Unexpected trends in the hydrophobicity of fluorinated amino acids reflect competing changes in polarity and conformation†
Received
13th November 2018
, Accepted 3rd January 2019
First published on 4th January 2019
Abstract
Fluorination can dramatically improve the thermal and proteolytic stability of proteins and their enzymatic activity. Key to the impact of fluorination on protein properties is the hydrophobicity of fluorinated amino acids. We use molecular dynamics simulations, together with a new fixed-charge, atomistic force field, to quantify the changes in hydration free energy, ΔGHyd, for amino acids with alkyl side chains and with 1 to 6 –CH → –CF side chain substitutions. Fluorination changes ΔGHyd by −1.5 to +2 kcal mol−1, but the number of fluorines is a poor predictor of hydrophobicity. Changes in ΔGHyd reflect two main contributions: (i) fluorination alters side chain–water interactions; we identify a crossover point from hydrophilic to hydrophobic fluoromethyl groups which may be used to estimate the hydrophobicity of fluorinated alkyl side-chains; (ii) fluorination alters the number of backbone–water hydrogen bonds via changes in the relative side chain-backbone conformation. Our results offer a road map to mechanistically understand how fluorination alters hydrophobicity of (bio)polymers.
1 Introduction
The preferential interaction between apolar solutes in water – the hydrophobic effect – is a key factor driving protein folding,1,2 structural stability with respect to changes in temperature3,4 (thermal stability) and interactions with other proteins and ligands.5,6 The hydrophobic effect reflects the balance between solute–water interactions and direct, predominantly dispersive, solute–solute interactions.7,8 Understanding how to use amino acid mutations to control the hydrophobic effect is critical to develop new protein-based drugs, biodevices and materials.9–13 Simultaneously, minimizing changes in solute–solute packing upon mutations is desirable to ensure that protein structure – and thus function – is preserved.14,15 This is, however, difficult with the limited pool of canonical hydrophobic amino acids because their side chains differ in structure and volume. Fluorinated versions of those amino acids, i.e. those where hydrogen atoms in side chain groups are substituted by fluorine (see Fig. 1), can solve this problem while simultaneously enhancing other properties of interest.3,16–19 Even fluorinating only a few residues may enhance the hydrophobicity and passive diffusion of peptides through membranes,20 the proteolytic resistance21 and anti-microbial activity of proteins,22 in addition to tuning their thermal stability,23 making this synthetic approach of wide interest.24–28
 |
| Fig. 1 Molecular structures, commonly used names and abbreviations for the amino acids under study. Each amino acid residue is capped at the N-terminus with an acetate group (ACE, –COCH3) and at the C-terminus with an N-methyl group (NME, –NHCH3). Abbreviations for fluorinated amino acids follow a three-character nomenclature: initial character of parent (non-fluorinated) amino acid name (E, P, V, I, L); number of fluorine atoms (1, 2, 3, 4, 6); fluorination site (δ carbon as D, γ carbon as G or, in the case of chiral center formation following fluorination, R or S). | |
Still, a caveat of using fluorination to control protein properties remains: do we understand the factors influencing the hydrophobic effect involving fluorinated amino acids? The answer is, simply, that we do not. Here we focus on one factor contributing to the hydrophobic effect: solute–water interactions, for simplicity referred to as solute hydrophobicity. The hydrophobicity of solutes is usually characterized by their hydration free energies.8 The hydrophobicity of fluorinated amino acids has been qualitatively estimated by considering the surface area of its side chain (the larger the surface area, the larger the hydrophobicity)29,30 and its side chain polarity (the larger the polarity, the smaller the hydrophobicity),23 but detailed mechanistic understanding is still lacking.16,19 Understanding the origins of fluorination-induced changes in hydrophobicity depends critically on our ability to accurately quantify interactions between amino acids and their environment. We demonstrate that this quantification is now possible using molecular dynamics simulations and fixed-charge, all-atom models. The approach presented here is general, and may be used to investigate the hydrophobicity of any fluorinated (bio)polymer or small molecule.
2 Computational methods
We used the TIP4P-Ew (ref. 31) water model, the AMBER14 (ff14sb; ref. 32) force field for the canonical amino acids and the GAFF force field for methane, ethane and propane. Note that AMBER14 and GAFF use the same Lennard-Jones parameters. For the remaining amino acids and for the fluorinated small molecules, we used a force field developed by us (previous own work33 and ESI† Sections 1 and 2) based on AMBER14 (amino acids) or GAFF (small molecules). The main difference between our force field for fluorinated molecules and GAFF/AMBER14 lies in the ε and σ Lennard-Jones (LJ) parameters of fluorine, and of hydrogen (HF) bonded to a fluorinated carbon. The LJ parameters of fluorine were optimized to reproduce the hydration free energies of CF4 and the molar volume of a 50% mix of CF4 and CH4; subsequently, those of HF were optimized to reproduce the hydration free energy and the molar volume of CHF3. We chose these target properties because the hydrophobic effect and packing in the hydrophobic core are key for protein stability. In total, we tested 46 (σFF,εFF) and 24 (σHFHF,εHFHF) pairs. LJ interactions between different atoms are calculated using the standard combination rules used in the AMBER force field. Atomic partial charges were obtained following the GAFF (small molecules) or AMBER (amino acids) protocols. We note that the requirement of compatibility with the protein force field implies that we retain the point charge representation. This representation has known shortcomings when modeling carbon-bound chlorine, bromine or iodine because these halogens exhibit σ-holes (a positive area in the electrodensity distribution of the halogen atom, surrounded by a negative belt) which this charge model cannot represent.34 Carbon-bound fluorine, however, retains a negative potential in its entire surface and the level of anisotropy in its electronic charge distribution is small.35–37 For that reason, single point charge models have been used to model the interactions of fluorinated sites with water and with other organic molecules: e.g., Schyman & Jorgensen,34 Ibrahim38 and Ho39 apply their models of σ-holes to carbon-bound chlorine, bromine and iodine, but retain a point charge representation for fluorinated sites. Point charge models perform less well in the case of fluorinated aryl groups because the σ-hole perturbation extends into the β-carbons; when modeling fluorinated aryl molecules, other promising models such as those based on permanent atomic multipole charges37,40,41 might be advantageous. This extended perturbation occurs via the π electrons,37 and consequently is not expected to be nearly as significant for fluorinated alkyl groups, which are the sole focus of the present work.
Free energy calculations were performed with Gromacs 5.042–48 and molecular dynamics simulations to calculate other observables were performed with AMBER 14.49 All systems were assembled using the built-in tools of the software package used to perform the simulations. A summary of the most relevant parameters used during the production runs is given in ESI† Table 2. Simulations used a time-step of 2 fs and constraints (LINCS50 in Gromacs, SHAKE51 in Amber) were applied to all bonds involving hydrogen atoms. Integration of the equations of motion was done using a leap-frog Langevin algorithm. van der Waals interactions were shifted to zero between 1.0 and 1.2 nm, and long-range dispersion corrections were applied to both pressure and energy. Long-range electrostatics were treated with the PME scheme with a 1.2 nm cutoff, a grid spacing of 0.1 nm (AMBER) or 0.12 nm (Gromacs) and a 4th (AMBER) or 6th (Gromacs) order interpolation. Production runs were done in the NpT ensemble. The Monte Carlo49,52 (AMBER) or Berendsen53 (Gromacs) barostats were used with a relaxation time of 1 ps for an isotropic coupling of system pressure to 1 bar; temperature coupling was handled by the leap-frog Langevin integrator with a collision frequency of 1 ps−1 and a target temperature of 298 K.
Hydration free energies were calculated using Free Energy Perturbation (FEP) and Bennett Acceptance Ratio54,55 (BAR), following the protocol we have previously adopted33 and described in ESI† Section 2.1. Briefly, in each case, simulations consisting of a single solute molecule in water were conducted, first decoupling coulombic interactions and then LJ interactions. The coupling parameter λC for the coulombic interactions was scaled linearly and assumed 21 equally-spaced values between 0 and 1; decoupling the LJ interactions was done over 59 states, with unevenly-spaced λLJ values between 0 and 1. At each state, we performed a steepest-descent minimization, BFGS minimization, 100 ps of NVT equilibration and 100 ps of NpT equilibration before collecting statistics for 2 ns. Five independent production runs were performed for each amino acid; three production runs were performed for each (fluoro)alkane.
Molecular dynamics simulations consisted of a steepest descent minimization, a conjugated gradient minimization, a 200 ps NVT heating from 0 K to 298 K, a 1 ns NpT equilibration and a 25 ns NpT production run; in the minimization, heating and equilibration steps the coordinates of the backbone atoms were restrained with a 20 kcal mol−1 potential. These trajectories were used to extract the data used as input for eqn (1), and were also used as input for the APBS56 software to estimate the electrostatic hydration free energy of the amino acids using the linearized Poisson–Boltzmann equation, as described in more detail in ESI† Section 3.1.
3 Results & discussion
Change in ΔGHyd with fluorination depends on the chirality and location of the fluorinated site and on amino acid identity
We calculated hydration free energies as a measure of the hydrophobicity of 16 fluorinated amino acids and their 5 non-fluorinated counterparts, totaling 21 aliphatic amino acids (see Fig. 1). These free energies are shown in ESI† Table 4 and Fig. 2; we reported some of these values in a prior publication.33 The ΔΔGHyd values have an associated standard deviation of 0.1 to 0.3 kcal mol−1 (see ESI† Table 4), enabling the precise detection of differences between amino acids.
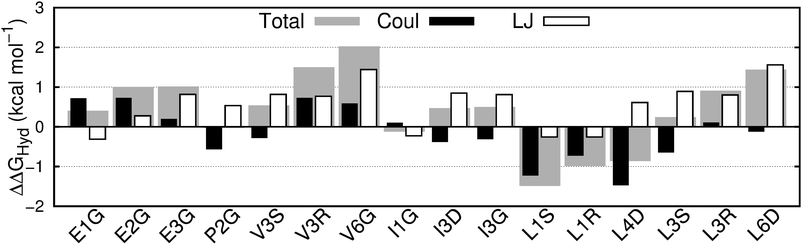 |
| Fig. 2 Coulombic contribution (Coul), Lennard-Jones contribution (LJ) and sum of the contributions (total) to the differences in hydration free energy (ΔΔGHyd) between fluorinated and non-fluorinated amino acids. Each bar is the average of five independent simulations. | |
The amino acids with one or more –CH3 → –CF3 substitutions (here termed fully fluorinated) are, as expected, always more hydrophobic (positive ΔΔGHyd) than their non-fluorinated counterparts,3,19,57 but the change in free energy is not constant per fluorinated group. Even more surprisingly, amino acids with –CH3 → –CH2F/–CHF2 and –CH2– → –CF2– substitutions (here termed partially fluorinated) display a range of ΔΔGHyd values from −1.5 kcal mol−1 to +1 kcal mol−1, regardless of the number of fluorine atoms. ΔΔGHyd depends strongly and non-intuitively on the chirality (R/S) and location (γ vs. δ) of the fluorinated sites, and on the identity of the amino acid.
Calculated ΔGHyd for fluorinated C1–C3 alkanes compares well with experiment
Experimental hydration free energies for amino acids are not available, so we cannot directly assess the accuracy of our predictions. To test the accuracy of our force field, we applied it to all fluorinated variants of methane, ethane and propane for which experimental hydration free energies could be found.58 These small molecules are the closest analogues to the side chains of the amino acids investigated here. The free energies of hydration for the fluorinated small molecules are shown in Fig. 3 and ESI† Table 4. We show also the free energies of hydration of methane, ethane and propane, to illustrate the accuracy of the AMBER force field for the alkyl side chains of amino acids. The force field for fluorinated molecules reproduces the experimental hydration free energy very well in most cases. The Average Unsigned Error (AUE) is 0.25 kcal mol−1, lower than reported previously for fluorinated molecules: AUE = 0.36 kcal mol−1 for 4 fluorinated (aromatic) molecules using a permanent multipole charge model for the molecules and the TIP3P water model;41 AUE = 0.5 to 0.9 kcal mol−1 for 6 fluorinated (primarily aliphatic) molecules using various version of the GAFF, CHARMM and OPLS force fields and SPC or TIP3P water.59–61 The largest deviation using our model is seen for CH3F, whose ΔGHyd is 0.7 kcal mol−1 too negative. Differences between di- and trifluorinated amino acids should be well captured, but monofluorinated alkyl groups are likely excessively hydrophilic in our model.
 |
| Fig. 3 Free energy of hydration (ΔGHyd) of the indicated fluorinated molecules (green), and of methane, ethane and propane (yellow) obtained with simulation (FEP) or experimentally (Exp.; from ref. 58). CF4 and CHF3 were used during parameterization. The statistical uncertainty in the FEP values is the size of the symbol. See ESI† Table 4 for free energy values. | |
The predicted hydration free energies for methane, ethane and propane are too positive by 0.5 to 0.8 kcal mol−1. These results suggest that the predicted ΔΔGHyd for amino acids (Fig. 2) may be systematically too negative by 0.5 to 0.8 kcal mol−1. The AMBER force field for proteins, upon which we build the force field for fluorinated amino acids, has been extensively improved for decades and is able to reproduce experimentally-measured structure and dynamics of folded small proteins.62–68 Efforts to continue developing this force field are on-going in multiple groups68 and are now preferentially driven by experimental data on peptides and both folded and intrinsically disordered proteins, rather than on small molecules. Given the state-of-the-art, we opted to keep the Lennard-Jones parameters for the alkane side chains.
Despite these quantitative deviations between our calculated ΔGHyd and experiment, our model yields the correct trend in hydrophobicity for all small molecules, fluorinated or not. Our main result – the large dependence of ΔΔGHyd,FEP on the identity of the amino acid and characteristics of the fluorinated site – is not affected by these force field shortcomings. Below we show that this dependence is in fact largely due to fluorination-induced conformational changes that alter the number of backbone–water hydrogen bonds. Fluorination-induced changes in conformation in our simulations result from steric hindrance and favorable electrostatic carbonyl–CF interactions, both of which can be captured, at least qualitatively, by all-atom, fixed charge force fields.
The surprising variation in ΔΔGHyd is largely coulombic in origin
Decomposing the hydration free energy into Lennard-Jones and coulombic contributions can be naturally done in simulations, and gives valuable insight into the origin of the observed trends. The Lennard-Jones contribution to ΔΔGHyd (Fig. 2) is constant and positive per fully fluorinated group, positive but not constant for difluorinated amino acids and negative and constant for monofluorinated amino acids. Despite this variation in the LJ contribution to the free energy of hydration, the wide variation in ΔΔGHyd, particularly in the case of the partially fluorinated amino acids, is actually dominated by the coulombic contribution to the free energy. This contribution varies seemingly unpredictably (Fig. 2), with each type of fluorination leading to either positive or negative ΔΔGCoulHyd: e.g., compare E2G with P2G, E1G with L1S, L1R and I1G, V3S with V3R. Previous reports on the dependence of lipophilicity on fluorine-induced polarity changes support the idea that the contribution of electrostatics to hydrophobicity is far from intuitive.69,70 Neither contribution shows visible correlation with local hydration around the fluorinated site, as measured by the radial distribution function of water around the fluorinated sites (ESI† Section 5.2.4, Fig. 8A and B).
Developing an analytical solvation model to understand how fluorination affects ΔGHyd
Can we understand the origin of these hydration free energies? To answer this question we model the fluorination-induced change in hydration free energy in terms of linear, multivariate models of the form
, where ki are fitting parameters and Yi are observables that should affect the hydration free energy and can be easily calculated in short molecular dynamics simulations. The LJ contribution to ΔΔGHyd is dominated by the energy (work) required to form a solute-sized cavity in water to accommodate the larger fluorine atoms. This contribution (Table 1) is proportional to the change in solvent accessible surface area (ΔSASA) of the amino acid,71,72 and can be easily quantified by measuring ΔSASA in molecular dynamics simulations, and multiplying it by the LJ component of ΔGHyd per surface area unit of methane, which is essentially identical to that of CF4 (ESI† Table 9).
Table 1 Values of the fitting parameters from eqn (1), and associated standard errors and P-values
Fitting parameter |
Value |
Error |
P-value |
NA: not applicable. Calculated via error propagation. |
k
1 (ΔA; kcal mol−1 Å−2) |
0.053 |
0.001a |
NA |
k
2 (ΔhCO; kcal mol−1 H-bond−1) |
−3.590 |
0.350 |
0.000 |
k
3 (ΔhNH; kcal mol−1 H-bond−1) |
−3.020 |
0.780 |
0.012 |
k
4 (ΔΦ+; kcal mol−1 H2O−1) |
0.115 |
0.044 |
0.022 |
k
5 (hCH2F; kcal mol−1 H-bond−1) |
−2.619 |
0.396 |
0.000 |
k
6 (hCF2; kcal mol−1 H-bond−1) |
−1.808 |
0.270 |
0.000 |
k
7 (hCF3; kcal mol−1 H-bond−1) |
−0.780 |
0.222 |
0.004 |
The polar contribution is dominated by the interaction of a distribution of atom-centered point charges with water. This contribution can be estimated in multiple manners.33,69,70,73 We first attempted to model the polar contribution as the sum of three terms, one proportional to a global quantity, the dipole moment μ, representing the molecular charge distribution, and the other two proportional to local quantities, the number of hydrogen bonds between water and amines (hNH) or carbonyls (hCO) in the backbone.‡ Fitting the ΔΔGHyd values calculated with FEP using a linear multivariate model (ESI† eqn (5)) consisting of the sum of the contributions arising from changes in SASA, μ, hNH, and hCO due to fluorination was unsuccessful: the resulting fitting parameters had unphysical values, e.g., a positive energetic contribution of the dipole moment, and overly large errors (ESI† Table 6). We also attempted to model our data using an analogous version of this model, but where the area term reflects the difference in hydration free energy between CH4 and CF4. This second model (ESI† eqn (6)) has proven successful to understand hydrophobicity of tri- and hexa-fluorinated amino acids,33 but it fails (ESI† Fig. 4) when applied to the partially fluorinated amino acids. Given that the contributions of solute–water hydrogen bonds and the Lennard-Jones interactions to the hydration free energies are well-known,33,71,73 we interpret these results as an inability of the molecular dipole moment to describe electrostatic solute–water interactions in a quantitative manner, at least for solutes as diverse as the current set of amino acids. We next attempted to characterize how fluorination alters the solute–water electrostatic interactions with another commonly used global descriptor: solving the linearized Poisson–Boltzmann (PB) equation, where the solvent is modeled as a continuum, as described in ESI† Section 3.1. Given the apparently simple problem we were trying to model, we were surprised to find that the electrostatic component of the hydration free energy calculated using PB hardly correlates with the reference FEP values (ESI† Fig. 2A). The absence of correlation suggests that it is imperative to model the solvent as discrete water molecules. Thus, when aiming for a correct characterization of how aqueous solvation of biopolymers changes with mutations, not only the solute but also the solvent must be modeled without the use of global or mean-field descriptors.
Backbone–water hydrogen bonds dominate the polar interactions between the backbone and water;73 the unresolved issue is how to describe polar interactions between the side chain and water. We find that these can be characterized by the electrostatic potential, Φ, at the position of the water oxygen atoms in the first hydration shell of the side chains, as described in ESI† Section 4. The corresponding probability distributions of Φ for the fluorinated amino acids, shown in ESI† Fig. 2B–F, show large negative potential regions together with, in some cases, regions of more positive potentials than observed for the parent amino acid. The more positive potential, almost exclusive to mono- and difluorinated species, arises from a larger exposure of the positively charged carbon skeleton of the side chain, left partially unshielded by hydrogen in mono- and difluorinated groups (see ESI† Section 4 and Fig. 3). The negative potential region, observed for all amino acids, can be attributed to the fluorine atoms. Water molecules near fluorine atoms often assume configurations that meet the geometric criteria for HOH⋯FC hydrogen bonds, as discussed in ESI† Section 5.2, so we consider that these weak hydrogen bonds exist. This interpretation is consistent with ab initio calculations, and spectroscopic measurements;74–77 the strong correlation between 19F NMR isotropic chemical shifts and the type of fluorine–protein interactions observed in the Protein Data Bank also suggest that hydrogen bonds to fluorinated alkyl groups exist, and are strongest for groups with low degrees of fluorination,78 as we also observe (ESI† Table 7).
Our final model (eqn (1)) reflects the above results. We capture the impact of the fluorination-induced differences on side chain–water electrostatic interactions in two ways, via the average number of water–fluorine hydrogen bonds established by each amino acid (the hCHnFm terms, where n = 0, 1, 2 and m = 1, 2, 3, in eqn (1)) and via the fluorination-induced change in the number of water molecules experiencing the positive potential region of the side chain (the ΔΦ+ term in eqn (1)). Fitting eqn (1) to the ΔΔGHyd data shown in Fig. 2 yields the parameters in Table 1; see ESI† Section 5.2 for details of the fit.
| ΔΔGHyd = k1ΔA + k2ΔhCO + k3ΔhNH + k4ΔΦ+ + k5hCH2F + k6hCF2 + k7hCF3 | (1) |
Fig. 4 shows the correlation between the FEP-calculated hydration free energies and the ones calculated using
eqn (1). The model describes the changes in Δ
GHyd following fluorination well for most cases, with an adjusted
R-squared of 0.86 and an AUE = 0.26 kcal mol
−1. Poorer agreement occurs for E1G, for which it yields a value of ΔΔ
GHyd which deviates 0.5 kcal mol
−1 from the value given by the FEP simulations and actually has the wrong sign, and for E2G, L4D and L3S, for which deviations are 0.7, 0.5 and 0.5 kcal mol
−1 each. Our prior work suggests that the source of these large deviations might be entropic,
33 and we speculate it might be related to changes in solute conformational entropy, which are not included in
eqn (1). Clarifying this point is outside the scope of the present work. The systematic error expected in ΔΔ
GHyd,FEP because of the inaccuracy associated with the AMBER force field for alkane side chains (see
Fig. 3 and associated discussion above) does not jeopardize the good correlation seen in
Fig. 4. That systematic error is also present in ΔΔ
GHyd,model, in the
k1 term associated with the change in area. Future applications of
eqn (1) to force field versions that better reproduce the hydration free energies of alkanes can easily be done by re-optimizing the
k1 term.
 |
| Fig. 4 Correlation between the hydration free energy differences between fluorinated and non-fluorinated amino acids calculated with FEP (ΔΔGHyd,FEP) or eqn (1) (ΔΔGHyd,model). Data points are presented as mean ± standard deviation of five independent simulations. The color code indicates the number of fluorine atoms: red = one; yellow = two; green = three; cyan = four; blue = six. The gray line indicates perfect correlation. | |
Decomposing the contributions to ΔΔGHyd
The performance of the multivariate model is sufficiently good to enable insight into the mechanisms of fluorination-induced changes in solvation, by decomposing the individual contributions to ΔΔGHyd as seen in Fig. 5.
 |
| Fig. 5 Contribution of the changes in surface area (ΔA), hydrogen bonds between water and carbonyls (ΔhCO) or amines (ΔhNH), water molecules exposed to a large positive electrostatic potential (ΔΦ+) and hydrogen bonds between water and fluorine in mono- (hCH2F), di- (hCF2) or trifluorinated (hCF3) amino acids to the total change in hydration free energy (ΔΔGHyd), between fluorinated and non-fluorinated amino acids, calculated using eqn (1). | |
Surface area.
Within derivatives of the same amino acid, the surface area increases ΔΔGHyd proportionally to the number of fluorine atoms (ESI† Fig. 5). The magnitude of the increase per fluorine atom depends on the amino acid identity, which implies that the hydration shells around each side chain are disturbed to a different degree, when accommodating the hydrogen → fluorine substitution – compare, e.g. E2G with P2G.
Backbone–water hydrogen bonds.
The contribution of hydrogen bonds between water and carbonyl groups is always positive because fluorination reduces the number of these hydrogen bonds by steric blockage. The magnitude of this contribution, for fluorinated variants of a given amino acid, is again proportional to the number of fluorine atoms in the side chain. In contrast, the contribution of amine–water hydrogen bonds varies between positive and negative because fluorination may increase or decrease the number of these hydrogen bonds. Steric blockage occurs because of fluorine's large size and, for some amino acids, because fluorination changes the preferential conformation of the side chain as discussed in ESI† Section 6. These results are consistent with previous reports indicating that CF and carbonyl groups interact favorably,79,80 and that changes in the conformational preference of fluorinated alkyl groups affect a molecule's lipophilicity, membrane permeability and inhibitory activity.25,81,82
Side-chain polarity.
The most interesting contributions arise from the polarity of the fluorinated side chain. The large, positive electrostatic potential affecting hydration waters adds an average +0.4 kcal mol−1 to the ΔΔGHyd of all partially fluorinated amino acids, and a near-zero, positive, contribution to the trifluorinated amino acids; the largest deviations come from I1G and I3D, for which this contribution is +0.8 kcal mol−1. As indicated above, this contribution stems from the partial shielding of the positively charged carbons occurring in partially fluorinated groups. Regarding the water–fluorine hydrogen bonds, they are much weaker than those with the carbonyl or amine groups, and decrease in stability in the order –CH2F > –CHF2/–CF2– > –CF3, as indicated by the relative magnitudes of the relevant parameters in Table 1. These trends are expected: our simulations show that the distance between the water oxygen and the fluorine is smaller in groups with fewer fluorines (ESI† Table 7) indicating an increase in hydrogen bond strength, and other experiments and ab initio calculations have shown the same trends.78,83 Despite the weakness of the hydrogen bonds between water and the di- and tri-fluorinated groups, they nevertheless play an important role: e.g., they are present ≈15% of the time per fluorine in CF3 groups (ESI† Table 8); for comparison, the number of water–methyl configurations per side chain CH group meeting the hydrogen bond criteria in the non-fluorinated amino acids is almost negligible (≈3%). The magnitude of the hydrogen bond contributions to ΔΔGHyd per fluorine atom also follows the order –CH2F > –CHF2/–CF2– > –CF3, with the average values being −1.79, −0.60 and −0.13 kcal mol−1 per fluorine, respectively;§ the weak water–fluorine hydrogen bonds to –CF3 contribute on average a non-negligible −0.39 kcal mol−1 –CF3−1.
Contributions of the side chain to ΔΔGHyd are largely constant per CFx substituent
The values of the free energy contribution per water–fluorine hydrogen bond yielded by the multivariate model anticorrelate surprisingly well with the LJ contribution of the area per fluorine atom (ESI† Fig. 6), suggesting that the energy cost associated with (overall repulsive) water–fluoromethyl LJ interactions is partially offset by the energy gain from the formation of water–fluorine hydrogen bonds. This point is illustrated in Fig. 6, where the ratio of the area and water–fluorine hydrogen bond contributions is plotted against the number of fluorine atoms. It is clear that the contribution of each fluorinated group to the hydration free energy is fairly constant. P2G, bearing the only non-terminal fluorination site in this set of amino acids, has a much lower cavity-forming penalty, thereby escaping this trend. The notable dependency of ΔΔGHyd on the chirality and location of the fluorinated site and the identity of the amino acids cannot be explained solely in terms of local changes in solvation around the fluorinated site.
 |
| Fig. 6 Ratio of the contribution of the water–fluorine hydrogen bonds to the contribution of the surface area to the ΔΔGHyd (k5–7hCHnFm(k1ΔA)−1) versus the number of fluorine atoms per fluoromethyl group. Contributions are calculated using eqn (1). The color code indicates the number of fluorine atoms: red = one; yellow = two; green = three; cyan = four; blue = six. The blue line is a linear fit to the data points (excluding P2G, shown as a circle) with the corresponding equation and regression coefficient at the top left. | |
For one hydrogen → fluorine substitution, the hydrogen bonding over-compensates the cavity-formation cost. As the number of substitutions increases, the penalty for cavity formation per fluorine is reduced, showing that the perturbation due to the insertion of the first fluorine atom is large but further insertions perturb the water network to a smaller extent. Simultaneously, the energy gain per fluorine from water–fluorine hydrogen bonds is decreased for multiple fluorine insertions, likely due to the less negative partial charges on fluorine and the fewer HF atoms, which interact favorably with water (see ESI† Section 2.3). Interestingly, there is a crossover point, associated with the number of fluorine substitutions, after which the energy cost surpasses the energy gain; in other words, there is a transition between a locally hydrophilic moiety to a locally hydrophobic moiety. Inspecting Fig. 6 we find that the number of fluorine atoms required to perform the transition is 2.8. This crossover point has associated uncertainty arising from the systematic deviations in the hydration free energies observed for small alkanes with the GAFF/Amber force field (see Fig. 3 and discussion above). Even considering this uncertainty, it appears that trifluoromethyl groups, by themselves, impart only a small increase in amino acid hydrophobicity, because the positive Lennard-Jones component of the hydration free energy is partially offset by the weak but still favorable water–fluorine hydrogen bonds.
RDFs of water around the fluorinated site do not give insight into local contributions to the ΔGHyd
Given that the impact of fluorination on local solvation is fairly constant for groups with the same number of fluorine atoms (Fig. 6), we investigated whether these local changes in solvation correlate well with the radial distribution function (RDF) of water around methyl or fluoromethyl groups. Specifically, we calculated the excess free energy, ΔΔGShell,PMF, necessary to populate the first hydration layer of methyl or fluoromethyl groups from the potential of mean force associated with each radial distribution function. Our results (ESI† Section 5.2.4 and Fig. 8C) show that such a correlation is at best very weak. We could also not find correlations between ΔΔGShell,PMF and the Coulomb and Lennard-Jones components of the hydration free energy (ESI† Fig. 8A and B). Radial distribution functions describe solvation along a single reaction coordinate, which may be insufficient to give quantitative or semi-quantitative insight into local hydrophobicity. In contrast, proximal radial distribution functions – those calculated perpendicular to the surface of the molecule – appear near universal for proteins84 and have proven useful to estimate hydration free energies of small molecules and peptides.85,86 Exploring their usefulness for fluorinated molecules is outside the scope of the present work.
4 Concluding remarks
We present an all-atom, fixed-charge force field for amino acids with fluorinated alkyl side chains that is compatible with the AMBER force field for proteins. With it we investigate how mono-, di- and trifluorination alter amino acid solvation. Our predictions indicate that side chain fluorination alters the hydration free energy of amino acids in surprising ways: ΔΔGHyd strongly depends on the chirality and location of the fluorinated site and on the identity of the amino acid. Using a simple, analytical solvation model (eqn (1)), we trace back these dependencies to the multiple mechanisms by which fluorination modifies solvation of amino acids: there is a cost of introducing larger fluorine atoms, gains and costs associated with the higher polarity of fluorinated alkyl groups, and gains or costs from altering the number of backbone–water hydrogen bonds as a result of changed conformational preferences. For small molecules, it is often possible to predict the sign and even estimate the magnitude of the change in hydrophobicity upon fluorination. In contrast, for complex molecules ‘the devil is in the details’: the contribution of each mechanism to the overall hydrophobicity depends on conformational preferences and interactions between different parts of the molecule, making rules-of-thumb insufficient. For example, monofluorination does not always make amino acids more hydrophilic; similar increases in the solvent-exposed surface area of different molecules do not imply that the molecules will experience similar increases in hydrophobicity. Solvent accessible surface area descriptors of hydration free energies remain useful for complex molecules, but only when other contributions are properly accounted for.
The parameter values associated with the solvation model we present will be quantitatively affected by inaccuracies in the force field, but the necessity of this type of approach, the functional form of the solvation model, and the importance of conformational changes and of backbone–water interactions in the non-intuitive variation of ΔGHyd with fluorination will remain.
Molecular dynamics studies with custom-tailored force fields and phenomenological solvation models based on discrete rather than mean-field descriptors are key to gain mechanistic insight on solvation, as exemplified in this work. The force field and solvation model we present lay the foundation to interpret how fluorination alters the hydrophobicity of other (bio)polymers. In its current form, the force field can be used to gain semi-quantitative insight on how fluorination affects packing in the hydrophobic core, or the contribution of water-protein interactions to protein stability. Further developing the force field for fluorinated proteins will require the experimental determination of the structure of both folded and intrinsically disordered proteins, the solubility of small peptides, and the free energies of hydration of amino acids or molecules of similar complexity. If the vapor pressure of the pure compound in liquid form is known together with its aqueous solubility, the free energy of hydration can be immediately calculated assuming ideality.58 We deliberately restricted the present study to amino acids for which synthesis protocols exist,19 and we hope that a direct comparison between experiment and simulation will be possible in the future.
The solvation model given by eqn (1) and applied here to amino acids can also be used to interpret molecular dynamics results of other small molecules containing the same functional groups, and extended for other functional groups. The solvation model is also directly relevant for proteins: together with short molecular dynamics simulations of proteins in the folded and unfolded ensembles, it can be used to gain quick insight into how fluorination-induced changes in protein–water interactions contribute to changes in the free energy of folding. Good sampling of the folded protein ensemble can easily be achieved in many cases with molecular dynamics simulations; to sample the unfolded protein ensemble, one can take advantage of a number of algorithms.87–91 Future work by our group will attempt to extend the solvation model to include mechanisms by which fluorination alters intra-protein non-bonded interactions. This extension is necessary to obtain a complete picture of the mechanisms by which fluorination alters the thermal stability of proteins.
Conflicts of interest
There are no conflicts to declare.
Acknowledgements
JRR was funded by the International Max Planck Research School on Multiscale Bio-Systems. We thank Marco Ehlert and René Genz from the IT team of the MPIKG for their help. We thank Dr Valerio Molinari and Dr Alexandra Latnikova for their critical reading of the manuscript. Open Access funding provided by the Max Planck Society.
References
- R. Zhou, X. Huang, C. J. Margulis and B. J. Berne, Science, 2004, 305, 1605–1609 CrossRef CAS PubMed.
- R. C. Harris and B. M. Pettitt, J. Phys.: Condens. Matter, 2016, 28, 083003 CrossRef PubMed.
- E. N. G. Marsh, Acc. Chem. Res., 2014, 47, 2878–2886 CrossRef CAS PubMed.
- C. N. Pace, H. Fu, K. L. Fryar, J. Landua, S. R. Trevino, B. A. Shirley, M. M. Hendricks, S. Iimura, K. Gajiwala, J. M. Scholtz and G. R. Grimsley, J. Mol. Biol., 2011, 408, 514–528 CrossRef CAS PubMed.
- S. Ye, B. Loll, A. A. Berger, U. Mülow, C. Alings, M. C. Wahl and B. Koksch, Chem. Sci., 2015, 6, 5246–5254 RSC.
- A. M. Davis and S. J. Teague, Angew. Chem., Int. Ed., 1999, 38, 736–749 CrossRef CAS.
- D. Ben-Amotz, J. Phys. Chem. Lett., 2015, 6, 1696–1701 CrossRef CAS.
- D. Ben-Amotz, Annu. Rev. Phys. Chem., 2016, 67, 617–638 CrossRef CAS.
- K. A. Brogden, Nat. Rev. Microbiol., 2005, 3, 238–250 CrossRef CAS PubMed.
- M. Zasloff, Nature, 2002, 415, 389–395 CrossRef CAS.
- S. T. Henriques, M. N. Melo and M. A. R. B. Castanho, Biochem. J., 2006, 399, 1–7 CrossRef CAS PubMed.
- Y.-W. Cui, H.-Y. Zhang, J.-R. Ding and Y.-Z. Peng, Sci. Rep., 2016, 6, 24825 CrossRef CAS PubMed.
- B. J. Harris, X. Cheng and P. Frymier, J. Phys. Chem. B, 2016, 120, 599–609 CrossRef CAS PubMed.
- B. C. Buer, J. L. Meagher, J. A. Stuckey and E. N. G. Marsh, Proc. Natl. Acad. Sci. U. S. A., 2012, 109, 4810–4815 CrossRef CAS PubMed.
- B. C. Buer, J. L. Meagher, J. A. Stuckey and E. N. G. Marsh, Protein Sci., 2012, 21, 1705–1715 CrossRef CAS PubMed.
- A. A. Berger, J.-S. Völler, N. Budisa and B. Koksch, Acc. Chem. Res., 2017, 50, 2093–2103 CrossRef CAS PubMed.
- F. Agostini, J.-S. Völler, B. Koksch, C. G. Acevedo-Rocha, V. Kubyshkin and N. Budisa, Angew. Chem., Int. Ed., 2017, 56, 9680–9703 CrossRef CAS PubMed.
- N. C. Yoder and K. Kumar, Chem. Soc. Rev., 2002, 31, 335–341 RSC.
- M. Salwiczek, E. K. Nyakatura, U. I. Gerling, S. Ye and B. Koksch, Chem. Soc. Rev., 2012, 41, 2135–2171 RSC.
- M. Oliver, C. Gadais, J. García-Pindado, M. Teixidó, N. Lensen, G. Chaume and T. Brigaud, RSC Adv., 2018, 8, 14597–14602 RSC.
- V. Asante, J. Mortier, H. Schlüter and B. Koksch, Bioorg. Med. Chem., 2013, 21, 3542–3546 CrossRef CAS PubMed.
- H. Meng and K. Kumar, J. Am. Chem. Soc., 2007, 129, 15615–15622 CrossRef CAS PubMed.
- C. Jäckel, M. Salwiczek and B. Koksch, Angew. Chem., Int. Ed., 2006, 45, 4198–4203 CrossRef PubMed.
- T. Liang, C. N. Neumann and T. Ritter, Angew. Chem., Int. Ed., 2013, 52, 8214–8264 CrossRef CAS PubMed.
- E. P. Gillis, K. J. Eastman, M. D. Hill, D. J. Donnelly and N. A. Meanwell, J. Med. Chem., 2015, 58, 8315–8359 CrossRef CAS PubMed.
- G. Pupo, F. Ibba, D. M. H. Ascough, A. C. Vicini, P. Ricci, K. E. Christensen, L. Pfeifer, J. R. Morphy, J. M. Brown, R. S. Paton and V. Gouverneur, Science, 2018, 360, 638–642 CrossRef CAS PubMed.
- J.-S. Völler, M. Dulic, U. I. Gerling-Driessen, H. Biava, T. Baumann, N. Budisa, I. Gruic-Sovulj and B. Koksch, ACS Cent. Sci., 2016, 3, 73–80 CrossRef PubMed.
- M. Imiołek, G. Karunanithy, W.-L. Ng, A. J. Baldwin, V. E. Gouverneur and B. G. Davis, J. Am. Chem. Soc., 2018, 140, 1568–1571 CrossRef PubMed.
- R. L. Baldwin, Proc. Natl. Acad. Sci. U. S. A., 2014, 111, 13052–13056 CrossRef CAS PubMed.
- D. Chandler, Nature, 2005, 437, 640–647 CrossRef CAS PubMed.
- H. W. Horn, W. C. Swope, J. W. Pitera, J. D. Madura, T. J. Dick, G. L. Hura and T. Head-Gordon, J. Chem. Phys., 2004, 120, 9665–9678 CrossRef CAS PubMed.
- J. A. Maier, C. Martinez, K. Kasavajhala, L. Wickstrom, K. E. Hauser and C. Simmerling, J. Chem. Theory Comput., 2015, 11, 3696–3713 CrossRef CAS PubMed.
- J. R. Robalo, S. Huhmann, B. Koksch and A. Vila Verde, Chem, 2017, 3, 881–897 CAS.
- W. L. Jorgensen and P. Schyman, J. Chem. Theory Comput., 2012, 8, 3895–3901 CrossRef CAS PubMed.
- P. Politzer, J. S. Murray and T. Clark, Phys. Chem. Chem. Phys., 2010, 12, 7748–7757 RSC.
- P. Metrangolo, J. S. Murray, T. Pilati, P. Politzer, G. Resnati and G. Terraneo, Cryst. Growth Des., 2011, 11, 4238–4246 CrossRef CAS.
- K. El Hage, T. Bereau, S. Jakobsen and M. Meuwly, J. Chem. Theory Comput., 2016, 12, 3008–3019 CrossRef CAS PubMed.
- M. A. A. Ibrahim, J. Phys. Chem. B, 2012, 116, 3659–3669 CrossRef CAS PubMed.
- M. Carter, A. K. Rappé and P. S. Ho, J. Chem. Theory Comput., 2012, 8, 2461–2473 CrossRef CAS PubMed.
- T. Bereau, C. Kramer and M. Meuwly, J. Chem. Theory Comput., 2013, 9, 5450–5459 CrossRef CAS PubMed.
- F. Hédin, K. El Hage and M. Meuwly, J. Chem. Inf. Model., 2016, 56, 1479–1489 CrossRef PubMed.
- H. Berendsen, D. van der Spoel and R. van Drunen, Comput. Phys. Commun., 1995, 91, 43–56 CrossRef CAS.
- E. Lindahl, B. Hess and D. van der Spoel, J. Mol. Model., 2001, 7, 306–317 CrossRef CAS.
- D. Van Der Spoel, E. Lindahl, B. Hess, G. Groenhof, A. E. Mark and H. J. C. Berendsen, J. Comput. Chem., 2005, 26, 1701–1718 CrossRef CAS.
- B. Hess, C. Kutzner, D. Van Der Spoel and E. Lindahl, J. Chem. Theory Comput., 2008, 4, 435–447 CrossRef CAS PubMed.
- S. Pronk, S. Páll, R. Schulz, P. Larsson, P. Bjelkmar, R. Apostolov, M. R. Shirts, J. C. Smith, P. M. Kasson, D. van der Spoel, B. Hess and E. Lindahl, Bioinformatics, 2013, 29, 845–854 CrossRef CAS PubMed.
-
S. Páll, M. J. Abraham, C. Kutzner, B. Hess and E. Lindahl, International Conference on Exascale Applications and Software, 2014, pp. 3–27 Search PubMed.
- M. J. Abraham, T. Murtola, R. Schulz, S. Páll, J. C. Smith, B. Hess and E. Lindahl, SoftwareX, 2015, 1, 19–25 CrossRef.
-
D. A. Case, V. Babin, J. T. Berryman, R. M. Betz, Q. Cai, D. S. Cerutti, T. E. Cheatham III, T. A. Darden, R. E. Duke, H. Gohlke, A. W. Goetz, S. Gusarov, N. Homeyer, P. Janowski, J. Kaus, I. Kolossváry, A. Kovalenko, T. S. Lee, S. LeGrand, T. Luchko, R. Luo, B. Madej, K. M. Merz, F. Paesani, D. R. Roe, A. Roitberg, C. Sagui, R. Salomon-Ferrer, G. Seabra, C. L. Simmerling, W. Smith, J. Swails, R. C. Walker, J. Wang, R. M. Wolf, X. Wu and P. A. Kollman, AMBER 14, 2014 Search PubMed.
- B. Hess, H. Bekker, H. J. C. Berendsen and J. G. E. M. Fraaije, J. Comput. Chem., 1997, 18, 1463–1472 CrossRef CAS.
- J.-P. Ryckaert, G. Ciccotti and H. J. Berendsen, J. Comput. Phys., 1977, 23, 327–341 CrossRef CAS.
-
M. P. Allen and D. J. Tildesley, Computer Simulation of Liquids, Oxford University Press, 1989 Search PubMed.
- H. J. Berendsen, J. v. Postma, W. F. van Gunsteren, A. DiNola and J. Haak, J. Chem. Phys., 1984, 81, 3684–3690 CrossRef CAS.
- C. H. Bennett, J. Comput. Phys., 1976, 22, 245–268 CrossRef.
- M. R. Shirts, E. Bair, G. Hooker and V. S. Pande, Phys. Rev. Lett., 2003, 91, 140601 CrossRef PubMed.
- N. A. Baker, D. Sept, S. Joseph, M. J. Holst and J. A. McCammon, Proc. Natl. Acad. Sci. U. S. A., 2001, 98, 10037–10041 CrossRef CAS PubMed.
- C. Gadais, E. Devillers, V. Gasparik, E. Chelain, J. Pytkowicz and T. Brigaud, ChemBioChem, 2018, 19, 1026–1030 CrossRef CAS PubMed.
-
A. V. Marenich, C. P. Kelly, J. D. Thompson, G. D. Hawkins, C. C. Chambers, D. J. Giesen, P. Winget, C. J. Cramer and D. G. Truhlar, Minnesota Solvation Database – version 2012, 2012 Search PubMed.
- D. Shivakumar, Y. Deng and B. Roux, J. Chem. Theory Comput., 2009, 5, 919–930 CrossRef CAS PubMed.
- D. Shivakumar, J. Williams, Y. Wu, W. Damm, J. Shelley and W. Sherman, J. Chem. Theory Comput., 2010, 6, 1509–1519 CrossRef CAS PubMed.
- D. Shivakumar, E. Harder, W. Damm, R. A. Friesner and W. Sherman, J. Chem. Theory Comput., 2012, 8, 2553–2558 CrossRef CAS PubMed.
- D. E. Shaw, P. Maragakis, K. Lindorff-Larsen, S. Piana, R. O. Dror, M. P. Eastwood, J. A. Bank, J. M. Jumper, J. K. Salmon, Y. Shan and W. Wriggers, Science, 2010, 330, 341–346 CrossRef CAS PubMed.
- K. Lindorff-Larsen, S. Piana, K. Palmo, P. Maragakis, J. L. Klepeis, R. O. Dror and D. E. Shaw, Proteins, 2010, 78, 1950–1958 CAS.
- K. Lindorff-Larsen, N. Trbovic, P. Maragakis, S. Piana and D. E. Shaw, J. Am. Chem. Soc., 2012, 134, 3787–3791 CrossRef CAS PubMed.
- K. A. Beauchamp, Y.-S. Lin, R. Das and V. S. Pande, J. Chem. Theory Comput., 2012, 8, 1409–1414 CrossRef CAS PubMed.
- S. Piana, J. L. Klepeis and D. E. Shaw, Curr. Opin. Struct. Biol., 2014, 24, 98–105 CrossRef CAS PubMed.
- P. Robustelli, S. Piana and D. E. Shaw, Proc. Natl. Acad. Sci. U. S. A., 2018, 115, E4758–E4766 CrossRef CAS PubMed.
- P. S. Nerenberg and T. Head-Gordon, Curr. Opin. Struct. Biol., 2018, 49, 129–138 CrossRef CAS PubMed.
- Q. A. Huchet, B. Kuhn, B. Wagner, H. Fischer, M. Kansy, D. Zimmerli, E. M. Carreira and K. Müller, J. Fluorine Chem., 2013, 152, 119–128 CrossRef CAS.
- Q. A. Huchet, B. Kuhn, B. Wagner, N. A. Kratochwil, H. Fischer, M. Kansy, D. Zimmerli, E. M. Carreira and K. Müller, J. Med. Chem., 2015, 58, 9041–9060 CrossRef CAS PubMed.
- C. Tan, Y.-H. Tan and R. Luo, J. Phys. Chem. B, 2007, 111, 12263–12274 CrossRef CAS PubMed.
- V. H. Dalvi and P. J. Rossky, Proc. Natl. Acad. Sci. U. S. A., 2010, 107, 13603–13607 CrossRef CAS PubMed.
- C. A. Hunter, Chem. Sci., 2013, 4, 1687–1700 RSC.
- S. Mondal, B. Biswas, T. Nandy and P. C. Singh, Phys. Chem. Chem. Phys., 2017, 19, 24667–24677 RSC.
- W. Caminati, S. Melandri, I. Rossi and P. G. Favero, J. Am. Chem. Soc., 1999, 121, 10098–10101 CrossRef CAS.
- H.-J. Schneider, Chem. Sci., 2012, 3, 1381–1394 RSC.
- J. Thomas, N. A. Seifert, W. Jäger and Y. Xu, Angew. Chem., Int. Ed., 2017, 56, 6289–6293 CrossRef CAS PubMed.
- C. Dalvit and A. Vulpetti, ChemMedChem, 2011, 6, 104–114 CrossRef CAS PubMed.
- J. A. Olsen, D. W. Banner, P. Seiler, B. Wagner, T. Tschopp, U. Obst-Sander, M. Kansy, K. Müller and F. Diederich, ChemBioChem, 2004, 5, 666–675 CrossRef CAS PubMed.
- D. O'Hagan, Chem. Soc. Rev., 2008, 37, 308–319 RSC.
- Q. A. Huchet, W. B. Schweizer, B. Kuhn, E. M. Carreira and K. Müller, Chem. – Eur. J., 2016, 22, 16920–16928 CrossRef CAS PubMed.
- Q. A. Huchet, N. Trapp, B. Kuhn, B. Wagner, H. Fischer, N. A. Kratochwil, E. M. Carreira and K. Müller, J. Fluorine Chem., 2017, 198, 34–46 CrossRef CAS.
- R. E. Rosenberg, J. Phys. Chem. A, 2012, 116, 10842–10849 CrossRef CAS PubMed.
- B. Lin and B. M. Pettitt, J. Chem. Phys., 2011, 134, 106101 CrossRef PubMed.
- B. Lin, K.-Y. Wong, C. Hu, H. Kokubo and B. M. Pettitt, J. Phys. Chem. Lett., 2011, 2, 1626–1632 CrossRef CAS PubMed.
- S.-C. Ou and B. M. Pettitt, J. Phys. Chem. B, 2016, 120, 8230–8237 CrossRef CAS PubMed.
- T. P. Creamer, R. Srinivasan and G. D. Rose, Biochemistry, 1995, 34, 16245–16250 CrossRef CAS PubMed.
- N. C. Fitzkee and G. D. Rose, Proc. Natl. Acad. Sci. U. S. A., 2004, 101, 12497–12502 CrossRef CAS PubMed.
- J. Estrada, P. Bernado, M. Blackledge and J. Sancho, BMC Bioinf., 2009, 10, 104 CrossRef PubMed.
- Y. Seki, Y. Shimbo, T. Nonaka and K. Soda, J. Chem. Theory Comput., 2011, 7, 2126–2136 CrossRef CAS PubMed.
- S. A. Ali, M. I. Hassan, A. Islam and F. Ahmad, Curr. Protein Pept. Sci., 2014, 15, 456–476 CrossRef CAS PubMed.
Footnotes |
† Electronic supplementary information (ESI) available: Description of force fields and simulation details, hydration free energies, electrostatic potential in the vicinity of amino acid side chains, linear models to reproduce the calculated hydration free energies, radial distribution functions, conformational changes in fluorinated side chains. AMBER-format force fields for mono- and di-fluorinated amino acids. See DOI: 10.1039/c8cp07025c |
‡ Hydrogen bonds exist if the donor–acceptor distance <3.5 Å and the donor-hydrogen-acceptor angle is between 135° and 180°. |
§ Values calculated from the relevant hCHmFn values in ESI† Table 8 and the corresponding k parameter in Table 1. |
|
This journal is © the Owner Societies 2019 |
Click here to see how this site uses Cookies. View our privacy policy here.