the amorphous nature of lignin. All of these factors made the solubility of hemicellulose higher than that of cellulose.74 Furthermore, the effect of glycosidic linkage types in hemicellulose was investigated by MD simulations. Berglund et al. concluded that type X (linkage between two xylan units) in xylan resulted in the most flexible hemicellulose backbones, type C (linkage between glucose–glucose or glucose–mannose) in xyloglucan and glucomannan was expected to be the stiffest with syn conformation, and type M (linkage between mannose–mannose or mannose–glucose) in glucomannan was similar to type C with an increased population of non-exo-anomeric conformation.75 The information gained contributes to an increased understanding of the function of hemicellulose both in the cell wall and in technical products.
4.3 Mechanism of catalytic conversion
Cellulose.
The dissolution and hydrolysis of cellulose into reduced molecular-weight sugars is just a beginning, but selectively transforming cellulose to targeted chemicals and products makes more sense. Li et al. explained the conversion of cellulose to glucose in a SO3H-functionalized IL [C4SO3Hmim][Cl].166 The SO3H group served as the proton donor to protonate the glycosidic bond and [Cl]− attacked the anomeric carbon, leading to the cleavage of glycosidic bonds. At the same time, adding new ion pairs to the reaction did not change the reaction mechanism but lowered the reaction barriers.166,167 It was identified that 1,2-enediol (IM3 in Fig. 14) is a necessary intermediate, followed by dehydration to HMF, and another pathway experiencing the fructose intermediate to produce HMF also showed a very low energy barrier. Importantly, [C3SO3Hmim][Cl] could act as both a proton donor (a Brønsted acid) and a proton acceptor (a conjugate base).167 As is known, glucose and fructose form a pair of isomers. The food, pharmaceutical, and healthcare fields have a great demand for products such as fructose. Recent studies have proposed a mechanism for glucose isomerization into fructose over Sn-BEA zeolite.168,169 The DFT calculations demonstrated that the main role of Sn-BEA was to initiate the isomerization of acyclic glucose into a deprotonated fructose intermediate via the deprotonation of O2 and then the H-shift from C2 to C1.168 Through DFT calculations, Riisager's group found that the formation of glucose–borate complexes could promote the conversion of glucose to fructose.170 Zhang et al. developed the conversion pathways of β-D-glucose in supercritical water, and the main outcomes, levoglucosan, hydroxylacetaldehyde, 5-hydroxymethylfurfural, glyceraldehyde, fructose, and erythrose, were identified. It was believed that water molecules served protons in dehydration, and the keto–enol rearrangement; thus the reaction barrier was dramatically decreased.171 In recent years, production of 5-hydroxymethylfurfural (5-HMF), lactic acid, levulinic acid, etc. has attracted much attention and a lot of studies dealing with the conversion of cellulose have been published.172–175 Starting from these platform chemicals, a wide range of value-added products can be derived. However, the selective transformation of cellulose into these platform chemicals has remained the major challenge. Parveen et al. proposed that the conversion of glucose to HMF is a two-step reaction: (1) glucose isomerization to fructose under basic conditions and (2) fructose dehydration to HMF under acidic conditions.176 This kind of oganocatalyst (sulfanilic acid) with dual basic and acidic properties is a promising multifunctional catalyst for the production of HMF. As a result, low exothermic reaction energies of 3.7 kcal mol−1 and 33.1 kcal mol−1 made steps (1) and (2) thermodynamically prone to occur. Wang's group explored the mechanism of the reaction of cellulose to lactic acid.177 The authors confirmed that the reaction proceeded according to the following steps: (1) hydrolysis of cellulose into glucose, (2) isomerization of glucose into fructose, (3) generation of a three-carbon sugar by aldol condensation of C3–C4 of fructose, and (4) isomerization of the three-carbon sugar into lactic acid. Their theoretical results further revealed the obvious effect of Pb(II) catalysts in reducing the activation energy of aldol condensation reaction.177 Therefore, this process has a higher selectivity to lactic acid than to HMF, and these results are of great significance for guiding the development of new processes for the preparation of chemicals or liquid fuels. Another important chemical, levulinic acid, can be obtained through the hydrolysis of cellulose. The conversion of cellulose to levulinic acid includes three steps: (1) cellulose to glucose, (2) glucose to HMF, and (3) hydration of HMF to levulinic acid.174 Cellulose can be selectively converted to levulinic acid in SO3H-functionalized ILs. The catalyzed conversion of 5-HMF to levulinic acid in the presence of [C3SO3Hmim][HSO4] IL was studied by Li et al.178 Their results showed that the acidity and nucleophilicity of the catalyst are the key factors affecting the catalytic performance. The overall reaction barrier of 34.7 kcal mol−1 was beneficial to the reaction process under mild conditions. In some cases, HMF and levulinic acid formed the aldol condensation product. Zhao et al. identified the condensation reaction using DFT calculations and found that a linear product formed between C5 and levulinic acid was favourable through the following overall steps: (1) deprotonation of levulinic acid, (2) addition of levulinate to HMF, and (3) final dehydration to form a condensation product.179 Huang et al. reported the highly efficient conversion of cellulose to methyl levulinate by the simple metal salt Al2(SO4)3 assisted by microwave radiation, in which the Lewis acid species [Al(OH)x(H2O)y]n+ and the Brønsted acid species [H]+ generated by hydrolysis of Al2(SO4)3 were thought to be responsible for the conversion. The reaction mechanism is instructive for regioselectivity designing conversion chemistry. However, the reaction mechanism is not verified by theoretical calculation.180
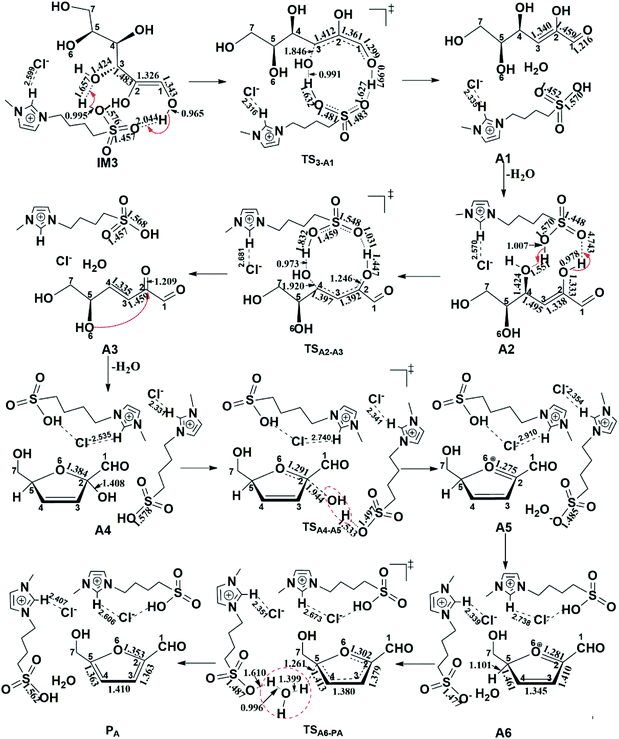 |
| Fig. 14 Mechanism details for the transformation of 1,2-enediol into HMF.167 | |
Lignin.
Due to the complicated chemical linkages of lignin and its cross-linkages with other components, determining the depolymerization mechanism of lignin is essential for the downstream processing of lignin into valuable aromatic monomers. In this case, simulation results could provide important insights into the chemical bonding in lignin or its model compounds. Acid-catalyzed processing is considered highly efficient at degrading lignin.32,181,182 Sturgeon et al. investigated the cleavage of the β-O-4 aryl-ether bond in consideration of the functionality's effect.183 It was proved that the presence of a phenolic or aliphatic hydroxyl group greatly accelerated the bond cleavage reaction. Additionally, the presence of methoxy groups could protect the carbocation intermediate from rapid self-condensation. Followed by Rahimi's experimental study,182 Qu et al. elucidated the depolymerization of lignin catalyzed by formic acid,184 and their results (Fig. 15) showed that the reaction proceeded following formylation, elimination and hydrolysis via 3c–4e bonds, which had a lower barrier than the known E1 and E2 elimination cases. Qin et al. studied the stability of common linkages of lignin in H3PO4, CH3COCH3, and H2O, and confirmed that β-O-4 and α-O-4 bonds were the most vulnerable to damage in the acid pretreatment steps, where the α-O-4 bond was more energetically accessible for phosphorylation.185 During acid hydrolysis, the carbocation intermediate was identified by Janesko's work.68 Their results indicated that the β-O-4 model compound, α-hydroxy-phenethyl phenyl ether, underwent E1 dehydration. The acidic IL 1-H-3-methylimidazolium chloride with AlCl3 exhibited higher catalytic activity. It seems that ILs in the presence of metals were efficient at lignin depolymerization. The catalytic reaction of lignin with noble metals was investigated. In the presence of metal catalysts Ni/SiO2 and Ni/HZSM-5 in undecane, almost complete radical reactions occurred, and the primary benzene and phenol products were generated from benzyl phenyl ether because the metals could provide dissociated hydrogen atoms.186 Wilson et al. reported the oxidative addition of the Cα–Cβ bond of the archetypal acylglycerol β-aryl ether to transition metals Ni, Cu, and Pd, and the activation of the Cα–Cβ bond was thermodynamically favourable with a low activation barrier.187 Beyond that, the aromatic chemistry on metallic surfaces was involved in many processes within the biomass valorization. Sautet et al. elucidated the stability of aromatic compounds with alkyl and alkoxy (or hydroxyl) groups on the Pt(III) surface, which was essential to understand their properties, such as the following: the van der Waals interaction was controlled by molecular weight and the deformation energy could be correlated to the position of the substitute.188 Another bond cleavage mechanism of the C–O ether bond in the lignin model 2-phenoxy-1-phenyl ethanol on the Pd surfaces was comprehensively studied by first-principles calculations,71 and the results are shown in Fig. 16. It was revealed that β-O-4 ether bond cleavage happened after the dehydrogenation of the reactant on α-C and then the hydroxyl group was dehydrogenated over Pd catalysts. It was also emphasized that keto–enol isomerization and the hydrogen atoms on α-C and β-C were vital for the cleavage of ether bonds. In the same year, Gu et al. reported the hydrodeoxygenation (HDO) of phenols on Pd catalysts, which proved that a single metal was sufficient to initiate the reaction.189 Partial hydrogenation rather than full hydrogenation is necessary to activate phenol rings. Recently, Ni-based catalysts have been reported as efficient catalysts for lignin depolymerization. To predict the depolymerization mechanisms, Li et al. performed first-principles calculations on the cleavage of β-O-4 bonds on Ni(III) surfaces. Their results (Fig. 17) showed that Ru doped Ni(III) surfaces provided preferential points for β-O-4 bonds because a small amount of Ru weakened the C–O bonds by increasing the metal–O strength.190 With the help of Rh/C supported catalysts, Yamaguchi et al. achieved the efficient cleavage of lignin model compounds into various aromatic monomers in supercritical water, while the mechanism should be much more complicated for so many products.191 Their results also provided insights into the exploitation of less noble metal catalysts, such as Ru, Rh, and Ni.192
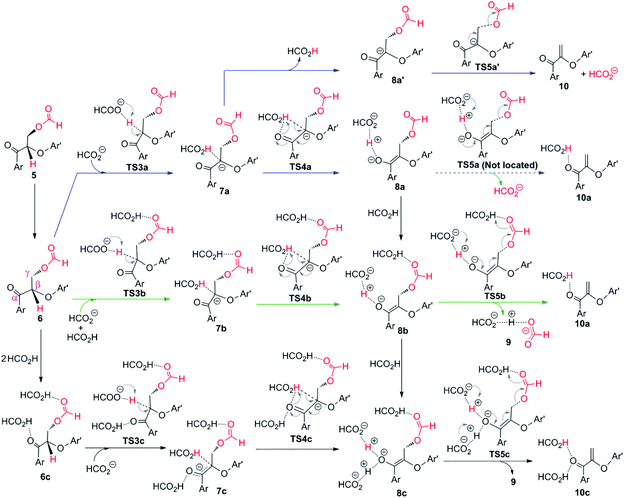 |
| Fig. 15 Possible pathways explored for the elimination stage by formic acid.184 | |
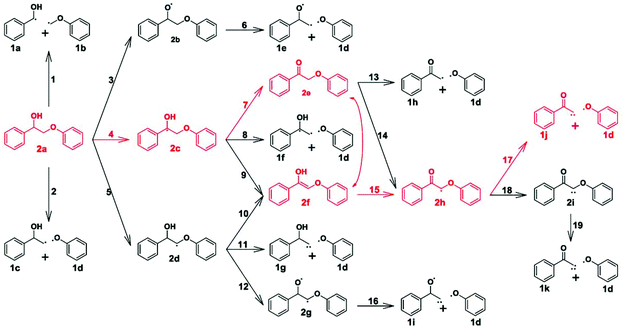 |
| Fig. 16 Reaction network for the β-O-4 bond cleavage of 2-phenoxy-1-phenylethanol over Pd(111). The predicted dominant reaction pathway is colored in red.71 | |
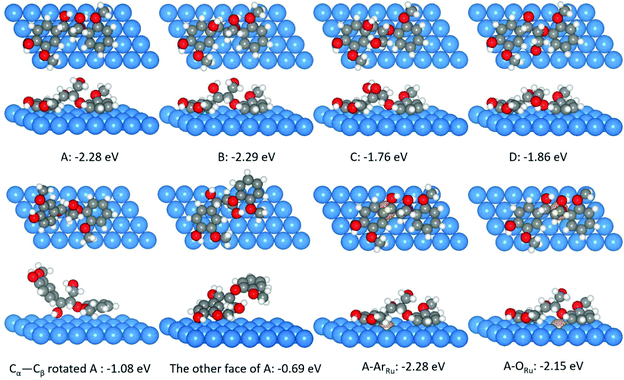 |
| Fig. 17 Adsorptions of four β-O-4 bond isomers, two structures from A isomer after rotating the Cα–Cβ bond and using the other face on the Ni(111) surface. ArRu and ORu are two surface A isomer structures on the Ru–Ni(111) surface. Ni, Ru, C, H, and O atoms are shown in blue, yellow, gray, white, and red, respectively.190 | |
Hemicellulose.
The catalytic conversion of lignocellulose currently focuses on the cellulose and lignin components, and there are few reports on the conversion of hemicellulose. Efficient catalytic systems for hemicellulose include acidic ILs, zeolite, and Brønsted acid. The main products involving furfural, furan, and xylose are available in cellulose as well, due to the similar structures of hemicellulose and cellulose.193 However, hemicellulose/xylose is observed to be more susceptible to degradation than cellulose/glucose, and hemicellulose could be hydrolyzed into xylose in a mineral acid/IL system.1 Furfural is a key derivative from hemicellulose, and many experimental results have shown the successful conversion of xylose to furfural,194,195 but there are only a few mechanism studies using simulation. Wang et al. reported the decomposition of xylose to furfural in gas and water phases using DFT calculations.76 It was found that the formation of furfural was more thermodynamically feasible in water than in the gas phase. Additionally, the explicit water molecules forced the formation of less distorted six-membered transition states, which significantly reduced the reaction barriers.76 In another study, Zhang et al. reported that the Lewis acid catalyst AlCl3/[Bmim][Cl] among various mineral acids (H2SO4, HCl, and H3PO4) and metal chlorides (i.e., CuCl, FeCl3·6H2O, and CrCl3/LiCl) showed the best performance for furfural production with yields of furfural from xylose and xylan of 82.2% and 84.8%, respectively.194 This was attributed to the fact that the glycosidic bonds could be weakened through hydrogen bonding interactions between the chloride anions and hydroxyl groups in xylose, and xylose was isomerized to form an enolate structure, followed by dehydration to produce furfural by AlCl3. Sievers et al. reported a successful dehydration of xylose to furfural with H2SO4 in [Bmim][Cl].196 Hence, a catalytic system containing both a Lewis acid and a Brønsted acid might efficiently promote the conversion of xylose to furfural. As reported by Choudhary et al.,197 the combination of a Lewis acid and a Brønsted acid could substantially improve the efficiency of furfural production, where the Lewis acid contributes to the first isomerization of xylose to xylulose and lyxose and subsequently of hydrate to furfural using a Brønsted acid. In comparison with aqueous-based processes, production of furfural in ILs is an effective alternative process.
4.4 Mechanism of pyrolysis
Biomass pyrolysis is a process in which biomass materials are thermally or thermochemically degraded in the absence of air or oxygen. The pyrolysis of lignocellulose is a promising route for the production of liquid fuels, charcoal and other gaseous products (e.g. H2, CO2, CH4, and CO). These pyrolysis products can be further upgraded or refined to value-added chemicals, transportation fuels, and electricity generation. Usually, the three components of the plant cell wall decompose at different temperature ranges. The hemicellulose firstly breaks down in the temperature range of 470 to 530 K, cellulose pyrolyzes in the temperature range of 510 to 620 K, and lignin pyrolyzes at a higher temperature range of 550 to 770 K.198 Currently, pyrolysis technologies are relatively well developed, and research studies are focusing on understanding the underlying processes from a molecular level or by numerical modelling. The chemical kinetics, heat transfer and mass transfer in the numerical modelling of pyrolysis were described in detail in reviews;20,199–201 thus we only focus on the method of quantum chemistry and MD simulations, which shed light on the mechanism for the pyrolysis of cellulose, hemicellulose, and lignin.
Cellulose.
In the pyrolysis of cellulose, a complete study on the reaction pathways is essential to understand the dehydration, the breaking of glycosidic linkages, and the ring-opening of glucose. Cellobiose has been applied as a good model compound to explore the characteristics of cellulose because it can represent the functionalities and complexity of cellulose.202 Zhang et al. studied the dehydration of cellulose using DFT calculations. The results confirmed that the location of hydroxyl groups C2–OH might make O2H difficult to leave unless at high temperature. The activity of hydroxyl groups followed the following sequence: O2H, O3H, and O6H.203 Auerbach's group modelled the decomposition processes in cellulose pyrolysis at temperatures of 600.15 K and 873.15 K using Car–Parrinello molecular dynamics (CPMD) simulations.204 The energy barriers for the generation of formic acid, 5-hydroxymethylfurfural (HMF) and levoglucosan precursor (pre-LGA) were estimated. It was demonstrated that the energy barriers at 873.15 K were in the following order: pre-LGA < pre-HMF < formic acid, which explains why LGA is the major product in the fast pyrolysis of cellulose. Chen et al. explored the initial pyrolysis of cellobiose using DFT calculations,205 the results of which are shown in Fig. 18. It was found that [H]+ cations played a significant role in cleaving glycosidic bonds to form primary products, such as 5-HMF, levoglucosan (LG), and hydroxyacetaldehyde.205 Recently, Zhang et al. calculated the pathways for levoglucosan in pyrolysis using DFT methods.206,207 In total, nine different pathways for C–O and C–C bond breaking and dehydration were obtained. It was concluded that C–O bonds bore a lower activation energy than C–C bonds such that it was easier to break them.206,207 Jiang et al. investigated the energies during the homolytic bond dissociation of cellulose dimers by the method of M06-2X. It was found that the cleavage of glycosidic bonds was thermodynamically more proper with respect to C–OH bonds.208 The bond dissociation energy (BDE) of glycosidic bonds was in the range of 45–51 kcal mol−1, while the BDE of C–OH bonds was in the range of 62–70 kcal mol−1. Furthermore, the results also suggested that temperature had a significant influence in controlling the selectivity of different types of reactions.208 Geng et al. provided the breaking mechanism of pyran rings during the pyrolysis of cellulose by DFT methods.209 It was reported that the rate-determining step for processing small molecular-weight products was the breaking of pyran rings. The easiest reaction pathway follows the Retro-Aldol mechanism, Retro-Diels–Alders mechanism, and C6–OH assisted cleavage reaction of pinacol rings. However, high temperatures caused the flipping of pyran rings into different chair positions, but chair positions had a negligible effect on the reaction mechanism.209 As is known, hydroxylactone (1R,5S)-1-hydroxy-3,6-dioxabicyclo[3.2.1]octan-2-one (LAC) is one of the most interesting products derived from the pyrolysis of cellulose, which is a useful building block in organic synthesis. Marforio et al. studied the reaction pathways to generate LAC by DFT methods.210 The results of their study revealed that water molecules assisted the proton transfers, thus lowering the reaction barrier by about 40 kcal mol−1. Recent research studies have enriched our perspectives on the mechanism of cellulose pyrolysis. Adjusting the temperatures and applying proper catalysts is beneficial to design potential chemical platforms. Additionally, the pyrolysis process of cellulose was studied by MD simulations. Zheng et al. performed the first GPU-enabled Reactive Force Field (ReaxFF) MD simulation to investigate the initial reaction mechanism of cellulose pyrolysis.211 In a temperature range of 500–1400 K, the major products glycolaldehyde, levoglucosan, and water were identified, which is in agreement with the experimental results. In turn, cellulose proved to be a good model to validate the GPU-enabled ReaxFF, which is a promising method to study the pyrolysis mechanism of lignin and other biomass materials.
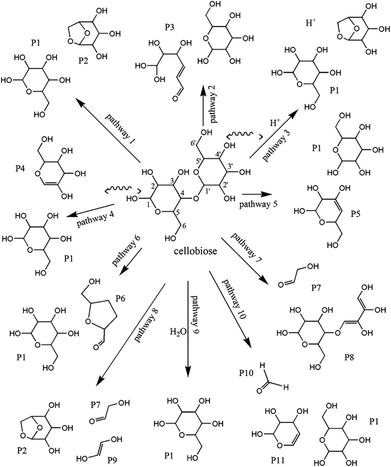 |
| Fig. 18 Reaction pathways of cellobiose pyrolysis.205 | |
Lignin.
As compared to hemicellulose and cellulose, lignin breaks down at a higher and wider temperature range because of its more complex linkages. Though some lines of evidence have been obtained for the type of linkage in lignin, the mechanism of lignin pyrolysis is not fully understood. Kim et al. computed the bond dissolution enthalpies (BDE) for the four prevalent linkages (β-O-4, α-O-4, β-5, and 5–5) in 69 lignin model compounds.212 The BDE revealed that the C–C bonds are more stable than the ether bonds, and the β-O-4 linkage is more durable than other types of ether bonds, but catalytic dehydrogenation would greatly reduce the bond energy for C–O bond cleavage.212 To better understand the pyrolysis mechanism, Huang et al. studied the decomposition processes of β-1 model compound of lignin using DFT calculations at the B3LYP/6-31G(d,p) level.213 Of three reaction pathways, the homolytic cleavage of Cα–Cβ was the major reaction channel, which is attributed to the lower bond dissociation energies.
Thus, the cleavage of Cα–Cβ is thought to be an important reaction step during the pyrolysis of lignin. Apart from the β-1 linkage, the β-O-4 and α-O-4 ether bonds were paid special attention. The α-O-4 linked lignin dimer was investigated by Huang et al., and they proposed that the main pyrolysis products of the lignin dimer were guaiacol, 2-hydroxybenzaldehyde, p-hydroxyphenyl-ethanol, and p-hydroxyphenyl-acetaldehyde.214 Chen et al. elucidated the pyrolytic products of a type of β-O-4 model compound.215 The β-O-4 lignin dimer experienced the hemolytic cleavage of Cβ–O bonds to 4-methoxystyrene and guaiacol. Wang et al. found that carbon monoxide (CO), benzene, catechol, phenol, and cyclopentadiene were the most characteristic products from the secondary pyrolysis of vanillin.216 Moreover, the presence of formyl, hydroxyl and methoxy groups facilitated the formation of CO.
Hemicellulose.
Thermal degradation of hemicellulose occurs firstly in a temperature range of 470–530 K.198 The decomposition mechanism of hemicellulose was investigated using the model compounds arabinofuranose, xylopyranose, xylobiose, etc. Huang et al. studied the thermal decomposition mechanism of hemicellulose using the DFT method at the B3LYP/6-31++G(d,p) level.217 The reaction pathways for the formation of major products were identified during decomposition. The low molecular-weight products such as furfural, CO, acetone, and acetaldehyde were in accordance with the experimental results. Recently, Huang et al. investigated the thermal degradation of xylose at the M06-2X/6-31++G(d,p) basis set, and their results showed that the low molecular products were glycolaldehyde, acetaldehyde, acetone and 2-furaldehyde, derived from eight possible pyrolytic reaction pathways.218 To reveal the influence of the adjacent xylose, the pyrolysis behaviour of xylobiose was studied by Li et al.219 It was indicated that the dominant stage was the ring-opening of xylose followed by the cleavage of β-1,4-glycosidic bonds. The cleavage of C1–O bonds was the major channel to generate the major product furfural. Another model compound of hemicellulose, arabinofuranose, was investigated as well.220 The presence of [H]+ facilitated the dehydrogenation reactions when decomposing xylose and O-acetyl xylose.77 According to the pyrolytic pathways, the pyrolysis of cellulose, hemicellulose and lignin all led to the production of CO and H2, but the furfural mainly came from cellulose and hemicellulose, CO2 was generated from hemicellulose, while the lignin component gave higher content of C2H2.77 To some extent, the pyrolysis of different model compounds gives the same type of pyrolytic products and competitive products.
5. Conclusions
The innovative valorization of the primary ingredients of lignocellulose has attracted attention for a long time, and most of the research has experimentally focused on the technologies in relation to dissolution and degradation, catalytic conversion, and pyrolysis processes. Computer-assisted modelling and simulation, including quantum chemical calculations and molecular dynamics simulations, have been invaluable in understanding the complex intramolecular interactions between lignocellulosic components and their intermolecular forces with solvents and catalysts, and the relationship to their thermodynamic and transport properties. The current simulation methods being used specifically study the cell wall ingredients, such as cellulose, lignin, and hemicellulose, and have become powerful approaches to explain the mechanisms behind utilizing lignocellulose. It has been convincingly evidenced that electrostatic interactions, van der Waals forces, the most important hydrogen bonds, and dispersion forces lead to the recalcitrance of lignocellulose. The differences in components, linkages, and final structures make cellulose, lignin, and hemicellulose unique to be dissolved in different solvents, be degraded at different temperature ranges and produce various fine and bulk chemicals, and to be degraded at different temperature ranges. Generally, the intrinsic mechanisms of lignocellulosic chemistry are extremely complicated and hard to elucidate. Although considerable effort has already been devoted to developing a wide variety of transformation routes for lignocellulosic components, several issues with respect to the microscopic mechanism should be clarified in the next step.
Firstly, proper models need to be developed. As is known, cellulose has a precise crystal structure which can be directly calculated by large-scale MD simulations. However, the structure of lignin is still ambiguous due to the different types of plants. The model compounds, such as monomers, dimers, and short-chained polymers, are usually used. However, none of them is the real model of natural lignin. The construction of lignin models close to real systems should be developed using a more sophisticated program with the help of experimental data.
Secondly, large systems and long time scales would be advantageous in achieving the structural and dynamic properties of lignocellulose in accordance with its macroscopic properties. With the development of computing ability, the evolution of microstructural features can be identified. To achieve this goal, theoretical and computational methods are used to predict the mechanism behaviours. One promising approach is the combination of semi-empirical QC, DFT QC, all-atom MD simulations, and coarse-grained MD simulations, which describe different parts of the model system. The proper combination of different methods enables studying with longer time scales and larger systems. The multiscale simulation methods could also be used to elucidate the evolution of microstructures with the help of statistical analysis.
Thirdly, special attention should be paid to the interfacial interaction between the three typical components. In the plant cell wall, cellulose, lignin, and hemicellulose are intertwined with each other and are hard to separate. Therefore, a simulation study should also be devoted to the cellulose–lignin, cellulose–hemicellulose, and lignin–hemicellulose interactions. The types of bonds and monomers, and their sequences should also be taken into consideration. Furthermore, research on the mechanical behaviours of lignocellulose should be strengthened in the near future. Following various functional materials from biomass, theoretical modelling can be used to capture the micro-scale characteristics of the materials.
Successful application of molecular simulation can provide rich information about the accurate reaction energies and atomistic interactions, which are experimentally unachievable. The development of simulation methods will undoubtedly promote the the development of technologies for the utilization of lignocellulosic materials.
Conflicts of interest
There are no conflicts to declare.
Acknowledgements
This work was supported by the National Natural Science Foundation of China (21406230, 21336002, and 21736003), Beijing Natural Science Foundation (2182068) and Youth Innovation Promotion Association of CAS (2017066).
References
- Z. R. Zhang, J. L. Song and B. X. Han, Chem. Rev., 2017, 117, 6834–6880 CrossRef CAS PubMed.
- J. X. Long, X. H. Li, B. Guo and F. R. Wang,
et al.
, Green Chem., 2012, 14, 1935–1941 RSC.
-
National Twelfth Five-Year Plan on biomass energy, Chinese National Energy Administration, Beijing, 2012 Search PubMed.
-
National Thirteen Five-Year Plan on biomass energy, Chinese National Energy Administration, Beijing, 2016 Search PubMed.
-
J. Spaeth, Task 42-country report United States, IEA Bioenergy, 2014 Search PubMed.
-
H. Jørgensen, Task 42-country report Denmark, IEA Bioenergy, 2014 Search PubMed.
- K. Sanderson, Nature, 2011, 474, S12–S14 CrossRef CAS PubMed.
- S. Sen, J. D. Martin and D. S. Argyropoulos, ACS Sustainable Chem. Eng., 2013, 1, 858–870 CrossRef CAS.
- C.-H. Zhou, X. Xia, C.-X. Lin and D.-S. Tong,
et al.
, Chem. Soc. Rev., 2011, 40, 5588–5617 RSC.
- J. Zakzeski, P. C. Bruijnincx, A. L. Jongerius and B. M. Weckhuysen, Chem. Rev., 2010, 110, 3552–3599 CrossRef CAS PubMed.
- C. Li, X. Zhao, A. Wang and G. W. Huber,
et al.
, Chem. Rev., 2015, 115, 11559–11624 CrossRef CAS PubMed.
- A. Corma, S. Iborra and A. Velty, Chem. Rev., 2007, 107, 2411–2502 CrossRef CAS PubMed.
- H. de Lasa, E. Salaices, J. Mazumder and R. Lucky, Chem. Rev., 2011, 111, 5404–5433 CrossRef CAS PubMed.
- K. H. Kim and J. Hong, Bioresour. Technol., 2001, 77, 139–144 CrossRef CAS PubMed.
- S. S. Y. Tan, D. R. MacFarlane, J. Upfal and L. A. Edye,
et al.
, Green Chem., 2009, 11, 339–345 RSC.
- R. P. Swatloski, S. K. Spear, J. D. Holbrey and R. D. Rogers, J. Am. Chem. Soc., 2002, 124, 4974–4975 CrossRef CAS PubMed.
- M. Stöcker, Angew. Chem., Int. Ed., 2008, 47, 9200–9211 CrossRef PubMed.
- Y. Sun and J. Cheng, Bioresour. Technol., 2002, 83, 1–11 CrossRef CAS PubMed.
- R. Kumar, S. Singh and O. V. Singh, J. Ind. Microbiol. Biotechnol., 2008, 35, 377–391 CrossRef CAS PubMed.
- S. Kersten and M. Garcia-Perez, Curr. Opin. Biotechnol., 2013, 24, 414–420 CrossRef CAS PubMed.
- B. D. Schutt, B. Serrano, R. L. Cerro and M. A. Abraham, Biomass Bioenergy, 2002, 22, 365–375 CrossRef CAS.
- F. Yue, C. M. Pedersen, X. Yan and Y. Liu,
et al.
, Green Energy Environ., 2018, 3, 163–171 CrossRef.
- S. K. Hanson, R. L. Wu and L. A. Silks, Angew. Chem., Int. Ed., 2012, 51, 3410–3413 CrossRef CAS PubMed.
- D. Klein-Marcuschamer, P. Oleskowicz-Popiel, B. A. Simmons and H. W. Blanch, Biomass Bioenergy, 2010, 34, 1914–1921 CrossRef CAS.
- G. Chatel and R. D. Rogers, ACS Sustainable Chem. Eng., 2014, 2, 322–339 CrossRef CAS.
- X. Zhang, W. Yang and W. Blasiak, Energy Fuels, 2011, 25, 4786–4795 CrossRef CAS.
- Y. Q. Zhang, H. Y. He, S. J. Zhang and M. H. Fan, ACS Omega, 2018, 3, 1823–1833 CrossRef CAS.
- Y. Li, X. Liu, Y. Zhang and K. Jiang,
et al.
, ACS Sustainable Chem. Eng., 2017, 5, 3417–3428 CrossRef CAS.
- S. Besombes and K. Mazeau, Biopolymers, 2004, 73, 301–315 CrossRef CAS PubMed.
- J. Kahlen, K. Masuch and K. Leonhard, Green Chem., 2010, 12, 2172–2181 RSC.
- A. Casas, J. Palomar, M. V. Alonso and M. Oliet,
et al.
, Ind. Crops Prod., 2012, 37, 155–163 CrossRef CAS.
- S. K. Singh and P. L. Dhepe, Green Chem., 2016, 18, 4098–4108 RSC.
- S. H. Lee, T. V. Doherty, R. J. Linhardt and J. S. Dordick, Biotechnol. Bioeng., 2009, 102, 1368–1376 CrossRef CAS PubMed.
- N. Mosier, C. Wyman, B. Dale and R. Elander,
et al.
, Bioresour. Technol., 2005, 96, 673–686 CrossRef CAS PubMed.
- H. Staudinger, Ber. Dtsch. Chem. Ges., 1920, 53, 1073–1085 CrossRef.
- S. Besombes and K. Mazeau, Plant Physiol. Biochem., 2005, 43, 299–308 CrossRef CAS PubMed.
- S. Nishikawa and S. Ono, Proc. Tokyo Math.-Phys. Soc., 1913, 7, 131–138 CAS.
- Y. Nishiyama, P. Langan and H. Chanzy, J. Am. Chem. Soc., 2002, 124, 9074–9082 CrossRef CAS PubMed.
- Y. Nishiyama, J. Sugiyama, H. Chanzy and P. Langan, J. Am. Chem. Soc., 2003, 125, 14300–14306 CrossRef CAS PubMed.
- L. M. J. KroonBatenburg and J. Kroon, Glycoconjugate J., 1997, 14, 677–690 CrossRef CAS.
- R. J. Moon, A. Martini, J. Nairn and J. Simonsen,
et al.
, Chem. Soc. Rev., 2011, 40, 3941–3994 RSC.
- M. Li, Y. Wang, X. Hou and X. Wan,
et al.
, Green Energy Environ., 2018, 3, 138–146 CrossRef.
- R. C. Dean, Biol. Bull., 1978, 155, 297–316 CrossRef CAS.
- H. Wang, G. Gurau and R. D. Rogers, Chem. Soc. Rev., 2012, 41, 1519–1537 RSC.
- F. G. Calvo-Flores and J. A. Dobado, ChemSusChem, 2010, 3, 1227–1235 CrossRef CAS PubMed.
- Z. Li, J. Nanjing For. Univ., Nat. Sci. Ed., 2012, 36, 1–7 CAS.
- C. S. Lancefield and N. J. Westwood, Green Chem., 2015, 17, 4980–4990 RSC.
- N. E. El Mansouri and J. Salvado, Ind. Crops Prod., 2007, 26, 116–124 CrossRef CAS.
- Y. S. Kim, H. M. Chang and J. F. Kadla, J. Wood Chem. Technol., 2007, 27, 225–241 CrossRef CAS.
- Y. S. Kim, H. M. Chang and J. F. Kadla, Holzforschung, 2008, 62, 38–49 CAS.
- T. L. Anni Lähdetie, T. Tamminen and A.-S. Jääskeläinen, BioResources, 2009, 4, 1600–1619 Search PubMed.
- W. Schutyser, T. Renders, S. Van den Bosch and S. F. Koelewijn,
et al.
, Chem. Soc. Rev., 2018, 47, 852–908 RSC.
- S. Rivas, C. Vila, V. Santos and J. C. Parajo, Holzforschung, 2016, 70, 901–910 CAS.
- F. M. Gírio, C. Fonseca, F. Carvalheiro and L. C. Duarte,
et al.
, Bioresour. Technol., 2010, 101, 4775–4800 CrossRef PubMed.
- F. Carvalheiro, L. C. Duarte and F. M. Girio, J. Sci. Ind.
Res., 2008, 67, 849–864 CAS.
- A. Ebringerova, Z. Hromadkova and T. Heinze, Adv. Polym. Sci., 2005, 186, 1–67 CrossRef CAS.
- A. S. Mamman, J. M. Lee, Y. C. Kim and I. T. Hwang,
et al.
, Biofuels, Bioprod. Biorefin., 2008, 2, 438–454 CrossRef CAS.
- R. Xing, W. Qi and G. W. Huber, Energy Environ. Sci., 2011, 4, 2193–2205 RSC.
- K. Jin, Z. Qin and M. J. Buehler, J. Mech. Behav. Biomed. Mater., 2015, 42, 198–206 CrossRef CAS PubMed.
- L. Charlier and K. Mazeau, J. Phys. Chem. B, 2012, 116, 4163–4174 CrossRef CAS PubMed.
- R. L. Silveira, S. R. Stoyanov, S. Gusarov and M. S. Skaf,
et al.
, J. Phys. Chem. Lett., 2015, 6, 206–211 CrossRef CAS PubMed.
- R. L. Silveira, S. R. Stoyanov, S. Gusarov and M. S. Skaf,
et al.
, J. Am. Chem. Soc., 2013, 135, 19048–19051 CrossRef CAS PubMed.
- P. Langan, L. Petridis, H. M. O'Neill and S. V. Pingali,
et al.
, Green Chem., 2014, 16, 63–68 RSC.
- Y. Yao, Y. Li, X. Liu and X. Zhang,
et al.
, Chin. J. Chem. Eng., 2015, 23, 1894–1906 CrossRef CAS.
- Z. D. Ding, Z. Chi, W. X. Gu and S. M. Gu,
et al.
, Carbohydr. Polym., 2012, 89, 7–16 CrossRef CAS PubMed.
- H. B. Liu, G. Cheng, M. Kent and V. Stavila,
et al.
, J. Phys. Chem. B, 2012, 116, 8131–8138 CrossRef CAS PubMed.
- B. Lindner, L. Petridis, R. Schulz and J. C. Smith, Biomacromolecules, 2013, 14, 3390–3398 CrossRef CAS PubMed.
- B. G. Janesko, Phys. Chem. Chem. Phys., 2014, 16, 5423–5433 RSC.
- Y. Zhang, H. He, K. Dong and M. Fan,
et al.
, RSC Adv., 2017, 7, 12670–12681 RSC.
- C. Balaji, T. Banerjee and V. V. Goud, J. Solution Chem., 2012, 41, 1610–1630 CrossRef CAS.
- J. M. Lu, M. Wang, X. C. Zhang and A. Heyden,
et al.
, ACS Catal., 2016, 6, 5589–5598 CrossRef CAS.
- L. Petridis and J. C. Smith, J. Comput. Chem., 2009, 30, 457–467 CrossRef CAS PubMed.
- L. Petridis, R. Schulz and J. C. Smith, J. Am. Chem. Soc., 2011, 133, 20277–20287 CrossRef CAS PubMed.
- R. S. Payal, R. Bharath, G. Periyasamy and S. Balasubramanian, J. Phys. Chem. B, 2012, 116, 833–840 CrossRef CAS PubMed.
- J. Berglund, T. A. d'Ortoli, F. Vilaplana and G. Widmalm,
et al.
, Plant J., 2016, 88, 56–70 CrossRef CAS PubMed.
- M. Wang, C. Liu, Q. B. Li and X. X. Xu, J. Mol. Model., 2015, 21, 296 CrossRef PubMed.
- X. Y. Huang, B. G. Cheng, F. Q. Chen and X. L. Zhan, Renewable Energy, 2016, 96, 490–497 CrossRef CAS.
- C. C. J. Roothaan, Rev. Mod. Phys., 1951, 23, 69–89 CrossRef CAS.
-
R. G. Parr, in Horizons of Quantum Chemistry, Springer, 1980, pp. 5–15 Search PubMed.
- S. Grimme, Wiley Interdiscip. Rev.: Comput. Mol. Sci., 2011, 1, 211–228 CAS.
- Y. Zhao and D. G. Truhlar, Theor. Chem. Acc., 2008, 120, 215–241 Search PubMed.
- K. Raghavachari, Theor. Chem. Acc., 2000, 103, 361–363 Search PubMed.
- C. Adamo and V. Barone, J. Chem. Phys., 1999, 110, 6158–6170 CrossRef CAS.
- S. Grimme, J. Comput. Chem., 2006, 27, 1787–1799 CrossRef CAS PubMed.
- S. Grimme, J. Antony, S. Ehrlich and H. Krieg, J. Chem. Phys., 2010, 132, 154104 CrossRef PubMed.
- L. Goerigk and S. Grimme, Phys. Chem. Chem. Phys., 2011, 13, 6670–6688 RSC.
- J. M. Ho, A. Klamt and M. L. Coote, J. Phys. Chem. A, 2010, 114, 13442–13444 CrossRef CAS PubMed.
- E. L. M. Miguel, C. I. L. Santos, C. M. Silva and J. R. Pliego, J. Braz. Chem. Soc., 2016, 27, 2055–2061 CAS.
- A. Kovalenko and F. Hirata, Chem. Phys. Lett., 1998, 290, 237–244 CrossRef CAS.
- A. Kovalenko and F. Hirata, J. Phys. Chem. B, 1999, 103, 7942–7957 CrossRef CAS.
- A. Kovalenko and F. Hirata, Phys. Chem. Chem. Phys., 2005, 7, 1785–1793 RSC.
- A. V. Marenich, C. J. Cramer and D. G. Truhlar, J. Phys. Chem. B, 2009, 113, 6378–6396 CrossRef CAS PubMed.
- J. R. Pliego, J. Braz. Chem. Soc., 2015, 26, 1737–1741 CAS.
- A. V. Marenich, C. J. Cramer and D. G. Truhlar, J. Phys. Chem. B, 2009, 113, 4538–4543 CrossRef CAS PubMed.
- K. Dong, X. M. Liu, H. F. Dong and X. P. Zhang,
et al.
, Chem. Rev., 2017, 117, 6636–6695 CrossRef CAS PubMed.
- S. J. Weiner, P. A. Kollman, D. T. Nguyen and D. A. Case, J. Comput. Chem., 1986, 7, 230–252 CrossRef CAS PubMed.
- A. D. MacKerell, D. Bashford, M. Bellott and R. L. Dunbrack,
et al.
, J. Phys. Chem. B, 1998, 102, 3586–3616 CrossRef CAS PubMed.
- W. L. Jorgensen, D. S. Maxwell and J. TiradoRives, J. Am. Chem. Soc., 1996, 118, 11225–11236 CrossRef CAS.
- C. Oostenbrink, A. Villa, A. E. Mark and W. F. Van Gunsteren, J. Comput. Chem., 2004, 25, 1656–1676 CrossRef CAS PubMed.
- A. Barducci, M. Bonomi and P. Derreumaux, J. Chem. Theory Comput., 2011, 7, 1928–1934 CrossRef CAS PubMed.
- G. D. Smith and W. Paul, J. Phys. Chem. A, 1998, 102, 1200–1208 CrossRef CAS.
- L. J. Yang and R. Luo, Biophys. J., 2005, 88, 512a–512a Search PubMed.
-
D. E. Shaw, R. O. Dror, J. K. Salmon and J. P. Grossman, et al., Proceedings of the Conference on High Performance Computing Networking, Storage and Analysis, 2009 Search PubMed.
- A. Klamt, J. Phys. Chem., 1995, 99, 2224–2235 CrossRef CAS.
- A. Klamt and G. Schuurmann, J. Chem. Soc., Perkin Trans. 2, 1993, 799–805 RSC.
- Z. Guo, B. M. Lue, K. Thomasen and A. S. Meyer,
et al.
, Green Chem., 2007, 9, 1362–1373 RSC.
- A. Klamt, Wiley Interdiscip. Rev.: Comput. Mol. Sci., 2018, 8, e1388 Search PubMed.
- M. Gonzaez-Miquel, M. Massel, A. DeSilva and J. Palomar,
et al.
, J. Phys. Chem. B, 2014, 118, 11512–11522 CrossRef PubMed.
- M. Diedenhofen and A. Klamt, Fluid Phase Equilib., 2010, 294, 31–38 CrossRef CAS.
- K. Mazeau, Carbohydr. Polym., 2011, 84, 524–532 CrossRef CAS.
- J. L. Faulon, G. A. Carlson and P. G. Hatcher, Org. Geochem., 1994, 21, 1169–1179 CrossRef CAS.
- S. Besombes and K. Mazeau, Plant Physiol. Biochem., 2005, 43, 277–286 CrossRef CAS PubMed.
- J. V. Vermaas, L. Petridis, X. H. Qi and R. Schulz,
et al.
, Biotechnol. Biofuels, 2015, 8, 217 CrossRef PubMed.
- A. D. French, D. P. Miller and A. Aabloo, Int. J. Biol. Macromol., 1993, 15, 30–36 CrossRef CAS PubMed.
- Y. Nishiyama, P. Langan and H. Chanzy, J. Am. Chem. Soc., 2002, 124, 9074–9082 CrossRef CAS PubMed.
- J. F. Matthews, C. E. Skopec, P. E. Mason and P. Zuccato,
et al.
, Carbohydr. Res., 2006, 341, 138–152 CrossRef CAS PubMed.
- T. Yui and S. Hayashi, Biomacromolecules, 2007, 8, 817–824 CrossRef CAS PubMed.
- A. P. Heiner, J. Sugiyama and O. Teleman, Carbohydr. Res., 1995, 273, 207–223 CrossRef CAS.
- M. Bergenstrahle, J. Wohlert, M. E. Himmel and J. W. Brady, Carbohydr. Res., 2010, 345, 2060–2066 CrossRef CAS PubMed.
- A. S. Gross, A. T. Bell and J. W. Chu, J. Phys. Chem. B, 2013, 117, 3280–3286 CrossRef CAS PubMed.
- B. Cao, J. Du, Z. Cao and X. Sun,
et al.
, Carbohydr. Polym., 2017, 169, 227–235 CrossRef CAS PubMed.
- H. Miyamoto, U. Schnupf, K. Ueda and C. Yamane, Nord. Pulp Pap. Res. J., 2015, 30, 67–77 CAS.
- E. Wernersson, B. Stenqvist and M. Lund, Cellulose, 2015, 22, 991–1001 CrossRef CAS.
- L. K. Tolonen, M. Bergenstrahle-Wohlert, H. Sixta and J. Wohlert, J. Phys. Chem. B, 2015, 119, 4739–4748 CrossRef CAS PubMed.
- Y. Li, X. Liu, S. Zhang and Y. Yao,
et al.
, Phys. Chem. Chem. Phys., 2015, 17, 17894–17905 RSC.
- X. Yuan and G. Cheng, Phys. Chem. Chem. Phys., 2015, 17, 31592–31607 RSC.
- A. G. Aleksandr and V. L. Valery, Russ. Chem. Rev., 2005, 74, 21–35 CrossRef.
- Y. Ogihara, R. L. Smith, H. Inomata and K. Arai, Cellulose, 2005, 12, 595–606 CrossRef CAS.
- D. A. Canter, M. D. Bermejo and M. J. Cocero, J. Supercrit. Fluids, 2015, 96, 21–35 CrossRef.
- A. Pinkert, K. N. Marsh, S. Pang and M. P. Staiger, Chem. Rev., 2009, 109, 6712–6728 CrossRef CAS PubMed.
- A. S. Gross, A. T. Bell and J. W. Chu, J. Phys. Chem. B, 2011, 115, 13433–13440 CrossRef CAS PubMed.
- Y. Zhao, X. Liu, J. Wang and S. Zhang, Carbohydr. Polym., 2013, 94, 723–730 CrossRef CAS PubMed.
- B. Lu, A. Xu and J. Wang, Green Chem., 2014, 16, 1326–1335 RSC.
- A. M. Fernandes, M. A. A. Rocha, M. G. Freire and I. M. Marrucho,
et al.
, J. Phys. Chem. B, 2011, 115, 4033–4041 CrossRef CAS PubMed.
- J.-M. Andanson, E. Bordes, J. Devémy and F. Leroux,
et al.
, Green Chem., 2014, 16, 2528–2538 RSC.
- S. Velioglu, X. Yao, J. Devémy and M. G. Ahunbay,
et al.
, J. Phys. Chem. B, 2014, 118, 14860–14869 CAS.
- F. Huo, Z. Liu and W. Wang, J. Phys. Chem. B, 2013, 117, 11780–11792 CrossRef CAS PubMed.
- K. M. Gupta, Z. Hu and J. Jiang, RSC Adv., 2013, 3, 4425–4433 RSC.
- H. Nawaz, P. A. R. Pires, E. P. G. Areas and N. I. Malek,
et al.
, Macromol. Chem. Phys., 2015, 216, 2368–2376 CrossRef CAS.
- R. C. Remsing, R. P. Swatloski, R. D. Rogers and G. Moyna, Chem. Commun., 2006, 1271–1273 RSC.
- S. Zhu, Y. Wu, Q. Chen and Z. Yu,
et al.
, Green Chem., 2006, 8, 325–327 RSC.
- J. W. Hao Zhang, J. Zhang and J. He, Macromolecules, 2005, 38, 8272–8277 CrossRef.
- K. A. Kurnia, C. M. Neves, M. G. Freire and L. M. Santos,
et al.
, J. Mol. Liq., 2015, 210, 264–271 CrossRef CAS PubMed.
- P. Matheswaran, C. D. Wilfred, K. A. Kurnia and A. Ramli, Ind. Eng. Chem. Res., 2016, 55, 788–797 CrossRef CAS.
- X. Zhao, Q. W. Yang, D. Xu and Z. B. Bao,
et al.
, AIChE J., 2015, 61, 2016–2027 CrossRef CAS.
- A. Casas, S. Omar, J. Palomar and M. Oliet,
et al.
, RSC Adv., 2013, 3, 3453–3460 RSC.
- C. Loschen and A. Klamt, Ind. Eng. Chem. Res., 2014, 53, 11478–11487 CrossRef CAS.
- J. Vitz, T. Erdmenger, C. Haensch and U. S. Schubert, Green Chem., 2009, 11, 417–424 RSC.
- Y. R. Liu, K. Thomsen, Y. Nie and S. J. Zhang,
et al.
, Green Chem., 2016, 18, 6246–6254 RSC.
- H. Xu, W. X. Pan, R. X. Wang and D. J. Zhang,
et al.
, J. Comput.-Aided Mol. Des., 2012, 26, 329–337 CrossRef CAS PubMed.
- M. N. A. Mohamed, H. D. Watts, J. Guo and J. M. Catchmark,
et al.
, Carbohydr. Res., 2010, 345, 1741–1751 CrossRef CAS PubMed.
- H. B. Du and X. H. Qian, Carbohydr. Res., 2011, 346, 1985–1990 CrossRef CAS PubMed.
- X. Wei, Z. Han and D. Zhang, Carbohydr. Res., 2013, 374, 40–44 CrossRef CAS PubMed.
- F. Parveen, T. Patra and S. Upadhyayula, Carbohydr. Polym., 2016, 135, 280–284 CrossRef CAS PubMed.
- A. Kamimura, T. Okagawa, N. Oyama and T. Otsuka,
et al.
, Green Chem., 2012, 14, 2816–2820 RSC.
- J. Y. Wang, M. D. Zhou, Y. G. Yuan and Q. Zhang,
et al.
, Bioresour. Technol., 2015, 197, 42–47 CrossRef CAS PubMed.
- K. L. Zhuo, Q. Z. Du, G. Y. Bai and C. Y. Wang,
et al.
, Carbohydr. Polym., 2015, 115, 49–53 CrossRef CAS PubMed.
- C. Loerbroks, R. Rinaldi and W. Thiel, Chem. – Eur. J., 2013, 19, 16282–16294 CrossRef CAS PubMed.
- A. M. Navarrete-Lopez, M. L. San-Roman and C. M. Zicovich-Wilson, Theor. Chem. Acc., 2016, 135, 136 Search PubMed.
- W. Ji, Z. Ding, J. Liu and Q. Song,
et al.
, Energy Fuels, 2012, 26, 6393–6403 CrossRef CAS.
- B. G. Janesko, Phys. Chem. Chem. Phys., 2011, 13, 11393–11401 RSC.
- J. Wang, X. Shi, X. Du and W. Cao, Russ. J. Phys. Chem. A, 2017, 91, 1468–1473 CrossRef CAS.
- J. J. Zhang, J. Q. Zhu, C. F. Lin and T. P. Wang,
et al.
, Int. J. Agric. Biol. Eng., 2016, 9, 127–136 Search PubMed.
- B. D. Mar, H. W. Qi, F. Liu and H. J. Kulik, J. Phys. Chem. A, 2015, 119, 6551–6562 CrossRef CAS PubMed.
- L. Petridis and J. C. Smith, ChemSusChem, 2016, 9, 289–295 CrossRef CAS PubMed.
- J. J. Li, J. H. Li, D. J. Zhang and C. B. Liu, ChemPhysChem, 2015, 16, 3044–3048 CrossRef CAS PubMed.
- J. L. Li, J. H. Li, D. J. Zhang and C. B. Liu, J. Phys. Chem. B, 2015, 119, 13398–13406 CrossRef CAS PubMed.
- G. Yang, E. A. Pidko and E. J. M. Hensen, ChemSusChem, 2013, 6, 1688–1696 CrossRef CAS PubMed.
- Y. Roman-Leshkov, M. Moliner, J. A. Labinger and M. E. Davis, Angew. Chem., Int. Ed., 2010, 49, 8954–8957 CrossRef CAS PubMed.
- T. Stahlberg, S. Rodriguez-Rodriguez, P. Fristrup and A. Riisager, Chem. – Eur. J., 2011, 17, 1456–1464 CrossRef CAS PubMed.
- Y. Y. Zhang, C. Liu and X. Chen, J. Anal. Appl. Pyrolysis, 2016, 119, 199–207 CrossRef CAS.
- R. J. van Putten, J. C. van der Waal, E. de Jong and C. B. Rasrendra,
et al.
, Chem. Rev., 2013, 113, 1499–1597 CrossRef CAS PubMed.
- J. M. Li, Z. C. Jiang, L. B. Hu and C. W. Hu, ChemSusChem, 2014, 7, 2482–2488 CrossRef CAS PubMed.
- H. F. Lin, J. Strull, Y. Liu and Z. Karmiol,
et al.
, Energy Environ. Sci., 2012, 5, 9773–9777 RSC.
- M. S. Holm, S. Saravanamurugan and E. Taarning, Science, 2010, 328, 602–605 CrossRef CAS PubMed.
- F. Parveen and S. Upadhyayula, Fuel Process. Technol., 2017, 162, 30–36 CrossRef CAS.
- Y. L. Wang, W. P. Deng, B. J. Wang and Q. H. Zhang,
et al.
, Nat. Commun., 2013, 4, 2141 CrossRef PubMed.
- J. J. Li, Y. R. Jing, C. B. Liu and D. J. Zhang, New J. Chem., 2017, 41, 8714–8720 RSC.
- L. W. Zhao, N. Elechi, R. Qian and T. B. Singhi-,
et al.
, J. Phys. Chem. A, 2017, 121, 1985–1992 CrossRef CAS PubMed.
- Y. B. Huang, T. Yang, Y. T. Lin and Y. Z. Zhu,
et al.
, Green Chem., 2018, 20, 1323–1334 RSC.
- R. Samuel, Y. Q. Pu, B. Raman and A. J. Ragauskas, Appl. Biochem. Biotechnol., 2010, 162, 62–74 CrossRef CAS PubMed.
- A. Rahimi, A. Ulbrich, J. J. Coon and S. S. Stahl, Nature, 2014, 515, 249–252 CrossRef CAS PubMed.
- M. R. Sturgeon, S. Kim, K. Lawrence and R. S. Paton,
et al.
, ACS Sustainable Chem. Eng., 2013, 2, 472–485 CrossRef.
- S. L. Qu, Y. F. Dang, C. Y. Song and J. D. Guo,
et al.
, ACS Catal., 2015, 5, 6386–6396 CrossRef CAS.
- W. Qin, L. N. Wu, Z. M. Zheng and C. Q. Dong,
et al.
, Molecules, 2014, 19, 21335–21349 CrossRef PubMed.
- J. Y. He, L. Lu, C. Zhao and D. H. Mei,
et al.
, J. Catal., 2014, 311, 41–51 CrossRef CAS.
- G. A. Oyedepo and A. K. Wilson, ChemPhysChem, 2011, 12, 3320–3330 CrossRef CAS PubMed.
- R. Reocreux, M. Huynh, C. Michel and P. Sautet, J. Phys. Chem. Lett., 2016, 7, 2074–2079 CrossRef CAS PubMed.
- G. H. Gu, C. A. Mullen, A. A. Boateng and D. G. Vlachos, ACS Catal., 2016, 6, 3047–3055 CrossRef CAS.
- Q. Li and N. Lopez, ACS Catal., 2018, 8, 4230–4240 CrossRef CAS.
- A. Yamaguchi, N. Mimura, M. Shirai and O. Sato, Sci. Rep., 2017, 7, 46172 CrossRef PubMed.
- D. N. Gao, Y. Xiao and A. Varma, Ind. Eng. Chem. Res., 2015, 54, 10638–10644 CrossRef CAS.
- J. Yi, T. He, Z. Jiang and J. Li,
et al.
, Chin. J. Catal., 2013, 34, 2146–2152 CrossRef CAS.
- L. X. Zhang, H. B. Yu, P. Wang and H. Dong,
et al.
, Bioresour. Technol., 2013, 130, 110–116 CrossRef CAS PubMed.
- X. D. Li, X. C. Lan and T. F. Wang, Green Chem., 2016, 18, 638–642 RSC.
- C. Sievers, I. Musin, T. Marzialetti and M. B. V. Olarte,
et al.
, ChemSusChem, 2009, 2, 665–671 CrossRef CAS PubMed.
- V. Choudhary, S. I. Sandler and D. G. Vlachos, ACS Catal., 2012, 2, 2022–2028 CrossRef CAS.
- M. H. Kucuk and A. Demirbas, Cellul. Chem. Technol., 1993, 27, 679–686 CAS.
- C. J. Deng, R. H. Liu and J. M. Cai, J. Energy Inst., 2008, 81, 211–217 CrossRef CAS.
- S. R. Wang, G. X. Dai, H. P. Yang and Z. Y. Luo, Prog. Energy Combust. Sci., 2017, 62, 33–86 CrossRef.
- B. V. Babu, Biofuels, Bioprod. Biorefin., 2008, 2, 393–414 CrossRef CAS.
- E. J. Cocinero, D. P. Gamblin, B. G. Davis and J. P. Simons, J. Am. Chem. Soc., 2009, 131, 11117–11123 CrossRef CAS PubMed.
- M. H. Zhang, Z. F. Geng and Y. Z. Yu, Energy Fuels, 2011, 25, 2664–2670 CrossRef CAS.
- V. Agarwal, P. J. Dauenhauer, G. W. Huber and S. M. Auerbach, J. Am. Chem. Soc., 2012, 134, 14958–14972 CrossRef CAS PubMed.
- Y. Y. Zhang, C. Liu and X. Chen, J. Anal. Appl. Pyrolysis, 2015, 113, 621–629 CrossRef CAS.
- X. L. Zhang, W. H. Yang and W. Blasiak, J. Anal. Appl. Pyrolysis, 2012, 96, 110–119 CrossRef CAS.
- X. L. Zhang, W. H. Yang and W. Blasiak, Fuel, 2012, 96, 383–391 CrossRef CAS.
- Y. Y. Jiang, H. Z. Yu and Y. Fu, Acta Chim. Sin., 2013, 71, 1611–1619 CrossRef CAS.
- M. H. Zhang, Z. F. Geng and Y. Z. Yu, Comput. Theor. Chem., 2015, 1067, 13–23 CrossRef CAS.
- T. D. Marforio, A. Bottoni, M. Calvaresi and D. Fabbri,
et al.
, ChemPhysChem, 2016, 17, 3948–3953 CrossRef CAS PubMed.
- M. Zheng, Z. Wang, X. X. Li and X. J. Qiao,
et al.
, Fuel, 2016, 177, 130–141 CrossRef CAS.
- S. Kim, S. C. Chmely, M. R. Nimlos and Y. J. Bomble,
et al.
, J. Phys. Chem. Lett., 2011, 2, 2846–2852 CrossRef CAS.
- J. B. Huang, C. He, C. Liu and H. Tong,
et al.
, Comput. Theor. Chem., 2015, 1054, 80–87 CrossRef CAS.
- J. B. Huang and C. He, J. Anal. Appl. Pyrolysis, 2015, 113, 655–664 CrossRef CAS.
- L. Chen, X. N. Ye, F. X. Luo and J. G. Shao,
et al.
, J. Anal. Appl. Pyrolysis, 2015, 115, 103–111 CrossRef CAS.
- M. Wang, C. Liu, X. X. Xu and Q. Li, Chem. Phys. Lett., 2016, 654, 41–45 CrossRef CAS.
- J. B. Huang, C. Liu, H. Tong and W. M. Li,
et al.
, Comput. Theor. Chem., 2012, 1001, 44–50 CrossRef CAS.
- J. B. Huang, C. He, L. Q. Wu and H. Tong, Chem. Phys. Lett., 2016, 658, 114–124 CrossRef CAS.
- Z. Y. Li, C. Liu, X. X. Xu and Q. B. Li, Comput. Theor. Chem., 2017, 1117, 130–140 CrossRef CAS.
- C. M. Wang, L. P. Guo, H. R. Li and Y. Wang,
et al.
, Green Chem., 2006, 8, 603–607 RSC.
Footnote |
† Co-first author. |
|
This journal is © The Royal Society of Chemistry 2019 |