DOI:
10.1039/C8CS00710A
(Review Article)
Chem. Soc. Rev., 2019,
48, 1826-1852
Flexible fiber-based optoelectronics for neural interfaces
Received
1st September 2018
First published on 28th February 2019
Abstract
Neurological and psychiatric conditions pose an increasing socioeconomic burden on our aging society. Our ability to understand and treat these conditions relies on the development of reliable tools to study the dynamics of the underlying neural circuits. Despite significant progress in approaches and devices to sense and modulate neural activity, further refinement is required on the spatiotemporal resolution, cell-type selectivity, and long-term stability of neural interfaces. Guided by the principles of neural transduction and by the materials properties of the neural tissue, recent advances in neural interrogation approaches rely on flexible and multifunctional devices. Among these approaches, multimaterial fibers have emerged as integrated tools for sensing and delivering of multiple signals to and from the neural tissue. Fiber-based neural probes are produced by thermal drawing process, which is the manufacturing approach used in optical fiber fabrication. This technology allows straightforward incorporation of multiple functional components into microstructured fibers at the level of their macroscale models, preforms, with a wide range of geometries. Here we will introduce the multimaterial fiber technology, its applications in engineering fields, and its adoption for the design of multifunctional and flexible neural interfaces. We will discuss examples of fiber-based neural probes tailored to the electrophysiological recording, optical neuromodulation, and delivery of drugs and genes into the rodent brain and spinal cord, as well as their emerging use for studies of nerve growth and repair.
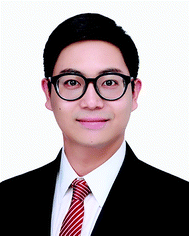
Seongjun Park
| Seongjun Park received his PhD in Electrical Engineering and Computer Science from the Massachusetts Institute of Technology (MIT) in 2018. Since then, he has worked as a postdoctoral research associate in the Research Laboratory of Electronics at MIT under the supervision of Prof. Polina Anikeeva. His research interests include the development of flexible optoelectronic neural probes and multifunctional scaffolds for neural tissue regeneration. |
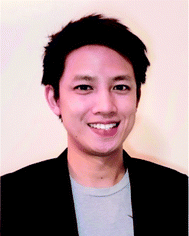
Gabriel Loke
| Gabriel Loke is currently working on his PhD in Material Science and Engineering at the Massachusetts Institute of Technology (MIT) under the supervision of Prof. Yoel Fink. He earned his dual degree in Physics and Material Science from National University of Singapore in 2015. His research interests include the fabrication of novel multifunctional fibers for optoelectronic and computing applications, as well as the synergy between multimaterial fibers and additive manufacturing. |
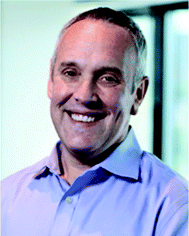
Yoel Fink
| Yoel Fink is a Professor of Materials Science and Engineering and Electrical Engineering and Computer Science at the Massachusetts Institute of Technology (MIT). He previously served as the director of the Research Laboratory of Electronics and is currently serving as the Chief Executive Officer of the Advanced Functional Fabrics of America (AFFOA). His research group at MIT works on the theory, design, fabrication and characterization of multimaterial multifunctional fibers and fabrics. |
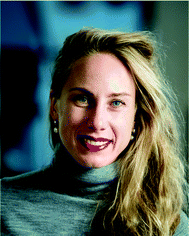
Polina Anikeeva
| Polina Anikeeva is an Associate Professor of Materials Science and Engineering and Brain and Cognitive Sciences at the Massachusetts Institute of Technology. She is an associate director of the Research Laboratory of Electronics and an associate member of the McGovern Institute for Brain Research. Her group works on the design of minimally-invasive fiber-based and magnetic approaches to study brain, spinal cord, and peripheral neural circuits. |
1. Introduction
Understanding the structure and dynamics of the mammalian nervous system is recognized as one of the most pressing issues for our aging society. Consequently, significant effort has been dedicated to the advancement of neural interfaces. In clinic, neural recording and modulation devices are routinely applied to diagnose and regulate neurological and psychiatric diseases such as Parkinson's disease,1 Alzheimer disease,2,3 epilepsy,4 and major depression.5 More recently, implantable neuroprostheses have been used to restore or rehabilitate sensorimotor function in patients affected by trauma to the central or peripheral nervous system. For example, the field of brain–computer interfaces uses recordings of neural activity acquired via electrode arrays implanted in the cortex of paralyzed human subjects to enable control of external systems such as artificial limbs, wheelchairs, and computer cursors (Fig. 1a).6,7 The signals from the cortex can similarly be applied to control subject's limb muscles, which effectively bypasses the circuits damaged by the spinal cord injury restoring motor function (Fig. 1b).8,9 Spinal neural stimulation with electrode arrays implanted epidurally and percutaneously, which are commonplace in chronic pain management, act synergistically with rehabilitation therapy to facilitate recovery of motor functions following spinal cord injury.10,11
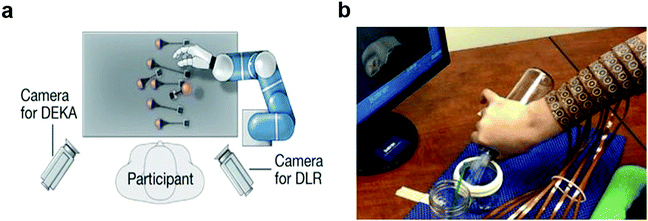 |
| Fig. 1 Clinical applications of neural Interfaces. (a) Control of robotic arm with brain-machine interfaces. Reproduced with permission from ref. 6. Copyright 2012 Nature Publishing Group. (b) Restoring cortical control of functional movement with neuromuscular electrical stimulation interface. Reproduced with permission from ref. 8. Copyright 2016 Nature Publishing Group. | |
In the peripheral nervous system, neural interfaces have been employed to restore sensory function. The hallmark example is the cochlear implant.12 Furthermore, as the understanding of how the central and peripheral nervous systems (CNS and PNS) work in unison to orchestrate the function of peripheral organs continues to expand, therapies involving peripheral nerve modulation have been proposed for a myriad of conditions previously not considered neurological. In particular, vagal nerve stimulation has been extensively studied as a way to modulate the immune system and to alleviate depression.13–15
When developing neural interfaces, it is essential to consider the function, structure, and biophysical properties of the target region of the nervous system. Since electrical and chemical signalling are at the core of neuronal function, neural interfaces should be designed to sense or modulate changes in neuronal potentials and neurotransmitters. While patch-clamp electrophysiology delivers intracellular potentials with unmatched spatiotemporal precision, it is a labour-intensive method with limited throughput and is challenging to implement in behaving subjects.16,17 Electrodes placed extracellularly permit recording of isolated high-frequency single-neuron potentials (spikes) and time- and space-averaged local field potentials (LFPs).18–21 While spike rates are often correlated to observed behaviours in fundamental neuroscience studies, LFPs are indispensable in clinical neuroscience and diagnostics of neurological disorders.22–24
Despite their essential role in neuronal signalling, there are fewer tools to detect neurotransmitters. The fast-scan cyclic voltammetry (FSCV), which records redox curves and microdialysis combined with spectroscopic detection of chemical signatures, remains the dominant chemical sensing technique in the nervous system.25–27 While FSCV delivers temporal precision comparable to electrophysiological recording of neuronal voltages, it is only applicable to a handful of molecules (dopamine, serotonin) with signatures sufficiently distinct from the other local species.28 In contrast, microdialysis is largely agnostic to the chemical properties of the compounds of interest, but has limited temporal and spatial resolution.29
The neural tissue comprises not only neuronal but also glial cells including astrocytes, oligodendrocytes, and microglia in the central nervous system (CNS) and Schwann cells in the peripheral nervous system (PNS).24,30,31 First considered as supportive cells, glia are increasingly recognized as mediators of signalling in the nervous system.32,33 Although these cells do not produce action potentials, they exchange ions with other glia and neurons via gap junctions and release growth factors and neurotransmitters.34 While only a handful of probes have been applied to study glial signalling in vivo,35 next generation neural interfaces will likely fill this niche to further our understanding of neuro-glial communication.
The functional role and geometric architecture of a particular region of the nervous system imparts constraints onto design of neural interfaces. In addition to controlling basic physiological homeostasis, the brain presides over all higher level functions including emotional response, memory, social interactions, and motor coordination.36 As the most evolutionary brain structure, the cortex is essential for information integration in the brain and plays a key role in cognition, consciousness, memory, and sensorimotor behaviours to name a few.37,38 Sensory and motor cortical areas have been extensively mapped to specific behaviours, and are frequently targeted by neural interfaces in the context of BCI-aided restoration of function.39,40 As the cortex forms a large-area relatively superficial structure, surface electrode arrays are often deployed to sense and modulate activity of many neurons from different cortical subregions.41 These electrocorticography (ECoG) probes generally record time and space-averaged potentials from large groups of neurons.42 While predominantly used to locate origins of seizures in epilepsy patients, these probes have recently been applied in BCI. Recently, the advances in complementary metal-oxide-semiconductor (CMOS) fabrication and signal multiplexing and demultiplexing have vastly increased the spatiotemporal resolution of these probes offering increase precision in seizure detection.43 In addition, advances in organic electronics permit for intimate interfaces of these surface probes with the cortex, which enables recording of activity of isolated single units.44
Despite these advances, deeper brain structures including deeper cortical layers and subcortical structures are usually accessed with penetrating probes. These range from insulated microwires45 and their assemblies,46 to silicon-based micro-machined arrays such as Utah arrays and Michigan probes,47,48 to fully CMOS-integrated neuropixels with hundreds of neural recording cites.49 Electrical stimulation of neural activity can be performed using the same or similar devices, and its efficacy relies on the electrode charge injection characteristics.50,51
Spinal cord and peripheral nerves present additional challenges to the design of neural interfaces. Unlike the brain which undergoes microscale movements (micromotion) with respect to the skull, the spinal cord and the peripheral nerves are subject to repeated bending and stretching deformation during normal movement.52 Furthermore, the reduced redundancy of the spinal and peripheral circuits makes these organs particularly sensitive to implantation of hardware. Hence spinal and peripheral nerve interfaces are often designed in conformal, sleeve, and cuff configurations.53 To distinguish between the thousands of axonal fibres carrying information from the CNS toward the organs and vice versa, it is often necessary to insert the recording and stimulating interfaces within the depth of the tissue.54,55
As it is essential to implant neural interfaces for extended periods of time, both the surface and penetrating probes should be reliable and biocompatible to avoid losses in performance and excessive tissue damage. A subject of several thorough reviews, foreign body response is in part be mitigated by minimizing the implant dimensions and reducing its stiffness.55–57 Both design principles are hypothesized to reduce the disruption of neuronal and glial networks, the breach of the blood–brain/spinal cord/nerve barrier, and the repeated impact on the tissue due to the (micro)motion of the brain, spinal cord, and the nerves with respect to the skull, vertebrae, or other bone to which the probe backend interfaces are commonly affixed.
Materials chemistry of the neural interfaces contributing to the interactions with the tissue, coatings mimicking extracellular matrix as well as a variety of neuro-attractive compounds are under investigation as the means of improving the long term performance of these probes.58,59
In this review, we will discuss design considerations for developing neural interfaces and introduce fiber-based neural probes as a one of the possible solutions to achieve multi-modal performance while minimizing tissue damage. We first highlight the challenges in matching the mechanical, chemical, and electrical characteristics of the nervous system, and those associated with the integration of multiple functional components into the devices necessary to achieve cell-type and circuit specificity in recording and modulation while maintaining long-term reliability. We then highlight neural engineering solutions to meet these challenges. This is followed by the discussion of the fundamental principles underlying fiber fabrication and examples of engineering applications of multimaterial fibers. Lastly, multimaterial fibers are introduced as a possible solution to achieve functional utility of optoelectronic neural interfaces capable of multimodal interrogation of brain and spinal cord circuits.
2. Design considerations for neural interfaces
2.1. Mechanical and structural properties
2.1.1. Modulus mismatch and foreign-body response.
When devices are implanted into or placed onto the surface of the neural tissue for extended periods of time, these interfaces often exhibit signatures of neuronal death and inflammatory response manifested as gliosis. Glial scars have been shown to increase in thickness and density over six weeks following implantation.60 Furthermore, axonal regrowth is inhibited in the vicinity of the implanted probes.61,62 Together, this creates a functionally insulating layer surrounding the implants, resulting in poor signal-to-noise ratio (SNR) in neural recording, reduced charge injection ability for electrical stimulation, enhanced scattering that interferes with optical interrogation, and impeded infusion and probing of (neuro)chemicals (Fig. 2).63–66
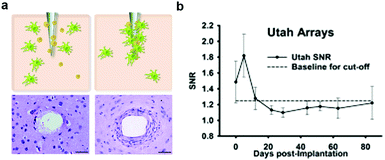 |
| Fig. 2 Glial scarring and failure of electrodes. (a) Development of glial encapsulation with implantation of microelectrode in the brain. Reproduced with permission from ref. 65. Copyright 2010 CRC Press. (b) Change of signal to noise ratio (SNR) from the recording by Utah array electrodes over a 12 week period. Reproduced with permission from ref. 66. Copyright 2013 Elsevier. | |
The foreign body response is hypothesized to stem largely from the acute damage caused by the insertion process of the probes, which evokes the destruction of cells, extracellular matrix, capillaries, and the breach of the blood–brain barrier (BBB). Repeated impact to the local environment due to relative displacement between neural tissue and probes also arise during respiration or heartbeat.55,56,65 This relative micromotion is thought to be accompanied with the disruption of glial networks67 and the breach of BBB68 which exacerbate as the stiffness of the device becomes higher.57 Consequently, significant effort has been dedicated to increasing the probes flexibility with the intent of extending their long-term function.69–71
When considering neural probes tethered to the skull or vertebrae, the bending stiffness serves as an indicator of the flexibility of the structure. The latter is defined as the force (F) required for reaching a certain deflection (d):72
|  | (1) |
Here,
E is the composite Young's modulus for the probe,
I is the moment of inertia, and
L is the length of the probe.
Therefore, the bending stiffness of implanted neural interfaces is determined by (1) the composite elastic modulus of the constituent material(s), and (2) the moment of inertia of the structure. This opens a broad design landscape to tune materials properties, as well as device dimensions and geometries, which will be described in the following sections (Sections 2.1.2 and 2.1.3).
2.1.2. Materials for neural interfaces.
Changing from hard to soft materials is one solution towards increasing flexibility of the devices. Historically materials used in neural probes had elastic moduli of few hundreds of GPa, five-to-six orders larger than that of the neural tissue. For example, microwires,73 stereotrodes (Fig. 3a),45 tetrodes (Fig. 3b),19,46 and microfabricated multi-electrode arrays (Fig. 3c and d)20,21,47,48 were produced from metals or silicon. With recent advancements in manufacturing technologies, soft materials can now be incorporated into the neural probes. For example, polymers including polyimide,74 polyurethane, poly(dimethyl sulfoxide) (PDMS), polycarbonate, polyetherimide, cyclic-olefin copolymer,70,71,75 and parylene C44,76 are commonly leveraged as substrates or packaging layers (Fig. 4a). Bioresorbable materials such as silk fibroin were also used in minimally invasive neural interfaces (Fig. 4b).77
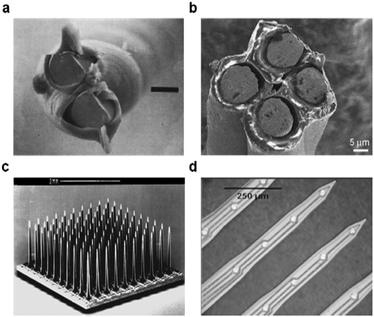 |
| Fig. 3 Conventional neural probes. (a) Scanning electronmicrograph of a stereotrode constructed by twisting together two Teflon-insulated wire. Reproduced with permission from ref. 45. Copyright 1983 Elsevier. (b) Scanning electron microscope images of a tetrode. Reproduced with permission from ref. 46. Copyright 2009 Elsevier. (c) The Utah electrode array with 100 microelectrodes projecting out from its silicon base. Reproduced with permission from ref. 47. Copyright 2006 Springer. (d) Michigan probe with four shank and 16 channels with 20 μm diameter recording sites. Reproduced with permission from ref. 48. Copyright 2004 IEEE. | |
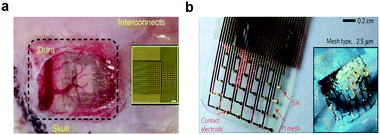 |
| Fig. 4 Neural probes with soft substrate. (a) NeuroGrid on the parylene C substrate for the recording neural activities from the surface of the brain. Reproduced with permission from ref. 44. Copyright 2015 Nature Publishing Group. (b) Devices with substrate of silk fibroin for the conformal bio-integrated electronics. Reproduced with permission from ref. 76. Copyright 2010 Nature Publishing Group. | |
In particular, hydrogels are gaining attention due to their established biocompatibility and compatible elastic moduli that could be tailored to match those of neural tissue. However, the use of hydrogels is hindered by their low-yield processing and poor electrical and optical properties. Consequently, these materials have been predominantly implemented as modulus-matching coatings on neural probes with their application as functional elements limited to transient optical interfaces.78–80 To maintain low impedance of the electrical interface with neural tissue for efficient recording and stimulation, metals are frequently used for electrodes deposited onto flexible polyimide, parylene C, or PDMS substrates via photolithographic or micro-contact printing techniques.43,81–86
Concomitantly, organic conductors are increasingly used as functional electrode materials boasting lower moduli and comparable or superior electrical properties to those of metals and crystalline semiconductors (Fig. 5). For example, a poly(vinyl alcohol) matrix doped with a workhorse material of organic electronics, poly(3,4-ethylene-dioxythiophene):poly(styrene sulfonate) (PEDOT:PSS), and a copolymer of collagen and organic semiconductor polypyrrole were used as the electrode materials.87–89 PEDOT:PSS and its chemical derivatives are vigorously studied in the context of neural interfaces due to their low elastic modulus (100 MPa) and electrochemical stability in high-salt physiological fluids.90–92 In addition to electronic conduction, PEDOT:PSS forms an ionic interface with the tissue, which facilitates high SNR recordings and enhanced charge injection capacity.90,93 In addition to its use in electrodes, PEDOT:PSS was recently integrated into organic electrochemical transistors, fabricated on flexible parylene C substrates, to function as the gate. These devices allowed for the recording of LFPs and single-unit spikes from the cortical surface with high SNR.44,94,95
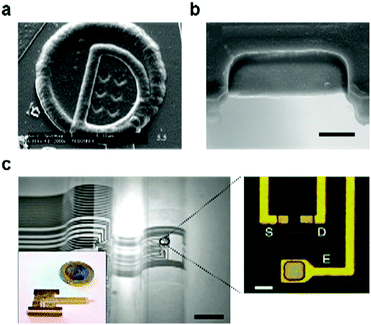 |
| Fig. 5 Organic materials for electrodes in neural interfaces. (a) PEDOT/PSS grown on the microelectrode sites of neural probes. Reproduced with permission from ref. 87. Copyright 2003 Elsevier. (b) SEM of gold electrodes after PEDOT electrochemical deposition. Reproduced with permission from ref. 94. Copyright 2011 Springer. (c) ECoG probes with organic transistors for in vivo recordings of brain activity. Reproduced with permission from ref. 95. Copyright 2013 Nature Publishing Group. | |
Carbon-based materials such as carbon nanotube (CNT) and graphene, with high mechanical robustness and conductivities comparable to those of metals, are increasingly considered as materials for miniaturized, flexible, and multifunctional neural interfaces (Fig. 6). For example, single carbon fibres with diameters of <10 μm and their arrays have recently enabled long-term recordings of isolated action potentials and LFPs with minimal damage to the surrounding tissue.62,76,96,97 Concomitantly, transparent graphene and graphene oxide electrodes deposited on planar substrates have offered electrical interface to the neural tissue coupled with simultaneous optical imaging.98–100
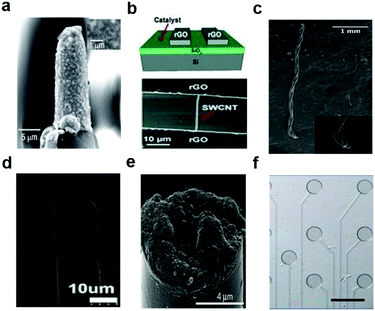 |
| Fig. 6 Carbon-based materials used in neural interfaces. (a) A sharp tungsten electrode coated with CNTs. Reproduced with permission from ref. 105. Copyright 2008 Nature Publishing Group. (b) All-carbon devices with single-walled carbon nanotubes on reduced graphene oxide electrodes. Reproduced with permission from ref. 101. Copyright 2010 Wiley. (c) Carbon nanotube (CNT) fiber electrodes for neural stimulation and recording. Reproduced with permission from ref. 109. Copyright 2015 American Chemistry Society. (d) A LBL-made CNT composite electrode in the parylene-C coating for the neural recording. Reproduced with permission from ref. 108. Copyright 2013 American Chemistry Society. (e) Microthread electrodes consisting of a carbon-fiber core with a poly(p-xylylene)-based thin-film coating. Reproduced with permission from ref. 97. Copyright 2012 Nature Publishing Group. (f) Graphene-based carbon-layered electrode array for neural recording and optogenetic stimulation. Reproduced with permission from ref. 99. Copyright 2014 Nature Publishing Group. | |
Composites of polymers with carbon, metal, or organic conductor nanoparticles offer an alternative route to reducing the electrode stiffness while maintaining conductivity.101–103 Composites of silver nanowires and styrene–butadiene–strylene elastomer fabricated as serpentine meshes were used to record and modulate cardiac signals,104 as well as enable interfaces with cortical, spinal, and peripheral nerve surfaces. Similarly, polymers doped with CNTs were used for recording electrodes.105 Graphene sheets were also deposited via layer-by-layer methods onto the CNT surfaces to achieve large-area soft/conductive electrodes.106–109 Being compatible with established microfabrication techniques, these carbon-based composites could also be processed into electrodes of low dimensions. These materials are mechanically and chemically stable, while simultaneously offering high conductivity.
2.1.3. Size and geometry.
Engineering the dimensions and geometry enables further reduction in the bending stiffness and the impact of the neural probes onto the surrounding tissue. The moment of inertia is proportional to the cubic thickness of the device,110,111 hence relatively small decreases in its dimensions can yield significant gains in flexibility. As mentioned above, carbon-fibre or CNT-based 5–10 μm electrodes trigger negligible immune response.62,109 Meshes, fibres, serpentines, and fractal-surfaces (Fig. 7) similarly imparted flexibility to the devices composed of materials with GPa moduli.70,112–114 Electrodes with a few micrometer thickness were deposited onto pre-strained stretchable substrates with a ribbon and serpentine patterns, which rendered them resilient to strain and allowed them to conform to curved and soft surfaces. Alternatively, ultrathin meshes with deposited electrodes could be delivered through syringes, resulting in open and floating interfaces with the brain tissue, thus minimizing the foreign body reaction.69,115 Fiber-based neural probes, which will be discussed in depth later in this review, are also examples of miniaturized neural interfaces.
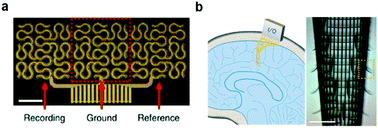 |
| Fig. 7 Flexible neural probes with different shapes. (a) Fractal-based structure for stretchable electronics. Reproduced with permission from ref. 113. Copyright 2014 Nature Publishing Group. (b) Macroporous nano-electronic networks for minimally invasive recording of brain activities. Reproduced with permission from ref. 69. Copyright 2015 Nature Publishing Group. | |
The process of inserting miniaturized and flexible neural probes into the brain, spinal cord, and nerve tissue still pose a challenge. Consequently, a variety of implantation techniques including removable guide fixtures, bioresorbable coatings, and slow insertion and injection methods have been implemented to ensure precise targeting to regions of interest within tissue depth.
2.1.4. Surface coatings to improve probe biocompatibility.
To reduce elastic and chemical mismatch between the probes and the surrounding tissue and to alleviate the inflammatory reaction to the device implantation, a variety of soft materials as well as anti-inflammatory, adhesion, and neuro-attractive factors has been explored.116–119
The environment near the implanted probe could be modified by controlling the hydrophilicity/hydrophobicity of the probe surface. For example, hydrogels composed of hyaluronic acid or polyethylene glycol (PEG) have been implemented.119,120 Furthermore, modification of surface topography was also explored.121,122 These approaches appeared to reduce gliosis near the implants, but were less effective in the long term due to the eventual degradation of the coatings.119,120
Bioactive molecules123,124 and anti-inflammatory drugs such as dexamethasone125–131 were deposited onto the probe surfaces to reduce accumulation of glia and promote neuronal survival and attachment.124,132–135 These approaches reduced the concentration of reactive oxidative species produced by the activated microglia, which minimized the size of the scar. More recently, the integration of a neuroattractive biomolecule L1 (Fig. 8)58,59,136,137 and incorporation of stem cells on the probe surface were shown to simultaneously prevent gliosis and encourage neurite growth.138,139
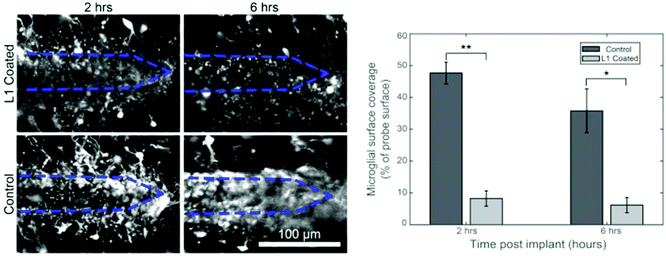 |
| Fig. 8 Coating of biomolecules on the electrode. Effect of L1 coating to prevent microglial surface coverage following 2 hours and 6 hours implantation of neural probe. Reproduced with permission from ref. 136. Copyright 2017 Elsevier. | |
2.2. Integration of functional features for selective sensing and modulation of neural circuits.
Selective modulation and sensing of specified cells within the nervous system are essential towards understanding the cells contributions to the control of physiological functions and observed behaviours. While electrical stimulation has been an indispensable tool in clinic, it lacks cell-type specificity and induces artefacts in electrophysiological recordings. Consequently, electrical tools are commonly augmented with a diversity of optical, genetic, chemical, and mechanical neural interrogation approaches.
2.2.1. Optogenetics and optical neural interfaces.
Optogenetics is the technique for modulation of genetically specified neurons and other electroactive cells with light.140–143 Optogenetics relies on optically responsive microbial ion channels and pumps, opsins, whose genes are introduced into the mammalian cells via viral transfection and electroporation, or via development of transgenic animal models. Following expression of the opsins, the modified cells, e.g. neurons, could be activated or inhibited on-demand via illumination with visible light.140 Cation channels, such as channelrhodopsin 2 (ChR2),140 cause membrane depolarization and are used for neural activation, while proton (e.g. archaerhodopsins144) and chloride (e.g. halorhodopsins145) pumps and anion channels (e.g. anion channel rhodopsins146) yield membrane hyperpolarization and are applied to neural inhibition. With optogenetics, it became possible to selectively sensitize the neuronal types of interest to certain wavelengths of light by choosing specific promoters. Furthermore, since the introduction of opsins to neuroscience more than a decade ago, a diversity of these proteins has been discovered and engineered to permit modulation of multiple independent cell types using light with wavelengths ranging across the entire visible spectrum.147–151
To apply optogenetics in the neural tissue, it is necessary to deliver the optical power equal or exceeding the threshold for opsin-mediated neural excitation or inhibition (e.g., 1 mW mm−2 for ChR2, 7 mW mm−2 for NpHR).152 The volume of the affected tissue depends on the numerical aperture of the fiber and the input power. Given the scattering and absorption within neural tissue, the light is attenuated by ∼10 fold over 1 mm.153 Therefore to manipulate neurons 1 mm below the fiber with a relatively low numerical aperture of 0.2, ∼10 mW mm−2 power density should be delivered at the tip. This translates into a relatively modest optical power of ∼180 μW for a 150 μm diameter fiber allowing to access 0.018 mm3, which contains approximately 1625 cells considering normal neuronal density of 9.2 × 104 neurons per mm.3,152 Tuning the input power and the numerical aperture of the fiber allow for broad tunability of the number of the affected cells. Recently reported tapered fibers further expand the accessible illuminated volumes and their geometries allowing for depth-tunable access to specific brain regions.154 To deliver optical power densities sufficient for optogenetic manipulation, such technical advances can be further paired with electrophysiology paralleling rapid development of the biomolecular tools with ever-increasing optical sensitivity.155
At first, light was delivered via conventional silica optical fibres adhered to tungsten156,157 or nickel–chromium158 microwires (Fig. 9a and b). Similarly, previously developed multi-electrode-arrays such as Utah-arrays159,160 and Michigan probes161–164 were outfitted with silica fibres. These combinatorial approaches have since been expanded to recording arrays164 (Fig. 9c) with integrated micro light-emitting devices (μLEDs) and transparent waveguide arrays of zinc oxide pillars165 (Fig. 9d). Fully wireless probes integrating μLEDs166,167 and laser diode chips168 have concomitantly emerged to enable optogenetics studies in complex and social environments. Most recently, tapered silica fibres were introduced to modulate depth and area of illumination which enabled illumination of multi-wavelength light to the multiple region at the same time.154
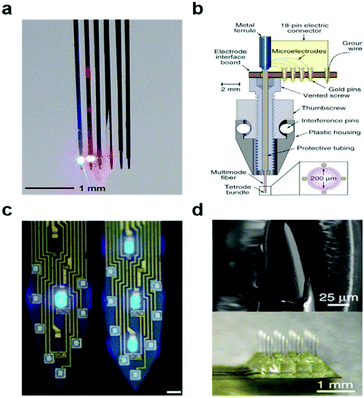 |
| Fig. 9 Optoelectronic neural devices. (a) Multidiode arrays integrated with silicon multielectrodes. Reproduced with permission from ref. 152. Copyright 2012 American Physiological Society. (b) Optetrode with combination of ferrule and Ni/Cr based tetrode bundles. Reproduced with permission from ref. 158. Copyright 2012 Nature Publishing Group. (c) Monolithically integrated μLED on silicon neural probe. Reproduced with permission from ref. 164. Copyright 2015 Cell Press. (d) ZnO MOA device for multichannel intracortical neural recording and optical stimulation. Reproduced with permission from ref. 165. Copyright 2015 Nature Publishing Group. | |
2.2.2. Neural interfaces for chemical modulation and sensing.
Neural activity can be modulated by delivering pharmacological agents such as agonists and antagonists of specific receptors into the nervous system.169 Additionally, chemogenetic tools such as DREADDs (Designer Receptors Exclusively Activated by Designer Drugs) enable chemical control of genetically identifiable neuronal populations.170 As chemogenetics and traditional pharmacology rely on infusion of compounds into the nervous system with the efficacy of intraperitoneal or intravenous injections often limited by off-target effects of the drugs or their poor transport across the BBB, there is a need for development of fluid delivery modules for neural interfaces. Soluble factors are traditionally delivered via a simple cannula composed of stainless steel or plastic connected by flexible tubing to an external or implanted pump.
More recently, microfluidic channels were integrated into neural probes to allow for simultaneous delivery of soluble factors and electrophysiological recording.171–174 These integrated channels were also used to deliver the viral vectors in studies reliant on genetic modification. Using these devices in conjunction with optogenetics and pharmacology further enabled identification of the recorded cell types.175,176 To minimize the impact on the surrounding tissue akin to the electrophysiological recording devices, these combined probes could be fabricated from softer materials including polyimide and parylene C.177
Chemical factors were also delivered into the tissue using liquid-loaded nanoparticles deposited onto electrodes and selectively triggered by external thermal, optical, and acoustic stimuli.178–182 Additionally, coatings of PEDOT nanotubes,183,184 CNT,185 nanoporous gold,186–188 and polymers131 deposited onto electrodes were loaded with small-molecule drugs, e.g. dexamethasone, which could then be released by applying voltage.
In addition to the introduction of chemical signals into the nervous system, sensing of chemical factors would offer insights into neurophysiological phenomena governed by exchanges of neurotransmitters or growth factors.
Due to its millisecond temporal resolution, FSCV is the most widely used method for chemical sensing in the nervous system.189 However, this method is restricted to electroactive molecules190–193 (e.g. dopamine, norepinephrine, and serotonin), and its selectivity is impeded in environments with comparable concentrations of multiple electroactive species. FSCV is commonly performed with carbon paste or carbon fibre electrodes and metal microwires.194–196 Similar to electrophysiological recording, probes miniaturization of the voltammetry electrodes can reduce the foreign body reaction.197,198 Small dimensions, however, limit its sensitivity. To compensate for this, nanostructures such as nanoporous gold, carbon nanofiber, CNTs, and graphene flakes were coated on the electrodes.199–202 Immobilization of enzymes specific to the neurotransmitters of interest can also improve the sensitivity and selectivity of the voltammetry probes (Fig. 10a).203 Applying FSCV to detect hydrogen peroxide produced in enzymatic reactions can further expand this method to non-electroactive neurotransmitters such as glutamate,204 acetylcholine,205 and adenosine.206
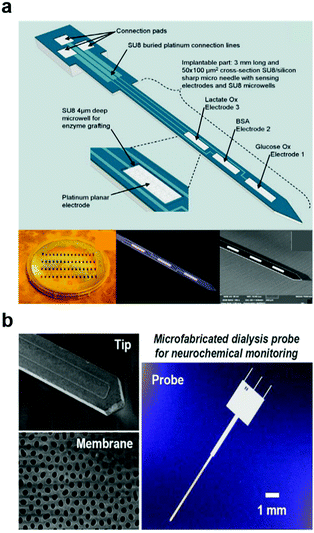 |
| Fig. 10 Neural devices for chemical monitoring. (a) Multi-electrode micro-needle for in vivo neurochemical monitoring. Reproduced with permission from ref. 204. Copyright 2015 Elsevier. (b) Microdialysis probe for neurochemical monitoring. Reproduced with permission from ref. 209. Copyright 2016 American Chemical Society. | |
In addition to voltammetry, chemical sensing can be achieved by sampling of the extracellular fluid via microdialysis (Fig. 10b)207,208 combined with external spectroscopic detection of species of interest. Microdialysis probes typically consist of two channels, one of which is outfitted with a semipermeable membrane that prevents microscale debris from contaminating the sample. The system then works by perfusing the physiological fluid in a push–pull fashion delivering a sample carrying a representative chemical load to the external analytic tools. In the past years, a diversity of microdialysis membranes was engineered including porous anodic alumina produced by deep reactive ion etching208 and nanoporous polymers obtained by laser-induced phase separation.209 Although microdialysis enables detection of non-electroactive molecules and large proteins such as beta amyloid,210,211 this method is limited by its low temporal resolution and the demand for large sample quantities. While recent advances such as introduction of capillary electrophoresis212 into microdialysis probes reduced the sample volume needs, new approaches are needed to access the temporal resolution and chemical diversity of neuronal and glial signalling.
2.2.3. Multifunctional probes.
To simultaneously monitor and modulate neural activity, multiple functional features, including electrodes, optical waveguides and light sources, chemical sensing and delivery modules, have been integrated into the probes.213–216 For example, electrophysiological recording and simultaneous release of an inhibitory neurotransmitter GABA from a nanostructured polymer reservoir were demonstrated using bioelectronic neural pixels49 (Fig. 11a). Likewise, Utah arrays and Michigan probes have been outfitted with microfluidic channels to allow for chemical delivery.216,217 Notably, it was possible to monitor electrical and chemical signals via the same electrodes. Suzuki and colleagues developed soft parylene-C based neural probes that integrated electrodes and microfluidic channels to target peripheral nerve activity (Fig. 11b).182,218 In these devices, gold electrodes for neural recording were deposited onto a 10 μm-thick photoresist (AZP4620) layer, which was sandwiched between two 10 μm-thick Parylene C films, resulting in a flexible structure. Minev et al. deposited soft platinum–silicone composite electrodes and stretchable gold interconnects onto the surface of low-modulus silicone substrates to produce a compliant and mechanically resilient probe compatible with chronic implantation onto the spinal cord (Fig. 11c).86 This device termed ‘e-dura’ allowed for drug delivery, electrical excitation, and electrophysiological recording which enabled combinatorial electrical and chemical neuromodulation to facilitate recovery of locomotor function following spinal cord injury in rodents.
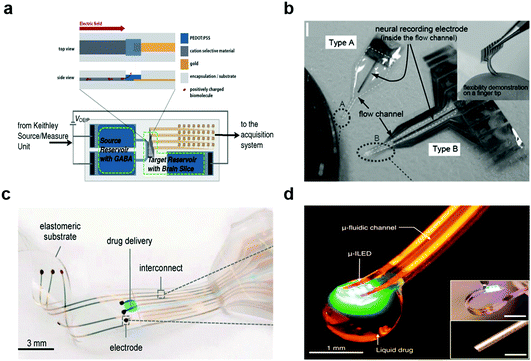 |
| Fig. 11 Multifunctional hybrid neural devices. (a) Bioelectronic neural pixel for simultaneous chemical simulation and electrical sensing. Reproduced with permission from ref. 49. Copyright 2016 Proceeding of the National Academy of Science of the United States of America. (b) Photo of Parylene flexible neural probes integrated with microfluidic channels. Reproduced with permission from ref. 218. Copyright 2005 Royal Society of Chemistry. (c) Electronic dura mater (e-dura) for long-term multimodal spinal cord interfaces. Reproduced with permission from ref. 86. Copyright 2015 American Association for the Advancement of Science. (d) Opto-fluidic neural probe during simultaneous drug delivery and photo-stimulation. Reproduced with permission from ref. 176. Copyright 2015 Cell Press. | |
As mentioned above, the introduction of optogenetics motivated the development of multifunctional probes equipped with light delivery capabilities.99,100,219–221 Kim and colleagues have leveraged microcontact printing techniques to integrate μLEDs, photodetectors, temperature, and later, microfluidic injection modules within flexible devices produced on polymer substrates.167 Similar probes have been outfitted with wireless antennae for power delivery (Fig. 11d).176 These devices enabled delivery of viral vectors carrying opsins into the mouse brain, and then afforded optogenetic neuromodulation in untethered subjects in open environments. Furthermore, flexible and stretchable optogenetic devices produced under similar design guidelines were compatible with applications in the spinal cord and peripheral nerves.222
Despite these advances, integration of electrophysiological recording with optical and chemical interrogation capabilities while tailoring the size and geometry of the micro/nano-sized devices remains a challenge. Lithographic and microcontact printing techniques rely on many fabrication steps, which often results in reduction of device yield or demands an increased probe footprint. More importantly, backend connectors necessary to interface with multiple functional features present a formidable challenge to insulation and packaging. Although wireless optogenetic devices open exciting applications in behavioral studies in freely moving animals, there remains a need for the wire-linked systems to transfer massive electrophysiological datasets as well as to minimize potential absorption of the radiofrequency electromagnetic waves by the tissue and heating of the optoelectronic components.223
In the following sections, we will introduce neural interface devices based on multimaterial fibers as potential solutions to these hurdles. We will first describe the underlying principles of fiber drawing and introduce applications of this technology as sensors, actuators, and optical components. We will then elaborate on the extension of fiber-based fabrication to applications in neuroscience and neural engineering.
3. Fundamentals of fiber drawing process and applications of fiber-based devices
With the emergence of multimaterial fibers, multifunctional optoelectronic devices with microscale and nanoscale features could be produced with high throughput from their macroscale models. Being compatible with polymers and composites, the fiber drawing process offered an intriguing route towards producing flexible and multifunctional devices. Prior to describing fiber-based optoelectronic neural probes, in this section we introduce the fundamental principles of thermal-drawing and fiber fabrication, and later discuss state-of-the-art engineering applications of multimaterial fibers.
3.1. From traditional fibers to multimaterial fibers
The word “fibers” is typically referred to string-like objects comprising of a single material. In the textile industry, fibers are the building blocks of fabrics. As we look beyond aesthetic and protective use of fibers, integration of functional features into an one dimensional form factor delivers a wealth of applications in engineering and biomedicine. For instance, silica fibers form the basis of the optical telecommunication224,225 to send and receive light signals over distances in the length scale of kilometres. In the medical field, laser oblation surgery often relies on fiber waveguides,226–228 while in the civil engineering field, kilometre-long optical fibers are placed in bridges229,230 and pipelines231,232 to monitor the emergence of structural damage. For decades, optical fibers remained as simple core-clad structures composed predominantly of single material, glassy silica. Fibers, however, can be designed and fabricated into complex structures containing multiple materials classes, such as metals, insulators, and semiconductors that form functional devices. Recent work based on the preform-to-fiber thermal drawing illustrates the broad range of functions achievable in multimaterial fibers that include photonic bandgap transmission,233 to sensors of optical,234 thermal,235 chemical,236 acoustic,237 and mechanical signals.238 Notably, the multifunctional fibers can be produced from polymers with moduli lower than that of metals and glasses, and with tunable cross sectional geometry and dimensions on the micrometer scale, which suggests their use as minimally invasive interfaces to biological systems.
3.2. Thermal drawing of multimaterial fibers
The fabrication of a multimaterial fiber begins with the construction of a macroscopic preform. This preform contains all materials in architecture identical to that of the desired fiber, but larger in its cross-sectional dimensions and shorter in length. The preform and the drawn fiber usually contain a multimaterial functional domain, in which the shapes, positions, and structures of all materials and interfaces are well defined. This domain is always cladded by an amorphous thermoplastic that supports the stresses that arise during thermal drawing. The preform materials are selected not only for the desired properties such as electrical conductivity or optical transparency but also for their ability to be co-drawn. This implies that the chosen materials possess similar viscosities at the drawing temperature to flow together and to avoid unintentional capillary breakup and intermixing due to flow instabilities.239
To build these preforms, the cladding material and the constituent materials within the functional domain are usually prepared separately.240 For instance, conductive metallic strips can be commercially purchased or machined to desired dimensions. Conductive carbon-loaded polymer composites can be similarly milled or laser cut into strips from bulk films. Semiconducting components, for example chalcogenide glasses, can be prepared by molding from their powder form (Fig. 12a). The chalcogenide glasses can also be fabricated as thin films (Fig. 12b) by thermal evaporation onto polymer substrates. The cladding material is assembled either by film rolling or film stacking. Alternatively, extruded polymer rods can be used as the cladding followed by milling, drilling or lathing to produce slots and holes where other materials could be inserted. The final step of the preform fabrication is consolidation in vacuum at an elevated temperature to eliminate air spaces between the functional components.
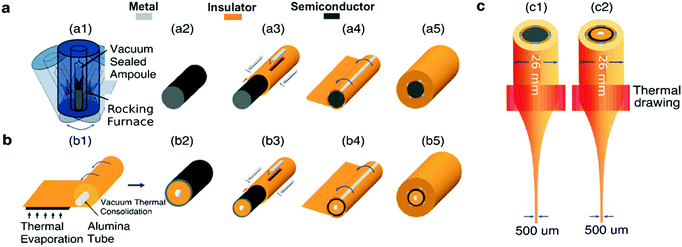 |
| Fig. 12 Preform-to-fiber steps. (a) Semiconducting chalcogenide glass powder melts and merge within a heated rocking furnace (a1) into a homogeneous rod (a2) that is inserted into the hollow slot of a drilled cylindrical macro-rod of the cladding material (a3). Metallic or conductive polymer strips are slotted into milled channels to interface with the chalcogenide glass rod, resulting in a metal–semiconductor–metal (MSM) architecture. The polymer rod with metallic and semiconducting components is then rolled (a4) with a polymeric film, before consolidation in a vacuum oven to obtain a homogenous solid preform (a5). (b) Chalcogenide glass thermally evaporated with a controlled thickness onto a polymeric sheet, before rolling (b1), consolidating (b2) and later inserting into a hollow rod together with metallic strips (b3). Similarly, the structure is enclosed with rolling films (b4) and consolidated (b5). (c) Thermal drawing of the assembled macroscopic preform within a vertical furnace results in sub-millimeter solid-core (c1) and thin-film fiber devices (c2). Reproduced with permission from ref. 240. Copyright 2007 Wiley. | |
This assembled multimaterial preform is then placed into a vertical furnace within a (thermal) draw tower, heated 50–100 °C above the glass transition (Tg) and melting (Tm) temperatures of the constituent materials and drawn into a fiber (Fig. 12c). At these temperatures, the materials within the preform possess reduced viscosity, which results in their flow. Application of a pulling force yields the preform necking and stretching into a fiber. This force is typically exerted by a capstan with a controlled speed, vcapstan. Simultaneously, the preform is advanced into the furnace with a given feed speed, vdownfeed (Fig. 12). Due to the conservation of volume, the draw-down ratio, r, between the dimensions of the preform (Dpreform) and drawn fiber (Dfiber) is defined by the following equation:
| 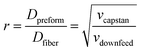 | (2) |
Hence, by using capstan speed significantly higher than the downfeed speed, one can draw kilometre-long fibers with preserved materials architectures and cross-sectional dimensions of the features reduced by a draw-down ratio as large as 500. In the context of neural interfaces, the thermal drawing method can potentially produce tens of thousands of centimetre-long probes from a single preform.
3.3. In-fiber devices
Afforded by a wide range of co-drawable material combinations, a diversity of device functionalities can be established within fibers. In this sub-section, we discuss several examples of multimaterial fibers with applications across various engineering fields (Table 1).
Table 1 Examples of fiber-based devices
Fiber functionalities |
Materials |
Current performance and capabilities |
Ref. |
Photodetectors (mechanism: makes use of the photoresistive effect) |
• Cladding: high transparency thermoplastic, e.g. polysulfone. |
• Bandwith: 390–830 nm. |
241–245
|
• Semiconductor: chalcogenide-based, e.g. As40Se49Te11Sn5. |
• Noise equivalent power: 25 nW cm−1/2 illuminated at 674 nm. |
• Electrodes: low melting point metal, e.g. Sn95Ag5. |
• Able to detect direction and spectrum of impinged light. |
|
Temperature sensing (mechanism: utilizes the thermal resistive effect) |
• Cladding: thermoplastic, e.g. polysulfone. |
• Flexible (conformable to curved objects). |
235 and 246
|
• Semiconductor: chalcogenide-based, e.g. Ge17As23Se14Te46. |
• Exponential decrease in resistance (R) as temperature (T) increases. |
• Electrodes: low melting point metal, e.g. Sn96Ag4. |
• R ∝ exp(ΔE/kBT), ΔE = 0.58 eV |
|
Chemical sensing (mechanism: detecting the light produced when sensing agents interact with chemicals of interest) |
• Fiber design: hollow channel coated with sensing agent and in-fiber Se-based photoconductor. |
• Sensitivity: detection of peroxide vapour concentration as low as 10 ppb. |
236 and 247
|
• Cladding: thermoplastic, e.g. polysulfone. |
|
• Electrodes: conductive polycarbonate (carbon black fillers). |
|
• Semiconductor used in photoconductor: Se97S3. |
|
|
Micro electro mechanical systems (mechanism: electrostriction or piezoelectricity) |
• Cladding: insulating polymer, e.g. polycarbonate. |
• Thickness mode: electric field of 25 MV m−1 results in 8% strain |
248
|
• Electrostrictive polymer: P(VDF–TrFE–CFE). |
• Bending mode: 200 V yields 80 μm deflection for a 3.5 cm fiber |
• Electrodes: carbon-loaded polyethlyene. |
• Tunable AC resonant frequency by varying fiber dimensions |
|
Elastic optical and conductive fibers (mechanism: based on stretched coiled helix) |
• Cladding: thermoplastic elastomers, e.g. SEBS. |
• Stretchable up to 500% strain. |
250
|
• Electrodes for elastic conductive fiber: liquid metals or alloys, e.g. gallium or galinstan. |
• Stretchable conductive fiber can be used as a strain sensor measuring force as low as 0.01 N. |
• Optical fiber design: coiled PC in SEBS cladding. |
• Optical loss is 0.5 dB cm−1 |
3.3.1. Photodetecting fibers.
Semiconductors are the building material blocks of modern integrated optoelectronics.241 A photodetector is a ubiquitous element of modern telecommunications; this device typically constitutes a semiconductor p–n diode junction contacted by adjacent electrodes. A simpler structure is a photoconductor with conductivity depending on optical illumination power. The latter structure has been realized by Abouraddy et al.242 in a fiber containing a low glass transition amorphous arsenic-based chalcogenide semiconductor (As40Se49Te11Sn5) and low melting-point metallic alloy of tin–silver (Sn–5% Ag) that could be co-drawn together (Fig. 13a) within a thermoplastic polysulfone (PSU) cladding. The PSU cladding was chosen for its transparency to visible and infrared light. Upon exposure to light (wavelengths 390–830 nm), As40Se49Te11Sn5 generates electron–hole pairs that can be collected as a current by the adjacent tin–silver electrodes in response to an applied voltage (Fig. 13b and c). Photodetecting elements disposed along the length of the fiber may enable sensing of light across large volumes. Furthermore, a photodetector or a photoconductor with a fiber form factor can be flexible due to its high aspect ratio and resulting low bending stiffness.
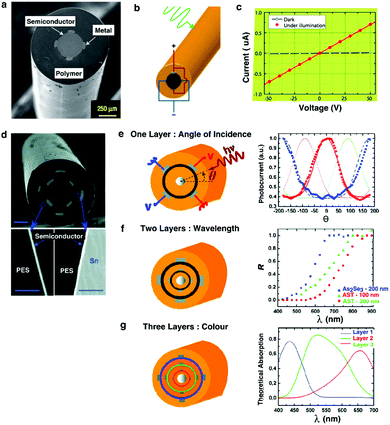 |
| Fig. 13 Metal–semiconductor–metal (MSM) fiber. (a) Cross-sectional SEM of the photodetecting MSM fiber. (b) Electrical connections on the fiber with external light impingment. (c) Current–voltage measurement of photodetecting fiber under the dark and in the light indicating the responsivity of fiber to visible light. Reproduced with permission from ref. 242. Copyright 2006 Nature Publishing Group. (d) SEM of a dual thin-film semiconducting fiber device with multiple tin electrodes. (e) Multilayered fiber device yields more information. (e1) A single layer of ring semiconductor informs about the angle of incidence. (e2) Two layers of semiconductor resolve the light wavelength. The graph indicates the ratio (R) of inner and outer layer photocurrents for different glass thicknesses and composition. (e3) Three layers give information of the light spectrum. The graph depicts the theoretical light absorption curve for each layer. Reproduced with permission from ref. 243. Copyright 2009 American Chemical Society. | |
Individual features within the fiber photodetector can be programmed into different shapes, dimensions and orientations (Fig. 13d).243 For example, in addition to simply collecting the signal due to the presence of light, one can also measure the angle of light incidence by shaping the semiconductor (As40Se60 or As40Se54Te6) as a ring (fabricated by thermal evaporation) within the fiber cladding. Each pair of electrodes adjacent to the semiconductor core establishes an independent photodetector. By applying the voltage across each pair of electrodes, 4 devices are effectively formed. Each of these photoconductors, assumed to be fixed in space, responds differently to the incident ray angle. From the 4 current values obtained from 4 independent photoconductors, one can resolve the angle at which the light is incident onto the fiber. Using the same approach, more photoconductors can be added into the fiber through the inclusion of additional concentric semiconducting rings to extract additional information about the incident light. For example, the spectrum of light can be detected (Fig. 13f and g).
The ability to detect light using fibers can, in the future, be leveraged in neuroscience studies reliant on fluorescent activity reporters. Such studies are now performed using conventional silica fibers244,245 and require sophisticated detection and amplification schemes, which can be eliminated by in-fiber conversion of optical signals into electrical currents.
3.3.2. Temperature sensing fibers.
Akin to photodetection, metal–semiconductor–metal architecture within fibers can be used for temperature sensing. Bayindir et al.235 fabricated thermal-sensing fibers (Fig. 14a) using a chalcogenide semiconductor Ge17As23Se14Te46 (GAST) core and 2 metallic electrodes (Sn96Ag4). Similar to the photoresistive mechanism in photodetecting fibers with As40Se60 or As40Se54Te6 cores described above, the heat sensing fibers make use of the thermoresistive effect. The chalcogenide semiconductor composition in GAST exhibits small electronic mobility gap allowing for large resistance and current changes in response to small changes in temperature.246 The in-fiber GAST thermoresisters exhibit ohmic behaviour in the voltage range ±50 V (Fig. 14b and c). Further characterizing the thermoresistive properties of this chalcogenide glass, an exponential relation between the resistance and the absolute temperature was determined (Fig. 14d). One can then collect temperature readings based on the measured resistance. Flexibility of fibers permits their weaving into meshes that conform to curved objects such as a human head (Fig. 14e). Collecting ohmic resistance readings from individual fibers within a woven mesh, a spatial temperature map across the head can be produced (Fig. 14f). This fiber array spatially resolved the positions of a contacting finger or an ice cube and delivered their corresponding temperature maps consistent with those obtained by a commercial infrared camera.
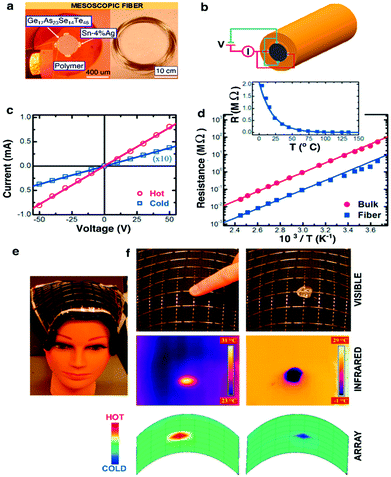 |
| Fig. 14 Thermal-electric properties of the metal–semiconductor–metal (MSM) fiber. (a) Left: Micrograph image of the MSM heat-sensing fiber. Right: Hundreds of meters of thermally drawn fiber. (b) Schematic of the MSM fiber and its electrical connections. (c) Current vs. voltage curves for two different thermal states. (d) Ohmic resistance measured for different temperatures. (e) Flexible fiber array covering the mannequin head. (f) Top: Fingertip and ice cube on top of the fiber array. Middle: Thermal map produced from a commercial IR camera. Bottom: Reconstructed thermal map from the fiber array. Reproduced with permission from ref. 235. Copyright 2006 Wiley. | |
In the context of biomedicine, thermosensing can enable studies of local inflammation or permit monitoring of heating effects during optical or electrical neuromodulation experiments akin to thermistors employed by Kim and colleagues.167
3.3.3. Chemical sensing fibers.
In-fiber chemical sensing can permit studies of chemical signalling between neurons or glia. Stolyarov et al.247 have exploited chemiluminescence mechanism to establish chemical sensing capability within fibers. In this proof-of-concept study a sensing agent for peroxide vapour was coated onto the walls of a hollow channel within a fiber (Fig. 15a). This hollow channel was surrounded by a photonic bandgap structure composed of concentric multilayers with differing indices of refraction (Fig. 15b) alternating between chalcogenide glass arsenic selenide (As2S3, refractive index n = 2.8) and polyetherimide (PEI, n = 1.66). Light emitted by oxamide-based sensing agent upon chemical reaction with the peroxide coupled into transmission modes of the photonic bandgap waveguide and was then detected at the end of the fiber (Fig. 15c). The limit of peroxide detection using this fiber architecture was 100 parts-per-billion (ppb) even following reduction of optical losses in the waveguide (Fig. 15d).
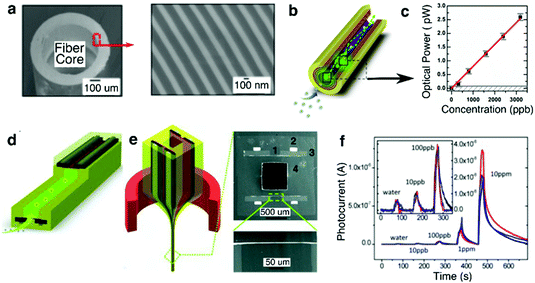 |
| Fig. 15 Chemical-sensing fibers. (a) SEM of the PBG hollow core fiber. (b) Chemical sensing mechanism: peroxide vapour (blue dots) reacts with the sensing agent (green dots), generating luminescence which is guided by the PBG structure towards the fiber end for detection. (c) Sensitivity (optical power) measurement from the PBG chemical sensing fiber. The horizontal grey line signifies the noise of the optical detector. The detection at signal-noise ratio of 1 is 100 ppb. Reproduced with permission from ref. 247. Copyright 2012 OSA Publishing. (d) Schematic of the hybrid chemical and photodetecting fiber. Light is generated from the chemiluminescence reaction, but is detected on the spot by the in-fiber detector. (e) Left: Thermal drawing of the hybrid preform. Right: Cross-sectional SEM images of the fiber: (1) PSU with a thin Se97S3 layer, (2) Sn63Pb37 electrodes, (3) CPC pads, and (4) PSU cladding. (f) Sensitivity of the hybrid fiber down to 10 ppb. Reproduced with permission from ref. 236. Copyright 2012 Wiley. | |
To further increase the sensitivity of a fiber-based chemical sensors, Gumennik et al.236 explored a hybrid fiber structure that contained a hollow channel coated with sensing agent and an integrated in-fiber selenium-based photoconductor (Fig. 15e). The photoconductor positioned along the fiber length locally collected optical signal from the sensing agent, thus eliminating the losses associated with light propagation through the photonic bandgap waveguide and external detection. This hybrid fiber was capable of detecting peroxide vapor concentration as low as 10 ppb (Fig. 15f).
3.3.4. Micro electro mechanical systems (MEMS).
In addition to sensing environmental stimuli, a fiber with actuator properties could be beneficial for stimulating neurons. Neurons and glia can perceive chemical, optical (afforded by optogenetics), and mechanical stimuli. The ability to deliver these stimuli using a fiber offered opportunities in multimodal interrogation of neural and glial circuits.
Khudiyev et al.248 reports the fabrication of an in-fiber microelectromechanical system (MEMS) based on an electrostrictive P(VDF–TrFE–CFE) (vinylidene fluoride–trifluoroethylene–chlorofluoroethylene terpolymer) ferrorelaxor (Fig. 16b and c). The choice of an electrostrictive polymer was motivated by its resilience to repeated actuation without observable hysteresis. In this fiber, the flat strip of electrorestrictive polymer was sandwiched between two conducting carbon-loaded polyethylene (CPE) electrodes, creating a parallel-plate capacitor configuration cladded by polycarbonate (PC) (Fig. 16a). The structure was surrounded by a concentric Bragg mirror composed of alternating layers of As25S75 (n = 2.27) and PC (n = 1.586) to enable the characterization of resonant vibration via optical reflectometry.249
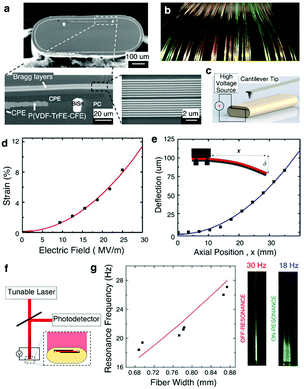 |
| Fig. 16 Fiber micro electro mechanical systems (MEMS). (a) SEM of the fiber showing the sandwiched structure of P(VDF–TrFE–CFE) polymer between CPE electrodes, as well as Bragg layers along the outer edge. BiSn contacts the CPE to increase the axial electrical conductivity. (b) Scalable large-length fabrication of the Fiber MEMS with the coloration attributed to the Bragg layers. (c) Set-up for contact profilometer measurements. (d) Thickness-mode actuation: electrostrictive strain with increasing electric field strength. Bending-mode actuation: fixed-end deflection of the fiber. (e) Optical reflectometry set-up to measure the vibration amplitude under varying voltages and frequencies. (f) Left: Resonant frequency of the fiber MEMS modulated by the fiber width. Right: Oscillation amplitude of the fiber for both off- and on-frequency points. Reproduced with permission from ref. 248. Copyright 2017 Nature Publishing Group. | |
This MEMS fiber exhibited multiple actuation modes, such as a thickness-mode, bending mode, and a resonant mode. In thickness-mode actuation, the application of an electric field of 25 MV m−1 across the CPEs contacts resulted in the contraction of the (P(VDF–TrFE–CFE)) layer in its thickness direction and expansion in its lateral direction. The strain value in this mode approached 8% exceeding that of typical piezoelectric polymers by two orders of magnitude (Fig. 16c and d). In bending mode, the application of 200 V yielded deflection of 80 μm for a 3.5 cm fiber (Fig. 16e). Lastly, by applying alternating current (AC) electric field, cantilever-like vibrations can be achieved. Its resonant frequency could be tuned by varying the fiber dimensions (Fig. 16f and g), which is readily done by changing the stresses during the thermal drawing.
3.3.5. Elastic and soft multifunctional fibers.
Elastiomers possess low stiffness, which makes them particularly well-suited to applications in biological tissues. A hallmark characteristic of elastomers is cross-linking between the polymer chains. To be compatible with thermal drawing, however, polymer chains must be able to slide along each other. Qu et al.250 overcame this challenge and demonstrated thermal-drawing of a superelastic fiber (Fig. 17a) comprised of a thermoplastic elastomer, poly(styrene-b-(ethylene-co-butylene)-b-styrene) (SEBS). SEBS contains a ratio of hard drawable phase (polystyrene) and soft elastomeric phase (poly(ethylene-co-butylene)) that can be adjusted to achieve different softening temperatures. Metallic electrodes can be embedded and co-drawn with SEBS to create a stretchable multimaterial electronic fiber. This, however, is contingent on producing stretchable electrodes. This obstacle could be circumvented by employing liquid metals or alloys such as gallium or galinstan251 that can flow, deform, and recover to maintain continuity and conductivity252–255 as the encapsulating SEBS cladding is stretched and released (Fig. 17b and c). The inclusion of such metallic electrodes did not affect the elastic properties of the SEBS-based fiber (Fig. 17d). The combination of the mechanical compliance and stretchability of SEBS fibers with the biocompatibility of Ga-based liquid metal electrodes suggests the application of these structures in biological tissue interfaces.86,256
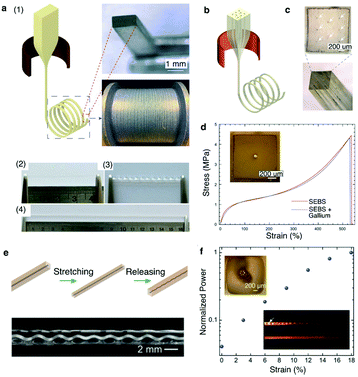 |
| Fig. 17 Superelastic multifunctional fibers. (a) (1) Thermal drawing of SEBS fiber with well-preserved rectangular cross-section. (2) SEBS fiber that can be (3) twisted and (4) stretched to 4 times its length. (b) Thermal drawing of SEBS fiber encapsulating multiple liquid metal electrodes. (c) Cross-sectional optical image of the SEBS fiber with 8 gallium electrodes at well-defined positions. (d) Stress–strain curves of the SEBS fiber with and without a single gallium electrode. (e) Top: Schematic of the PC core in SEBS cladding upon stretching and releasing. Bottom: Plastic deformation of the PC leads to its helical transformation. (f) Normalized power output at different strain levels. The top-left inset shows the shifting of the PC core away from the center due to its helical recoiling. The bottom-right inset shows light scattering from an unstrained (top) and a strained (bottom) helical PC-SEBS fiber. Reproduced with permission from ref. 250. Copyright 2018 Wiley. | |
Stretchable fibers have also been fabricated to form tunable optical waveguides by co-drawing transparent PC as the core material within the SEBS cladding. As PC is a glassy polymer, it plastically deformed following stretching of the PC/SEBS fiber with its structure coiling up into a helix upon release (Fig. 17d). The coils of the helix increased optical losses in the PC/SEBS waveguides. Upon stretching, however, the PC core returned into a straight configuration resulting in an increase in optical transmission. This tunable transmission can be used as strain gauges in engineering and biomedical applications (Fig. 17e).
3.4. Limitations of fibers and approaches to overcome them
3.4.1. Probing multiple sites along the fiber length.
Current fiber-based probes exhibit translational symmetry and only offer two exposed interfaces at the fiber ends with insulating thermoplastic cladding surrounding the rest of the structure. To increase the number of active interface sites per fiber, the fiber translational symmetry has to be broken. One approach is to leverage Rayleigh capillary instability257 which describes the transformation of a cylindrical core into thermodynamically stable spheres. This phenomenon was first exploited by Kaufman et al.,258 who demonstrated the capillary break-up of an arsenic selenide (As2S3) core embedded within a polyethersulfone (PES) fiber cladding by heating the fiber to temperatures above the As2S3 glass transition (Fig. 18a–c). Adjusting the temperature and heating time, as well as the initial diameter of the glass core (Fig. 18d), the size of the resulting spheres could be tuned from millimetres to nanometers (Fig. 18e and f).
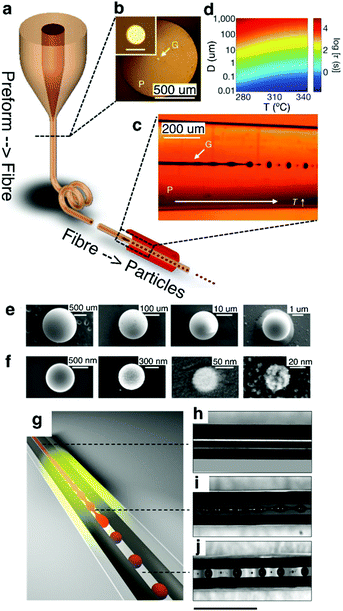 |
| Fig. 18 In-fiber capillary breakup. (a) Thermal drawing of a glassy As2S3 core-PES cladded fiber. (b) Cross-section optical image of the fiber. Inset shows a zoomed-in view of the core. Scale bar, 20 μm. (c) Calculated instability time (τ) for various temperature (T) and core diameters (D). (d) Transmission side-view optical image of the fiber after thermal processing of the fiber illustrating a clear transition from unstable continuous core to discrete spheres. SEM images of (e) microparticles and (f) nanoparticles with varying diameters. Reproduced with permission from ref. 258. Copyright 2012 Nature Publishing Group. (g) Schematic of selective breakup of As2Se5 core without affecting the continuous CPE electrodes. (h–j) Side-view optical image of the CPE–As2Se5–CPE fiber (h) before break-up where all three materials are continuous, (i) during the onset of break-up when the glass core develops instability, while electrodes remain continuous, and (j) after break-up where the chalcogenide glass spheres make connections to the continuous CPE electrodes. Scale bar, 1 mm. Reproduced with permission from ref. 259. Copyright 2016 Nature Publishing Group. | |
Rein et al.259 have extended this concept by demonstrating that the discrete spheres formed through capillary breakup could be interfaced with adjacent electrodes to create translationally discontinuous devices (Fig. 18g). In their work, arsenic selenide (As2Se5) core was first co-drawn with two parallel non-contacting conducting CPE electrodes (Fig. 18h). The semiconducting As2Se5 core was selectively broken up into discrete spherical photoconductors that formed interconnects with the continuous CPE buses (Fig. 18i and j). The next steps in the realization of multiple probing sites along the fiber length will likely require exploration of capillary breakup of electrically conductive cores into spatially localized surface-exposed spheres.
3.4.2. Optical stimulation from the sides of the fiber.
In addition to multiple probing electrodes, it is also beneficial to include multiple optical stimulation sites along the fiber.260 Conventionally, fibers transmit light along their axes with a single light output point at the fiber tip. One approach to tune the position of optical interface with the tissue is fiber tapering, which results in consecutive reduction of number of supported optical modes within the fiber core as its dimensions are reduced.154 Coupling of light at different angles then results in the modes exiting at different vertical positions along the taper. Segev and colleagues suggested new paradigm for photonic probes which employs wavelength division multiplexing.261 Photonic circuits, comprising of grating couplers, photonic waveguides, and arrayed waveguide gratings, were developed to route multispectral light through a single waveguide.
Shapira et al.262 offered yet another approach to establishing vertically defined surface illumination in fibers. In their work an inclusion of a fluorescent dye within a photonic bandgap fiber resulted in stimulated emission in the radial direction. The dye acted as an optical gain medium pumped by higher energy photons transmitted via the photonic bandgap core with the lower-frequency stimulated emission being restricted to the transverse direction. Multiple fluorescent dyes could be used to produce multiple different emitted spectra. Furthermore, the dye(s) could be incorporated at multiple positions, making it possible to achieve optical stimulation at multiple sites along the fiber.
3.4.3. Materials engineering for functional fiber performance.
Amorphous chalcogenide semiconductors exhibit limited mobilities, which translates into poor efficiencies of optoelectronic devices based on these materials as compared to crystalline semiconductors such as silicon, germanium, or III–V materials.243,263 Yet, chalcogenide semiconductors are consistently used in the thermal-drawing process due to their low Tg, which is comparable to those of polymers. To improve the fiber photoconducting efficiency, new semiconducting materials that can be co-drawable with polymers have to be investigated. Conductive materials for electrodes are also typically constrained to low-Tm metals and alloys (tin, indium, bismuth–tin, indium–bismuth, and tin–silver) or conductive polymers such as carbon-loaded polyethylene.
Some of these low-Tm metals, while highly conductive, have limited electrochemical stability and are unsuitable for charge injection. They may also pose biocompatibility concerns stemming from undesired foreign body response leading to the accumulation of glia and neuronal death.65,264,265 It is also challenging to obtain continuous sections of thermally-drawn metals, due to the tendency of cylindrical metallic cores to undergo capillary breakup at elevated draw temperatures.
In contrast, carbon-loaded composites such as CPE can be thermally drawn into long continuous sections with high fidelity and consistency of the resulting features. These polymer carbon composites are also biocompatible and flexible, which makes them attractive alternatives to low-Tm metals for biomedical applications. CPE electrical conductivity, however, is approximately 6–7 orders of magnitude lower than that of low Tm metals. To address this shortcoming in carbon composite electrodes, Guo et al.266 harnessed the alignment of polymeric chains that occurs during the thermal drawing to form carbon-fiber loaded electrodes with enhanced conductivity. High aspect ratio carbon nanofiber (CNF) was mixed into polyethylene matrix, and the resulting composite was drawn at progressively increasing stresses, which resulted in the alignment of polyethylene chains, which, in turn, drove the alignment of the CNFs. This alignment manifested in 2 orders of magnitude improvement in electrical conductivity.
While this work highlights the potential in obtaining highly conductive organic electrodes, further work is required to achieve electrical conductivity approaching that of high Tm metals such as platinum, iridium, gold, or tungsten that are typically used for recording of neuronal potentials.
3.4.4. Introducing discrete devices into fibers.
The limitations on performance of drawable semiconductors discussed above motivate alternative approaches to include active optoelectronic components within fibers. For example, direct embedment of packaged solid-state devices could circumvent the need for thermal drawing of semiconductors. Mature optoelectronic devices based on group IV or group III–V semiconductors boast high optoelectronic efficiencies, but the melting temperatures of the constituent materials make them incompatible with co-drawing with polymer claddings.
Recently, Rein et al.267 developed a method to introduce discrete microscale solid state devices such as μLEDs and photodetectors into fibers at the stage of preforms. The electrical connections to these devices are then established during the thermal drawing process (Fig. 19a). These hybrid optoelectronic fibers are capable of light emission and detection with performance characteristics determined by the embedded optoelectronic components (Fig. 19b–e). This work also details the introduction of commercially-produced microwires of high-Tm metals such as tungsten into the preform in situ during the thermal draw process, which offers the possibility to increase electrical conductivity along the fiber.
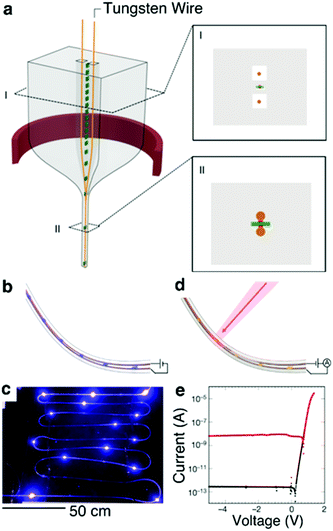 |
| Fig. 19 Diode fibers. (a) Illustration of the preform drawing process. Metallic conductive wires (orange) are fed through milled channels within the preform, which is heated and drawn (red ring). The metallic wires and devices are later embedded within the fiber during the draw process. Inset I, Schematic of the preform cross-section showing devices (green with red contact pads), and wires (orange) placed within the milled channels. Inset II, Schematic of the fiber cross section showing that the devices and wires are embedded in the fiber cladding with the wires touching the device contact pads. (b) Schematic and (c) photograph of a light-emitting fiber with discrete InGaN blue LEDs spaced throughout its length. (d) Schematic of photodiodes positioned along a light-detecting fiber. (e) Current–voltage curve of one GaAs photodiode device showing rectifying behaviour. Black and red curve is measured in darkness and under illumination, respectively. Reproduced with permission from ref. 267. Copyright 2018 Springer Nature. | |
4. Multifunctional fiber-based neural interfaces
The thermal drawing process and the multimaterial fiber devices described in Section 3 can be created to satisfy the neural probe design guidelines discussed in Section 2, which invites their applications as multifunctional interfaces to the nervous system. Fiber drawing process enables tailoring of the size and geometry of the fabricated devices,240 which can be applied to reduce the modulus mismatch between the implanted fibers and the surrounding tissue and to minimize the foreign-body response.70,71,75 In addition, simultaneous drawing of multiple materials within a single fiber allows for straightforward integration of multiple functional features enabling selective sensing and modulation of neural circuits. In this section, we will describe examples of multifunctional fibers featuring different structures and materials designed to address questions in neuroscience and neural engineering.
4.1. Multifunctional fibers for interrogating brain function
With emergence of optogenetics and, more recently, photometry with fluorescence indicators, optical fibers have become commonplace in neuroscience experiments, where they are used to transmit light to and from the nervous system. Researchers have expanded the use of glass fibers by augmenting them with additional functionalities268–270 or combining them with the systems for neural recording,154,271–274 (Table 2). However, the high-Tg materials used in these pioneering efforts possess moduli in GPa range, which may evoke a foreign body response and the formation of scattering glial scars at the optical interfaces with the tissue.
Table 2 Examples of fiber-based neural interfaces
Size |
Optical waveguides |
Electrodes |
Substrate/jacket |
Target region |
Ref. |
Material |
Number |
Loss |
Material |
Number |
Impedance |
Material |
d = diameter. Average is used for the values for optical loss and impedance. SS = stainless steel, Zeonex, PMMA = polymethylmethacrylate, PC = polycarbonate, COC = cyclic olefin copolymer, CPE = conductive polyethylene, PEI = poly(etherimide), COCE = cyclic olefin copolymer elastomer, AgNW = silver nanowire. |
Waveguide only (multi-wavelength, multi-angle, arrays) |
60 μm × 360 μm |
Quartz |
12 |
Total: 17.3 dB |
— |
— |
— |
Si |
Brain |
268
|
Propagation: 3.1 dB cm−1 |
150 μm × 150 μm |
Glass |
1 |
1.49 dB cm−1 |
— |
— |
— |
SiO2 |
Brain |
269
|
9 μm × 60 μm |
Oxynitride |
1 |
10 dB, 0.4 dB cm−1 |
— |
— |
— |
Quartz |
Brain |
270
|
|
Probes for electrical recording with optical waveguide |
d = 200 μm |
Glass |
1 |
— |
Ni/Cr alloy |
4 |
0.25 MΩ |
— |
Brain |
158
|
d = 225 μm (tapered to 50–100 μm) |
Si |
1 |
— |
Au coated Ti |
1 |
0.6 MΩ |
— |
Brain |
159
|
30 μm × 86 μm |
SU-8 |
1 |
14.3 dB, 2.3 dB mm−1 |
Ir |
8 |
1 MΩ |
Si |
Brain |
163
|
d = 310 μm (tapered to 20 μm) |
Glass |
1 |
— |
Au coated SS |
1 |
0.4 MΩ |
SS |
Brain |
219
|
d = 350 μm (tapered to 50 μm) |
Glass |
4 |
— |
W |
1 |
0.7 MΩ |
Polyimide |
Brain |
220
|
d = 260 μm |
Zeonex |
1 |
40 dB |
Au plated In |
16 |
0.15 MΩ |
PMMA |
Brain |
271
|
d = 900 μm (tapered to 6–20 μm) |
Glass |
1 |
— |
W or SS microwire |
1 |
— |
— |
Brain |
272
|
d = 100 μm (tapered to 10 μm) |
Glass |
1 |
— |
Al |
1 |
— |
— |
Brain |
273
|
15 μm × 70 μm |
Oxynitride |
1 |
10.5 dB |
Si |
8 |
1.37 MΩ |
Si |
Brain |
274
|
|
Multifunctional/flexible probes |
d = 300 μm |
PC/COC |
1 |
2.7 dB cm−1 |
CPE |
4 |
0.5 MΩ |
PC |
Brain |
70
|
d = 300 μm |
PC/COC |
1 |
1.6 dB cm−1 |
CPE |
4 |
3.0 MΩ |
PC |
Brain |
70
|
d = 85 μm |
— |
— |
— |
Sn |
7 |
0.9 MΩ |
PEI |
Brain |
70
|
d = 180 μm |
PC/COC |
1 |
1.5 dB cm−1 |
Graphite mixed CPE |
6 |
0.62 MΩ |
PC |
Brain |
71
|
d = 140 μm |
PC/COC |
1 |
1.07 dB cm−1 |
CPE |
2 |
1.3 MΩ |
PC |
Spinal cord |
281
|
125 μm × 100 μm |
COCE/PDMS |
1 |
3.98 dB cm−1 |
AgNW |
1 |
0.34 MΩ |
— |
Spinal cord |
75
|
d = 105 μm |
PC/COC |
1 |
1.9 dB cm−1 |
AgNW |
1 |
0.05 MΩ |
— |
Spinal cord |
75
|
Multimaterial fibers have offered a facile solution to combining optogenetics with other means of neural interrogation while reducing the elastic mismatch between the implanted devices and the tissue70,71,75 (Table 2). Multiple components such as optical waveguides, electrodes, or microfluidics consisting of polymers could be straightforwardly integrated into a macroscale preform and then drawn down to scales compatible with implantation into the brain, spinal cord, or peripheral nerves. Due to the nature of thermal drawing, the materials constituting the functional features of the desired probe should possess similar Tg and Tm to flow simultaneously at the drawing temperature.
Electrophysiological recording of extracellular potentials typically requires electrodes with tip impedances <1 MΩ. This is easily achieved with metal microwires with dimensions down to <10 μm. To maintain low bending stiffness and achieve resilience of the probes to repeated deformation which arises during tissue motion associated with heartbeat, respiration, and locomotion, polymer claddings offer advantages over hard and brittle glasses. Consequently, the choice of metals is limited to those with Tm sufficiently low to be co-drawn with biochemically stable polymers. Among low-Tm metals and alloys, tin and indium have emerged as non-toxic materials for neural recording electrodes compatible thermal drawing.271 High-resolution electrophysiological recording was demonstrated with fiber probes containing 7 or 36 tin electrodes embedded in poly(etherimide) (PEI) insulating cladding (Fig. 20a).70 These fiber-based electrode arrays were produced by a two-step drawing process, during which individual PEI-embedded tin electrodes of ∼200 μm in diameter were produced from macroscale preforms. The chopped sections of this fiber were then assembled into a secondary preform that was then subjected to another drawing step. The resulting electrodes had diameters 5–8 μm and tip impedances 570–1200 kΩ at 1 kHz. These miniature electrodes allowed for recording of isolated single-unit potentials in the brain of freely moving mice with SNR of 13 ± 6.
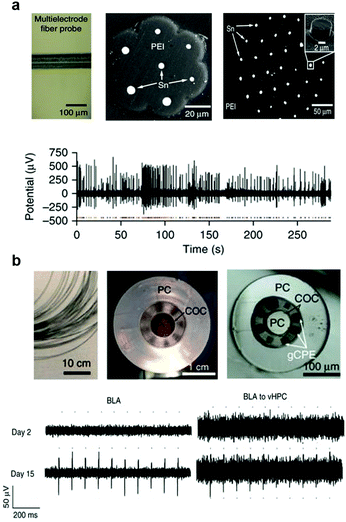 |
| Fig. 20 Flexible and multifunctional fiber probes. (a) Multielectrode PEI/Sn fiber probe to record spontaneous single-neuron activity. Reproduced with permission from ref. 70. Copyright 2015 Nature Publishing Group. (b) Multifunctional probes with PC/COC/gCPE combination for one-step optogenetics. Reproduced with permission from ref. 71. Copyright 2017 Nature Publishing Group. | |
While tin electrodes enabled electrophysiological recording within multimaterial fibers, Tm of tin (232 °C) restricted the set of available polymer claddings to high Tg plastics such as PEI (Tg = 215 °C) and poly(phenylsulfone) (PPSU, Tg = 220 °C). The latter however, exhibit substantial absorption in the visible range of optical spectrum and autofluorescence, making them unsuitable for application for optogenetics or imaging experiments.
This challenge was overcome by replacing tin electrodes with CPE, which was easily co-drawn with transparent polymers PC and cyclic olefin copolymer (COC) that exhibit disparate refractive indices to form the core and cladding of an optical waveguide. The fibers integrating PC/COC optical waveguides, two CPE electrodes, and two microfluidic channels enabled optical excitation of neurons expressing channelrhodopsin 2, ChR2, a blue-light sensitive microbial cation channel. This optically evoked activity was recorded in freely moving mice by the CPE electrodes within the fiber-probes and could be modulated by an infusion of a synaptic blocker via integrated microfluidic channels. The relatively low conductivity of commercially available CPE (0.3 s m−1 as compared to 3.5 s m−1 for tin), however, posed challenges to electrode miniaturization and limited the maximum attainable resolution and channel count within these probes.
To improve the electrophysiological recording fidelity and resolution of the all-polymer fiber based probes, custom composites of graphite and CPE (gCPE) were engineered, which reduced the sheet resistance by a factor of 4 and resulted in a 700 kΩ tip impedance at 1 kHz for six 20 × 20 μm2 electrodes drawn within a 200 μm fiber that also contained 2 microfluidic channels and a PC/COC optical core (Fig. 20b).71 These flexible fiber-probes with low-impedance electrodes allowed for tracking of spontaneous isolated action potentials in the brain of freely moving mice for 12 weeks following implantation. This long-lasting capability could likely be attributed to the reduced foreign body response to the miniature all-polymer low-bending stiffness devices. These multifunctional all-polymer fibers have also enabled recording of optically evoked potentials mediated by ChR2. In this study, ChR2 gene was delivered into the brain via injection of an adeno-associated virus through the integrated microfluidic channels.
Optogenetic interrogation of specific projections allows for manipulation of neural circuits in the context of behaviour. With the development of various viral-strategies such as retrograde viruses275 or Cre-dependent expression schemes,276 it became possible to investigate the specific projection circuits in freely moving subjects. Being miniature and light-weight, multifunctional fiber based probes can be implanted into multiple brain regions even in mice, enabling dynamic interrogation of projection circuits. As an example, wild type mice were virally transduced with ChR2 via the abovementioned fiber probes implanted in basolateral amygdala (BLA). The projection from this area to the ventral hippocampus (vHPC) was investigated via the identical fiber-based probes implanted locally within the region. This enabled tracking of the emergence of optically-evoked neuronal potentials allowing for investigation of the functional onset of ChR2 expression in cell bodies and axonal terminals. Furthermore, optical ChR2-mediated stimulation of the BLA inputs to the vHPC resulted in a previously documented anxiety phenotype,277 which could be abolished by an infusion of the synaptic blocker cyanquixaline (CNQX) into the vHPC through the microfluidic channels of the fiber.
4.2. Stretchable fiber-based spinal cord interfaces
In addition to flexibility, spinal cord interfaces must be sufficiently stretchable to be resilient to repeated strains up to 12% experienced by the spinal cord during normal movements. Furthermore, the reduced redundancy of the spinal cord circuits and the low viscoelastic modulus (0.25–0.3 MPa) of the spinal cord tissue demand minimally-invasive interfaces.54,278–280 To meet these challenges probes produced on soft substrates such as parylene C or PDMS were produced as discussed above. These compliant probes enabled optical and electrical stimulation and drug delivery into the spinal cord. Recording of corresponding neural activity, however, remained elusive. The latter may facilitate basic studies of spinal cord circuits as well as elucidate the electrophysiological origins of loss and recovery of sensorimotor functions following spinal cord injuries.
Akin to probes designed for studies of brain circuits, fiber drawing offered a strategy for integration of optical stimulation and neural recording capabilities within the spinal cord probes (Fig. 21).75,281 For instance, the stretchable and optically transparent COC elastomer was thermally drawn and outfitted with a concentric mesh of silver nanowires with 1 μm thickness. Following encapsulation with PDMS, these compliant and resilient probes integrated electrodes with exposed ring-shape tips with impedances 35–60 kΩ and optical cores with losses of 3.9 dB cm−1. These properties were sufficient to record neuronal potentials in the spinal cords of freely moving mice up to 3 months after implantation. Furthermore, the optical stimulation applied through the cores of these stretchable fibers evoked neural activity in transgenic mice broadly expressing ChR2 in the spinal cord neurons. Applied in lumbar spinal cord this optically evoked activity manifested in correlated hind-limb movements. Due to their compliance, no significant inflammatory reaction of spinal cord tissue was observed surrounding these elastomeric fiber probes.
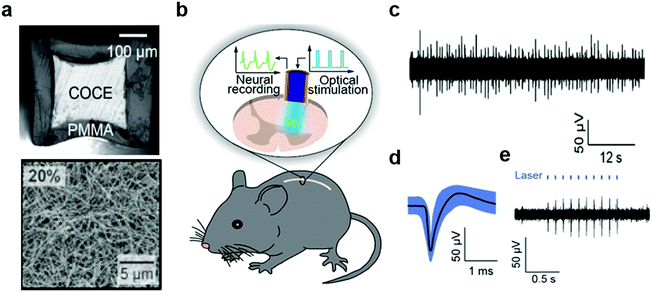 |
| Fig. 21 Stretchable nanowire-coated fiber for optoelectronic probing of spinal cord circuits. (a) Cross-sectional image of stretchable fiber probe and scanning electron microscopy image of silver nanowire mesh deposited onto the probe. (b) Schematic of experimental plan including optical stimulation and electrophysiological recording with stretchable fiber probe in a mouse spinal cord. (c) Spontaneous activity and (d) isolated action potential recorded with stretchable fiber probes in spinal cord of wild-type mouse, and (e) optically-evoked potentials recorded in Thy1-chR2-YFP mouse. Reproduced with permission from ref. 75. Copyright 2017 American Association for the Advancement of Science. | |
4.3. Optical sensing with fiber photometry
Fiber photometry has become a ubiquitous tool in neuroscience to monitor activity of genetically-identifiable neurons in deep brain regions.282–284 In photometry, excitation light is coupled into an optical fiber that transmits it into the brain where it interacts with fluorescent activity indicators, such as genetically encoded calcium indicators, for example GCaMPs.285,286 The dynamic fluorescence signal emitted by the indicators then couples back into the same fiber. The fluorescence is then transmitted to the other end of the fiber, separated from the excitation light via a dichroic mirror and finally coupled into an external photodetector (Fig. 22). So far, these experiments have relied on hard and brittle silica fibers, which is in part due to the comparatively higher losses in polymer waveguides. Materials exploration and refinement of fiber fabrication techniques is, therefore, required for the development of soft and flexible optical fibers with low transmission losses and negligible autofluorescence. Such improvements in optical properties will further advance applications in optogenetics and may permit spatially resolved imaging using fiber bundles.
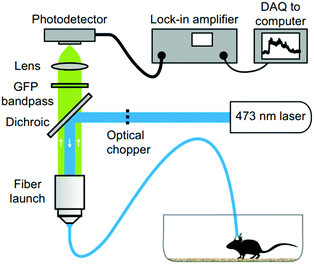 |
| Fig. 22 Fiber photometry setup for investigating neural dynamics during social interaction. Reproduced with permission from ref. 282. Copyright 2014 Nature Publishing Group. | |
4.4. Regenerative neural interfaces
Neural tissue exhibits limited regenerative ability after peripheral nerve injury, and the recovery is particularly challenging for injuries exceeding 3 cm in length. Consequently, approximately half of such injuries result in life-long disabilities.287–289 Synthetic nerve guidance channels have shown promise in facilitating regeneration in nerve gaps beyond 3 cm.290–292 Furthermore, it was revealed that engineering the geometry of the channel and the microscale topographical features of the nerve growth substrates can be used to facilitate appropriate orientation of growing neurites and enhance their growth. However, there remained a need for scalable manufacturing techniques to establish topographical features on the interior surfaces of the nerve growth scaffolds.293,294
Fiber drawing process offers straightforward means to fabricate nerve guidance scaffolds of arbitrary channel geometries from biocompatible and chemically stable polymers. By applying fiber drawing to hollow PEI preforms with cylindrical, rectangular, and grooved channels, it was possible to produce nerve guidance channels with varied geometries. Furthermore, tuning the stress experienced by the fiber during the drawing, a range of dimensions could be delivered (Fig. 23a).295 In contrast to the clinical applications of nerve guidance channels that rely on commercially available cylindrical devices with millimetre dimensions, it was found that rectangular cross sections and enhanced confinement offer superior neurite alignment and enhanced growth. Additionally, introduction of microtopographic features into the channel walls permits decoupling of the neurite alignment and directional growth from the scaffold dimensions, thus suggesting the possibility in the efficient repair of larger nerves.
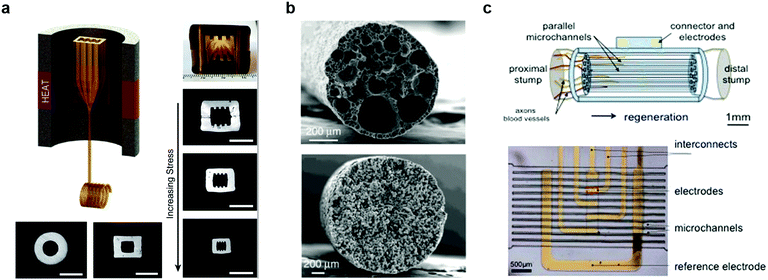 |
| Fig. 23 Fiber-based regenerative neural interfaces. (a) Thermally drawn fibers with micro-topography for the nerve guidance. Reproduced with permission from ref. 295. Copyright 2015 Elsevier. (b) Cross-sectional SEM image of porous fibers made of polycaprolactone (PCL, top) and polyvinylidene fluoride (bottom) after cladding dissolution. Reproduced with permission from ref. 296. Copyright 2017 Nature Publishing Group. (c) Micro-channels with electrodes for sciatic nerve regeneration and neural recording. Reproduced with permission from ref. 308. Copyright 2015 Nature Publishing Group. | |
Although PEI provided a favourable surface for neurite adhesion and growth in vitro, applications in vivo may benefit from improved nutrient diffusion, especially in long-gap injuries. Introducing porosity into the fiber scaffold walls will likely improve chemical exchange between the growing neurites and the external environment.
Grena et al. have recently demonstrated that porosity in fibers can be established via thermally induced phase segregation of a polymer–solvent mixture during the thermal drawing process296 (Fig. 23b). Porous fibers were fabricated from multiple polymers including biodegradable polycaprolactone (PCL) with pore dimensions ranging between 500 nm to 10 μm.
In addition to guidance scaffolds, a diversity of methods to overcome the limited regenerative ability of the peripheral nervous system have been investigated. These included delivery of growth factors,297 addition of decellularized neural tissue matrix,298 electrical stimulation,299,300 or application of electric fields.301,302 Optogenetics was also recently explored as cell-type specific alternative to electrical stimulation.303 It is expected, that the next generation scaffolds would permit precise control over the environment of growing neurites by delivering electrical, optical, chemical, and hydrodynamic stimuli via integrated electrodes, waveguides, and microfluidic channels.
Investigation of the neuronal activity and recovery within the scaffolds can be aided by in situ electrophysiological recording. Examples of devices capable of promoting regeneration and simultaneous recording from peripheral nerves include flexible cuff electrodes,304,305 transversal intrafascicular multichannel electrode (TIME),306,307 and PDMS-based microchannel scaffolds equipped with external electrodes (Fig. 23c).308,309 These scaffolds facilitated axonal regrowth 8–13 weeks following sciatic nerve trans-section, which was corroborated by the recording of neuronal firing patterns across the connected nerve. These signals were also correlated with behaviour in freely moving rodents.
Thermally drawn fibers may offer a versatile platform for development of multifunctional multimaterial scaffolds for tissue repair and in situ monitoring. By extending the principles of electrophysiology, optogenetics, and drug delivery demonstrated with flexible and stretchable fiber-based probes to porous and topographically enhanced scaffolds, it may become possible to modulate and observe nerve growth and define synaptic connections between the tissues within and outside the scaffolds.310,311
5. Conclusion and outlook
In this review, we discussed the challenges and potential approaches for developing synthetic devices to study, modulate, and repair the nervous system. Foreign body response and subsequent loss of functional performance remain key barriers to establishing long-term interfaces with the neural tissue. Although advances in soft materials and manufacturing techniques have recently delivered flexible and stealthy neural interfaces, integration of multiple functions and robust packaging continue to pose a challenge to multimodal investigation of neural circuit dynamics with spatiotemporal resolution of individual neurons and isolated action potentials.
Thermally drawn fiber-based probes are emerging as a promising solution for stable multifunctional interrogation of neural dynamics by offering scalable and straightforward integration of multiple functional features within a mechanically compliant and miniature form factor. Combined with selective neuromodulation techniques such as optogenetics and pharmacology, these tools have enabled long-term study of brain and spinal cord circuits and shown promise in enhancing tissue regeneration.
Although the thermal drawing process offered advantages in producing integrated and flexible neural interfaces, a number of engineering challenges demand additional innovation. First, the thermomechanical properties of the materials constituting the functional elements of the fiber are constrained by conditions experienced by the preform during the drawing. This can potentially be resolved by synthesis of new soft materials including polymers, composites, and hydrogels compatible with thermal drawing. Alternatively, hybrid fabrication techniques combining fiber-based processing with complementary techniques may enable integration of components that otherwise cannot be processed simultaneously. This will subsequently expand the palette of materials and features within fiber-based probes. Second, the complexity and the size of the fiber-based neural interfaces are currently limited by the back-end connectorization, which relies on labour-intensive semi-manual assembly. By coupling fiber-probes to microfabricated geometrically matching backends compatible with traditional CMOS technologies, it may be possible to establish on-board processing of electrophysiological recording as well as wireless transfer of data and power to these devices.
Overcoming the current challenges in the design and manufacturing of the fiber-based probes will facilitate their deployment as affordable tools for systems neuroscience studies that are scalable across species. The biological and materials insights delivered by these probes may then transcend studies in neuroscience and open applications in other fields of biomedicine and engineering.
Conflicts of interest
There are no conflicts to declare.
Acknowledgements
This work was supported in part by the National Institute of Neurological Disorders and Stroke (5R01NS086804), by the National Science Foundation through the Center for Materials Science and Engineering (DMR-1419807) and the Center for Neurotechnology (EEC-1028725), and by the McGovern Institute for Brain Research at MIT.
References
- P. Krack, A. Batir, N. Van Blercom, S. Chabardes, V. Fraix, C. Ardouin, A. Koudsie, P. D. Limousin, A. Benazzouz and J. F. LeBas, N. Engl. J. Med., 2003, 349, 1925–1934 CrossRef CAS PubMed.
- Z. S. Khachaturian, Arch. Neruol., 1985, 42, 1097–1105 CrossRef CAS.
- G. McKhann, D. Drachman, M. Folstein, R. Katzman, D. Price and E. M. Stadlan, Neurology, 1984, 34, 939 CrossRef CAS PubMed.
- M. Hodaie, R. A. Wennberg, J. O. Dostrovsky and A. M. Lozano, Epilepsia, 2002, 43, 603–608 CrossRef PubMed.
- H. S. Mayberg, A. M. Lozano, V. Voon, H. E. McNeely, D. Seminowicz, C. Hamani, J. M. Schwalb and S. H. Kennedy, Neuron, 2005, 45, 651–660 CrossRef CAS PubMed.
- L. R. Hochberg, D. Bacher, B. Jarosiewicz, N. Y. Masse, J. D. Simeral, J. Vogel, S. Haddadin, J. Liu, S. S. Cash and P. van der Smagt, Nature, 2012, 485, 372–375 CrossRef CAS PubMed.
- L. R. Hochberg, M. D. Serruya, G. M. Friehs, J. A. Mukand, M. Saleh, A. H. Caplan, A. Branner, D. Chen, R. D. Penn and J. P. Donoghue, Nature, 2006, 442, 164–171 CrossRef CAS PubMed.
- C. E. Bouton, A. Shaikhouni, N. V. Annetta, M. A. Bockbrader, D. A. Friedenberg, D. M. Nielson, G. Sharma, P. B. Sederberg, B. C. Glenn, W. J. Mysiw, A. G. Morgan, M. Deogaonkar and A. R. Rezai, Nature, 2016, 533, 247–250 CrossRef CAS PubMed.
- N. Bhadra and P. H. Peckham, J. Clin. Neurophysiol., 1997, 14, 378–393 CrossRef CAS PubMed.
- R. B. North, D. H. Kidd, J. C. Olin and J. M. Sieracki, Neurosurgery, 2002, 51, 381–390 Search PubMed.
- S. Harkema, Y. Gerasimenko, J. Hodes, J. Burdick, C. Angeli, Y. Chen, C. Ferreira, A. Willhite, E. Rejc and R. G. Grossman, Lancet, 2011, 377, 1938–1947 CrossRef.
- B. S. Wilson, C. C. Finley, D. T. Lawson, R. D. Wolford, D. K. Eddington and W. M. Rabinowitz, Nature, 1991, 352, 236–238 CrossRef CAS PubMed.
- D. A. Groves and V. J. Brown, Neurosci. Biobehav. Rev., 2005, 29, 493–500 CrossRef PubMed.
- M. S. George, H. A. Sackeim, A. J. Rush, L. B. Marangell, Z. Nahas, M. M. Husain, S. Lisanby, T. Burt, J. Goldman and J. C. Ballenger, Biol. Psychiatry, 2000, 47, 287–295 CrossRef CAS PubMed.
- L. V. Borovikova, S. Ivanova, M. Zhang, H. Yang, G. I. Botchkina, L. R. Watkins, H. Wang, N. Abumrad, J. W. Eaton and K. J. Tracey, Nature, 2000, 405, 458–462 CrossRef CAS PubMed.
- Y. Zhao, S. Inayat, D. Dikin, J. Singer, R. Ruoff and J. Troy, Proc. Inst. Mech. Eng., Part N, 2008, 222, 1–11 Search PubMed.
-
A. Molleman, Patch clamping: an introductory guide to patch clamp electrophysiology, John Wiley & Sons, 2003 Search PubMed.
- G. Buzsáki, Nat. Neurosci., 2004, 7, 446–451 CrossRef PubMed.
- C. M. Gray, P. E. Maldonado, M. Wilson and B. McNaughton, J. Neurosci. Methods, 1995, 63, 43–54 CrossRef CAS PubMed.
- K. L. Drake, K. D. Wise, J. Farraye, D. J. Anderson and S. L. Bement, IEEE Trans. Biomed. Eng., 1988, 35, 719–732 CrossRef CAS PubMed.
- P. K. Campbell, K. E. Jones, R. J. Huber, K. W. Horch and R. A. Normann, IEEE Trans. Biomed. Eng., 1991, 38, 758–768 CrossRef CAS PubMed.
- P. Silberstein, A. A. Kühn, A. Kupsch, T. Trottenberg, J. K. Krauss, J. C. Wöhrle, P. Mazzone, A. Insola, V. Di Lazzaro and A. Oliviero, Brain, 2003, 126, 2597–2608 CrossRef PubMed.
- A. A. Kühn, T. Trottenberg, A. Kivi, A. Kupsch, G.-H. Schneider and P. Brown, Exp. Neurol., 2005, 194, 212–220 CrossRef PubMed.
- P. Brown and D. Williams, Clin. Neurophysiol., 2005, 116, 2510–2519 CrossRef PubMed.
- M. L. Heien, M. A. Johnson and R. M. Wightman, Anal. Chem., 2004, 76, 5697–5704 CrossRef CAS PubMed.
- D. L. Robinson, B. J. Venton, M. L. Heien and R. M. Wightman, Clin. Chem., 2003, 49, 1763–1773 CrossRef CAS.
- R. Kuczenski and D. Segal, J. Neurosci., 1989, 9, 2051–2065 CrossRef CAS PubMed.
- M. Perry, Q. Li and R. T. Kennedy, Anal. Chim. Acta, 2009, 653, 1–22 CrossRef CAS PubMed.
- C. J. Watson, B. J. Venton and R. T. Kennedy, Anal. Chem., 2006, 78, 1391–1399 CrossRef PubMed.
- J. W. Fawcett and R. A. Asher, Brain Res. Bull., 1999, 49, 377–391 CrossRef CAS PubMed.
- B. A. Barres, Neuron, 2008, 60, 430–440 CrossRef CAS PubMed.
- P. G. Haydon, Nat. Rev. Neurosci., 2001, 2, 185–193 CrossRef CAS PubMed.
- R. D. Fields and G. Burnstock, Nat. Rev. Neurosci., 2006, 7, 423–436 CrossRef CAS PubMed.
- P. Bezzi and A. Volterra, Curr. Opin. Neurobiol., 2001, 11, 387–394 CrossRef CAS PubMed.
- E. Shigetomi, S. Patel and B. S. Khakh, Trends Cell Biol., 2016, 26, 300–312 CrossRef CAS PubMed.
-
E. R. Kandel, J. H. Schwartz, T. M. Jessell, S. A. Siegelbaum and A. J. Hudspeth, Principles of Neural Science, McGraw-Hill Companies, New York City, 2000 Search PubMed.
- E. K. Miller and J. D. Cohen, Annu. Rev. Neurosci., 2001, 24, 167–202 CrossRef CAS PubMed.
- R. L. West, Psychol. Bull., 1996, 120, 272–292 CAS.
- J. R. Wolpaw and D. J. McFarland, Proc. Natl. Acad. Sci. U. S. A., 2004, 101, 17849–17854 CrossRef CAS PubMed.
- A. Kübler, F. Nijboer, J. Mellinger, T. M. Vaughan, H. Pawelzik, G. Schalk, D. J. McFarland, N. Birbaumer and J. R. Wolpaw, Neurology, 2005, 64, 1775–1777 CrossRef PubMed.
- M. A. Lebedev and M. A. Nicolelis, Trends Neurosci., 2006, 29, 536–546 CrossRef CAS PubMed.
- S. Waldert, T. Pistohl, C. Braun, T. Ball, A. Aertsen and C. Mehring, J. Physiol., 2009, 103, 244–254 CrossRef PubMed.
- J. Viventi, D. H. Kim, L. Vigeland, E. S. Frechette, J. A. Blanco, Y. S. Kim, A. E. Avrin, V. R. Tiruvadi, S. W. Hwang, A. C. Vanleer, D. F. Wulsin, K. Davis, C. E. Gelber, L. Palmer, J. Van der Spiegel, J. Wu, J. Xiao, Y. Huang, D. Contreras, J. A. Rogers and B. Litt, Nat. Neurosci., 2011, 14, 1599–1605 CrossRef CAS PubMed.
- D. Khodagholy, J. N. Gelinas, T. Thesen, W. Doyle, O. Devinsky, G. G. Malliaras and G. Buzsaki, Nat. Neurosci., 2015, 18, 310–315 CrossRef CAS PubMed.
- B. L. Mcnaughton, J. Okeefe and C. A. Barnes, J. Neurosci. Methods, 1983, 8, 391–397 CrossRef CAS PubMed.
- J. E. Ferguson, C. Boldt and A. D. Redish, Sens. Actuators, A, 2009, 156, 388–393 CrossRef CAS PubMed.
- S. J. Kim, S. C. Manyam, D. J. Warren and R. A. Normann, Ann. Biomed. Eng., 2006, 34, 300–309 CrossRef PubMed.
- R. J. Vetter, J. C. Williams, J. F. Hetke, E. A. Nunamaker and D. R. Kipke, IEEE Trans. Biomed. Eng., 2004, 51, 896–904 CrossRef PubMed.
- A. Jonsson, S. Inal, I. Uguz, A. J. Williamson, L. Kergoat, J. Rivnay, D. Khodagholy, M. Berggren, C. Bernard, G. G. Malliaras and D. T. Simon, Proc. Natl. Acad. Sci. U. S. A., 2016, 113, 9440–9445 CrossRef CAS PubMed.
- G. S. Brindley and W. Lewin, J. Physiol., 1968, 196, 479–493 CrossRef CAS.
- J. V. Macpherson, Phys. Chem. Chem. Phys., 2015, 17, 2935–2949 RSC.
- S. Grillner and T. M. Jessell, Curr. Opin. Neurobiol., 2009, 19, 572–586 CrossRef CAS PubMed.
- D. Debanne, E. Campanac, A. Bialowas, E. Carlier and G. Alcaraz, Physiol. Rev., 2011, 91, 555–602 CrossRef CAS PubMed.
- S. Cheng, E. C. Clarke and L. E. Bilston, Med. Eng. Phys., 2008, 30, 1318–1337 CrossRef PubMed.
- W. M. Grill, S. E. Norman and R. V. Bellamkonda, Annu. Rev. Biomed. Eng., 2009, 11, 1–24 CrossRef CAS PubMed.
- V. S. Polikov, P. A. Tresco and W. M. Reichert, J. Neurosci. Methods, 2005, 148, 1–18 CrossRef PubMed.
- J. W. Jeong, G. Shin, S. I. Park, K. J. Yu, L. Xu and J. A. Rogers, Neuron, 2015, 86, 175–186 CrossRef CAS PubMed.
- C. L. Kolarcik, D. Bourbeau, E. Azemi, E. Rost, L. Zhang, C. F. Lagenaur, D. J. Weber and X. T. Cui, Acta Biomater., 2012, 8, 3561–3575 CrossRef CAS PubMed.
- K. Webb, E. Budko, T. J. Neuberger, S. Z. Chen, M. Schachner and P. A. Tresco, Biomaterials, 2001, 22, 1017–1028 CrossRef CAS PubMed.
- S. S. Yao and Y. Zhu, Adv. Mater., 2015, 27, 1480–1511 CrossRef CAS PubMed.
- S. Kirkpatrick, Rev. Mod. Phys., 1973, 45, 574–588 CrossRef.
- G. Guitchounts, J. E. Markowitz, W. A. Liberti and T. J. Gardner, J. Neural Eng., 2013, 10, 046016 CrossRef PubMed.
- Y. B. Lu, I. Iandiev, M. Hollborn, N. Korber, E. Ulbricht, P. G. Hirrlinger, T. Pannicke, E. Q. Wei, A. Bringmann, H. Wolburg, U. Wilhelmsson, M. Pekny, P. Wiedemann, A. Reichenbach and J. A. Kas, FASEB J., 2011, 25, 624–631 CrossRef CAS PubMed.
- M. O'Toole, P. Lamoureux and K. E. Miller, Biophys. J., 2008, 94, 2610–2620 CrossRef PubMed.
- C. Marin and E. Fernandez, Front. Neuroeng., 2010, 3, 8 Search PubMed.
- L. Karumbaiah, T. Saxena, D. Carlson, K. Patil, R. Patkar, E. A. Gaupp, M. Betancur, G. B. Stanley, L. Carin and R. V. Bellamkonda, Biomaterials, 2013, 34, 8061–8074 CrossRef CAS PubMed.
- D. H. Szarowski, M. D. Andersen, S. Retterer, A. J. Spence, M. Isaacson, H. G. Craighead, J. N. Turner and W. Shain, Brain Res., 2003, 983, 23–35 CrossRef CAS PubMed.
- T. Saxena, L. Karumbaiah, E. A. Gaupp, R. Patkar, K. Patil, M. Betancur, G. B. Stanley and R. V. Bellamkonda, Biomaterials, 2013, 34, 4703–4713 CrossRef CAS PubMed.
- C. Xie, J. Liu, T. M. Fu, X. C. Dai, W. Zhou and C. M. Lieber, Nat. Mater., 2015, 14, 1286–1292 CrossRef CAS PubMed.
- A. Canales, X. Jia, U. P. Froriep, R. A. Koppes, C. M. Tringides, J. Selvidge, C. Lu, C. Hou, L. Wei, Y. Fink and P. Anikeeva, Nat. Biotechnol., 2015, 33, 277–284 CrossRef CAS PubMed.
- S. Park, Y. Guo, X. Jia, H. K. Choe, B. Grena, J. Kang, J. Park, C. Lu, A. Canales, R. Chen, Y. S. Yim, G. B. Choi, Y. Fink and P. Anikeeva, Nat. Neurosci., 2017, 20, 612–619 CrossRef CAS PubMed.
-
F. P. Beer, Vector Mechanics for Engineering, McGraw-Hill Education, New York City, 1972 Search PubMed.
- F. Strumwasser, Science, 1958, 127, 469–470 CrossRef CAS PubMed.
- B. Rubehn and T. Stieglitz, Biomaterials, 2010, 31, 3449–3458 CrossRef CAS PubMed.
- C. Lu, S. Park, T. J. Richner, A. Derry, I. Brown, C. Hou, S. Rao, J. Kang, C. T. Mortiz, Y. Fink and P. Anikeeva, Sci. Adv., 2017, 3, e1600955 CrossRef PubMed.
- P. R. Patel, H. Zhang, M. T. Robbins, J. B. Nofar, S. P. Marshall, M. J. Kobylarek, T. D. Kozai, N. A. Kotov and C. A. Chestek, J. Neural Eng., 2016, 13, 066002 CrossRef PubMed.
- D. H. Kim, J. Viventi, J. J. Amsden, J. L. Xiao, L. Vigeland, Y. S. Kim, J. A. Blanco, B. Panilaitis, E. S. Frechette, D. Contreras, D. L. Kaplan, F. G. Omenetto, Y. G. Huang, K. C. Hwang, M. R. Zakin, B. Litt and J. A. Rogers, Nat. Mater., 2010, 9, 511–517 CrossRef CAS PubMed.
- K. C. Spencer, J. C. Sy, K. B. Ramadi, A. M. Graybiel, R. Langer and M. J. Cima, Sci. Rep., 2017, 7, 1952 CrossRef PubMed.
- M. Choi, M. Humar, S. Kim and S. H. Yun, Adv. Mater., 2015, 27, 4081–4086 CrossRef CAS PubMed.
- C. Keplinger, J.-Y. Sun, C. C. Foo, P. Rothemund, G. M. Whitesides and Z. Suo, Science, 2013, 341, 984–987 CrossRef CAS PubMed.
- B. J. Kim, J. T. Kuo, S. A. Hara, C. D. Lee, L. Yu, C. A. Gutierrez, T. Q. Hoang, V. Pikov and E. Meng, J. Neural Eng., 2013, 10, 045002 CrossRef CAS PubMed.
- J. T. Kuo, B. J. Kim, S. A. Hara, C. D. Lee, C. A. Gutierrez, T. Q. Hoang and E. Meng, Lab Chip, 2013, 13, 554–561 RSC.
- E. Delivopoulos, D. J. Chew, I. R. Minev, J. W. Fawcett and S. P. Lacour, Lab Chip, 2012, 12, 2540–2551 RSC.
- A. Srinivasan, M. Tahilramani, J. T. Bentley, R. K. Gore, D. C. Millard, V. J. Mukhatyar, A. Joseph, A. S. Haque, G. B. Stanley, A. W. English and R. V. Bellamkonda, Biomaterials, 2015, 41, 151–165 CrossRef CAS PubMed.
- J. J. FitzGerald, N. Lago, S. Benmerah, J. Serra, C. P. Watling, R. E. Cameron, E. Tarte, S. P. Lacour, S. B. McMahon and J. W. Fawcett, J. Neural Eng., 2012, 9, 016010 CrossRef PubMed.
- I. R. Minev, P. Musienko, A. Hirsch, Q. Barraud, N. Wenger, E. M. Moraud, J. Gandar, M. Capogrosso, T. Milekovic and L. Asboth, Science, 2015, 347, 159–163 CrossRef CAS PubMed.
- X. Y. Cui and D. C. Martin, Sens. Actuators, B, 2003, 89, 92–102 CrossRef CAS.
- K. A. Ludwig, J. D. Uram, J. Y. Yang, D. C. Martin and D. R. Kipke, J. Neural Eng., 2006, 3, 59–70 CrossRef PubMed.
- K. A. Ludwig, N. B. Langhals, M. D. Joseph, S. M. Richardson-Burns, J. L. Hendricks and D. R. Kipke, J. Neural Eng., 2011, 8, 014001 CrossRef PubMed.
- D. J. Lipomi, J. A. Lee, M. Vosgueritchian, B. C.-K. Tee, J. A. Bolander and Z. Bao, Chem. Mater., 2012, 24, 373–382 CrossRef CAS.
- U. Lang, N. Naujoks and J. Dual, Synth. Met., 2009, 159, 473–479 CrossRef CAS.
- A. M. Nardes, M. Kemerink, M. De Kok, E. Vinken, K. Maturova and R. Janssen, Org. Electron., 2008, 9, 727–734 CrossRef CAS.
- Y. Xiao, X. Cui, J. M. Hancock, M. Bouguettaya, J. R. Reynolds and D. C. Martin, Sens. Actuators, B, 2004, 99, 437–443 CrossRef CAS.
- J. P. Seymour, N. B. Langhals, D. J. Anderson and D. R. Kipke, Biomed. Microdevices, 2011, 13, 441–451 CrossRef PubMed.
- D. Khodagholy, T. Doublet, P. Quilichini, M. Gurfinkel, P. Leleux, A. Ghestem, E. Ismailova, T. Herve, S. Sanaur, C. Bernard and G. G. Malliaras, Nat. Commun., 2013, 4, 1575 CrossRef PubMed.
- M. Armstrong-James and J. Millar, J. Neurosci. Methods, 1979, 1, 279–287 CrossRef CAS PubMed.
- T. D. Kozai, N. B. Langhals, P. R. Patel, X. Deng, H. Zhang, K. L. Smith, J. Lahann, N. A. Kotov and D. R. Kipke, Nat. Mater., 2012, 11, 1065–1073 CrossRef CAS PubMed.
- X. Luo, C. L. Weaver, S. Tan and X. T. Cui, J. Mater. Chem. B, 2013, 1, 1340–1348 RSC.
- D. W. Park, A. A. Schendel, S. Mikael, S. K. Brodnick, T. J. Richner, J. P. Ness, M. R. Hayat, F. Atry, S. T. Frye, R. Pashaie, S. Thongpang, Z. Ma and J. C. Williams, Nat. Commun., 2014, 5, 5258 CrossRef CAS PubMed.
- D. Kuzum, H. Takano, E. Shim, J. C. Reed, H. Juul, A. G. Richardson, J. de Vries, H. Bink, M. A. Dichter, T. H. Lucas, D. A. Coulter, E. Cubukcu and B. Litt, Nat. Commun., 2014, 5, 5259 CrossRef CAS PubMed.
- B. Li, X. Cao, H. G. Ong, J. W. Cheah, X. Zhou, Z. Yin, H. Li, J. Wang, F. Boey, W. Huang and H. Zhang, Adv. Mater., 2010, 22, 3058–3061 CrossRef CAS PubMed.
- N. Rakhilin, B. Barth, J. Choi, N. L. Munoz, S. Kulkarni, J. S. Jones, D. M. Small, Y. T. Cheng, Y. Cao, C. LaVinka, E. Kan, X. Dong, M. Spencer, P. Pasricha, N. Nishimura and X. Shen, Nat. Commun., 2016, 7, 11800 CrossRef CAS PubMed.
- H. C. Tian, J. Q. Liu, D. X. Wei, X. Y. Kang, C. Zhang, J. C. Du, B. Yang, X. Chen, H. Y. Zhu, Y. N. Nuli and C. S. Yang, Biomaterials, 2014, 35, 2120–2129 CrossRef CAS PubMed.
- J. Park, S. Choi, A. H. Janardhan, S. Y. Lee, S. Raut, J. Soares, K. Shin, S. X. Yang, C. Lee, K. W. Kang, H. R. Cho, S. J. Kim, P. Seo, W. Hyun, S. Jung, H. J. Lee, N. Lee, S. H. Choi, M. Sacks, N. S. Lu, M. E. Josephson, T. Hyeon, D. H. Kim and H. J. Hwang, Sci. Transl. Med., 2016, 8, 344ra86 CrossRef PubMed.
- E. W. Keefer, B. R. Botterman, M. I. Romero, A. F. Rossi and G. W. Gross, Nat. Nanotechnol., 2008, 3, 434–439 CrossRef CAS PubMed.
- E. Jan and N. A. Kotov, Nano Lett., 2007, 7, 1123–1128 CrossRef CAS PubMed.
- Y. Bai, S. Ho and N. A. Kotov, Nanoscale, 2012, 4, 4393–4398 RSC.
- H. Zhang, P. R. Patel, Z. Xie, S. D. Swanson, X. Wang and N. A. Kotov, ACS Nano, 2013, 7, 7619–7629 CrossRef CAS PubMed.
- F. Vitale, S. R. Summerson, B. Aazhang, C. Kemere and M. Pasquali, ACS Nano, 2015, 9, 4465–4474 CrossRef CAS PubMed.
- J. A. Rogers, T. Someya and Y. Huang, Science, 2010, 327, 1603–1607 CrossRef CAS PubMed.
- D. H. Kim, R. Ghaffari, N. Lu and J. A. Rogers, Annu. Rev. Biomed. Eng., 2012, 14, 113–128 CrossRef CAS PubMed.
- D. H. Kim, N. Lu, R. Ma, Y. S. Kim, R. H. Kim, S. Wang, J. Wu, S. M. Won, H. Tao, A. Islam, K. J. Yu, T. I. Kim, R. Chowdhury, M. Ying, L. Xu, M. Li, H. J. Chung, H. Keum, M. McCormick, P. Liu, Y. W. Zhang, F. G. Omenetto, Y. Huang, T. Coleman and J. A. Rogers, Science, 2011, 333, 838–843 CrossRef CAS PubMed.
- J. A. Fan, W. H. Yeo, Y. Su, Y. Hattori, W. Lee, S. Y. Jung, Y. Zhang, Z. Liu, H. Cheng, L. Falgout, M. Bajema, T. Coleman, D. Gregoire, R. J. Larsen, Y. Huang and J. A. Rogers, Nat. Commun., 2014, 5, 3266 CrossRef PubMed.
- R. H. Kim, D. H. Kim, J. Xiao, B. H. Kim, S. I. Park, B. Panilaitis, R. Ghaffari, J. Yao, M. Li, Z. Liu, V. Malyarchuk, D. G. Kim, A. P. Le, R. G. Nuzzo, D. L. Kaplan, F. G. Omenetto, Y. Huang, Z. Kang and J. A. Rogers, Nat. Mater., 2010, 9, 929–937 CrossRef CAS PubMed.
- B. Tian, J. Liu, T. Dvir, L. Jin, J. H. Tsui, Q. Qing, Z. Suo, R. Langer, D. S. Kohane and C. M. Lieber, Nat. Mater., 2012, 11, 986–994 CrossRef CAS PubMed.
- T. D. Kozai and A. L. Vazquez, J. Mater. Chem. B, 2015, 3, 4965–4978 RSC.
- T. D. Y. Kozai, A. L. Vazquez, C. L. Weaver, S. G. Kim and X. T. Cui, J. Neural Eng., 2012, 9, 066001 CrossRef PubMed.
- E. Azemi, W. R. Stauffer, M. S. Gostock, C. F. Lagenaur and X. T. Cui, Acta Biomater., 2008, 4, 1208–1217 CrossRef CAS PubMed.
- A. W. Bridges and A. J. Garcia, J. Diabetes Sci. Technol., 2008, 2, 984–994 CrossRef PubMed.
- H. Zhang and M. Chiao, J. Med. Biol. Eng., 2015, 35, 143–155 CrossRef PubMed.
- H. Craighead, C. James and A. Turner, Curr. Opin. Solid State Mater. Sci., 2001, 5, 177–184 CrossRef CAS.
- X. Cui, J. F. Hetke, J. A. Wiler, D. J. Anderson and D. C. Martin, Sens. Actuators, A, 2001, 93, 8–18 CrossRef CAS.
- R. A. Green, R. T. Hassarati, L. Bouchinet, C. S. Lee, G. L. M. Cheong, J. F. Yu, C. W. Dodds, G. J. Suaning, L. A. Poole-Warren and N. H. Lovell, Biomaterials, 2012, 33, 5875–5886 CrossRef CAS PubMed.
- D. E. Labaye, C. Jerome, V. M. Geskin, P. Louette, R. Lazzaroni, L. Martinot and R. Jerome, Langmuir, 2002, 18, 5222–5230 CrossRef CAS.
- T. D. Y. Kozai, A. S. Jaquins-Gerstl, A. L. Vazquez, A. C. Michael and X. T. Cui, Biomaterials, 2016, 87, 157–169 CrossRef CAS PubMed.
- A. Mercanzini, S. T. Reddy, D. Velluto, P. Colin, A. Maillard, J. C. Bensadoun, J. A. Hubbell and P. Renaud, J. Controlled Release, 2010, 145, 196–202 CrossRef CAS PubMed.
- W. Shain, L. Spataro, J. Dilgen, K. Haverstick, S. Retterer, M. Isaacson, M. Saltzman and J. N. Turner, IEEE Trans. Neural Syst. Rehabil. Eng., 2003, 11, 186–188 CrossRef PubMed.
- L. Spataro, J. Dilgen, S. Retterer, A. J. Spence, M. Isaacson, J. N. Turner and W. Shain, Exp. Neurol., 2005, 194, 289–300 CrossRef CAS PubMed.
- R. Wadhwa, C. F. Lagenaur and X. T. Cui, J. Controlled Release, 2006, 110, 531–541 CrossRef CAS PubMed.
- D. H. Kim and D. C. Martin, Biomaterials, 2006, 27, 3031–3037 CrossRef CAS PubMed.
- Y. Zhong and R. V. Bellamkonda, Brain Res., 2007, 1148, 15–27 CrossRef CAS PubMed.
- W. R. Stauffer and X. T. Cui, Biomaterials, 2006, 27, 2405–2413 CrossRef CAS PubMed.
- J. C. Sanchez, N. Alba, T. Nishida, C. Batich and P. R. Carney, IEEE Trans. Neural Syst. Rehabil. Eng., 2006, 14, 217–221 CrossRef PubMed.
- E. Patrick, M. E. Orazem, J. C. Sanchez and T. Nishida, J. Neurosci. Methods, 2011, 198, 158–171 CrossRef CAS PubMed.
- A. Prasad, Q. S. Xue, R. Dieme, V. Sankar, R. C. Mayrand, T. Nishida, W. J. Streit and J. C. Sanchez, Front. Neuroeng., 2014, 7, 2 Search PubMed.
- J. R. Eles, A. L. Vazquez, N. R. Snyder, C. Lagenaur, M. C. Murphy, T. D. Y. Kozai and X. T. Cui, Biomaterials, 2017, 113, 279–292 CrossRef CAS PubMed.
- E. Azemi, C. F. Lagenaur and X. T. Cui, Biomaterials, 2011, 32, 681–692 CrossRef CAS.
- P. Kennedy, D. Andreasen, J. Bartels, P. Ehirim, H. Mao, M. Velliste, T. Wichmann and J. Wright, Prog. Brain Res., 2011, 194, 1–25 Search PubMed.
- E. Azemi, G. T. Gobbel and X. T. Cui, J. Neurosurg., 2010, 113, 673–681 Search PubMed.
- E. S. Boyden, F. Zhang, E. Bamberg, G. Nagel and K. Deisseroth, Nat. Neurosci., 2005, 8, 1263–1268 CrossRef CAS PubMed.
- F. Zhang, V. Gradinaru, A. R. Adamantidis, R. Durand, R. D. Airan, L. de Lecea and K. Deisseroth, Nat. Protoc., 2010, 5, 439–456 CrossRef CAS PubMed.
- O. Yizhar, L. E. Fenno, T. J. Davidson, M. Mogri and K. Deisseroth, Neuron, 2011, 71, 9–34 CrossRef CAS PubMed.
- L. Fenno, O. Yizhar and K. Deisseroth, Annu. Rev. Neurosci., 2011, 34, 389–412 CrossRef CAS PubMed.
- B. Y. Chow, X. Han, A. S. Dorby, X. Qian, A. S. Chuong, M. Li, M. A. Henninger, G. M. Belfort, Y. Lin, P. E. Monahan and E. S. Boyden, Nature, 2010, 463, 98–102 CrossRef CAS PubMed.
- F. Zhang, L. P. Wang, M. Brauner, J. F. Liewald, K. Kay, N. Watzke, P. G. Wood, E. Bamberg, G. Nagel, A. Gottschalk and K. Deisseroth, Nature, 2007, 446, 633–639 CrossRef CAS PubMed.
- E. G. Govorunova, O. A. Sineshcheko, R. Janz, X. Liu and J. L. Spudich, Science, 2015, 349, 647–650 CrossRef CAS PubMed.
- F. Zhang, J. Vierock, O. Yizhar, L. E. Fenno, S. Tsunoda, A. Kianianmomeni, M. Prigge, A. Berndt, J. Cushman, J. Polle, J. Magnuson, P. Hegemann and K. Deisseroth, Cell, 2011, 147, 1446–1457 CrossRef CAS PubMed.
- F. Zhang, M. Prigge, F. Beyriere, S. P. Tsunoda, J. Mattis, O. Yizhar, P. Hegemann and K. Deisseroth, Nat. Neurosci., 2008, 11, 631–633 CrossRef CAS PubMed.
- O. Yizhar, L. Fenno, M. Prigge, P. Hegemann and K. Deisseroth, J. Mol. Neurosci., 2012, 48, 128–129 Search PubMed.
- M. Prigge, F. Schneider, S. P. Tsunoda, C. Shilyansky, J. Wietek, K. Deisseroth and P. Hegemann, J. Biol. Chem., 2012, 287, 31804–31812 CrossRef CAS PubMed.
- J. Y. Lin, P. M. Knutsen, A. Muller, D. Kleinfeld and R. Y. Tsien, Nat. Neurosci., 2013, 16, 1499–1508 CrossRef CAS PubMed.
- E. Stark, T. Koos and G. Buzsaki, J. Neurophysiol., 2012, 108, 349–363 CrossRef PubMed.
- A. M. Aravanis, L. P. Wang, F. Zhang, L. A. Meltzer, M. Z. Mogri, M. B. Schneider and K. Deisseroth, J. Neural Eng., 2007, 4, S143 CrossRef PubMed.
- F. Pisanello, G. Mandelbaum, M. Pisanello, I. A. Oldenburg, L. Sileo, J. E. Markowitz, R. E. Peterson, A. Della Patria, T. M. Haynes, M. S. Emara, B. Spagnolo, S. R. Datta, M. De Vittorio and B. L. Sabatini, Nat. Neurosci., 2017, 20, 1180–1188 CrossRef CAS PubMed.
- B. Fan and W. Li, Lab Chip, 2015, 15, 3838–3855 RSC.
- V. Gradinaru, K. R. Thompson, F. Zhang, M. Mogri, K. Kay, M. B. Schneider and K. Deisseroth, J. Neurosci., 2007, 27, 14231–14238 CrossRef CAS PubMed.
- V. Gradinaru, M. Mogri, K. R. Thompson, J. M. Henderson and K. Deisseroth, Science, 2009, 324, 354–359 CrossRef CAS PubMed.
- P. Anikeeva, A. S. Andalman, I. Witten, M. Warden, I. Goshen, L. Grosenick, L. A. Gunaydin, L. M. Frank and K. Deisseroth, Nat. Neurosci., 2012, 15, 163–170 CrossRef CAS PubMed.
- J. Wang, F. Wagner, D. A. Borton, J. Y. Zhang, I. Ozden, R. D. Burwell, A. V. Nurmikko, R. van Wagenen, I. Diester and K. Deisseroth, J. Neural Eng., 2012, 9, 016001 CrossRef PubMed.
- J. Y. Zhang, F. Laiwalla, J. A. Kim, H. Urabe, R. Van Wagenen, Y. K. Song, B. W. Connors, F. Zhang, K. Deisseroth and A. V. Nurmikko, J. Neural Eng., 2009, 6, 055007 CrossRef PubMed.
- S. Royer, B. V. Zemelman, M. Barbic, A. Losonczy, G. Buzsaki and J. C. Magee, Eur. J. Neurosci., 2010, 31, 2279–2291 CrossRef PubMed.
- A. V. Kravitz, B. S. Freeze, P. R. L. Parker, K. Kay, M. T. Thwin, K. Deisseroth and A. C. Kreitzer, Nature, 2010, 466, 622–626 CrossRef CAS PubMed.
- Y. Son, H. J. Lee, J. Kim, H. Shin, N. Choi, C. J. Lee, E. S. Yoon, E. Yoon, K. D. Wise, T. G. Kim and I. J. Cho, Sci. Rep., 2015, 5, 15466 CrossRef CAS PubMed.
- F. Wu, E. Stark, P.-C. Ku, K. D. Wise, G. Buzsáki and E. Yoon, Neuron, 2015, 88, 1136–1148 CrossRef CAS PubMed.
- J. Lee, I. Ozden, Y. K. Song and A. V. Nurmikko, Nat. Methods, 2015, 12, 1157–1162 CrossRef CAS PubMed.
- K. Y. Kwon, H. M. Lee, M. Ghovanloo, A. Weber and W. Li, Front. Syst. Neurosci., 2015, 9, 69 Search PubMed.
- T. I. Kim, J. G. McCall, Y. H. Jung, X. Huang, E. R. Siuda, Y. Li, J. Song, Y. M. Song, H. A. Pao, R. H. Kim, C. Lu, S. D. Lee, I. S. Song, G. Shin, R. Al-Hasani, S. Kim, M. P. Tan, Y. Huang, F. G. Omenetto, J. A. Rogers and M. R. Bruchas, Science, 2013, 340, 211–216 CrossRef CAS PubMed.
- M. Schwaerzle, O. Paul and P. Ruther, J. Micromech. Microeng., 2017, 27, 065004 CrossRef.
- R. van den Brand, J. Heutschi, Q. Barraud, J. DiGiovanna, K. Bartholdi, M. Huerlimann, L. Friedli, I. Vollenweider, E. M. Moraud, S. Duis, N. Dominici, S. Micera, P. Musienko and G. Courtine, Science, 2012, 336, 1182–1185 CrossRef CAS PubMed.
- D. J. Urban and B. L. Roth, Annu. Rev. Pharmacol. Toxicol., 2015, 55, 399–417 CrossRef CAS PubMed.
- A. Pongracz, Z. Fekete, G. Marton, Z. Berces, I. Ulbert and P. Furjes, Sens. Actuators, B, 2013, 189, 97–105 CrossRef CAS.
- M. O. Heuschkel, L. Guerin, B. Buisson, D. Bertrand and P. Renaud, Sens. Actuators, B, 1998, 48, 356–361 CrossRef CAS.
- H. Shin, H. J. Lee, U. Chae, H. Kim, J. Kim, N. Choi, J. Woo, Y. Cho, C. J. Lee, E. S. Yoon and I. J. Cho, Lab Chip, 2015, 15, 3730–3737 RSC.
- K. Seidl, S. Spieth, S. Herwik, J. Steigert, R. Zengerle, O. Paul and P. Ruther, J. Micromech. Microeng., 2010, 20, 105006 CrossRef.
- R. Muller, H. P. Le, W. Li, P. Ledochowitsch, S. Gambini, T. Bjorninen, A. Koralek, J. M. Carmena, M. M. Maharbiz, E. Alon and J. M. Rabaey, IEEE J. Solid-State Circuits, 2015, 50, 344–359 Search PubMed.
- J. W. Jeong, J. G. McCall, G. Shin, Y. H. Zhang, R. Al-Hasani, M. Kim, S. Li, J. Y. Sim, K. I. Jang, Y. Shi, D. Y. Hong, Y. H. Liu, G. P. Schmitz, L. Xia, Z. B. He, P. Gamble, W. Z. Ray, Y. G. Huang, M. R. Bruchas and J. A. Rogers, Cell, 2015, 162, 662–674 CrossRef CAS PubMed.
- S. Metz, A. Bertsch, D. Bertrand and P. Renaud, Biosens. Bioelectron., 2004, 19, 1309–1318 CrossRef CAS PubMed.
- K. S. Soppimath, T. M. Aminabhavi, A. R. Kulkarni and W. E. Rudzinski, J. Controlled Release, 2001, 70, 1–20 CrossRef CAS PubMed.
- E. J. Anglin, L. Y. Cheng, W. R. Freeman and M. J. Sailor, Adv. Drug Delivery Rev., 2008, 60, 1266–1277 CrossRef CAS PubMed.
- I. I. Slowing, J. L. Vivero-Escoto, C. W. Wu and V. S. Y. Lin, Adv. Drug Delivery Rev., 2008, 60, 1278–1288 CrossRef CAS PubMed.
- P. Ghosh, G. Han, M. De, C. K. Kim and V. M. Rotello, Adv. Drug Delivery Rev., 2008, 60, 1307–1315 CrossRef CAS PubMed.
- D. Ziegler, T. Suzuki and S. Takeuchi, J. Microelectromech. Syst., 2006, 15, 1477–1482 CAS.
- M. R. Abidian, D. H. Kim and D. C. Martin, Adv. Mater., 2006, 18, 405–409 CrossRef CAS PubMed.
- M. R. Abidian and D. C. Martin, Adv. Funct. Mater., 2009, 19, 573–585 CrossRef CAS.
- X. L. Luo, C. Matranga, S. S. Tan, N. Alba and X. Y. T. Cui, Biomaterials, 2011, 32, 6316–6323 CrossRef CAS PubMed.
- S. D. Gittard, B. E. Pierson, C. M. Ha, C. A. M. Wu, R. J. Narayan and D. B. Robinson, Biotechnol. J., 2010, 5, 192–200 CrossRef CAS PubMed.
- E. Seker, Y. Berdichevsky, K. J. Staley and M. L. Yarmush, Adv. Healthcare Mater., 2012, 1, 172–176 CrossRef CAS PubMed.
- O. Polat and E. Seker, J. Phys. Chem. C, 2015, 119, 24812–24818 CrossRef CAS.
- D. L. Robinson, A. Hermans, A. T. Seipel and R. M. Wightman, Chem. Rev., 2008, 108, 2554–2584 CrossRef CAS PubMed.
- X. Borue, S. Cooper, J. Hirsh, B. Condron and B. J. Venton, J. Neurosci. Methods, 2009, 179, 300–308 CrossRef CAS PubMed.
- J. Park, P. Takmakov and R. M. Wightman, J. Neurochem., 2011, 119, 932–944 CrossRef CAS PubMed.
- E. A. Kiyatkin and K. T. Wakabayashi, ACS Chem. Neurosci., 2015, 6, 108–116 CrossRef CAS PubMed.
- E. A. Kiyatkin, K. T. Wakabayashi and M. Lenoir, ACS Chem. Neurosci., 2013, 4, 652–665 CrossRef CAS PubMed.
- P. E. Sheehan and L. J. Whitman, Nano Lett., 2005, 5, 803–807 CrossRef CAS PubMed.
- P. R. Nair and M. A. Alam, Appl. Phys. Lett., 2006, 88, 233120 CrossRef.
- T. M. Squires, R. J. Messinger and S. R. Manalis, Nat. Biotechnol., 2008, 26, 417–426 CrossRef CAS PubMed.
- M. L. Huffman and B. J. Venton, Analyst, 2009, 134, 18–24 RSC.
- A. L. Sanford, S. W. Morton, K. L. Whitehouse, H. M. Oara, L. Z. Lugo-Morales, J. G. Roberts and L. A. Sombers, Anal. Chem., 2010, 82, 5205–5210 CrossRef CAS PubMed.
- J. Patel, L. Radhakrishnan, B. Zhao, B. Uppalapati, R. C. Daniels, K. R. Ward and M. M. Collinson, Anal. Chem., 2013, 85, 11610–11618 CrossRef CAS PubMed.
- D. A. Zhang, E. Rand, M. Marsh, R. J. Andrews, K. H. Lee, M. Meyyappan and J. E. Koehne, Mol. Neurobiol., 2013, 48, 380–385 CrossRef CAS PubMed.
- P. Daggumati, Z. Matharu, L. Wang and E. Seker, Anal. Chem., 2015, 87, 8618–8622 CrossRef CAS PubMed.
- S. Saraf, C. J. Neal, S. Park, S. Das, S. Barkam, H. J. Cho and S. Seal, RSC Adv., 2015, 5, 46501–46508 RSC.
- N. Vasylieva, S. Marinesco, D. Barbier and A. Sabac, Biosens. Bioelectron., 2015, 72, 148–155 CrossRef CAS PubMed.
- W. H. Oldenziel and B. H. C. Westerink, Anal. Chem., 2005, 77, 5520–5528 CrossRef CAS PubMed.
- M. Sarter, V. Parikh and W. M. Howe, Nat. Rev. Neurosci., 2009, 10, 383–386 CrossRef CAS PubMed.
- L. I. Schmitt, R. E. Sims, N. Dale and P. G. Haydon, J. Neurosci., 2012, 32, 4417–4425 CrossRef CAS PubMed.
- U. Ungerstedt, J. Intern. Med., 1991, 230, 365–373 CrossRef CAS PubMed.
- W. H. Lee, T. Ngernsutivorakul, O. S. Mabrouk, J. M. T. Wong, C. E. Dugan, S. S. Pappas, H. J. Yoon and R. T. Kennedy, Anal. Chem., 2016, 88, 1230–1237 CrossRef CAS PubMed.
- S. Song, A. K. Singh, T. J. Shepodd and B. J. Kirby, Anal. Chem., 2004, 76, 2367–2373 CrossRef CAS PubMed.
- P. M. Vespa, D. McArthur, K. O'Phelan, T. Glenn, M. Etchepare, D. Kelly, M. Bergsneider, N. A. Martin and D. A. Hovda, J. Cereb. Blood Flow Metab., 2003, 23, 865–877 CrossRef CAS PubMed.
- A. W. Bero, P. Yan, J. H. Roh, J. R. Cirrito, F. R. Stewart, M. E. Raichle, J. M. Lee and D. M. Holtzman, Nat. Neurosci., 2011, 14, 750–756 CrossRef CAS PubMed.
- T. R. Slaney, J. Nie, N. D. Hershey, P. K. Thwar, J. Linderman, M. A. Burns and R. T. Kennedy, Anal. Chem., 2011, 83, 5207–5213 CrossRef CAS PubMed.
- J. K. Chen, K. D. Wise, J. F. Hetke and S. C. Bledsoe, IEEE Trans. Biomed. Eng., 1997, 44, 760–769 CrossRef CAS PubMed.
- D. P. Papageorgiou, S. E. Shore, S. C. Bledsoe and K. D. Wise, J. Microelectromech. Syst., 2006, 15, 1025–1033 CrossRef.
- D. T. Simon, S. Kurup, K. C. Larsson, R. Hori, K. Tybrandt, M. Goiny, E. H. Jager, M. Berggren, B. Canlon and A. Richter-Dahlfors, Nat. Mater., 2009, 8, 742–746 CrossRef CAS PubMed.
- A. Altuna, E. Bellistri, E. Cid, P. Aivar, B. Gal, J. Berganzo, G. Gabriel, A. Guimera, R. Villa, L. J. Fernandez and L. Menendez de la Prida, Lab Chip, 2013, 13, 1422–1430 RSC.
- R. Sheybani, A. Cobo and E. Meng, Biomed. Microdevices, 2015, 17, 74 CrossRef PubMed.
- S. Takeuchi, D. Ziegler, Y. Yoshida, K. Mabuchi and T. Suzuki, Lab Chip, 2005, 5, 519–523 RSC.
- I. Ozden, J. Wang, Y. Lu, T. May, J. Lee, W. Goo, D. J. O'Shea, P. Kalanithi, I. Diester, M. Diagne, K. Deisseroth, K. V. Shenoy and A. V. Nurmikko, J. Neurosci. Methods, 2013, 219, 142–154 CrossRef PubMed.
- K. Tamura, Y. Ohashi, T. Tsubota, D. Takeuchi, T. Hirabayashi, M. Yaguchi, M. Matsuyama, T. Sekine and Y. Miyashita, J. Neurosci. Methods, 2012, 211, 49–57 CrossRef CAS PubMed.
- S. Y. Chen, W. H. Pei, Q. Gui, Y. F. Chen, S. S. Zhao, H. Wang and H. D. Chen, J. Neural Eng., 2013, 10, 046020 CrossRef PubMed.
- S. Il Park, D. S. Brenner, G. Shin, C. D. Morgan, B. A. Copits, H. U. Chung, M. Y. Pullen, K. N. Noh, S. Davidson, S. J. Oh, J. Yoon, K. I. Jang, V. K. Samineni, M. Norman, J. G. Grajales-Reyes, S. K. Vogt, S. S. Sundaram, K. M. Wilson, J. S. Ha, R. X. Xu, T. S. Pan, T. I. Kim, Y. G. Huang, M. C. Montana, J. P. Golden, M. R. Bruchas, R. W. Gereau and J. A. Rogers, Nat. Biotechnol., 2015, 33, 1280–1286 CrossRef PubMed.
- R. Qazi, C. Y. Kim, S. H. Byun and J. W. Jeong, Fort. Neurosci., 2018, 12, 764 Search PubMed.
-
G. P. Agrawal, Fiber-Optic Communication System, Wiley Micro, 2010, vol. 222, pp. 1–603 Search PubMed.
-
R. Ramaswami, K. N. Sivarajan and G. H. Sasaki, Optical Networks: A Practical Perspective, 3rd edn, 2010 Search PubMed.
- S. Shabahang, S. Kim and S. H. Yun, Adv. Funct. Mater., 2018, 28, 1706635 CrossRef.
- J. I. Peterson and G. G. Vurek, Science, 1984, 224, 123–127 CrossRef CAS PubMed.
- A. G. Mignani and F. Baldini, Rep. Prog. Phys., 1996, 59, 1–28 CrossRef CAS.
- G. T. Webb, P. J. Vardanega, N. A. Hoult, P. R. A. Fidler, P. J. Bennett and C. R. Middleton, J. Bridge Eng., 2017, 22, 05017002 CrossRef.
- F. Xiao, J. L. Hulsey and R. Balasubramanian, Struct. Control Health Monit., 2017, 24, e2020 CrossRef.
- N. Mohamed, I. Jawhar, J. Al-Jaroodi and L. R. Zhang, Sensors, 2011, 11, 10738–10764 CrossRef PubMed.
- Z. Zhou, J. P. He, M. H. Huang, J. He, J. P. Ou and G. D. Chen, Proc. SPIE, 2010, 7649 Search PubMed.
- S. D. Hart, G. R. Maskaly, B. Temelkuran, P. H. Prideaux, J. D. Joannopoulos and Y. Fink, Science, 2002, 296, 510–513 CrossRef CAS PubMed.
- M. Bayindir, F. Sorin, A. F. Abouraddy, J. Viens, S. D. Hart, J. D. Joannopoulos and Y. Fink, Nature, 2004, 431, 826–829 CrossRef CAS PubMed.
- M. Bayindir, A. E. Abouraddy, J. Arnold, J. D. Joannopoulos and Y. Fink, Adv. Mater., 2006, 18, 845–849 CrossRef CAS.
- A. Gumennik, A. M. Stolyarov, B. R. Schell, C. Hou, G. Lestoquoy, F. Sorin, W. McDaniel, A. Rose, J. D. Joannopoulos and Y. Fink, Adv. Mater., 2012, 24, 6005–6009 CrossRef CAS PubMed.
- N. Chocat, G. Lestoquoy, Z. Wang, D. M. Rodgers, J. D. Joannopoulos and Y. Fink, Adv. Mater., 2012, 24, 5327–5332 CrossRef CAS PubMed.
- S. Egusa, Z. Wang, N. Chocat, Z. M. Ruff, A. M. Stolyarov, D. Shemuly, F. Sorin, P. T. Rakich, J. D. Joannopoulos and Y. Fink, Nat. Mater., 2010, 9, 643–648 CrossRef CAS PubMed.
- J. J. Wylie, H. X. Huang and R. M. Miura, J. Fluid Mech., 2007, 570, 1–16 CrossRef.
- F. Sorin, A. F. Abouraddy, N. Orf, O. Shapira, J. Viens, J. Arnold, J. D. Joannopoulos and Y. Fink, Adv. Mater., 2007, 19, 3872–3877 CrossRef CAS.
- L. Yang, Proc. SPIE, 2016, 9686 Search PubMed.
- A. F. Abouraddy, O. Shapira, M. Bayindir, J. Arnold, F. Sorin, D. S. Hinczewski, J. D. Joannopoulos and Y. Fink, Nat. Mater., 2006, 5, 532–536 CrossRef CAS PubMed.
- F. Sorin, O. Shapira, A. F. Abouraddy, M. Spencer, N. D. Orf, J. D. Joannopoulos and Y. Fink, Nano Lett., 2009, 9, 2630–2635 CrossRef CAS PubMed.
- Q. Guo, J. Zhou, Q. Feng, R. Lin, H. Gong, Q. Luo, S. Zeng, M. Luo and L. Fu, Biomed. Opt. Express, 2015, 6, 3919–3931 CrossRef CAS PubMed.
- S. L. Resendez and G. D. Stuber, Neuropsychopharmacology, 2015, 40, 238–239 CrossRef CAS PubMed.
- V. Q. Nguyen, J. S. Sanghera, F. H. Kung, P. C. Pureza and I. D. Aggarwal, J. Lightwave Technol., 2000, 18, 1395–1401 CAS.
- A. M. Stolyarov, A. Gumennik, W. McDaniel, O. Shapira, B. Schell, F. Sorin, K. Kuriki, G. Benoit, A. Rose, J. D. Joannopoulos and Y. Fink, Opt. Express, 2012, 20, 12407–12415 CrossRef CAS PubMed.
- T. Khudiyev, J. Clayton, E. Levy, N. Chocat, A. Gumennik, A. M. Stolyarov, J. Joannopoulos and Y. Fink, Nat. Commun., 2017, 8, 1435 CrossRef PubMed.
- G. Benoit, K. Kuriki, J. F. Viens, J. D. Joannopoulos and Y. Fink, Opt. Lett., 2005, 30, 1620–1622 CrossRef PubMed.
- Y. P. Qu, N. D. Tung, A. G. Page, W. Yan, T. Das Gupta, G. M. Rotaru, R. M. Rossi, V. D. Favrod, N. Bartolomei and F. Sorin, Adv. Mater., 2018, 30, 1700681 CrossRef PubMed.
- M. Knoblauch, J. M. Hibberd, J. C. Gray and A. J. E. van Bel, Nat. Biotechnol., 1999, 17, 906–909 CrossRef CAS PubMed.
- M. D. Dickey, Adv. Mater., 2017, 29, 1606425 CrossRef PubMed.
- S. Zhu, J. H. So, R. Mays, S. Desai, W. R. Barnes, B. Pourdeyhimi and M. D. Dickey, Adv. Funct. Mater., 2013, 23, 2308–2314 CrossRef CAS.
- C. B. Cooper, K. Arutselvan, Y. Liu, D. Armstrong, Y. L. Lin, M. R. Khan, J. Genzer and M. D. Dickey, Adv. Funct. Mater., 2017, 27, 1605630 CrossRef.
- A. Hirsch, H. O. Michaud, A. P. Gerratt, S. de Mulatier and S. P. Lacour, Adv. Mater., 2016, 28, 4507–4512 CrossRef CAS PubMed.
- R. Guo and J. Liu, J. Micromech. Microeng., 2017, 27, 104002 CrossRef.
- L. Rayleigh, London, Edinburgh Dublin Philos. Mag. J. Sci., 1892, 34, 145–154 CrossRef.
- J. J. Kaufman, G. Tao, S. Shabahang, E.-H. Banaei, D. S. Deng, X. Liang, S. G. Johnson, Y. Fink and A. F. Abouraddy, Nature, 2012, 487, 463 CrossRef CAS PubMed.
- M. Rein, E. Levy, A. Gumennik, A. F. Abouraddy, J. Joannopoulos and Y. Fink, Nat. Commun., 2016, 7, 12807 CrossRef CAS PubMed.
- A. F. Abouraddy, M. Bayindir, G. Benoit, S. D. Hart, K. Kuriki, N. Orf, O. Shapira, F. Sorin, B. Temelkuran and Y. Fink, Nat. Mater., 2007, 6, 336–347 CrossRef CAS PubMed.
- E. Segev, J. Reimer, L. C. Moreaux, T. M. Fowler, D. Chi, W. D. Sacher, M. Lo, K. Deisseroth, A. S. Tolias and A. Faraon, Neurophotonics, 2016, 4, 011002 CrossRef PubMed.
- O. Shapira, K. Kuriki, N. D. Orf, A. F. Abouraddy, G. Benoit, J. F. Viens, A. Rodriguez, M. Ibanescu, J. D. Joannopoulos and Y. Fink, Opt. Express, 2006, 14, 3929–3935 CrossRef PubMed.
- F. Sorin, G. Lestoquoy, S. Danto, J. D. Joannopoulos and Y. Fink, Opt. Express, 2010, 18, 24264–24275 CrossRef PubMed.
- A. Prasad, Q.-S. Xue, V. Sankar, T. Nishida, G. Shaw, W. J. Streit and J. C. Sanchez, J. Neural Eng., 2012, 9, 056015 CrossRef PubMed.
- V. Sankar, E. Patrick, R. Dieme, J. C. Sanchez, A. Prasad and T. Nishida, Front. Neuroeng., 2014, 7, 13 Search PubMed.
- Y. Guo, S. Jiang, B. J. Grena, I. F. Kimbrough, E. G. Thompson, Y. Fink, H. Sontheimer, T. Yoshinobu and X. Jia, ACS Nano, 2017, 11, 6574–6585 CrossRef CAS PubMed.
- M. Rein, V. D. Favrod, C. Hou, T. Khudiyev, A. Stolyarov, J. Cox, C. C. Chung, C. Chhav, M. Ellis, J. Joannopoulos and Y. Fink, Nature, 2018, 560, 214–218 CrossRef CAS PubMed.
- A. N. Zorzos, E. S. Boyden and C. G. Fonstad, Opt. Lett., 2010, 35, 4133–4135 CrossRef PubMed.
- T. V. F. Abaya, S. Blair, P. Tathireddy, L. Rieth and F. Solzbacher, Biomed. Opt. Express, 2012, 3, 3087–3104 CrossRef CAS PubMed.
- A. N. Zorzos, J. Scholvin, E. S. Boyden and C. G. Fonstad, Opt. Lett., 2012, 37, 4841–4843 CrossRef PubMed.
- C. J. Davey, A. Argyros, S. C. Fleming and S. G. Solomon, Appl. Opt., 2015, 54, 10068–10072 CrossRef CAS PubMed.
- Y. LeChasseru, S. Dufour, G. Lavertu, C. Bories, M. Deschenes, R. Vallee and Y. D. Koninck, Nat. Methods, 2011, 8, 319–325 CrossRef PubMed.
- S. Dufour, G. Lavertu, S. Dufour-Beausejour, A. Junaeu-Fecteau, N. Calakos, M. Deschenes, R. Vallee and Y. D. Koninck, PLoS One, 2013, 8, e57703 CrossRef CAS PubMed.
- F. Wu, E. Stark, M. Im, I. J. Cho, E. S. Yoon, G. Buzsaki, K. D. Wise and E. Yoon, J. Neural Eng., 2013, 10, 056012 CrossRef PubMed.
- F. Osakada, T. Mori, A. H. Cetin, J. H. Marshel, B. Virgen and E. M. Callaway, Neuron, 2011, 71, 617–631 CrossRef CAS PubMed.
- L. Madisen, T. Mao, H. Koch, J. M. Zhuo, A. Berenyi, S. Fujisawa, Y. W. Hsu, A. J. Garcia 3rd, X. Gu, S. Zanella, J. Kidney, H. Gu, Y. Mao, B. M. Hooks, E. S. Boyden, G. Buzsaki, J. M. Ramirez, A. R. Jones, K. Svoboda, X. Han, E. E. Turner and H. Zeng, Nat. Neurosci., 2012, 15, 793–802 CrossRef CAS PubMed.
- A. C. Felix-Ortiz, A. Beyeler, C. Seo, C. A. Leppla, C. P. Wildes and K. M. Tye, Neuron, 2013, 79, 658–664 CrossRef CAS PubMed.
- S. P. Lacour, G. Courtine and J. Guck, Nat. Rev. Mater., 2016, 1, 16063 CrossRef CAS.
- R. Chen, A. Canales and P. Anikeeva, Nat. Rev. Mater., 2017, 2, 16093 CrossRef CAS.
- V. R. Edgerton and S. Harkema, Expert Rev. Neurother., 2011, 11, 1351–1353 CrossRef PubMed.
- C. Lu, U. P. Froriep, R. A. Koppes, A. Canales, V. Caggiano, J. Selvidge, E. Bizzi and P. Anikeeva, Adv. Funct. Mater., 2014, 24, 6594–6600 CrossRef CAS.
- L. A. Gunaydin, L. Grosenick, J. C. Finkelstein, I. V. Kauvar, L. E. Fenno, A. Adhikari, S. Lammel, J. J. Mirzabekov, R. D. Airan, K. A. Zalocusky, K. M. Tye, P. Anikeeva, R. C. Malenka and K. Deisseroth, Cell, 2014, 157, 1535–1551 CrossRef CAS PubMed.
- L. Li, Y. J. Tang, L. Q. Sun, K. Rahman, K. Huang, W. Z. Xu, J. S. Yu, J. X. Dai and G. Cao, J. Innovative Opt. Health Sci., 2017, 10, 1743001 CrossRef CAS.
- S. L. Resendez and G. D. Stuber, Neuropsychopharmacology, 2015, 40, 238–239 CrossRef CAS PubMed.
- L. Tian, S. A. Hires, T. Mao, D. Huber, M. E. Chiappe, S. H. Chalasani, L. Petreanu, J. Akerboom, S. A. McKinney and E. R. Schreiter, Nat. Methods, 2009, 6, 875–881 CrossRef CAS PubMed.
- T.-W. Chen, T. J. Wardill, Y. Sun, S. R. Pulver, S. L. Renninger, A. Baohan, E. R. Schreiter, R. A. Kerr, M. B. Orger and V. Jayaraman, Nature, 2013, 499, 295–300 CrossRef CAS PubMed.
- C. E. Schmidt and J. B. Leach, Annu. Rev. Biomed. Eng., 2003, 5, 293–347 CrossRef CAS PubMed.
- J. Noble, C. A. Munro, V. S. Prasad and R. Midha, J. Trauma, 1998, 45, 116–122 CrossRef CAS PubMed.
- Z. L. Chen, W. M. Yu and S. Strickland, Annu. Rev. Neurosci., 2007, 30, 209–233 CrossRef CAS PubMed.
- V. Carriel, M. Alaminos, I. Garzon, A. Campos and M. Cornelissen, Expert Rev. Neurother., 2014, 14, 301–318 CrossRef CAS PubMed.
- I. I. Stoyanova, R. J. van Wezel and W. L. Rutten, J. Neural Eng., 2013, 10, 066018 CrossRef PubMed.
- K. Pawar, R. Mueller, M. Caioni, P. Prang, U. Bogdahn, W. Kunz and N. Weidner, Acta Biomater., 2011, 7, 2826–2834 CrossRef CAS PubMed.
- D. Hoffman-Kim, J. A. Mitchel and R. V. Bellamkonda, Annu. Rev. Biomed. Eng., 2010, 12, 203–231 CrossRef CAS PubMed.
- A. Mobasseri, A. Faroni, B. M. Minogue, S. Downes, G. Terenghi and A. J. Reid, Tissue Eng., Part A, 2015, 21, 1152–1162 CrossRef CAS PubMed.
- R. A. Koppes, S. Park, T. Hood, X. T. Jia, N. A. Poorheravi, A. H. Achyuta, Y. Fink and P. Anikeeva, Biomaterials, 2016, 81, 27–35 CrossRef CAS PubMed.
- B. Grena, J. B. Alayrac, E. Levy, A. M. Stolyarov, J. D. Joannopoulos and Y. Fink, Nat. Commun., 2017, 8, 364 CrossRef PubMed.
- M. Ikeda, T. Uemura, K. Takamatsu, M. Okada, K. Kazuki, Y. Tabata, Y. Ikada and H. Nakamura, J. Biomed. Mater. Res., Part A, 2014, 102, 1370–1378 CrossRef PubMed.
- S. Baiguera, C. Del Gaudio, E. Lucatelli, E. Kuevda, M. Boieri, B. Mazzanti, A. Bianco and P. Macchiarini, Biomaterials, 2014, 35, 1205–1214 CrossRef CAS PubMed.
- A. A. Al-Majed, C. M. Neumann, T. M. Brushart and T. Gordon, J. Neurosci., 2000, 20, 2602–2608 CrossRef CAS PubMed.
- A. A. Al-Majed, S. L. Tam and T. Gordon, Cell. Mol. Neurobiol., 2004, 24, 379–402 CrossRef CAS PubMed.
- D. M. Thompson, A. N. Koppes, J. G. Hardy and C. E. Schmidt, Annu. Rev. Biomed. Eng., 2014, 16, 397–430 CrossRef CAS PubMed.
- A. N. Koppes, A. M. Seggio and D. M. Thompson, J. Neural Eng., 2011, 8, 046023 CrossRef PubMed.
- S. Park, R. A. Koppes, U. P. Froriep, X. T. Jia, A. K. H. Achyuta, B. L. McLaughlin and P. Anikeeva, Sci. Rep., 2015, 5, 9669 CrossRef CAS PubMed.
- F. J. Rodriguez, D. Ceballos, M. Schuttler, A. Valero, E. Valderrama, T. Stieglitz and X. Navarro, J. Neurosci. Methods, 2000, 98, 105–118 CrossRef CAS PubMed.
- S. H. Lee, J. H. Jung, Y. M. Chae, J. K. F. Suh and J. Y. Kang, J. Micromech. Microeng., 2010, 20, 035015 CrossRef.
- T. Boretius, J. Badia, A. Pascual-Font, M. Schuettler, X. Navarro, K. Yoshida and T. Stieglitz, Biosens. Bioelectron., 2010, 26, 62–69 CrossRef CAS PubMed.
- C. Y. Dai, Y. J. Li, A. Christie, P. Bonato, K. C. McGill and E. A. Clancy, IEEE Trans. Neural Syst. Rehabil. Eng., 2015, 23, 32–40 Search PubMed.
- K. M. Musick, J. Rigosa, S. Narasimhan, S. Wurth, M. Capogrosso, D. J. Chew, J. W. Fawcett, S. Micera and S. P. Lacour, Sci. Rep., 2015, 5, 14363 CrossRef CAS PubMed.
- R. K. Gore, Y. S. Choi, R. Bellamkonda and A. English, J. Neural Eng., 2015, 12, 016017 CrossRef PubMed.
- K. Famm, Nature, 2013, 496, 300 CrossRef PubMed.
- K. Birmingham, V. Gradinaru, P. Anikeeva, W. M. Grill, V. Pikov, B. McLaughlin, P. Pasricha, D. Weber, K. Ludwig and K. Famm, Nat. Rev. Drug Discovery, 2014, 13, 399–400 CrossRef CAS PubMed.
|
This journal is © The Royal Society of Chemistry 2019 |
Click here to see how this site uses Cookies. View our privacy policy here.