DOI:
10.1039/C9FO01398A
(Paper)
Food Funct., 2019,
10, 6030-6041
Artificial intelligence identified peptides modulate inflammation in healthy adults†
Received
28th June 2019
, Accepted 8th August 2019
First published on 3rd September 2019
Abstract
Dietary bioactive peptides have been, among many functionalities, associated with immune modulation and thereby may improve resolution of inflammation. The goals of this research were to assess (1) whether specific peptides with immune-modulating activity consumed as part of a rice protein hydrolysate could be absorbed into blood and (2) whether they modulate inflammation markers. Artificial intelligence algorithms were applied to target, predict and unlock inflammation-modulating peptides from rice protein. A food application was developed containing four bioactive peptides. Protein docking simulation studies revealed high binding energies of these peptides with inflammation markers. In a small kinetic study 10 healthy subjects consumed the peptides with a single bolus of 20 g protein hydrolysate. Although absorption of the four predicted peptides at plasma concentrations deemed biologically relevant could not be confirmed (quantitative LC-MS/MS), several cytokines responded (ELISA kits). The 24-hour kinetic study revealed a slight suppression of pro-inflammatory TNF-α, IP-10 and NOx, whereas IL-6 increased temporarily (timepoints 2–12 hours). These markers returned to the baseline after 24 hours whereas others were not affected significantly (IL-10, hs-CRP, IL-8, and MCP-1). Consumption of a single dose protein hydrolysate containing immune modulatory peptides induced a mild temporary response most likely through intestinal signaling. Forthcoming studies will examine dietary supplementation in situations of stress.
Introduction
Bioactive peptides can be defined as specific protein sequences that impact human health.1 Where these amino acid sequences derive from common food proteins, the peptides can be considered bioactive food peptides, depending on the regulatory environment.2 Bioactive peptides have the potential to play a prominent role in human health by affecting the digestive system, endocrine functions, cardiovascular risk, immune response and nervous systems,3 and infant growth and development through several lactational stages.4 Publicly accessible databases such as Biopep,5 StraPep,6 STAPdb,7 PepBank8 and others continuously update the knowledge on biologically active food peptides with physiological significance beyond their pure nutritional value. Nevertheless, the relationship between bioactive peptide structural properties and physiological functionalities in most cases is still not completely elucidated.1
All dietary protein and their fragments are subject to digestion via upper intestinal enzymes, stomach acid, duodenal enzymes and mechanical forces, before they may be physiologically active in the intestine or absorbed into blood circulation. Different absorption mechanisms apply for peptides surviving digestion. For di- and tri-peptides permeation through intestinal membranes in their intact forms via peptide transporter systems has been demonstrated.9 Di- and tripeptides are thus the most readily absorbed form of protein-derived nitrogen.10 Mechanisms for the absorption of peptides with a medium chain length (4–20 amino acids) are less clear. Although transporter-mediated and vesicular transport systems have been described, there is little unequivocal evidence that medium chain peptides can cross the gut wall intact and enter the hepatic portal system in physiologically relevant concentrations.10
Humans are exposed to bioactive peptides either by the result of gastrointestinal digestion of food proteins that can generate physiologically active peptides or by the products of food processing. Human diets evolved with technology applying food maturation, fermentation and enzymatic processing using proteases to achieve food functionalities. In these cases peptides derive from protein hydrolysates.2 Alternatively, nutritional peptides can be synthesized using fermentative processes to match identical sequences found in food proteins.2
Protein hydrolysates today are usually produced for foods intended to facilitate digestion, to remove allergens, to achieve a specific taste or to preserve the food. Specific protein hydrolysates have been described to act on immune markers and typically contain immunomodulatory peptides up to 20 amino acids long and are hydrophobic.11 Modulation of immunity and inflammation has been ascribed to hydrolysates of animal origin e.g. whey, casein, chicken egg, salmon, tuna, carp, oyster and plant-based pea, soy, and wheat hydrolysates.11 Rice protein hydrolysates were among the early food products associated with the immune-modulating functionality. Oryzatensin, a nona peptide derived from the tryptic digest of rice albumin, was identified as a specific bioactive peptide with immune-modulating activity in vitro.12 Specific enzymatic hydrolysis of brown rice protein has recently been shown useful to unlock multifunctional properties and characteristic flavor profiles.13
Bioinformatic approaches are increasingly recognized in the discovery of bioactive food peptides.14 The approach allows one to discover and leverage structure–activity relationships observed between peptides and their potential effector interaction sites and thus enable in silico prediction of bioactive sequences encrypted in food proteins. Together, with peptidomics, bioinformatics approaches provide a rapid tool for predicting, profiling, and screening bioactive protein hydrolysates and peptides from food.15 In this study an artificial intelligence-based approach was applied to discover immune-modulating peptides in rice. A protein hydrolysate was developed using a common food process, and the predicted peptides conferring the immune-modulating functionality were quantified. In an early kinetic study healthy volunteers tolerated the hydrolysate well. Measurements showed that the immune-modulating predicted peptides remained under detection limits in blood, but selected immune markers indicated bioactivity in the subjects.
Materials and methods
Artificial intelligence approach to discover inflammation-modulating peptides
A proprietary predictive model was trained using three discrete data sources: (1) public unstructured (peer reviewed scientific papers and patents) and structured data (curated bioactivity annotations, biological pathways, structural annotations, and genomic and proteomic data) relevant to immunomodulation; (2) proprietary peptidomic data amassed through LC-MS/MS; and (3) phenotypic data accrued from experimental screening of proprietary peptides in various human cell lines. The resulting peptide knowledge base was used to train a predictive architecture via an ensemble of deep learning models.
The fully trained model was used to predict novel peptides with immunomodulatory potential from a large input set of peptide sequences of plant origin. Filtering strategies were applied to remove peptides with undesirable characteristics (i.e. a high toxicity profile) and those from non-commercially viable sources. Peptides exhibiting homology to known peptides were excluded. A set of four distinct, novel peptides from the rice proteome were selected for further study. Oryza sativa subspecies japonica homology searches were performed using BLASTP from the BLAST+16 suite of programs using the following parameters: word size = 2; matrix = PAM30, E-value = 10
000.
In silico valuation of peptide activity through docking/association energy calculations
The inflammation-modulating potential of the four predicted peptides was evaluated by a molecular modelling approach. Peptide docking was performed using FlexPepDock from the Rosetta software suite. Known 3D structures for 10 immunomodulatory targets of interest were retrieved from the Protein Data Bank (PDB), http://www.rcsb.org. They are as follows: TNF-α (PDB 4TWT), TNF-α-R1 (PDB 1FT4), TNF-α-R2 (PDB 1CA9), IL-6 (PDB 1ALU), IL-6R (PDB 5FUC), IL-10 (PDB 1Y6K), IL-10-R1 (PDB 1Y6K), IL-1β (PDB 5MVZ), MyD88 (PDB 3MOP) and TLR4 (PDB 3UL9).
The interacting interfaces as per PDB entries were used to set the initial pose for the tested peptides. Full flexibility was afforded to the peptides, and to the side chains of the immunomodulatory targets. A total of 1000 simulations were performed and the resulting models were ranked according to the lowest interface energy score (sum over energy contributed by interface residues of both interactors).
Production of the hydrolysate containing specific bioactive peptides
The hydrolysate was prepared from a food grade protein derived from a natural brown rice protein by hydrolysis in an aqueous dispersion using a food grade protease enzyme preparation. Specifically, for the hydrolysis the rice protein was dispersed in potable water and pasteurized at temperatures exceeding 80 °C. After cooling the pH value was adjusted to approx. 6.5 by using sodium hydroxide. Protease was added and hydrolyzation carried out for several hours in a stirred reactor under monitoring and control of temperature and pH-value. After the hydrolysis was finished the protease enzyme is inactivated by raising the temperature above 80 °C. The dispersion was spray-dried at air inlet temperatures >160 °C by a standard spray-drying process with fluid bed cooling. Procedures were performed under HACCP conditions. The spray dried powder was used as the study product.
Peptide analysis in the test product and blood samples
Processing of the rice protein hydrolysate for targeted peptide quantification.
Between 5 and 15 mg of the rice protein hydrolysate was first suspended in acetonitrile/water (1
:
1, v/v) and then diluted with 0.1% aqueous formic acid to a final concentration of 2 mg mL−1 rice protein and 5% acetonitrile. After centrifuging, 100 μL of the supernatant was mixed with an equal amount of internal standard solution. 20 μl of this mixture was analyzed by liquid chromatography-tandem mass spectrometry (LC-MS/MS).
Targeted peptide quantification by triple quadrupole LC-MS/MS.
Liquid chromatography was performed using a 13 minute gradient from 0 to 95% acetonitrile on an Aeris 1.7 μm Peptide XB-C18 150 × 2.1 mm reverse-phase column (Phenomenex) coupled to a QTRAP 5500 mass spectrometer (AB Sciex). Peptides were detected by scheduled multiple reaction monitoring (sMRM) of two or three mass transitions per peptide: SEEGYYGEQQQQPGMTR, IYGPDTGVDYKDNQMR, GYYGEQQQQPGMTR and FYNEGDAPVVAL. Chromatographic peaks corresponding to predicted peptides were integrated using Skyline version 3.5 (University of Washington). Isotope-labeled peptides with 13C,15N-labeled C-terminal leucine or arginine residues were synthesized at BASF Plant Science LP, Research Triangle Park, NC, USA and used as internal and calibration standards.
The performance of the analytical method was estimated using a dilution series of the isotope-labeled standards. The sample preparation steps were the same as for regular samples. The limit of detection (LOD) and limit of quantification (LOQ) for each peptide were estimated using a signal-to-noise (S/N) approach. The LOD and LOQ were defined as the standard concentrations which yield a peak with a S/N of ≥3 and ≥10, respectively. The linearity of the peak area plotted against the standard concentration was R2 > 0.99 for all peptides.
Untargeted peptide identification in blood samples by microflow LC-QTOF-MS/MS.
Human and rice peptides were identified in the plasma samples using an untargeted approach. To achieve maximum sensitivity, several samples were pooled after elution from the SPE plates (see above) and diluted to 5% acetonitrile before injection. Multiple injections per pooled sample were used to collect peptides on the trapping column. Fragmentation spectra from putative peptides were collected by data-dependent acquisition during a 25 min chromatographic gradient from 0% to 95% acetonitrile on a ZORBAX SB C18 5 μm, 150 mm × 0.3 mm column (Agilent) coupled to a TripleTOF 5600+ mass spectrometer (AB Sciex). Putative peptides were indicated by multiple charge states. Fragmentation spectra were converted to centroided peak lists in mascot generic format (MGF) before searching on a Mascot server installation (software version 2.6.1) against human and rice sequences downloaded from the Swiss-Prot sequence database (https://www.uniprot.org/).17
Peptides of interest were identified in a three-step approach.
(1) All acquired MS/MS spectra were searched against a custom database comprising highly abundant proteins typically found in human plasma (collagens, fibrinogens, albumins). This first step allowed for proline and lysine oxidation, which are typical modifications of these proteins. (2) All MS/MS spectra not assigned to any of these highly abundant proteins were searched against all human sequences from the Swiss-Prot sequence database. (3) All MS/MS spectra not assigned to any human sequence were searched against all rice sequences from the Swiss-Prot sequence database.
Assessment of resistance of predicted peptides to digestion
Simulated in vitro gastrointestinal digestion.
Simulated in vitro gastrointestinal digestion was performed on the rice protein hydrolysate according to the gastric and intestinal phases of the in vitro digestion method of Minekus et al.18 Briefly, the hydrolysate was mixed with simulated gastric fluid and pepsin for 2 h at pH 3 (gastric phase). The resultant gastric chyme was then mixed with pancreatin and porcine bile extract for 2 h at pH 7 to simulate intestinal transit (intestinal phase). Digested samples (gastric and intestinal) were analyzed by LC-MS/MS for peptide detection.
Processing of simulated in vitro gastrointestinal digestion samples for label-free peptide quantification.
Post GID samples were filtered through a 10 kDa MWCO filter (Vivaspin 500 centrifugal concentrators, Sigma Aldrich) to eliminate enzymes. Filtered samples were cleaned utilizing SPE (Oasis HLB cartridges, 10 mg sorbent, Waters) and lyophilized. Samples were reconstituted in Optima water (Fisher Scientific) to assess the peptide concentration (Pierce BCA protein concentration kit, ThermoFisher Scientific). An aliquot, containing 5 μg of peptides, was lyophilized and reconstituted in Optima water containing 0.1% TFA and 5 μg peptide standard mixture (Pierce Peptide Retention Time Calibration Mixture, ThermoFisher Scientific).
Label-free (relative) peptide quantification.
Liquid chromatography was performed using a 60 min gradient from 5 to 75% acetonitrile on an EasySpray reverse-phase column (ES804, ThermoFisher Scientific) coupled to a Q Exactive mass spectrometer (Thermo Fisher). Peptides were loaded on a trapping column and eluted over a 15 cm analytical column with a 1 h gradient at a flow rate of 300 nL min−1. The mass spectrometer was operated in the data-dependent mode, with MS and MS/MS performed in the Orbitrap at 70
000 FWHM and 17
500 FWHM resolution, respectively. From the MS scan, the fifteen most intense ions were selected for MS/MS. Fragmentation spectra from putative peptides collected by data-dependent acquisition were used for peptide identification. Relative quantification was performed by integrating precursor signal intensities using PEAKS software (Bioinformatics Solutions Inc., Waterloo, ON, Canada).19 Integrated signals from the four target peptides in the undigested rice protein hydrolysate were taken as 100%.
Clinical testing of peptide absorption and biological activity
The investigation was a single center, kinetic study, performed in orientation with the guidelines set forth by the International Council for Harmonization (ICH) Guidelines for Good Clinical Practice (GCP), and the Declaration of Helsinki regarding the treatment of human subjects. The study protocol was approved by the Institutional Review Board (IRB) of Landesärztekammer Baden-Württemberg. All subjects gave written informed consent. The study was registered with DRKS-ID: DRKS00014718.
Inclusion criteria.
Subject was able and willing to sign the informed consent form prior to screening evaluations, age: 18–50 years, BMI ≥19 and ≤30 kg m−2, non-smoker, subject was in good physical and mental health as established by medical history, physical examination, electrocardiogram, vital signs and results of biochemistry and hematology during the screening visit.
Exclusion criteria.
Relevant history or the presence of any severe medical disorder potentially interfering with this study (e.g. malabsorption, chronic gastro-intestinal diseases, heavy depression, diabetes, acute cancers within the last 3 years). Within the 3 months prior to the study significant cardiovascular disease events, changes in lifestyle or medication, surgical intervention, application of corticoids and other immune-suppressing drugs, or blood donation. Current vegan lifestyle, weight loss diet, regular intake of drugs or supplements possibly interfering with this study (e.g. omega-3, curcumin, protein shakes or bars) within 2 weeks prior to study start or during study, coffee consumption >3 cups per day, subjects with a history of drug, alcohol or other substance abuse, or other factors limiting their ability to co-operate during the study. Known HIV-infection, chronic hepatitis B and C infection, pregnancy, breast feeding or intention to become pregnant, or participation in another clinical study within the last 4 weeks.
Study setup.
The study consisted of a screening visit and a kinetic day over 24 h after a single dose intake. Furthermore, the subjects came the evening prior to the kinetic day to the study site and ate a standardized low protein meal. During kinetic day subjects were not allowed to consume any other foods and beverages than given by the study personnel. Screening for subject eligibility included a differentiated hemogram and clinical laboratory as well as a 12-lead ECG and a 3-day dietary record. 13 young to middle aged women and men (18–50 years) were screened for their eligibility in May 2018. A total of five women and five men were enrolled. All subjects received allocated intervention. Nobody was lost to follow up or discontinued intervention and all subjects were included in the analysis. Further details are summarized in the ESI (Fig. S1†).
At the morning of the kinetic day subjects received a permanent venous catheter for blood sampling during the study day. Blood sampling was performed directly after placement of the venous catheter (0 h) and 0.5 h, 1 h, 2 h, 3 h, 4 h, 8 h, and 12 h after intake of the study product, respectively. Directly after placement of the venous catheter, subjects received the study product. During the 24 h intervention period, subjects received standardized amounts of water and standardized meals (2 h, 5 h, 9 h, 11 h and 14 h post dosing). After the 12 h blood sampling subjects were released to go home and returned to the study site for the 24 h blood sampling. The clinical study was performed at the study center of BioTeSys GmbH in Esslingen, Germany. Plasma peptides were analyzed as described above.
Study product.
The study product was dissolved in 200 mL still water together with 15 mL sugar free syrup (lemon, raspberry or woodruff flavor, KClassic, Kaufland, Germany) to sweeten and flavor the product by stirring and was drunk immediately after baseline blood sampling. Subjects received 100 mL still water after intake. The study product was a peptide powder (20 g) containing the 4 peptides of interest as part of a native protein hydrolysate.
Analysis of blood routine and inflammatory response parameters.
The analysis of blood routine parameters and hsCRP was performed at Synlab MVZ Leinfelden-Echterdingen GmbH, Germany. The analysis of the parameters TNF-α, IL-6, IL-10, NOx (nitrate + nitrite), IP-10, IL-8 and MCP-1 was performed at BioTeSys GmbH in Esslingen, Germany. Briefly, enzyme immunoassays were used for the quantitative analyses of TNF-α (HSTA00E), IL-6 (HS600C), IL-10 (HS100C) and IP-10 (DIP100), all obtained from R&D Systems, Minneapolis, USA. IL-8 (ELH-IL8) and MCP-1 (ELH-MCP-1) from RayBiotech, Peachtree Corners Gerogia, USA. The ELISA tests were performed according to the respective instruction manuals. The sensitivities of the tests were indicated as 0.049 pg mL−1 (TNF-α), 0.09 pg mL−1 (IL-6), 0.17 pg mL−1 (IL-10), 4.46 pg mL−1 (IP-10), 1 pg mL−1 (IL-8) and 2 pg mL−1 (MCP-1), respectively. NOx was measured on behalf of a biochemical assay (Nitrite/Nitrate Assay Kit 23479-1KT-F, Merck Darmstadt, Germany).
Statistical methods
All efficacy parameters were evaluated in an exploratory manner. All data obtained in this study and documented in eCRFs were listed and summarized with descriptive statistics (N, arithmetic mean, standard deviation, minimum, 1st quartile, median, 3rd quartile, maximum) or frequency tables as appropriate. Changes over time were analyzed with parametric or non-parametric tests depending on distribution of data sets. Non-normality was evaluated with the Shapiro–Wilk test with a significance level of 0.10. Accordingly, ANOVA with repeated measures or the Friedman test was used. Additionally, pair wise tests versus the baseline (0 h) were performed using the paired t-test or Wilcoxon signed rank test, respectively. Statistical analysis was performed using the software GraphPad Prism. All statistical tests were performed two-sided with a significance level of 0.05.
Results
Properties of immune active peptides identified by artificial intelligence
Using a unique AI and machine learning approach, four novel peptides with predicted inflammation-modulating activity were identified from the proteome of Oryza sativa subsp. japonica. Physicochemical properties of the predicted peptides are displayed in Table 1. Peptides ranged in size from 12–17 amino acids and have either a net neutral or negative charge. Two peptides originate from the same region of alpha-globulin 138–154, while the parent proteins of the two remaining peptides are glutelin and granule-bound starch synthase 1, respectively (see Table 1 for parent protein Uniprot IDs).
Table 1 Physio-chemical properties and concentrations of bioactive peptides in the rice protein hydrolysate
Parent protein Uniprot ID |
Peptide sequence |
Length |
Molecular weight |
Global charge |
Concentration: measured in rice protein hydrolysate (mg kg−1) |
Batch L25848 |
Batch 0019552865 |
Batch 0020667018 |
P29835 |
SEEGYYGEQQQQPGMTR |
17 |
1988.01 |
−2 |
11.5 |
31.9 |
53.0 |
Q0DEV5 |
IYGPDTGVDYKDNQMR |
16 |
1871.97 |
−1 |
33.1 |
26.6 |
14.2 |
P29835 |
GYYGEQQQQPGMTR |
14 |
1642.72 |
0 |
7.0 |
13.4 |
18.9 |
Q0E261 |
FYNEGDAPVVAL |
12 |
1294.37 |
−2 |
13.6 |
15.6 |
6.1 |
|
|
|
|
Total |
65.2 |
87.5 |
92.2 |
Peptide docking with potential target protein sequences
Elevated levels of inflammatory cytokines are observed in response to exercise.20 Owing to the availability of 3D protein structures for various inflammatory targets involved in the exercise response, the interaction of the 4 predicted peptides with these key mediators was evaluated in silico via a molecular docking approach (Fig. 1). Two peptides (SEEGYYGEQQQQPGMTR and FYNEGDAPVVAL) revealed a high binding affinity to IL-6 with a normalized energy score of −11. In addition, peptide SEEGYYGEQQQQPGMTR revealed a high affinity to IL-10 and TNF-αR1. Fig. 2 illustrates the binding of FYNEGDAPVVAL to IL-6.
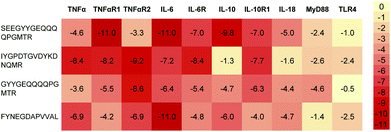 |
| Fig. 1 Heatmap representation of the binding affinity of 4 predicted peptides docked to 10 inflammation-modulating targets. The energy scores were normalized by the average score per target. | |
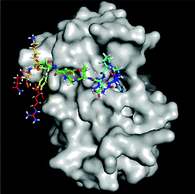 |
| Fig. 2 Docking simulation of predicted peptide FYNEGDAPVVAL against IL-6. Molecular graphics were generated using the molecular visualization software PyMOL (the PyMOL Molecular Graphics System, Version 1.5.03, Schrödinger, LLC). | |
Protein hydrolysate containing specific bioactive peptides
The study product was a beige powder with light vegetable smell and a solubility of 30–40% in water. The typical nutritional composition is: energy (kJ per 100 g) 1550–1680 and (g per 100 g) protein 80, carbohydrates < 1, moisture < 5, ash < 5, total fat 3–6, dietary fiber 5–10.
Concentrations of AI (artificial intelligence) identified peptides in rice
In silico search algorithms predicted rice protein as a source of four TNF-α modulating peptides. LC-MS/MS analysis of the rice protein hydrolysate confirmed that the predicted peptides were present in the study product (Table 1). Expectedly, the concentrations in the hydrolysate were much lower than the theoretical concentrations calculated from rice protein expression data.
Digestibility of the predicted peptides
Using a simulated in vitro digestion approach, the ability of the four AI-predicted peptides to resist proteolytic digestion was investigated. Relative peptide abundances were assessed by label-free quantification in gastric digested samples in relation to the undigested rice protein hydrolysate: SEEGYYGEQQQQPGMTR, 8%; IYGPDTGVDYKDNQMR, 20%; GYYGEQQQQPGMTR, >100%; and FYNEGDAPVVAL, 24%. Samples were adjusted to contain equal amounts of total soluble peptides before the analysis. All four target peptides continue to be detected in the gastric digested sample, and thus demonstrate their (partial) resistance to modulated proteolysis by gastric cleavage. As expected, concentrations of peptides SEEGYYGEQQQQPGMTR, IYGPDTGVDYKDNQMR, and FYNEGDAPVVAL decreased during gastric digestion. The increase in GYYGEQQQQPGMTR may have been generated from the hydrolysis of insoluble parts of the rice protein hydrolysate. None of the four target peptides was detectable at the end of the full gastrointestinal protocol, suggesting that peptides may have interacted with small intestinal response elements early in the digestive process.
Kinetic study
A first small study with healthy volunteers was performed applying a single bolus of the rice protein hydrolysate to probe (1) whether predicted peptides are absorbed into blood and (2) whether they modulate inflammation markers.
Study subjects
On average, women (n = 5) were slightly older (38.2 years, 95% CI: 25.4–51.0) than men (n = 5, 33.2 years, 95% CI: 19.4–47.0), and had a slightly lower BMI 23.1 kg m−2 (95% CI: 19.1–27.0) than male subjects (BMI 25.7 kg m−2, 95% CI: 24.3–27.1). Thus, 60% of the female and 40% of the male subjects had a BMI of <25 kg m−2, whereas 40% of the female subjects and 60% of the male subjects showed BMI levels between 25–30 kg m−2, classified as overweight. However, in this study men were very well-trained explaining for some of them the BMI within the category “overweight”. All study participants were healthy based on the assessment of medical history and lifestyle, engaged regularly in exercise activities, and reported in a 3-day dietary record an energy intake of 7.5 MJ for women and 10.4 MJ for men, below the German Society for Nutrition recommendation.
Peptide detection in blood
One of the objectives of the study was to determine absorption of the four peptides (Table 1) as part of the study product consumed by subjects, determine absorption rate, time of maximum blood concentration (Tmax), 24 h AUC, half-time and elimination. Since none of the selected peptides was detected in any of the blood samples, defined pharmacokinetic parameters could not be evaluated.
This raised the question whether there was any physiologically significant uptake of the selected peptides into the circulation. The limit of detection (LOD) in human plasma was determined by spiking experiments with isotope-labeled synthetic peptides: SEEGYYGEQQQQPGMTR, 55 pM; IYGPDTGVDYKDNQMR, 55 pM; GYYGEQQQQPGMTR, 110 pM; FYNEGDAPVVAL, <28 pM. These concentrations are comparable to the typical concentrations of selected gastrointestinal peptides reported in the literature: insulin, 5735 Da, 50–80 pM; cholecystokinin, 3918 Da, 50–100 pM; ghrelin, 3371 Da, 60–450 pM.21,22
The absence of the selected peptides at the indicated LOD raised further the question whether any partial sequences (e.g. resulting from partial digestion in the stomach) would be detectable in the blood samples. However, also an untargeted peptidomics approach failed to detect the selected peptides or any of their partial sequences. Instead, other unrelated peptides originating from rice proteins were detected. Homeobox-leucine zipper protein TF1 (UniProt accession Q5ZAY0)17 and β-galactosidase 5 (Q10RB4) were represented by 2–3 peptides each in samples from different volunteers and at several time points after the intake of the rice protein hydrolysate. While the determination of the LOD is not possible for an untargeted approach, the sensitivity of the analytical method was confirmed by the detection of peptide fragments of several low-abundancy human proteins (UniProt accession, typical concentrations from literature references): prothrombin, P00734, 1.2 ng mL−1; multimerin-1, Q13201, 7.2 ng mL−1; osteocalcin, P02818, 2–15 ng mL−1; insulin C-peptide, P01308, 0.8–3.9 ng mL−1; neurosecretory protein VGF, O15240, 3 ng mL−1; neurogranin, Q92686, 1.4 ng mL−1.23–26 Taken together, the selected peptides were absent from blood samples at physiologically relevant concentrations down to the lower picomolar range.
Tumor necrosis factor alpha (TNF-α)
The other objective was to monitor inflammation parameters in the blood over the 24-hour period. Considering the healthy subject group, the highly sensitive TNF-α assay was used to measure the low concentrations. Despite the low TNF-α levels an overall significant reduction of TNF-α (P = 0.0062) was seen with on average minimum concentrations at 8 h after the intake of the study product and then returning to the baseline. Pair-wise tests versus the baseline did not indicate a significant reduction, however, significant changes were seen for 0.5 h vs. 8 h (P = 0.0024) and 0.5 h vs. 12 h (P = 0.0319) (Fig. 3).
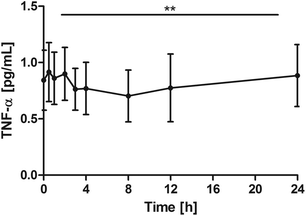 |
| Fig. 3 Distribution of TNF-α [pg mL−1] at the baseline and after the intake of rice peptides (mean ± 95% CI); **P = 0.0062. | |
Interleukin-6 (IL-6)
All subjects showed a distinct increase of serum IL-6, with different individual maxima between 2 and 12 hours. On average, Cmax was 10.8 ± 6.4 pg mL−1. Changes over time were significant (P = 0.0002) with significant pair wise tests at: 0 h vs. 2 h, P = 0.0039; 0 h vs. 3 h, P = 0.0039; 0 h vs. 4 h, P = 0.0098; 0 h vs. 8 h, P = 0.0371. After 24 h, IL-6 returned to baseline levels (Fig. 4).
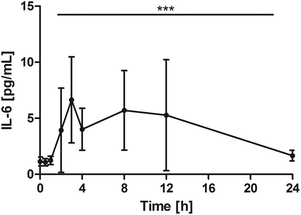 |
| Fig. 4 Distribution of IL-6 [pg mL−1] at the baseline, and after the intake of rice peptides (mean ± 95% CI); ***P = 0.0002. | |
TNF-α vs. IL-6 ratio
Changes over time were highly significant (P < 0.0001). Pair-wise tests indicated that the ratio significantly declined in comparison with the baseline from 2 h onwards (0 h vs. 2 h: P = 0.0039; 0 h vs. 3 h: P = 0.0098; 0 h vs. 4 h: P = 0.0039; 0 h vs. 8 h: P = 0.0195; 0 h vs. 12 h: P = 0.0273). After 24 h, the ratio returned towards baseline conditions (Fig. 5).
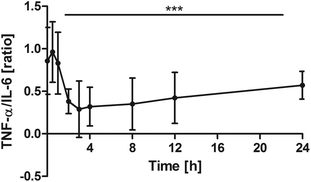 |
| Fig. 5 Distribution of TNF-α/IL-6 [ratio] at the baseline and after the intake of rice peptides (mean ± 95% CI); ***P < 0.0001. | |
Interferon γ-induced protein 10 (IP-10 or CXCL10)
Blood samples for IP-10 were taken at the baseline, 12 h and 24 h. Values for most subjects decreased slightly between the baseline and the 12 h sampling time point. Changes over time were significant (P = 0.0005). Pair-wise tests confirmed the significant reduction at 12 h (P = 0.0195) and return to the baseline at 24 h (12 h vs. 24 h: P = 0.0020; Fig. 6).
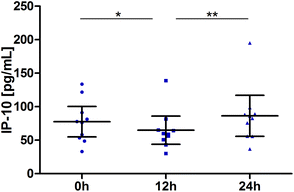 |
| Fig. 6 Distribution of IP-10 [pg mL−1] at the baseline, after 12 h and 24 h (scatter diagram with mean ± 95% CI); *P < 0.05, **P < 0.01. | |
Nitrogen oxides (NOx)
NOx levels significantly declined over time (P < 0.0001). The pair-wise tests against the baseline confirmed a significant reduction from 1 h through 8 h and additionally at post 24 h (0 h vs. 0.5 h: P = 0.0645; 0 h vs. 1 h: P = 0.0078; 0 h vs. 2 h: P = 0.0137; 0 h vs. 3 h: P = 0.0059; 0 h vs. 4 h: P = 0.0020; 0 h vs. 8 h: P = 0.0020; 0 h vs. 24 h: P = 0.0371, Fig. 7).
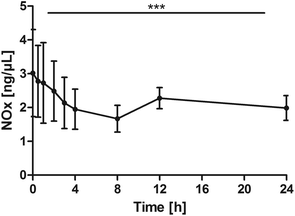 |
| Fig. 7 Distribution of NOx [ng μL−1] at the baseline, and after the intake of rice peptides (mean ± 95% CI); ***P < 0.0001. | |
Interleukin-8 (IL-8), monocyte chemoattractant protein 1 (MCP-1) and high-sensitive C-reactive protein (hsCRP)
On average, IL-8 was determined with values between 18.2 pg mL−1 and 25.6 pg mL−1 with no significant changes over time (P = 0.5659, data not shown). The same applies to MCP-1 with on average levels between 9.1 pg mL−1 and 12.9 pg mL−1 at the different time points. Over the observation period of 24 h, no significant changes were observed after the intake of the rice protein hydrolysate (P = 0.7945).
hsCRP is an acute phase protein which occurs during the inflammatory response. An increase is expected within 6–12 h after the start of acute inflammation. Besides this, hsCRP is also used as a sensitive systemic marker of inflammation and risk factor e.g. for cardiovascular disease. Serum concentrations of hsCRP were <0.1 mg dL−1 for most study participants and at the lower healthy level for the other participants indicating that in none of the subjects acute inflammation was present. The hsCRP levels were very stable at all three assessment time points (0 h, 12 h and 24 h) and characteristic for subjects.
Discussion
The way to AI discovered bioactive peptides
In this work, we demonstrate the value of AI and machine learning in unlocking therapeutic potential from food sources. By using existing public scientific information, and bespoke peptidomic and phenotypic data as training sets, an AI predictive architecture was capable of learning key underlying features relevant for targeting inflammation. When applied to a large set of plant data, this predictive model identified four peptides from the rice proteome with suitable inflammation-modulating properties. Informed by predicted peptide sequences, intelligent hydrolysis strategies were used to unlock the predicted peptides, and in turn their therapeutic potential from rice.
Peptides interact with inflammation relevant markers
Inflammation has traditionally been viewed as detrimental for recovery from physical exercise. More knowledge is being gathered to understand how tightly regulated inflammatory responses are essential to muscle repair and regeneration, and how cytokines are relevant in this process of recovery.27In silico molecular docking studies can help to further elucidate interaction of the predicted peptide sequences in the relevant physiological pathways.28 The analysis of docking energies of the predicted peptides with inflammation relevant cytokines and receptors (Fig. 1) suggests different levels of direct peptide interaction in silico. For example, peptide SEEGYYGEQQQQPGMTR showed the highest affinity with TNF-α receptor 1, IL-6 and IL-10, IYGPDTGVDYKDNQMR showed affinity with TNF-α receptor 2 and FYNEGDAPVVAL showed a high binding affinity to IL-6 (Fig. 2). Thus, the overall interaction with IL-6 seemed the most pronounced, but multiple docking affinities indicate that in sum the peptides interact simultaneously through several mechanisms.
Interestingly, volunteers in this study also appeared to respond to the dietary intake of the hydrolysate with a temporary increase in IL-6 blood concentrations even though it is unlikely that the peptides physically encountered IL-6 in the blood. Relatively high docking energies were also calculated between SEEGYYGEQQQQPGMTR and IL-10, with the latter not changing in the volunteers. However, because of the healthy subject group, cytokine levels of IL-10 were overall very low and in the range of the detection limit for quantification which does not allow final conclusions.
From in silico peptides to a nutritional product
Exact mechanisms by which bioactive dietary peptides affect human physiology are often insufficiently understood.11 The opportunity of achieving health benefits from a brown rice protein hydrolysate produced using a food source and common food enzymes applied in a specific process led us to the development of a food ingredient. The predicted peptides in this study served as a quantitative quality parameter for the functional protein hydrolysate. The process was optimized to improve the yield of predicted peptides to about 1 mg per 10 g product or 0.01% w/w at the commercial scale, whereas the early product applied in the clinical kinetic study yielded 0.7 mg per 10 g product (Table 1). Protein hydrolysates contain a wide array of peptides.3 For the test product in this study AI algorithms identified the most active peptide motifs in rice. It is very likely that beyond our predicted peptides a larger number of peptides contributed to physiological effects of the bioactive protein hydrolysates.29
Early clinical observations
A clinical kinetic study was performed with 10 healthy men and women to assess whether the immune-modulating peptides, discovered by AI, are absorbed and whether oral intake of the protein hydrolysate containing them affects immune markers. Although low concentrations of rice protein specific peptides could be detected in the plasma of the volunteers, the predicted peptides could not be quantified at concentrations expected for peptide hormones, such as fasting insulin (40–80 pM)30 or total ghrelin (60–120 pM).31 Selected markers of immunity, however, responded suggesting the physiologically relevant nutritional bioactivity of peptides within the protein hydrolysate tested.
The bolus of 20 g protein hydrolysate applied in this study contained 1.3 mg of predicted peptides. Assuming for a model calculation a 1% absorption rate of the peptide with the highest concentration (IYGPDTGVDYKDNQMR, 0.6 mg per 20 g study product) from the intestinal lumen unhydrolyzed into the systemic circulation, the concentration in the blood would be approximately 1 μg L−1 (assuming distribution into 6 L blood) or 0.5 nM (assuming MW 1988, Table 1). The detection limit (LOQ) was 0.05 nM, thus well below the theoretical concentration at 1% absorption.
Under normal human digestion conditions peptides derive from the hydrolysis of larger proteins mostly through acid hydrolysis in the stomach and through a variety of proteases in the duodenum. Transporters for di- and tripeptides have been well established as an efficient mechanism of absorption into the gastrointestinal enterocytes and a fraction of these peptides then enter the blood circulation.32,33 However, to what extent larger peptides enter the circulation is still controversial.9
In vitro results suggest that predicted peptides were resistant to gastric acid, but only partly to intestinal enzymatic digestion in the standardized static model applied.18 This indicates that any peptide activity observed in the clinical study must have happened early in the digestion process through receptors in the upper gastrointestinal tract.
Indeed, changes in blood cytokines TNF-α, IL-6 and IP-10, and in NOx were observed after consumption of the 20 g protein hydrolysate containing bioactive peptides. Although the molecular inflammatory cascade in the gut is still insufficiently understood some of the characterized intestinal receptors may have been involved in the molecular signaling. Vasoactive intestinal peptide receptor 1 (VPAC1) appears to be the predominant receptor with high levels in the small intestine and the colon.34 Apical VPAC1 in intestinal epithelial cells are considered to be relevant in recognizing dietary peptides35 and may therefore respond to the inflammation-modulating peptides tested in this study. However, the role of VPAC1 with respect to the predicted peptides has to be further elucidated.36 Our slightly bitter tasting rice peptides may have addressed intestinal G-protein-coupled receptor based signaling elements.37 These bitter receptors were described to activate multiple health related signaling pathways, at low concentrations of the active substance, without requiring uptake into the body.
Similarly, chemokine receptor type 9 (CCR9), also designated CDw199, and chemokine receptor 3 from the CXC chemokine receptor family (CXCR3) are expressed in the small intestine and constitute principal targets for anti-chemokine therapy in inflammatory bowel disease.38 Moreover, food-derived opioid peptides may be involved in the regulation of inflammatory processes.39 Intestinal Mu opioid receptors are well described as responding to peptides in their opioid function and a variety of other functionalities including hormone-like, analgesia, and anti-hypertensive effects.40 Peptides from bovine, casein and soy protein were characterized for their functionality.41,42 For example, dietary A1 β-casein peptides were associated with inflammatory markers in humans.43 β-Casein peptides modulated immunoglobulins and cytokines through the gut, but in contrast to our study, effects on IL-6 were not reported. Additionally, multiple protein hydrolysates were found to affect Toll-like receptors (TLRs), but the effects were mostly hydrolysate-dependent rather than focused on individual peptides.11 Next to the potential of receptor binding, immunomodulatory effects of the dietary peptides can also be mediated by direct uptake via the peptide transporter or by endocytosis.
Inflammation markers respond to dietary peptides
Cytokines are key modulators of inflammation, participating in acute and chronic inflammation via a complex network of interactions which complicate interpretation.44 To get a comprehensive picture of possible effects a broad set of cytokines was selected in the pilot study. Overall, cytokines measured in the healthy collective in our kinetic study were rather low. Nevertheless, data support an immune-modulatory reaction described mainly by IL-6, TNF-α, IP-10 and NOx after the intake of the rice protein hydrolysate under fasting conditions. Other cytokines were not changed in the healthy study collective. Of note, the rice hydrolysate was provided without the combination of an immunomodulating stimulus.
TNF-α is an important cytokine involved in the regulation of immune cells where it plays a central role in inflammation. TNF-α is produced by a wide variety of immune, epithelial, endothelial, and tumor cells. It facilitates pro- and anti-inflammatory signaling in the intestinal epithelium.45 After the intake of the rice protein hydrolysate, a decrease of TNF-α, IP-10 and NOx was observed in contrast to a significant increase of IL-6 values. In an early innate immune response, an increase of TNF-α followed by elevated IL-6 levels would have been expected.46 As there was rather a reduction of TNF-α (Fig. 3), the increase of IL-6 (Fig. 4) seems to be triggered by a TNF-α-independent pathway.
The data do not allow conclusions about cells responsible for the release of IL-6. But TNF-α production may in turn be negatively affected by the increase of IL-6. IL-6 can act as a proinflammatory cytokine playing important roles in acute phase reaction, inflammation, and hematopoiesis.47 Normal human circulating IL-6 averages around 1 pg mL−1
48 with slight elevation during the menstrual cycle,49 in line with our baseline data (Fig. 4). IL-6 along with TNF-α drives the acute inflammatory response. It is important in the transition from acute inflammation to either acquired immunity or chronic inflammatory disease. However, IL-6 can also function as an anti-inflammatory molecule, as in skeletal muscle where it is secreted in response to exercise.46 In exercise physiology IL-6 has been associated with physical recovery and inflammation resolving functionality.50
Further immunomodulatory stimuli are reported in the literature, e.g. a postprandial recruitment of neutrophils has demonstrated an increased leukocyte count in response to postprandial lipidemia (fat load with 50 g m−2), which was accompanied by increased cytokine levels and impaired endothelial function.51,52 Moreover, postprandial recruitment of neutrophils and IL-6 in response to a mixed meal composed of fat and glucose appear much lower than to fat alone.52 Lundman et al.53 demonstrated that a lipid challenge does not affect plasma IL-6 for the first 2 h postprandially and reached on average less than a 2-fold increase, whereas increases in our study were on average 5-fold (Fig. 4). A negative or no response in circulating inflammatory mediators to a bolus of dietary macronutrients was recently confirmed.54 Proteins exerted an even weaker influence on postprandial inflammation compared with carbohydrates and lipids. After the 2 h time point subjects of the current study received a breakfast consisting of a pretzel (white bread) with less than 20 g butter. The profiles of all measured cytokines and NOx indicate that responses already started before or at the 2 h time point and thus before breakfast was consumed (Fig. 4, 5 and 7).
Next to the neutrophil increase, a significant meal-independent rise in lymphocytes was observed in the study of van Oostrom et al.52 suggesting a diurnal rhythm. Anyhow, both reported impact factors do not explain the distinct IL-6 increase in our study and we rather suggest the IL-6 increase as a response to the dietary protein hydrolysate intake. However, observations in our kinetic study lacked a placebo control and we cannot exclude effects/interactions with other food consumption or diurnal shifts on blood inflammation markers. Worth mentioning is the temporal shift returning to baseline conditions within 24 h. Our observations do not allow any conclusions about long term shifts. Still, immunomodulatory effects observed are beyond other reported responses from macronutrients.54 Thus, this pilot study gives first proof of concept and effects will further be investigated with adequate controls. In this context, an adequate control needs to be thoroughly discussed e.g. other peptides of non-rice origin, non-hydrolyzed rice proteins, or other macronutrients. Moreover, these observations were made in rested individuals and will have to be verified during conditions of exercise stress.
Within the study, the greatest impact was seen on IL-6. IL-6 is involved both in innate and adaptive immunity and thus as a multifunctional cytokine not only involved in recovery from sporting activities,55 but also involved in different pathological conditions such as gastrointestinal disorders or rheumatoid arthritis, diabetes and cardiovascular disease.47,50 The immunomodulatory properties of the specific protein hydrolysates under such conditions deserve additional investigation. The work presented outlines a targeted approach and provides promising first proof of concept for the immunomodulatory properties of predicted peptides encouraging further research.
Conclusions
Protein hydrolysates have previously been shown to have functionalities beyond providing protein building blocks in the form of easily absorbable amino acids. We demonstrate the targeted discovery of bioactive peptides using a predictive AI approach. A rice protein-based food ingredient containing four predicted peptides was developed and clinically tested for bioefficacy. Results from this first proof of concept approach confirmed our in silico observation that the peptides may modulate inflammation markers. Such properties are of interest for application areas like sports nutrition, but can also be developed into applications benefitting target groups with wider inflammatory conditions.
Conflicts of interest
The investigators were at the time of study execution employees of BASF SE, D.R.; BASF Metabolome Solutions GmbH, P.T. and R.D.; BASF Personal Care and Nutrition GmbH, J.G. and T.H.; and BioTeSys GmbH, C.S.
Acknowledgements
The authors thank Nuritas, Ltd, Dublin, Ireland for providing data on the artificial intelligence approach, the discovery of inflammation-modulating peptides, the in silico valuation of peptide docking/association, and the assessment of resistance of predicted peptides to digestion, as well as for helpful discussions. Sincere gratitude is dedicated to Lucyna Wild, Johanna Schäfer and the BioTeSys team for their valuable advice and support contributing to the analytical and clinical success of the study. The study was financially supported by BASF SE, Germany. The planning and organization of the study as well as data analyses were performed solely by the investigators.
References
- D. D. Kitts and K. Weiler, Bioactive proteins and peptides from food sources. Applications of bioprocesses used in isolation and recovery, Curr. Pharm. Des., 2003, 9, 1309–1323 CrossRef CAS PubMed.
- S. Chakrabarti, S. Guha and K. Majumder, Food-Derived Bioactive Peptides in Human Health: Challenges and Opportunities, Nutrients, 2018, 10, pii: E1738 CrossRef PubMed.
- A. Sanchez and A. Vazquez, Bioactive Peptides: A Review, Food Qual. Saf., 2017, 1, 29–46 CrossRef CAS.
- K. A. Dingess, W. M. de, S. Boeren, J. Vervoort, T. T. Lambers, J. B. van Goudoever and K. Hettinga, Human milk peptides differentiate between the preterm and term infant and across varying lactational stages, Food Funct., 2017, 8, 3769–3782 RSC.
- P. Minkiewicz, J. Dziuba, A. Iwaniak, M. Dziuba and M. Darewicz, BIOPEP database and other programs for processing bioactive peptide sequences, J. AOAC Int., 2008, 91, 965–980 CAS.
- J. Wang, T. Yin, X. Xiao, D. He, Z. Xue, X. Jiang and Y. Wang, StraPep: a structure database of bioactive peptides, Database, 2018, 2018 Search PubMed.
- S. Singh, K. Chaudhary, S. K. Dhanda, S. Bhalla, S. S. Usmani, A. Gautam, A. Tuknait, P. Agrawal, D. Mathur and G. P. Raghava, SATPdb: a database of structurally annotated therapeutic peptides, Nucleic Acids Res., 2016, 44, D1119–D1126 CrossRef CAS PubMed.
- T. Shtatland, D. Guettler, M. Kossodo, M. Pivovarov and R. Weissleder, PepBank–a database of peptides based on sequence text mining and public peptide data sources, BMC Bioinf., 2007, 8, 280 CrossRef PubMed.
- W. Shen and T. Matsui, Current knowledge of intestinal absorption of bioactive peptides, Food Funct., 2017, 8, 4306–4314 RSC.
- W. M. Miner-Williams, B. R. Stevens and P. J. Moughan, Are intact peptides absorbed from the healthy gut in the adult human?, Nutr. Res. Rev., 2014, 27, 308–329 CrossRef CAS PubMed.
- M. B. G. Kiewiet, M. M. Faas and P. de Vos, Immunomodulatory Protein Hydrolysates and Their Application, Nutrients, 2018, 10, pii: E904 CrossRef PubMed.
- M. Takahashi, S. Moriguchi, M. Yoshikawa and R. Sasaki, Isolation and characterization of oryzatensin: a novel bioactive peptide with ileum-contracting and immunomodulating activities derived from rice albumin, Biochem. Mol. Biol. Int., 1994, 33, 1151–1158 CAS.
- O. Selamassakul, N. Laohakunjit, O. Kerdchoechuen and K. Ratanakhanokchai, A novel multi-biofunctional protein from brown rice hydrolysed by endo/endo-exoproteases, Food Funct., 2016, 7, 2635–2644 RSC.
- D. Agyei, A. Tsopmo and C. C. Udenigwe, Bioinformatics and peptidomics approaches to the discovery and analysis of food-derived bioactive peptides, Anal. Bioanal. Chem., 2018, 410, 3463–3472 CrossRef CAS PubMed.
- T. A. V. Holton, V. Vijayakumar and N. Khaldi, Bioinformatics: Current perspectives and future directions for food and nutritional research facilitated by a Food-Wiki database, Trends Food Sci. Technol., 2013, 34, 5–17 CrossRef CAS.
- C. Camacho, G. Coulouris, V. Avagyan, N. Ma, J. Papadopoulos, K. Bealer and T. L. Madden, BLAST+: architecture and applications, BMC Bioinf., 2009, 10, 421 CrossRef PubMed.
- C. UniProt, UniProt: a worldwide hub of protein knowledge, Nucleic Acids Res., 2019, 47, D506–D515 CrossRef PubMed.
- M. Minekus, M. Alminger, P. Alvito, S. Ballance, T. Bohn, C. Bourlieu, F. Carriere, R. Boutrou, M. Corredig, D. Dupont, C. Dufour, L. Egger, M. Golding, S. Karakaya, B. Kirkhus, S. Le Feunteun, U. Lesmes, A. Macierzanka, A. Mackie, S. Marze, D. J. McClements, O. Menard, I. Recio, C. N. Santos, R. P. Singh, G. E. Vegarud, M. S. Wickham, W. Weitschies and A. Brodkorb, A standardised static in vitro digestion method suitable for food - an international consensus, Food Funct., 2014, 5, 1113–1124 RSC.
- B. Ma, K. Zhang, C. Hendrie, C. Liang, M. Li, A. Doherty-Kirby and G. Lajoie, PEAKS: powerful software for peptide de novo sequencing by tandem mass spectrometry, Rapid Commun. Mass Spectrom., 2003, 17, 2337–2342 CrossRef CAS PubMed.
- K. Ostrowski, T. Rohde, S. Asp, P. Schjerling and B. K. Pedersen, Pro- and anti-inflammatory cytokine balance in strenuous exercise in humans, J. Physiol., 1999, 515(Pt 1), 287–291 CrossRef CAS PubMed.
- D. Crona and R. MacLaren, Gastrointestinal hormone concentrations associated with gastric feeding in critically ill patients, JPEN, J. Parenter. Enteral Nutr., 2012, 36, 189–196 CrossRef CAS PubMed.
- B. Keselman, M. Vergara, S. Nyberg and F. H. Nystrom, A randomized cross-over study of the acute effects of running 5 km on glucose, insulin, metabolic rate, cortisol and Troponin T, PLoS One, 2017, 12, e0179401 CrossRef PubMed.
- F. Capra, M. Casaril, G. B. Gabrielli, A. Stanzial, S. Ferrari, G. Gandini, G. Falezza and R. Corrocher, Plasma osteocalcin levels in liver cirrhosis, Ital. J. Gastroenterol., 1991, 23, 124–127 CAS.
- Y. Chai, J. Wang, Y. Gao, T. Wang, F. Shi, J. Su, Y. Yang, X. Zhou, L. Song and Z. Liu, Identification of biomarkers for radiation-induced acute intestinal symptoms (RIAISs) in cervical cancer patients by serum protein profiling, J. Radiat. Res., 2015, 56, 134–140 CrossRef CAS PubMed.
- Z. Liu, S. Fan, H. Liu, J. Yu, R. Qiao, M. Zhou, Y. Yang, J. Zhou and P. Xie, Enhanced Detection of Low-Abundance Human Plasma Proteins by Integrating Polyethylene Glycol Fractionation and Immunoaffinity Depletion, PLoS One, 2016, 11, e0166306 CrossRef PubMed.
- A. De Vos, M. Bjerke, R. Brouns, N. De Roeck, D. Jacobs, L. Van den Abbeele, K. Guldolf, H. Zetterberg, K. Blennow, S. Engelborghs and E. Vanmechelen, Neurogranin and tau in cerebrospinal fluid and plasma of patients with acute ischemic stroke, BMC Neurol., 2017, 17, 170 CrossRef PubMed.
- J. M. Peake, O. Neubauer, P. A. Della Gatta and K. Nosaka, Muscle damage and inflammation during recovery from exercise, J. Appl. Physiol., 2017, 122, 559–570 CrossRef CAS PubMed.
- S. Chen, Z. Feng, Y. Wang, S. Ma, Z. Hu, P. Yang, Y. Chai and X. Xie, Discovery of Novel Ligands for TNF-alpha and TNF Receptor-1 through Structure-Based Virtual Screening and Biological Assay, J. Chem. Inf. Model., 2017, 57, 1101–1111 CrossRef CAS PubMed.
- M. B. G. Kiewiet, R. Dekkers, M. P. van Gool, L. H. Ulfman, A. Groeneveld, M. M. Faas and P. de Vos, Identification of a TLR2 Inhibiting Wheat Hydrolysate, Mol. Nutr. Food Res., 2018, 62, e1800716 CrossRef PubMed.
- M. E. Daly, C. Vale, M. Walker, A. Littlefield, K. G. Alberti and J. C. Mathers, Acute effects on insulin sensitivity and diurnal metabolic profiles of a high-sucrose compared with a high-starch diet, Am. J. Clin. Nutr., 1998, 67, 1186–1196 CrossRef CAS PubMed.
- E. Korek, H. Krauss, M. Gibas-Dorna, J. Kupsz, M. Piatek and J. Piatek, Fasting and postprandial levels of ghrelin, leptin and insulin in lean, obese and anorexic subjects, Przegl. Gastroenterol., 2013, 8, 383–389 CrossRef CAS.
- F. Rohm, T. Skurk, H. Daniel and B. Spanier, Appearance of di- and Tripeptides in Human Plasma after a Protein Meal does not Correlate with PEPT1 Substrate Selectivity, Mol. Nutr. Food Res., 2018, e1801094, DOI:10.1002/mnfr.201801094.
- M. Foltz, E. E. Meynen, V. Bianco, C. van Platerink, T. M. Koning and J. Kloek, Angiotensin converting enzyme inhibitory peptides from a lactotripeptide-enriched milk beverage are absorbed intact into the circulation, J. Nutr., 2007, 137, 953–958 CrossRef CAS PubMed.
- D. Jayawardena, G. Guzman, R. K. Gill, W. A. Alrefai, H. Onyuksel and P. K. Dudeja, Expression and localization of VPAC1, the major receptor of vasoactive intestinal peptide along the length of the intestine, Am. J. Physiol.: Gastrointest. Liver Physiol., 2017, 313, G16–G25 CrossRef PubMed.
- A. J. Harmar, J. Fahrenkrug, I. Gozes, M. Laburthe, V. May, J. R. Pisegna, D. Vaudry, H. Vaudry, J. A. Waschek and S. I. Said, Pharmacology and functions of receptors for vasoactive intestinal peptide and pituitary adenylate cyclase-activating polypeptide: IUPHAR review 1, Br. J. Pharmacol., 2012, 166, 4–17 CrossRef CAS PubMed.
- X. Peyrassol, T. Laeremans, V. Lahura, M. Debulpaep, H. El Hassan, J. Steyaert, M. Parmentier and I. Langer, Development by Genetic Immunization of Monovalent Antibodies Against Human Vasoactive Intestinal Peptide Receptor 1 (VPAC1), New Innovative, and Versatile Tools to Study VPAC1 Receptor Function, Front. Endocrinol., 2018, 9, 153 CrossRef PubMed.
- B. Ekstrand, J. F. Young and M. K. Rasmussen, Taste receptors in the gut - A new target for health promoting properties in diet, Food Res. Int., 2017, 100, 1–8 CrossRef CAS PubMed.
- P. J. Trivedi and D. H. Adams, Chemokines and Chemokine Receptors as Therapeutic Targets in Inflammatory Bowel Disease; Pitfalls and Promise, J. Crohns Colitis., 2018, 12, S641–S652 CrossRef PubMed.
- Z. Liu and C. C. Udenigwe, Role of food-derived opioid peptides in the central nervous and gastrointestinal systems, J. Food Biochem., 2019, 43, e12629 CrossRef PubMed.
- T. Yamamoto, P. Nair, J. Vagner, T. Largent-Milnes, P. Davis, S. W. Ma, E. Navratilova, S. Moye, S. Tumati, J. Lai, H. I. Yamamura, T. W. Vanderah, F. Porreca and V. J. Hruby, A structure-activity relationship study and combinatorial synthetic approach of C-terminal modified bifunctional peptides that are delta/mu opioid receptor agonists and neurokinin 1 receptor antagonists, J. Med. Chem., 2008, 51, 1369–1376 CrossRef CAS PubMed.
- C. Chatterjee, S. Gleddie and C. W. Xiao, Soybean Bioactive Peptides and Their Functional Properties, Nutrients, 2018, 10, pii: E1211 CrossRef PubMed.
- A. Stefanucci, A. Mollica, G. Macedonio, G. Zengin, A. A. Ahmed and E. Novellino, Exogenous opioid peptides derived from food proteins and their possible uses as dietary supplements: A critical review, J. Food Rev. Int., 2018, 34, 70–86 CrossRef CAS.
- S. Brooke-Taylor, K. Dwyer, K. Woodford and N. Kost, Systematic Review of the Gastrointestinal Effects of A1 Compared with A2 beta-Casein, Adv. Nutr., 2017, 8, 739–748 CrossRef CAS PubMed.
- M. D. Turner, B. Nedjai, T. Hurst and D. J. Pennington, Cytokines and chemokines: At the crossroads of cell signalling and inflammatory disease, Biochim. Biophys. Acta, 2014, 1843, 2563–2582 CrossRef CAS PubMed.
- M. E. Delgado and T. Brunner, The many faces of tumor necrosis factor signaling in the intestinal epithelium, Genes Immun., 2019 DOI:10.1038/s41435-019-0057-0.
- B. K. Pedersen, A. Steensberg, C. Fischer, C. Keller, K. Ostrowski and P. Schjerling, Exercise and cytokines with particular focus on muscle-derived IL-6, Exerc. Immunol. Rev., 2001, 7, 18–31 CAS.
- M. Mihara, M. Hashizume, H. Yoshida, M. Suzuki and M. Shiina, IL-6/IL-6 receptor system and its role in physiological and pathological conditions, Clin. Sci., 2012, 122, 143–159 CrossRef CAS PubMed.
- L. Ferrucci, A. Corsi, F. Lauretani, S. Bandinelli, B. Bartali, D. D. Taub, J. M. Guralnik and D. L. Longo, The origins of age-related proinflammatory state, Blood, 2005, 105, 2294–2299 CrossRef CAS PubMed.
- L. D'Auria, C. Bonifati, A. Mussi, G. D'Agosto, C. De Simone, B. Giacalone, C. Ferraro and F. Ameglio, Cytokines in the sera of patients with pemphigus vulgaris: interleukin-6 and tumour necrosis factor-alpha levels are significantly increased as compared to healthy subjects and correlate with disease activity, Eur. Cytokine Netw., 1997, 8, 383–387 Search PubMed.
- B. K. Pedersen, Anti-inflammatory effects of exercise: role in diabetes and cardiovascular disease, Eur. J. Clin. Invest., 2017, 47, 600–611 CrossRef CAS PubMed.
- A. J. Van Oostrom, T. P. Sijmonsma, T. J. Rabelink, B. S. Van Asbeck and M. C. Cabezas, Postprandial leukocyte increase in healthy subjects, Metabolism, 2003, 52, 199–202 CrossRef CAS PubMed.
- A. J. van Oostrom, T. P. Sijmonsma, C. Verseyden, E. H. Jansen, E. J. de Koning, T. J. Rabelink and M. Castro Cabezas, Postprandial recruitment of neutrophils may contribute to endothelial dysfunction, J. Lipid Res., 2003, 44, 576–583 CrossRef CAS PubMed.
- P. Lundman, S. Boquist, A. Samnegard, M. Bennermo, C. Held, C. G. Ericsson, A. Silveira, A. Hamsten and P. Tornvall, A high-fat meal is accompanied by increased plasma interleukin-6 concentrations, Nutr. Metab. Cardiovasc. Dis., 2007, 17, 195–202 CrossRef CAS PubMed.
- M. A. Martinez-Garcia, S. Moncayo, M. Insenser, R. Montes-Nieto, E. Fernandez-Duran, F. Alvarez-Blasco, M. Luque-Ramirez and H. F. Escobar-Morreale, Postprandial inflammatory responses after oral glucose, lipid and protein challenges: Influence of obesity, sex and polycystic ovary syndrome, Clin. Nutr., 2019 DOI:10.1016/j.clnu.2019.03.027.
- B. K. Pedersen, A. Steensberg, P. Keller, C. Keller, C. Fischer, N. Hiscock, G. van Hall, P. Plomgaard and M. A. Febbraio, Muscle-derived interleukin-6: lipolytic, anti-inflammatory and immune regulatory effects, Pflugers Arch., 2003, 446, 9–16 CrossRef CAS PubMed.
Footnote |
† Electronic supplementary information (ESI) available: Fig. S1: consort 2010 flow diagram. See DOI: 10.1039/c9fo01398a |
|
This journal is © The Royal Society of Chemistry 2019 |