DOI:
10.1039/C8FD00140E
(Paper)
Faraday Discuss., 2019,
214, 105-121
Hot electron effects during reactive scattering of H2 from Ag(111): assessing the sensitivity to initial conditions, coupling magnitude, and electronic temperature
Received
1st October 2018
, Accepted 30th October 2018
First published on 10th December 2018
Abstract
Low-lying electronic excitations in metals, so-called hot electrons, efficiently mediate molecule-metal energy transfer and contribute to energy loss during molecular reactions at surfaces. They furthermore play an important role in plasmon-driven chemistry. Electronic friction represents a simple and effective concept to model hot electron-induced energy loss under ambient conditions. Different methods exist that vary in their description of magnitude, coordinate and directional dependence of friction during reactive molecular scattering at metal surfaces. Using molecular dynamics simulations with electronic friction, we systematically study the effect of hot electrons on measurable state-to-state scattering probabilities of molecular hydrogen from a (111) surface of silver. We assess the ability of ab initio electronic friction methods to accurately describe hot electron-mediated energy loss as a function of initial reaction conditions and electronic temperature. We furthermore find that dynamic scattering results and the ensuing energy loss are highly sensitive to the magnitude of electronic friction. Therefore, existing approximate models of electronic friction, which exhibit inherent uncertainties with respect to the magnitude of electronic friction, may not be applicable for a quantitative prediction of plasmon driven hot electron effects in their current state. We outline a development direction to potentially overcome these limitations.
1 Introduction
Plasmonic science1 and hot electron chemistry2–4 are based on the idea of controlling the flow of energy between electronic excitations in metallic surfaces or nanostructures,5 so-called hot electrons, and molecular adsorbate degrees of freedom, such as molecular vibrations. A high level of control of this molecule–surface energy flow can facilitate chemical bond breaking events with light by funneling energy into the reaction coordinate or impair a reaction by efficiently dissipating energy away from a reaction centre.6,7 The underlying mechanism for this energy exchange is based on the efficient coupling of molecular adsorbate motion and metallic electrons due to the vanishing band-gap in metallic systems.8,9 The consequence of this coupling is a deviation from thermal chemistry as described by the Born–Oppenheimer approximation and a failure of existing methods to describe chemical reaction dynamics.10,11
The proposed technological applications of hot electron effects in plasmonic sensors,12 plasmon-enhanced catalysts,13 and locally-enhanced cancer radiation treatment,14 are driven by exciting fundamental experiments that provide quantitative evidence for hot electron effects. These include molecular beam scattering experiments that explicitly measure the state-to-state scattering probability and the energy loss profile of a gas–surface reaction,15 but also the measurement of electric currents,11,16 so-called chemicurrents, that occur due to hot electrons that are generated upon adsorption, desorption, or chemical transformation of molecules.
The ability to computationally predict the molecular details that underlie hot electron-mediated processes and the intricate coupling of electrons and atomic motion is essential for the design of new devices and catalysts based on plasmonics. As such, it is imperative to develop first-principles computational simulation methods that correctly capture how molecular adsorbate motion generates hot electrons or how hot electrons affect measurable dynamical properties of chemical reactions. The intrinsic dimensionality of systems involving many hundreds of atoms and thousands of electrons make exact nonadiabatic quantum dynamics simulations close to unfeasible and therefore ask for efficient approximate mixed quantum-classical dynamics methods.17,18 Such methods are typically based on stochastic surface hopping models based on chemical master equations, where hot electron effects on the reaction dynamics are modelled as explicit electronic transitions.19,20 An alternative is the molecular dynamics with electronic friction (MDEF) method,21–23 where the classical motion of nuclei follows a single potential energy surface and hot electron effects are described as electronic friction forces that act on the adsorbate nuclei. These forces are governed by the so-called electronic friction tensor,24 which arises from nonadiabatic coupling and the resulting equations of motion follow a stochastic Langevin model. The electronic friction tensor is in general frequency-dependent and anisotropic but is often approximated to be frequency independent and spatially isotropic, i.e., nonadiabatic relaxation rates are equal in all spatial directions for rigid atomic and molecular motion. The most common is the Local Density Friction Approximation (LDFA),25,26 where nonadiabatic coupling is expressed as a simple function of the metallic electron density within which the adsorbate atom is embedded. This model is spatially isotropic for rigid molecular motion, but does account for some anisotropic effects along intramolecular degrees of freedom.26,27 Due to its simplicity and ease of computation, LDFA-based MDEF is a particularly appealing approach that, in the past, has been applied to describe hot electron-mediated dynamics in ambient28 and laser-driven conditions.29,30 However, especially for molecular scattering and light-driven plasmonics, the underlying neglect of frequency dependence and the role of molecular anisotropy of electronic friction forces and their effects on measurable observables have been the reason for exciting debates in recent literature.24,31–33
In recent work, we have achieved a description of electronic friction that captures the directional and the explicit vibrational mode dependence and tensorial nature of electronic friction using an efficient implementation of first-order time-dependent perturbation theory (TDPT) based on Density Functional Theory (DFT).24,31 On the examples of vibrational energy loss of metal-adsorbed diatomics31 and the dissociative chemisorption of molecular hydrogen on Ag(111),34 we were able to show that the overall magnitude of friction is, on average, similar to the LDFA prediction, but the directional and vibrational mode dependences and their effect on the dynamic energy loss are different. Before us, Spiering and Meyer have reached a similar conclusion for reactive scattering of H2 on Cu(111),35 where mode-dependent electronic friction forces as described by TDPT provide a measurable signature of energy loss during state-to-state scattering of vibrationally excited molecules. We have recently developed a general method to construct an analytical representation of the electronic friction tensor as a function of the adsorbate coordinates that enabled us to simulate dynamic scattering probabilities and to discuss the subtle differences in electronic friction that give rise to the observation that hot electron effects are much more pronounced during reactive scattering of H2 on Cu(111) than on Ag(111).36
In this work, we use this analytical representation of electronic friction for H2 on Ag(111) further to systematically assess the validity and robustness of the current frequency-independent MDEF method based on TDPT electronic friction. We do this by studying the sensitivity of dynamic observables such as the vibrational excitation/de-excitation probability during molecular scattering and the integrated energy loss along a specific degree of freedom as a function of various initial conditions and the type of electronic friction description. The former includes the effects of the initial and final vibrational excited states and the electronic temperature of the substrate. The latter includes a comparison between LDFA and TDPT and a discussion of their limitations and sensitivity to intrinsic numerical parameters. We find that the energy loss predicted by LDFA and TDPT shows different trends with respect to the parameters we studied and that dynamic observables are particularly sensitive to the absolute magnitude of tensorial friction. The intrinsic sensitivity of friction to numerical parameters such as the broadening width will represent a particular challenge for quantitative predictions of hot electron mediated reaction dynamics at high electronic temperatures that correspond to light-driven conditions. We therefore stress the importance of overcoming this limitation introduced by assuming frequency independence of the friction tensor. This will enable further study of the applicability of electronic friction approaches for light-driven hot electron chemistry.
2 Theory
2.1 Electronic friction based on time-dependent perturbation theory
In MDEF simulations, the nuclear dynamics are defined via a Langevin equation (LE):21 | 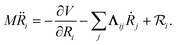 | (1) |
Herein, the three force components are the conservative force due to the potential energy V, the electronic friction force given as a product between the electronic friction tensor Λ and the velocity of the atoms, and a temperature- and friction-dependent random force term that ensures detailed balance. The second and third terms describe the interaction of the adsorbate degrees of freedom with the bath degrees of freedom that represent the metal electrons. The two major assumptions to arrive at an LE are that the electron-nuclear coupling is weak and that it occurs instantaneously, i.e., coupling due to electron–hole pair (EHP) excitations has no memory of previous times and no dependence on the perturbing frequency (Markov approximation). The first assumption is problematic in cases where molecule–metal electron transfer significantly changes the underlying potential energy surfaces, such as in the case of nitrous oxide on Au(111).37,38 The second approximation is equivalent to assuming that the electron-nuclear coupling is constant regardless of nuclear velocities or vibrational frequencies. The result is that, within 1st order TDPT, we evaluate the electronic friction tensor using Fermi’s golden rule:23,31 |  | (2) |
Herein, the factor 2 accounts for spin multiplicity in the case of non-spin-polarised calculations and we have not included occupation factors that arise from finite temperature state populations. As our DFT calculations generate a finite number of states at discrete points k in momentum space, we need to interpolate to describe the friction tensor elements, which correspond to relaxation rates due to electron-nuclear coupling along the (mixed) Cartesian directions. This can be done in two ways, as follows. By choosing a broadening large enough to achieve convergence and small enough not to affect relaxation rates one approaches the zero-frequency limit, which is the formally correct limit within 1st order TDPT. It was recently shown that this level of description struggles to capture vibrational energy loss for dense molecular overlayers33 and it does not correspond to the correct limit for gas–surface scattering with incidence energies above several hundred meV. As detailed in our previous work34 and by Spiering and Meyer,35 we choose the second approach, namely to replace the delta function with a normalized Gaussian function of a finite width (0.6 eV). With such a broadening, we effectively model the inclusion of higher energy EHPs. It has recently been pointed out that such broadening introduces contributions that are not rigorously contained in a zero-frequency 1st order approximation32 and leads to relaxation rates that depend on the choice of broadening. This dependence remains a weak spot in this theory and has been discussed in detail in our previous work,31 yet, in lieu of a feasible non-Markovian MDEF approach,39 this pragmatic choice delivers a meaningful way to calculate relaxation rates beyond the LDFA24,31,40,41 that correctly capture the directional and electronic structure dependence of the friction tensor. All elements of the friction tensor are calculated as a function of the adsorbate atom position using the all-electron, local atomic orbital code FHI-aims42 and the Perdew–Burke–Ernzerhof (PBE) functional.43 Our computational settings regarding model set-up, basis set, and Brillouin zone sampling have been detailed in a previous publication.34
2.2 Neural network representation of the friction tensor
2.2.1 Symmetry mapping scheme.
To solve eqn (1) efficiently, one has to evaluate the potential energy and friction tensor at each trajectory step analytically. Neural network (NN) based representations of the PES44 and the embedding electron density45 (directly associated with the LDFA friction coefficient) which fully comply with the surface symmetry have been described previously. We thus focus here on the more complex representation of the anisotropic friction tensor computed by TDPT. Because the TDPT-based friction tensor is directionally-dependent on molecular coordinates, the symmetry equivalent molecular configurations that share the same potential energy and LDFA friction coefficient would have totally different but symmetrically-correlated friction tensor elements. This feature immediately renders the extension of any standard methodology for PES construction difficult for representing the friction tensor as a function of molecular geometry. Specifically, we discuss the case of a diatomic molecule (H2) on a rigid metal surface, in which six coordinates are involved. These six coordinates can either be the Cartesian coordinates (x1, y1, z1, x2, y2, z2) or internal coordinates (X, Y, Z, r, θ, φ). In the latter, the first three specify the center of mass of the molecule while the rest denote the internuclear distance and the polar and azimuthal angles to the surface normal.
Spiering and Meyer have very recently reported an approximate NN-based representation of the 6 × 6 friction tensor for the H2 + Cu(111) system.35 They first described individual friction coefficients in terms of four symmetry independent internal coordinates, as illustrated in Fig. 1, namely X, Y, Z, and r with angular coordinates fixed (e.g., θ0 = φ0 = 90°). Since the tensorial friction (Λ) is a symmetric matrix, only the upper triangular elements Λij(i ≤ j) are to be determined in practice. This treatment avoids the complex symmetry involved in the full six-dimensional representation. Once Λ(X, Y, Z, r) with θ0 = φ0 = 90° is obtained, the friction tensor at an arbitrary configuration depending on all six internal coordinates can be approximately generated by the following transformation,35
| Λ(X, Y, Z, r, θ, φ) ≈ T(θ, φ)Λ(X, Y, Z, r)T−1(θ, φ), | (3) |
where
T(
θ,
φ) is the transformation matrix that rotates configuration (
θ,
φ) to the corresponding reference geometry with
θ0 =
φ0 = 90°. Although this decomposition of the full 6 × 6 friction tensor certainly depends on the choice of the reference orientation, Spiering and Meyer have shown that the dependence is relatively weak near the minimum energy path (MEP).
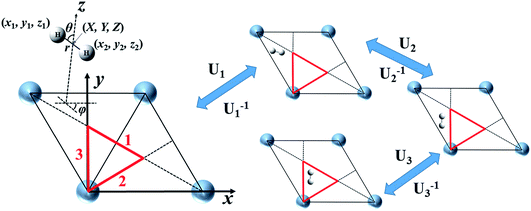 |
| Fig. 1 Cartesian (x1, y1, z1, x2, y2, z2) and internal (X, Y, Z, r, θ, φ) coordinates to describe H2 interacting with a rigid Ag(111) surface represented by a unit cell, with the symmetry unique triangle marked in red (left panel). Transformation matrices (U1, U2, U3) and their inverses that move a molecular configuration outside the triangle into it and back to the original position, corresponding to the three reflection lines (1, 2, 3) that enclose the triangle. | |
In a previous publication36 and in this present work, we propose an alternative strategy. We choose to fit the elements of the full 6 × 6 matrix form of the electronic friction tensor in Cartesian coordinates inside an irreducible triangle in the (111) surface unit cell (marked in red in Fig. 1). In this triangle, each friction tensor element is symmetry unique with a one-to-one mapping to a molecular geometry, thus posing no problems for fitting. In practice, since we have collected many data scattered inside and out the unit cell, we first move those data distributed outside this triangle into it. This can be done by first translating a data point outside the unit cell into this region, which does not change the friction tensor, followed by a series of reflections when necessary.46 For each reflection, the new Cartesian coordinate vector q′ and friction tensor Λ′ can be obtained by,
where
q and
Λ are the original coordinate vector and friction tensor and
U is the transformation matrix. For Ag(111),
U1,
U2 and
U3 are given below and illustrated in
Fig. 1,
| 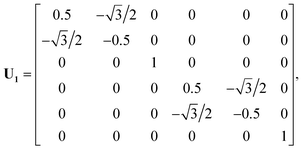 | (6) |
| 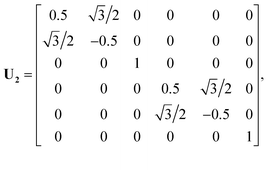 | (7) |
| 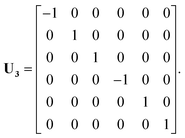 | (8) |
In practice, the number of reflections operated on a given point may be less than three and, in the case that the molecule is already situated inside the triangle, no transformation is necessary. It should be noted that this treatment does not account for exchange symmetry of the two H atoms. We solve this issue by ordering the positions of the two hydrogen atoms when necessary (i.e. with z1 ≤ z2) so that the related matrix elements in the friction tensor are exchanged accordingly. Similar mapping schemes have been successfully applied in constructing PESs for gas–surface systems using either NNs47–49 or the modified Shepard interpolation.46 One drawback of these PESs is that the gradients of PESs (i.e. forces) may not be continuous at the boundary, resulting in non-conservation of total energy when running classical trajectories. However, this is not a problem for the friction tensor as we only need values of friction coefficients but no gradients in eqn (1). Similar schemes can be devised for other facets of the metal surface.
2.2.2 Fitting and evaluation.
After the mapping procedure, a single NN is utilized to describe the one-to-one mapping between the molecular geometry and each individual element of the friction tensor in the triangle. Six Cartesian coordinates of the molecule are set as the input layer of the NN, which pass geometric information to two hidden layers with 20 and 40 neurons via hyperbolic tangent activation functions. A hybrid algorithm combining the extreme learning machine and the Levenberg–Marquardt (ELM–LM) algorithm was used to train the NN.50,51 The data set consists of ∼1200 points sampled by previous AIMD trajectories34 and ∼2600 additional points used for constructing the PES44 that cover the dynamically relevant region. Since the friction coefficients are very small far above the surface where the electron density vanishes, we exclude data points in that region. Instead, a switching function was imposed to smoothly dampen the friction coefficients to zero when the molecule–surface interaction is negligible (Z → ∞, in Å), | 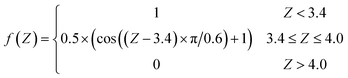 | (9) |
One should note that the NN fitting of each friction coefficient is essentially the same as that of the PES in previous works and very robust.47–49 However, for our purpose, to evaluate a friction tensor for any point outside this symmetry-unique region, we need to first find the symmetry identical geometry of that point in the irreducible triangle by the transformation described above, then calculate the NN interpolated friction tensor there, followed by inversely transforming the obtained full 6 × 6 friction tensor back to that corresponding to the original geometry.
2.3 Molecular dynamics simulations with tensorial friction
The molecular dynamics (MD) and MDEF trajectories have been computed with various initial conditions sampled in a quasi-classical way as described in our previous publication.36 The initial molecular center is chosen to be far above the Ag(111) surface (Z = 8.0 Å) with random lateral positions in the surface unit cell. The molecular internal coordinates and conjugate momenta are sampled semi-classically for given vibrational and rotational quantum numbers v and j.52 The molecular orientation is randomly selected in the polar and azimuthal angles for most cases. For those mj specific states, however, the molecular orientation is chosen randomly on a plane perpendicular to the angular momentum vector
, which forms an angle of
with the space-fixed Z axis (surface normal here). For the scattered molecule, the vibrational action number is determined by Einstein–Brillouin–Keller (EBK) semi-classical quantization and rotational quantum number j by the quantum mechanical expression for rotational angular momentum.53 As in ref. 35, the standard histogram binning is used to obtain the state distributions with integer quantum numbers.
Given an initial condition, eqn (1) is propagated using third-order Beeman’s algorithm with a time step of 0.1 fs. The MD and MDEF with LDFA simulations are easily implemented as friction coefficients only depend on the value of the electron density of the clean substrate.25,28 The tensorial nature of the TDPT based electronic friction makes the equations of all coordinates coupled. In practice, we need to transform the coordinates and velocities into the friction eigenspace and apply the friction eigenvalues as damping coefficients onto the transformed velocities, and then transform the products back to the Cartesian space. This requires a diagonalization of the friction tensor at each time step. In addition, the random force term on the right-hand side of the Langevin equation, given a finite surface temperature (T), is described as a Gaussian white noise of zero mean and a standard deviation of
, where ηi is the ith friction eigenvalue (or simply the ith friction coefficient in LDFA) and k is the Boltzmann constant. However, we neglect surface temperature in most simulations because the contribution of the random force is typically small, except where we discuss temperature effects explicitly.
3 Results and discussion
3.1 Validation of the NN interpolation of the friction tensor
Based on our previously published analysis,54 we have further examined the quality of our NN representation of the friction tensor. Fig. 2a shows the root mean square errors (RMSEs) averaged over all data for the 21 independent friction coefficients. The overall RMSEs uniformly range from ∼0.01 ps−1 to ∼0.03 ps−1 without any outliers, which are generally comparable or smaller than those reported for the H2 + Cu(111) system.35 In Fig. 2b, we compare several large components in the TDPT friction tensor with the NN interpolated values along the minimum energy path (MEP) for H2 dissociative chemisorption, corresponding to the route for H2 dissociation over the bridge site with the H–H bond parallel to the surface and stretching to two hollow sites. Clearly, there are strong couplings between the vibration (r) and translation (Z) directions, and between parallel motion (X/Y) and rotation (θ). Although the coupling terms can be both negative and positive, their absolute values increase as the molecule approaches the transition state. It has been previously shown that our NN diagonal tensor elements agree well with TDPT ones,36 and we also find here excellent agreement between NN and TDPT off-diagonal friction coefficients. As a further validation, Fig. 2c compares two-dimensional electronic friction contour plots, as a function of Z and r along the MEP, generated from NN interpolation and direct DFT calculations, respectively. Our NN representation faithfully captures the multi-dimensional topography of the friction tensor element, even though these data points were actually not included in the training set. These results indicate that the NN representation of the friction tensor is sufficiently accurate for subsequent MDEF simulations.
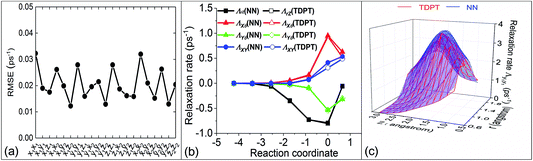 |
| Fig. 2 (a) Root Mean Square Errors (RMSEs) of Neural Network (NN) fits for the 21 independent friction tensor elements with TDPT. (b) Comparison of several off-diagonal elements in the friction tensor with regard to internal coordinates obtained by NNs and TDPT along the minimum energy path (MEP). (c) Comparison of the original TDPT and NN interpolated Λy1y1 as a function of Z and r with the H2 molecule dissociating with parallel orientation from the bridge site to two hollow sites. | |
3.2 The physical differences between LDFA and TDPT
In our previous work,36 we have shown different behaviors of the electronic friction in the H2 + Cu(111) and H2 + Ag(111) systems, which can be traced back to the differences in the underlying electronic structure of the two metals. Within the framework of the independent atom LDFA method, the friction coefficient depends only on the local electron density (ρ) at the position of the adsorbate atoms, whereas TDPT accounts for the response of the electronic wave functions (and therefore the nonadiabatic density response) of the combined molecule–surface system to the adsorbate motion. In Fig. 3a, we plot the clean surface density ρ varying with the position of one of the H atoms in the molecule along the MEP (the other H atom moves almost symmetrically to the opposite and hence feels nearly the same electron density). Because the transition state structures for H2 dissociation on Cu(111) and Ag(111) appear at different distances from the surface, the comparison of ρ is best made as a function of the relative height with respect to that of the hydrogen atom at the respective transition state. Surprisingly, the embedded electron densities for H2 scattering on Ag and Cu are very similar, resulting in very close values in LDFA friction coefficients. In the case of Fermi’s golden rule, electronic friction does not depend on the density, but on the local density of states around the Fermi level and on the nonadiabatic coupling matrix elements that encode the difference in response along different directions, which sensitively depend on the underlying electronic structure and the nature of the metal. This level of difference is position and direction dependent and can be directly seen in Fig. 3b. In the following we will study how these different descriptions translate into measurable dynamic observables and how they depend on system parameters.
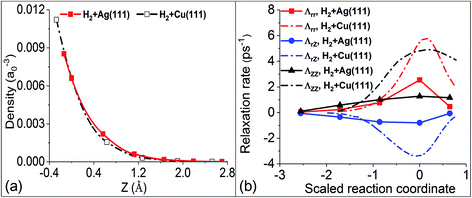 |
| Fig. 3 (a) Comparison of the clean metal substrate electron density evaluated at the position of an H atom moving along the MEPs for dissociation on Cu(111) and Ag(111), as a function of the relative height with respect to the molecular center at the transition state (i.e. ZTS = 0). (b) Comparison of the mode-specific electronic friction computed by TDPT along the MEPs for H2 dissociation on Cu(111) and Ag(111). | |
3.3 The sensitivity of the vibrational deexcitation probability on the magnitude of friction
In our previous results on the vibrational deexcitation probabilities from H2 (v = 2, j = 0) to H2 (v = 1, j = 0) (i.e. P2→1) during H2 scattering on Ag(111), we found that both TDPT and LDFA yield comparable deexcitation probabilities that are slightly elevated compared to the adiabatic MD case (see Fig. 4a). This is in contrast to findings for H2 on Cu(111), where particularly the TDPT friction has been shown to lead to a significantly elevated deexcitation probability at intermediate translational incidence energies.35 This suggests that dynamic observables are highly sensitive to the absolute magnitude of electronic friction. To test this sensitivity, we monitor the P2→1 varying with the electronic friction multiplied with a scaling factor for H2 + Ag(111). We scale all tensor elements in Cartesian coordinates by the same factor. As shown in Fig. 4a, rather unsurprisingly, P2→1 increases for both TDPT and LDFA with increasing magnitude of electronic friction. When the strength of the TDPT friction tensor is doubled, corresponding to a similar magnitude as was found for the H2 + Cu(111) system, the P2→1 with TDPT becomes much more significantly increased and sharply peaks at a translational energy (Ei) of 0.3 eV. At this specific Ei, we obtain a P2→1 with TDPT that is roughly 4 (3) times larger than the adiabatic (LDFA) results, in general accord with the observation in the H2 + Cu(111) system. On the other hand, the doubly-increased LDFA friction coefficients also promote vibrational deexcitation at higher energies, yielding a greater enhancement of P2→1 at translational energies higher than 0.4 eV. The different dependence of P2→1 on translational energy with TDPT and LDFA can be understood by the corresponding mode-specific magnitude of electronic friction and nonadiabatic energy loss. As discussed in our previous work,36 the LDFA electronic friction is dominated by the component along the translational coordinate, leading to almost linearly increased energy dissipation with increasing translational energy. This behavior becomes more remarkable when the strength of the friction coefficient is doubled, as shown in Fig. 4b, giving rise to the greater enhancement at high translational energies for LDFA. On the other hand, the fact that the energy loss due to the TDPT electronic friction shows a peak at 0.3 eV is consistent with the same peak in the P2→1 distribution. This can be related to a balanced effect between the longer interaction time and smaller molecular velocity at this incidence energy.
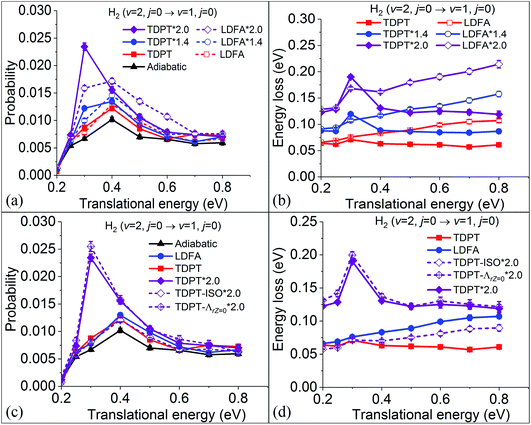 |
| Fig. 4 (a) Vibrational deexcitation probabilities from H2 (v = 2, j = 0) to H2 (v = 1, j = 0) as a function of translational energy, with adiabatic dynamics (triangles), with electronic friction by LDFA (open symbols) and TDPT (solid symbols) models, and with 1.4 (circles) and 2.0 (diamonds) times larger friction coefficients. (b) Mean nonadiabatic energy losses for various simulations in panel (a). (c) On the basis of (a) but adding new data computed by removing anisotropy (TDPT-ISO*2) and the Z–r coupling term (TDPT-ΛrZ=0*2.0) in the TDPT friction tensor. (d) Mean nonadiabatic energy losses for various simulations in panel (b). | |
Furthermore, it is interesting to analyse whether the nonadiabatic effects stem from the anisotropy along different directions or the mode-coupling between these directions in the friction tensor. In Fig. 4c, we compare the P2→1 with TDPT electronic friction where the off-diagonal coupling between the intra-atomic coordinate and the molecule-adsorbate coordinate (TDPT) is included and where it is artificially set to zero (TDPT-ΛrZ=0). To make the comparison clearer, a factor of two is also multiplied in both cases. Although the magnitude of ΛrZ is not small as displayed in Fig. 2b, interestingly, the results with zero ΛrZ are quite close to the original ones in the entire energy range, implying the negligible effect of the mode-coupling between the translational and vibrational DOFs in this case. In addition, to study the importance of directional anisotropy, we average the three diagonal components of the TDPT friction tensor (twice in magnitude) in the Cartesian space for each atom, then assign the same friction coefficient for each atomic Cartesian coordinate in the following way,
|  | (10) |
and zero all off-diagonal terms (TDPT-ISO). This converts the TDPT friction tensor to a form comparable with LDFA. It is found in
Fig. 4c that the calculated
P2→1 in this condition becomes drastically decreased, but very similar to the unscaled LDFA ones, indicating that the anisotropy of the friction tensor plays an important role in this process. This is consistent with what Meyer and Spiering have found for the H
2 + Cu(111) system
35 and hints to the fact that the larger translational energy loss as predicted by LDFA really stems from its neglect of the directional anisotropy of the electronic friction tensor. It should be noted that while statically isotropic, the LDFA (much as TDPT) description does provide a certain level of dynamic directional dependence during molecular motion within MDEF due to the directional dependence of the velocity profile.
3.4 Dependence on initial conditions and final states
Our previous analyses suggested that the velocity profile and potential energy landscape also play a significant role in determining the nonadiabatic effects in the molecular scattering process.36 One can of course change the velocity profile by choosing various initial conditions. We first study the influence of the angular velocity by performing MDEF simulations with initially different rotational and orientational states at the same (v = 2) vibrational state. Fig. 5a compares the vibrational deexcitation probabilities for H2 (v = 2, j = 5, mj = 0/5) to H2 (v = 1, j = 5) with and without electronic friction. These two initial states correspond to the cartwheel (mj = 0) and helicopter (mj = j) orientations, respectively, which would impact the surface with similar vibrational and translational velocities but distinctly different angular velocities for a given incidence energy. Compared to previous results for the rotational ground state, the nonadiabatic energy dissipation is more important for these two states, resulting in up to an ∼80% increase of the vibrational deexcitation probability at low translational energies. Both friction methods provide similar results with small EHP-induced enhancement for the cartwheel motion and larger effects for the helicopter motion. The latter can be understood in terms of an increased residence time of the molecule and rovibrational coupling during the scattering process. Our findings show that, despite some rotational state dependence of friction, there is little change of the nonadiabatic effects on P2→1 among different product rotational states (partly shown in ref. 36), suggesting that the angular velocities only play a minor role in such cases.
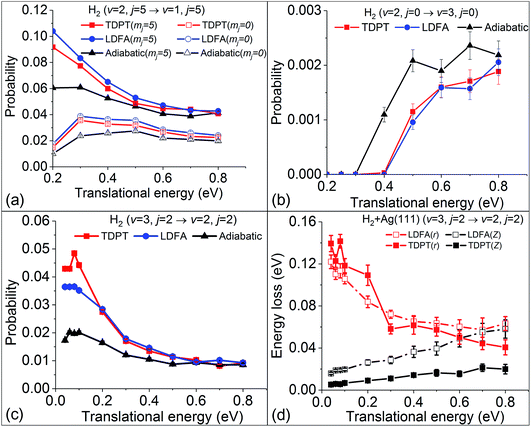 |
| Fig. 5 (a) Vibrational state-to-state deexcitation probabilities from H2 (v = 2, j = 5) to H2 (v = 1, j = 5) for rotationally excited initial conditions as predicted with MD, MDEF(LDFA), and MDEF(TDPT). (b) Vibrational excitation probabilities upon scattering. (c) Vibrational deexcitation probability from H2 (v = 3, j = 2) to H2 (v = 2, j = 2). (d) Energy loss during vibrational deexcitation from H2 (v = 3, j = 2) to H2 (v = 2, j = 2). | |
Following this line of thought, we show the excitation probability (P2→3) from H2 (v = 2, j = 0) to H2 (v = 3, j = 0) in Fig. 5b and deexcitation probability (P3→2) from H2 (v = 3, j = 2) to H2 (v = 2, j = 2) in Fig. 5c. Both scattering processes involve a higher vibrational state which naturally increases the molecular velocity with respect to the intramolecular stretching mode. We see much stronger nonadiabatic effects in both cases, leading to the significant decrease of P2→3 and increase of P3→2 due to electronic friction at low translational energies. In such cases, the translational velocity is low enough for the molecule to remain close to the surface for an extended time, and the vibrational velocity is high enough to cause electronic excitations. While the results with TDPT and LDFA are very similar with respect to P2→3, the former become qualitatively different from the latter in P3→2 below Ei = 0.2 eV. This interesting behavior is reflected by the nonadiabatic energy loss given in Fig. 5d, where the TDPT induced energy losses along the molecular vibration (r direction) are more significant than the LDFA ones in that range. Such a large vibrational energy loss with TDPT at low energy seems to be surprising given the similar magnitude of Λrr with TDPT and LDFA. We therefore monitor the velocity and friction coefficient profiles in the Z and r directions as a function of time for an exemplary trajectory at Ei = 0.1 eV in Fig. 6a and b. It appears that Λrr with TDPT is remarkably higher than those with LDFA in the strong interaction region, implying that the trajectory significantly departs from the MEP. Unlike our previous results (Fig. 4d in ref. 36) for H2 (v = 2 → v = 1), the H2 (v = 3) molecules do not necessarily follow the MEP. As a result, the multidimensional topography of the friction tensor determines the total nonadiabatic energy loss and thus the scattering probability. This reflects the importance of correctly capturing this tensorial topology.
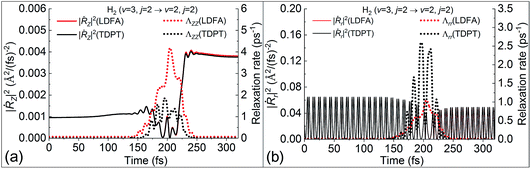 |
| Fig. 6 Variation of the square of velocity (solid lines) in the Z (|Ṙz|2, panel (a)) and r (|Ṙr|2, panel (b)) directions along with the corresponding friction coefficients (dotted lines), as a function of time for a representative trajectory for H2 (v = 3, j = 2) to H2 (v = 2, j = 2) scattering on Ag(111), at a translational energy of 0.1 eV. | |
3.5 Electronic temperature
Within the electronic friction picture, plasmon driven or laser-heated hot electron effects are typically simulated via an increase of the electronic temperature that defines the heat of the electronic bath and the strength of the random force contribution. This can be done in the form of a constant elevated temperature or by simulating a time-dependent temperature profile that is coupled with a two-temperature model of the coupled lattice-electron dynamics of the metallic substrate.29,30 Electronic temperatures that arise from laser-heating a surface can reach transient temperatures up to several thousands of Kelvin. It should be noted that this approach will assume that all hot electrons are thermalised and the weak coupling assumption will neglect any possible resonant electron-nuclear coupling effects due to high-lying excitations. Furthermore, the use of a temperature ramp is not strictly valid within an equilibrium Langevin theory and corresponding implications on detailed balance need to be carefully considered. In previous work, we studied the effect of including electronic temperature in eqn (2) and found that over a range of up to 6000 K, electronic relaxation rates within our currently employed level of theory depend very weakly on temperature.31 We therefore describe the electronic friction tensor as temperature independent. However, within the dynamic formalism of eqn (1) temperature still enters the Langevin expression and it is interesting to study how dynamic state-to-state scattering is affected by varying the electronic bath temperature. Whereas previous sections studied scattering at low temperature conditions and neglected the random force, Fig. 7 shows the dependence of vibrational deexcitation as a function of different electronic temperatures (0 K, 1000 K, and 6000 K). We find that, while overall deexcitation probabilities do not significantly change for both friction models, higher temperatures lead to higher integrated energy loss and increased deexcitation probabilities for very low translational energies. For the LDFA method, this effect becomes relevant at lower temperatures than for the TDPT method. This can be related to the higher average friction magnitude of the LDFA method. The increase of deexcitation probability at low translational energies can be directly understood by the increased residence time of molecules close to the surface and the increased exposure to random force perturbations. From our results, it appears that state-to-state scattering is not strongly affected by plasmonic heating. This will, however, be different for rates of reactions such as recombinative desorption and dissociation reactions with high barriers and physisorbed precursor states where surface residence times are much larger.
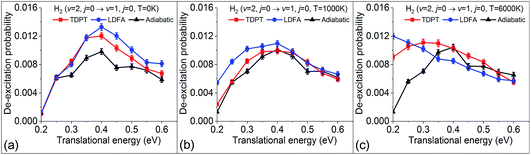 |
| Fig. 7 Vibrational deexcitation probabilities from H2 (v = 2, j = 0) to H2 (v = 1, j = 0) simulated by MD, MDEF(LDFA), and MDEF(TDPT), with electronic temperatures of 0 K (a), 1000 K (b), and 6000 K (c). | |
4 Conclusions
We have presented extensive MDEF simulations within the Markov approximation for the state-to-state scattering of molecular hydrogen from a Ag(111) surface. These simulations are enabled by the construction of neural-network based potential energy surfaces and an electronic friction tensor with data calculated from density functional theory and 1st order TDPT. We find that nonadiabatic dynamic state-to-state scattering is strongly dependent on the absolute magnitude of friction with respect to the relevant internal coordinate, whereas coupling between internal coordinates does not appear to play a significant role. Different vibrational and rotational states are affected very differently by electronic friction, however, the two different friction models did not yield drastically different results. The rotational state dependence hereby is mostly due to the changed molecular orientation and particular trajectory route with respect to the surface during impingement that boost vibrational or translational energy loss. We have furthermore shown that the inclusion of elevated electronic temperatures as they appear in plasmon-driven hot electron chemistry dominantly affects reaction probabilities at low incidence energy, where surface residence times are larger than at high incidence energies. In particular the latter finding suggests that plasmon-driven MDEF studies with electronic friction directly computed from DFT orbitals are possible and could provide a basis for further systematic improvement beyond the current state-of-the-art represented by LDFA.29,30 As guided by recent work of Novko et al.,33 a word of warning on the Markov and zero-frequency approximation is in order. Our methodology is numerically robust but suffers from the choice of a broadening parameter that, despite a weak dependence in the chosen regime, does introduce some variation of frictional magnitude. Our results have shown that this dependence can strongly affect the dynamical scattering results on a level that would, at best, allow a qualitative prediction of reaction rate trends for hot electron chemistry. We conclude from this that, to investigate the ability of MDEF to act as a predictive tool for plasmonic science, the Markov approximation needs to be overcome. This endeavor is currently under way.
Conflicts of interest
There are no conflicts to declare.
Acknowledgements
YZ and BJ acknowledge support by the National Key R&D Program of China (2017YFA0303500), the National Natural Science Foundation of China (91645202, 21722306, and 21573203), the Anhui Initiative in Quantum Information Technologies, and computational resources offered by the Supercomputing Center of USTC and Advanced Materials High-Performance Company. RJM acknowledges computational resources provided by the University of Warwick Scientific Computing Research Technology Platform, and the EPSRC-funded HPC Midlands Plus centre (EP/P020232/1). HG is supported by the U.S. National Science Foundation (Grant No. CHE-1462109).
References
- M. L. Brongersma, N. J. Halas and P. Nordlander, Nat. Nanotechnol., 2015, 10, 25–34 CrossRef CAS PubMed.
- W. Xie and S. Schlücker, Nat. Commun., 2015, 6, 7570 CrossRef PubMed.
- J. Y. Park, S. W. Lee, C. Lee and H. Lee, Catal. Lett., 2017, 147, 1851–1860 CrossRef CAS.
- J. Y. Park, S. M. Kim, H. Lee and I. I. Nedrygailov, Acc. Chem. Res., 2015, 48, 2475–2483 CrossRef CAS PubMed.
- S. D. Forno, L. Ranno and J. Lischner, J. Phys. Chem. C, 2018, 122, 8517–8527 CrossRef.
- E. Cortés, W. Xie, J. Cambiasso, A. S. Jermyn, R. Sundararaman, P. Narang, S. Schlücker and S. A. Maier, Nat. Commun., 2017, 8, 14880 CrossRef PubMed.
- S. Mukherjee, F. Libisch, N. Large, O. Neumann, L. V. Brown, J. Cheng, J. B. Lassiter, E. A. Carter, P. Nordlander and N. J. Halas, Nano Lett., 2013, 13, 240–247 CrossRef CAS PubMed.
- A. M. Wodtke, D. Matsiev and D. J. Auerbach, Prog. Surf. Sci., 2008, 83, 167–214 CrossRef CAS.
- S. P. Rittmeyer, V. J. Bukas and K. Reuter, Adv. Phys.: X, 2018, 3, 1381574 Search PubMed.
- A. M. Wodtke, J. C. Tully and D. J. Auerbach, Int. Rev. Phys. Chem., 2004, 23, 513–539 Search PubMed.
- A. Kandratsenka, H. Jiang, Y. Dorenkamp, S. M. Janke, M. Kammler, A. M. Wodtke and O. Bünermann, Proc. Natl. Acad. Sci. U. S. A., 2018, 115, 680–684 CrossRef CAS PubMed.
- D. Sil, K. D. Gilroy, A. Niaux, A. Boulesbaa, S. Neretina and E. Borguet, ACS Nano, 2014, 8, 7755–7762 CrossRef CAS PubMed.
- P. Christopher, H. Xin and S. Linic, Nat. Chem., 2011, 3, 467–472 CrossRef CAS PubMed.
- X. Huang, P. K. Jain, I. H. El-Sayed and M. A. El-Sayed, Nanomedicine, 2007, 2, 681–693 CrossRef CAS PubMed.
- O. Bunermann, H. Jiang, Y. Dorenkamp, A. Kandratsenka, S. M. Janke, D. J. Auerbach and A. M. Wodtke, Science, 2015, 350, 1346–1349 CrossRef PubMed.
- H. Nienhaus, H. S. Bergh, B. Gergen, A. Majumdar, W. H. Weinberg and E. W. McFarland, Phys. Rev. Lett., 1999, 82, 446–449 CrossRef CAS.
- J. C. Tully, J. Chem. Phys., 2012, 137, 22A301 CrossRef PubMed.
- Y. Zhang, T. R. Nelson, S. Tretiak, H. Guo and G. C. Schatz, ACS Nano, 2018, 12, 8415–8422 CrossRef CAS PubMed.
- N. Shenvi, S. Roy and J. C. Tully, J. Chem. Phys., 2009, 130, 174107 CrossRef PubMed.
- H. Guo, P. Saalfrank and T. Seideman, Prog. Surf. Sci., 1999, 62, 239–303 CrossRef CAS.
- M. Head Gordon and J. C. Tully, J. Chem. Phys., 1995, 103, 10137–10145 CrossRef CAS.
- W. Dou and J. E. Subotnik, J. Chem. Phys., 2018, 148, 230901 CrossRef PubMed.
- B. Hellsing and M. Persson, Phys. Scr., 1984, 29, 360–371 CrossRef CAS.
- M. Askerka, R. J. Maurer, V. S. Batista and J. C. Tully, Phys. Rev. Lett., 2016, 116, 217601 CrossRef PubMed.
- J. Juaristi, M. Alducin, R. Muiño, H. Busnengo and A. Salin, Phys. Rev. Lett., 2008, 100, 116102 CrossRef CAS PubMed.
- D. Novko, M. Blanco-Rey, M. Alducin and J. I. Juaristi, Phys. Rev. B, 2016, 93, 245435 CrossRef.
- S. P. Rittmeyer, J. Meyer and K. Reuter, Phys. Rev. Lett., 2017, 119, 176808 CrossRef PubMed.
- M. Blanco-Rey, J. I. Juaristi, R. Díez Muiño, H. F. Busnengo, G. J. Kroes and M. Alducin, Phys. Rev. Lett., 2014, 112, 103203 CrossRef CAS PubMed.
- J. I. Juaristi, M. Alducin and P. Saalfrank, Phys. Rev. B, 2017, 95, 125439 CrossRef.
- I. Lončarić, M. Alducin, P. Saalfrank and J. I. Juaristi, Phys. Rev. B, 2016, 93, 014301 CrossRef.
- R. J. Maurer, M. Askerka, V. S. Batista and J. C. Tully, Phys. Rev. B, 2016, 94, 115432 CrossRef.
- D. Novko, M. Alducin, M. Blanco-Rey and J. I. Juaristi, Phys. Rev. B, 2016, 94, 224306 CrossRef.
- D. Novko, M. Alducin and J. I. Juaristi, Phys. Rev. Lett., 2018, 120, 156804 CrossRef CAS PubMed.
- R. J. Maurer, B. Jiang, H. Guo and J. C. Tully, Phys. Rev. Lett., 2017, 118, 256001 CrossRef PubMed.
- P. Spiering and J. Meyer, J. Phys. Chem. Lett., 2018, 9, 1803–1808 CrossRef CAS PubMed.
- Y. Zhang, R. J. Maurer, H. Guo and B. Jiang, Chem. Sci., 2019, 10, 1089–1097 RSC.
- S. Roy, N. A. Shenvi and J. C. Tully, J. Chem. Phys., 2009, 130, 174716 CrossRef PubMed.
- N. Shenvi, S. Roy and J. C. Tully, Science, 2009, 326, 829–832 CrossRef CAS PubMed.
- T. Olsen and J. Schiøtz, J. Chem. Phys., 2010, 133, 134109 CrossRef PubMed.
- M. Forsblom and M. Persson, J. Chem. Phys., 2007, 127, 154303 CrossRef PubMed.
- A. C. Luntz and M. Persson, J. Chem. Phys., 2005, 123, 074704 CrossRef CAS PubMed.
- V. Blum, R. Gehrke, F. Hanke, P. Havu, V. Havu, X. Ren, K. Reuter and M. Scheffler, Comput. Phys. Commun., 2009, 180, 2175–2196 CrossRef CAS.
- J. P. Perdew, K. Burke and M. Ernzerhof, Phys. Rev. Lett., 1996, 77, 3865–3868 CrossRef CAS PubMed.
- B. Jiang and H. Guo, Phys. Chem. Chem. Phys., 2014, 16, 24704–24715 RSC.
- B. Jiang, M. Alducin and H. Guo, J. Phys. Chem. Lett., 2016, 7, 327–331 CrossRef CAS PubMed.
- C. Crespos, M. A. Collins, E. Pijper and G. J. Kroes, J. Chem. Phys., 2004, 120, 2392–2404 CrossRef CAS PubMed.
- T. Liu, B. Fu and D. H. Zhang, Sci. China: Chem., 2014, 57, 147–155 CrossRef CAS.
- T. Liu, Z. Zhang, B. Fu, X. Yang and D. H. Zhang, Chem. Sci., 2016, 7, 1840–1845 RSC.
- X. Shen, J. Chen, Z. Zhang, K. Shao and D. H. Zhang, J. Chem. Phys., 2015, 143, 144701 CrossRef PubMed.
- Y. Zhang, X. Zhou and B. Jiang, Chin. J. Chem. Phys., 2017, 30, 727–734 CrossRef CAS.
- M. T. Hagan and M. B. Menhaj, IEEE Trans. Neural Network., 1994, 5, 989–993 CrossRef CAS PubMed.
- X. Hu, W. L. Hase and T. Pirraglia, J. Comput. Chem., 1991, 12, 1014–1024 CrossRef CAS.
-
M. C. Gutzwiller, Chaos in classical and quantum mechanics, Springer, New York, 1990 Search PubMed.
- P. Shi, Y. Yang, B. Ao, P. Zhang and X. Wang, J. Phys. Chem. C, 2014, 118, 26634–26640 CrossRef CAS.
Footnote |
† These two authors contributed equally to this work. |
|
This journal is © The Royal Society of Chemistry 2019 |
Click here to see how this site uses Cookies. View our privacy policy here.