DOI:
10.1039/C9AY01132C
(Paper)
Anal. Methods, 2019,
11, 5289-5300
Analysis of multiclass cyanotoxins (microcystins, anabaenopeptins, cylindrospermopsin and anatoxins) in lake waters using on-line SPE liquid chromatography high-resolution Orbitrap mass spectrometry†
Received
28th May 2019
, Accepted 12th September 2019
First published on 16th September 2019
Abstract
Harmful algal blooms (HABs) of cyanobacterial origin have the potential to generate hundreds of secondary metabolites referred to as cyanotoxins. Freshwater resources have been threatened by HABs and an increase of these episodes is of major concern worldwide for risk management and ecosystem impacts. To meet the need to rapidly screen a wide range of cyanotoxins, a multi-toxin method based on on-line solid-phase extraction ultra-high performance liquid chromatography high-resolution mass spectrometry (SPE-UHPLC-HRMS) was developed and validated. This method enabled high-throughput screening of cylindrospermopsin, anatoxin-a, homoanatoxin-a, anabaenopeptins A and B, and twelve microcystins (-RR, [Asp3]-RR, -YR, -HtyR, -LR, [Asp3]-LR, -HilR, -WR, -LA, -LY, -LW and -LF) in recreational lake waters. Extraction and separation were achieved in 8 minutes, with limits of detection between 8 and 53 ng L−1. The method offered suitable precision for environmental samples (generally <20%), accuracy (81–113%), and low relative matrix effects (<29%). The method was used to analyze lake samples collected in Canada through a collaborative citizen-science project (Adopt a Lake campaign). The determination of 8 out of 17 targeted cyanotoxins from low ng L−1 to μg L−1 levels in these surface water samples showed the versatility of the method. MC-LR was detected in 75% of samples (0.03–3.5 μg L−1) and anabaenopeptins A and B in 38% of samples at concentrations of up to 10 μg L−1. A retrospective screening of extracted full scan HRMS chromatograms also suggested the presence of infrequently monitored MCs in these lake samples.
1. Introduction
Cyanobacteria are on the radar worldwide due to the increase of harmful algal bloom (HAB) episodes caused by global climate change and eutrophication due to anthropogenic activities such as agriculture.1–5 Under favorable conditions, these prokaryotic organisms produce harmful secondary metabolites known as cyanotoxins. These are noxious to aquatic ecosystems and may impact human activities through contamination of drinking water resources and recreational waters.6 Some cyanotoxins from the two main groups, peptides and alkaloids, have been linked to acute, chronic and lethal poisoning of wild and domestic animals, and many cases were also reported in humans.7 Considering the future global warming model, one can assume that these harmful episodes are on the rise.8,9 A broad and effective monitoring of cyanotoxins is therefore needed to better understand and predict the emergence of HABs.
Among the secondary metabolites produced by cyanobacteria, the first group includes non-ribosomal oligopeptides also called cyanopeptides, counting hundreds of identified bioactive compounds to date.10 We mainly associate the presence of toxic cyanobacterial blooms with the appearance of hepatotoxic microcystins (MCs) (Fig. 1). These are the most documented cyanotoxins and are associated with numerous cases of human intoxication due to their high occurrence during HAB episodes.11 Their hepatotoxicity ultimately results from the binding to protein phosphatases 1 and 2A, followed by disruption of cellular homeostasis. The most acute cases lead to liver necrosis and colorectal and liver cancer.12 Other bioactive cyanopeptides, such as anabaenopeptins (APs), cyanopeptolins and microginins, are, so far, not considered in most monitoring plans. However, it was demonstrated that MCs rarely appear alone during HAB episodes, and specific congeners from other cyanopeptides can even be dominant.13 Some studies suggest that some APs inhibit protease and protein phosphatases.14 AP-B and F have also been shown to induce lysis of cyanobacteria, which could influence the bioavailability of cell-bound cyanotoxins.15 Over 500 cyanopeptides have been structurally identified, including more than 240 MCs.16,17 The factors that lead to their production and their impact on human and animal health have only been studied for a few compounds. Cyanopeptides other than MCs have received little attention in recent years, yet their impact on ecosystems and human and animal health is of concern.10 For this reason, a more in-depth study of the presence of these compounds and their toxicological impact would be essential for a better implementation of risk management.
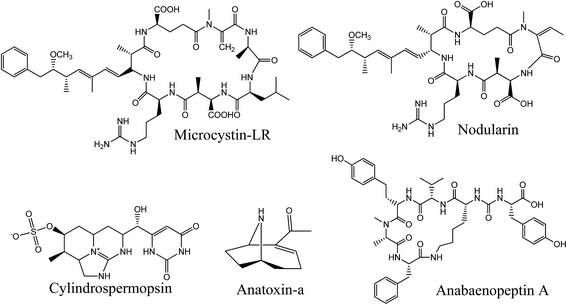 |
| Fig. 1 Structures of principal congeners from the targeted cyanotoxins. | |
Besides bioactive and toxic peptides, some cyanobacteria can also produce toxic alkaloids. Among them, the most common are anatoxin-a (ANA-a), homoanatoxin-a (HANA-a) and cylindrospermopsin (CYN). ANA-a and HANA-a are neurotoxins with a vast distribution in water sources.18,19 To date, many documented animal mortalities have been attributed to ANA-a.20 They mimic the neurotransmitter acetylcholine and if ingested at high doses, continuous stimulation of the respiratory muscles may lead to immediate asphyxiation.21 CYN is originally present in tropical and subtropical climates. Nevertheless, due to increasing temperature and cyanobacteria adaptation, the presence of CYN may be increasingly reported in originally temperate climates such as in Europe and North America.22,23 CYN exhibits cytotoxic, neurotoxic and hepatotoxic effects, acts as a tumor promoter and carcinogen, and affects organs by inhibiting protein synthesis.24
In recent years, new guidelines have been proposed for several cyanotoxins and different age groups. The World Health Organization (WHO) proposed a 1 μg L−1 threshold in drinking water for both free and cell-bound MC-LR.25,26 Several countries have adopted this regulatory value for the sum of all MC congeners that can be quantified, but some countries have also suggested maximum levels for ANA-a and CYN.27,28 The US EPA has implemented guidelines for MCs and CYN in drinking water for adults and children. Four US states (Minnesota, Ohio, Oregon and Vermont) have also included ANA-a and the “paralytic shellfish poison” saxitoxin in their guidelines.29,30 Ohio has introduced “do not drink” and “do not use” notices when cyanotoxin thresholds for drinking and recreational waters are reached, respectively.31 Quebec, on the other hand, has adopted a guideline for the quality of drinking water which must not contain more than 1.5 μg L−1 of MCs, expressed as MC-LR toxic equivalents.32 While guideline harmonization is not always achieved between different states, upstream work is also needed for understanding and predicting HABs. Improving toxin tracking using effective analytical methods appears as a critical research need, which is still hindered by the limited availability of certified standards and materials.
Environmental monitoring authorities face major challenges in the analysis of cyanotoxins. The wide variety of cyanotoxins and increasing number of water sources affected by cyanobacteria require high-throughput analytical approaches able to quantify multi-class toxins in environmental matrices.10,33 The method of choice to obtain such a degree of selectivity is liquid chromatography (LC) coupled to mass spectrometry (MS). Few methods have been able to quantify multi-class toxins, mainly due to extraction limitations and the range of properties of the targeted cyanotoxins. Solid-phase extraction (SPE) is usually preconized, as it provides better sensitivity and reproducibility.13,34–36 However, SPE sample preparation is time consuming and can be limiting when one has to promptly process hundreds of samples during a bloom season. One solution was to develop an automated extraction method directly coupled to LC-MS analysis. The use of on-line SPE has proven effective in past studies aiming at including diverse cyanotoxins in a single high-throughput method, without significantly affecting sensitivity.37–43 The methods proposed earlier can still be improved by expanding the scope of targeted cyanotoxins for a wider screening. Some studies have also explored the possibility of using high-resolution MS to quantify known cyanotoxins and identify unknown cyanopeptide variants using LC-QTOF or LC-Orbitrap.35,40,41,44 HRMS is increasingly used as a powerful tool to perform targeted analysis, with the further advantage of allowing suspect-target and non-target screening when certified standards are not available.
This study presents the application of an optimized rapid chromatographic method using on-line SPE-UHPLC coupled to quadrupole-Orbitrap HRMS for the determination of multi-class cyanotoxins in environmental surface water samples. For method development and validation purposes, 17 quantitatively targeted cyanotoxins were first listed, which included CYN, ANA-a, HANA-a, AP-A, AP-B and 12 MCs ([Asp3]RR, RR, YR, HtyR, [Asp3]LR, LR, HilR, WR, LA, LY, LW and LF). As part of the method optimization, different filters were tested for adequate sample pre-treatment. On-line SPE parameters were also investigated for a faster analysis without sacrificing recovery and accuracy performance. In this respect, the addition of a washing step after sample loading was critical for efficient matrix effect removal. Detection through HRMS allowed the mass distinction of ANA-a from its isobaric interference, the naturally encountered amino acid phenylalanine (Phe).45 Full scan (FS) and parallel reaction monitoring (PRM) modes were investigated to keep a maximum of information in the acquired data and improve the identification of difficult-to-measure compounds. Samples were collected through Adopt a Lake, a citizens' campaign throughout Quebec and Canada where people volunteered to collect samples from a nearby lake or waterbody (Fig. 2).46 The samples from the various lakes (N = 16) were analyzed for the 17 quantitatively targeted cyanotoxins. In addition, a retrospective screening of extracted full scan HRMS chromatograms was conducted to investigate the presence of less frequently monitored compounds. To the best of the authors' knowledge, this method includes the widest range of cyanobacterial toxins in a single automated workflow.
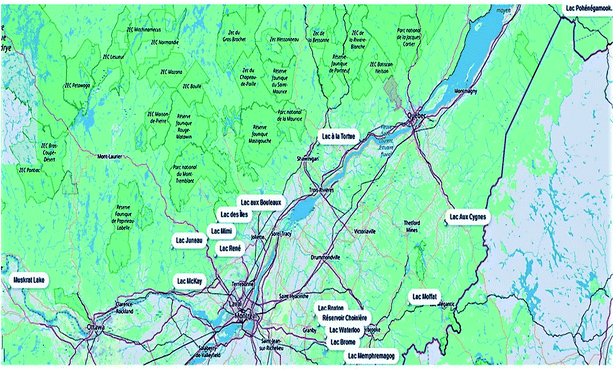 |
| Fig. 2 Geographical distribution of the lake samples (n = 16) collected during the Adopt a Lake campaign (Québec and Ontario, Canada). | |
2. Experimental
2.1. Chemicals, reagents and stock solutions
Anatoxin-a (ANA-a), cylindrospermopsin (CYN), microcystin-LR (MC-LR), [Asp3]MC-LR and MC-RR (purity ≥ 99%) were purchased from the National Research Council of Canada (Halifax, NS, Canada). Nodularin-R (NOD-R), [Asp3]MC-RR, MC-YR, LA, LY, LW, LF, WR, HtyR and HilR (purity ≥ 95%) were purchased from Enzo Life Sciences (Farmingdale, NY, USA). Homoanatoxin-a (HANA-a, purity ≥ 99%) was obtained from Abraxis, Inc. (Warminster, PA, USA), Anabaenopeptin A and B (AP-A, AP-B, purity ≥ 90%) from Cyano Biotech GmbH (Berlin, Germany), and MC-LR(15N10) (95%) from Cambridge Isotopes Laboratories, Inc. (Tewksbury, MA, USA). Individual stock solutions of ANA-a, CYN, MC-LR, [Asp3] MC-LR and MC-RR were kept at −20 °C for a maximum of six months. All other individual stock solutions were prepared in methanol (MeOH) at a concentration of 25 mg L−1 and were kept at −20 °C for a maximum of one year. Primary working solutions were prepared at a concentration of 100 μg L−1 for targeted cyanotoxins and 9 μg L−1 for internal standards (ISs: MC-LR(15N10) and NOD-R) by dilution in MeOH of individual stock solution aliquots. Subsequent working solutions were prepared daily in water to give solutions of desired concentration. All organic solvents and water used for dilutions were of HPLC grade purity from Fisher Scientific (Whitby, ON, Canada).
2.2. Sample collection and preparation
Samples were collected through Adopt a Lake, a citizens' campaign throughout Quebec and Ontario, Canada. The participants of this campaign volunteer by collecting a sample from a lake. Samples were collected in 2018 between May and the end of September, mostly from recreational lakes (Fig. 2). At each sampling location, a duplicate set of samples was collected in 125 mL amber polyethylene terephthalate glycol-modified (PETG) bottles (Thermo Scientific Nalgene, Waltham, MA, USA).47 The PETG bottles were previously rinsed three times with the surface water from the site. The bottles were then filled to the brim, sealed, stored at −20 °C until shipment and sent to the laboratory within 3 days. Both free and cell-bound cyanotoxins were assessed by quantifying both fractions together. Upon arrival at the laboratory, the whole samples underwent three freeze-thawing cycles (to lyse cyanobacterial cells) and were subsequently filtered through 25 mm diameter, 0.2 μm pore size Acrodisc GH Polypro (GHP) filters (Waters, Milford, MA, USA) using a vacuum filtration unit with a vacuum pump. A volume of 1450 μL of each filtered sample was transferred into 2 mL amber glass vials and kept at −20 °C until analysis. Prior to analysis, the internal standards were added for a final concentration of 300 ng L−1.
2.3 Instrumental conditions
A Thermo Scientific Dionex UltiMate 3000 RS pump and column compartment were used for chromatographic separation. A Dionex UltiMate 3000 pump was coupled to the system used for on-line SPE. Both pumps are controlled by Chromeleon 7.2 Software (Thermo Fisher Scientific, Waltham, MA and Dionex Softron GMbH part of Thermo Fisher Scientific, Germany). A PAL system RTC autosampler was used (Zwingen, Switzerland) for sample injection. A Hypersil Gold aQ (20 × 2 mm, 12 μm particle size, 175 Å pore size) column was used for on-line SPE. Chromatographic separation was performed with a Hypersil Gold C18 column (100 × 2.1 mm, 1.9 μm particle size, 175 Å pore size) kept at 55 °C. A Q-Exactive mass spectrometer was controlled by Xcalibur 3.0 software (Thermo Fisher Scientific, Waltham, MA). Instrument calibration was performed every 5 days with direct infusion of an LTQ Velos ESI Positive Ion Calibration Solution (Pierce Biotechnology Inc. Rockford, IL). The mass accuracy for all target compounds was within ±5 ppm.
2.3.1 On-line solid-phase extraction and chromatographic conditions.
On-line SPE and chromatographic conditions are presented in Table 1 and were adapted from a previous study with some modifications.38 A washing step was added to the initial method for matrix removal. The wash volume was investigated at three levels (0, 0.5 and 1.0 mL of wash volumes), using surface water (lake) samples spiked at 250 ng L−1 with native compounds. Under the optimized conditions, 1 mL of the sample was injected and the sample transfer time (loading speed) from the injection loop to the SPE column was 1 mL min−1. An additional wash volume of 0.5 mL was allowed to flow through the column following the sample loading step. The on-line pre-concentration column was then eluted using the analytical pump gradient; the eluting analytes were transferred directly to the analytical column for separation. Chromatographic separation proceeded using a gradient program with solvents ACN (B) and water (A) (both with 0.1% formic acid) at a flow rate of 550 μL min−1. The method run time was 8 min per sample.
Table 1 Details on the on-line SPE-UHPLC chromatographic gradient program
|
Loading pump (SPE) |
Analytical pump (UHPLC) |
|
Time (min) |
Aa (%) |
Bb (%) |
Flow rate (μL min−1) |
Time (min) |
Ac (%) |
Bd (%) |
Flow rate (μL min−1) |
A: H2O + 0.1% formic acid.
B: methanol + 0.1% formic acid.
A: H2O + 0.1% formic acid.
B: acetonitrile + 0.1% formic acid.
|
On-line SPE loading step and wash |
0 |
100 |
0 |
1000 |
0 |
90 |
10 |
550 |
Column re-equilibration |
1.5 |
100 |
0 |
1000 |
1.5 |
90 |
10 |
550 |
Loop wash |
1.6 |
0 |
100 |
1500 |
4.4 |
0 |
100 |
550 |
Elution and chromatographic separation |
5.4 |
0 |
100 |
1500 |
6.4 |
0 |
100 |
550 |
SPE column conditioning |
5.5 |
100 |
0 |
1000 |
6.5 |
90 |
10 |
550 |
Column re-equilibration |
8 |
100 |
0 |
1000 |
8 |
90 |
10 |
550 |
2.3.2 HRMS conditions.
A heated electrospray ionization interface (HESI-II) operated in positive mode was used for analyte ionization. The source parameters were set as follows: the ionization spray voltage was set at +3500 V; capillary temperature was set at 350 °C; the vaporizer temperature was set at 250 °C; sheath gas and auxiliary gas flow were set at 60 and 15 arbitrary units, respectively. HRMS parameters such as resolving powers, automatic gain control (AGC) and maximum injection time (IT) were selected for optimal selectivity and sensitivity. CYN, ANA-a, HANA-a, MC-LA and MC-LW were monitored using the parallel reaction monitoring (PRM) scan mode to ensure a better selectivity. High noise was observed for complex matrices using the full scan (FS) mode at different tested resolving powers. Therefore, a PRM scan was set between 1.5 and 4.7 min for those difficult-to-measure compounds. For the PRM mode, the resolving power was set at 17
500 full width at half maximum (FWHM) at m/z 200 and the AGC and IT parameters were set respectively at 1 × 105 ions capacity and 50 ms filling. Other targeted cyanotoxins were acquired using the FS mode. For the FS mode, a mass range of m/z 150–1200 was applied, and the resolving power was set at 70
000 FWHM at m/z 200 (for singly charged microcystins, the corresponding resolution at m/z 1000 was ∼31
000 FWHM). The scan rate was 3 Hz, yielding between 9 and 30 points per chromatographic peak depending on the particular compound (on average, 12 points per chromatographic peak). Table 2 presents the detailed HRMS optimized parameters including retention time (RT), selected scan mode, ionization form, normalized collision energy (NCE) if applicable, exact masses of precursors and fragment ions, and the corresponding IS.
Table 2 Details on the optimized HRMS parameters
Cyanotoxins |
RT (min) |
Ionization |
PRM scan time (min) |
Exp. massa (m/z) |
Mass accuracy (ppm) |
NCEb (%) |
IS |
Precursor ion → fragment ion.
NCE: normalized collision energy.
|
CYN |
2.31 |
[M + H]+ |
1.5–3.0 |
416.1 → 194.12879 |
1.3 |
45 |
MC-LR(15N10) |
416.1 → 336.16663 |
1.2 |
40 |
ANA-a |
2.32 |
[M + H]+ |
1.5–3.0 |
166.1 → 149.09609 |
3.8 |
10 |
NOD |
166.1 → 131.08553 |
4.0 |
15 |
HANA-a |
2.63 |
[M + H]+ |
2.0–3.1 |
180.1 → 163.11174 |
2.6 |
40 |
NOD |
180.1 → 145.10118 |
3.0 |
45 |
MC-LA |
4.05 |
[M + H]+ |
3.7–4.5 |
910.5 → 776.41888 |
1.3 |
16 |
NOD |
910.5 → 402.21090 |
1.5 |
20 |
MC-LW |
4.22 |
[M + H]+ |
3.7–4.7 |
1025.5 → 891.46108 |
0.6 |
10 |
MC-LR(15N10) |
1025.5 → 517.25301 |
1.5 |
20 |
AP-A |
3.55 |
[M + H]+ |
— |
844.42297 |
1.8 |
— |
NOD |
AP-B |
3.29 |
[M + H]+ |
— |
837.46228 |
0.02 |
— |
MC-LR(15N10) |
[Asp3]MC-RR |
3.45 |
[M + 2H]2+ |
— |
519.79071 |
0.04 |
— |
MC-LR(15N10) |
MC-RR |
3.42 |
[M + 2H]2+ |
— |
512.78351 |
1.2 |
— |
MC-LR(15N10) |
MC-YR |
3.61 |
[M + H]+ |
— |
1045.53528 |
0.6 |
— |
MC-LR(15N10) |
MC-HtyR |
3.62 |
[M + H]+ |
— |
1059.55139 |
0.1 |
— |
NOD |
MC-LR |
3.66 |
[M + H]+ |
— |
995.5564 |
0.2 |
— |
MC-LR(15N10) |
[Asp3]MC-LR |
3.66 |
[M + H]+ |
— |
981.54083 |
0.1 |
— |
MC-LR(15N10) |
MC-HilR |
3.70 |
[M + H]+ |
— |
1009.57178 |
0.5 |
— |
MC-LR(15N10) |
MC-WR |
3.70 |
[M + H]+ |
— |
1068.55139 |
0.4 |
— |
MC-LR(15N10) |
MC-LY |
4.06 |
[M + H]+ |
— |
1002.52014 |
1.3 |
— |
NOD |
MC-LF |
4.28 |
[M + H]+ |
— |
986.52448 |
0.6 |
— |
MC-LR(15N10) |
NOD |
3.57 |
[M + H]+ |
— |
825.44977 |
1.6 |
— |
— |
MC-LR(15N10) |
3.66 |
[M + H]+ |
— |
1005.52686 |
0.5 |
— |
— |
2.4 Analyte identification and quantification
The positive identification of target analytes was confirmed by matching chromatographic retention times within ±2% of those from standards spiked in matrix-matched surface water. For a suitable selectivity in data analysis, the exact mass tolerance was set to ±5 ppm for the extracted m/z values from acquisition.48 The quantification of targeted cyanotoxins relied on relative responses of the area of chromatographic peaks, considering the area ratio of native analyte to the selected ISs, NOD-R or MC-LR(15N10). The concentration in field samples was derived from the area ratio of chromatographic peaks in the particular sample and the matrix-matched surface water calibration curve. If results were found to be outside the dynamic range, a new sample preparation was performed using a dilution factor so that the concentration can fit in the calibration curve. The full scan HRMS extracted chromatograms were also subjected to retrospective screening of additional cyanotoxins. These compounds were previously reported in Meriluoto et al. (2017) and were screened within a ±5 ppm window of their theoretical exact mass.17
2.5 Method validation
For all the validation steps and quantification quality controls (QCs), analytes were spiked in composite surface waters consisting of analyte-free lake water sampled before HAB seasons (referred to as matrix-matched surface water) and the absence of analyte was confirmed by LC-MS analysis.
A filtration step was used prior analysis to remove suspended cell debris from the surface water samples. To lessen the impact of the filter material on cyanotoxin concentrations, we set out to compare two filter types: 0.2 μm GH polypro (GHP) filters vs. 0.2 μm nylon filters (Sterlitech Corporation, Kent, WA), both reported to be optimal.38,41 Five replicates were spiked at a mid-level concentration (200 ng L−1) and recovery values (%) were obtained with the ratio between samples spiked before filtration and after filtration.
Method detection limits (MDLs) and method quantification limits (MQLs) were derived as 3 and 10 times, respectively, the standard deviation of replicate analyses of spiked surface water samples (n = 10). The spike concentration was selected as approximately 5 times the estimated MDL (between 10 and 50 ng L−1, depending on the analyte). Linearity was evaluated based on an 8-point internal calibration curve prepared with matrix-matched surface water at concentration levels ranging from the MQL to 1000 ng L−1 (IS concentration set at 300 ng L−1). Relative matrix effects were evaluated by using standard addition calibration curves. The slopes in matrix-matched surface water samples were compared to those in three selected samples from this study. Extraction recoveries were determined in a previous study by comparing the mean peak areas of the selected cyanotoxins from a direct chromatographic injection with those from on-line SPE injection.38 Accuracy, defined as the quotient of the obtained vs. expected concentration (%), and intra-day precision, expressed as relative standard deviation (RSD, %), were determined in matrix-matched surface water at 4 spiking levels selected within the linearity range (75 and 120 ng L−1, n = 7; 200 and 800 ng L−1, n = 5). Inter-day precision was also evaluated at 4 spiking levels and was derived from the relative standard deviation (%RSD) obtained over 3 different days.
2.6 Data handling and statistical analyses
Data treatment was performed using Xcalibur 3.0 Software (Thermo Fisher Scientific, Waltham, MA, USA). Chromatographic peaks of targeted cyanotoxins were integrated using Xcalibur Qual Browser according to their experimental mass (m/z) and retention time (Table 1). Calibration curves and sample quantification were based on area ratios of native compounds to their corresponding IS. Statistical tests were performed with the Statistical Package for Social Science (SPSS 21.0, Chicago, IL, USA). ANOVA and Tukey's post hoc tests were used with statistical significance defined as P < 0.05.
3. Results and discussion
3.1. Filtration conditions
The choice of an appropriate filtration material to remove suspended cell debris is critical to ensure sample integrity. Undesirable interactions between the filter material and the analytes of interest may negatively affect the total recovery of the method. This was extensively discussed in previous cyanotoxin studies and it was demonstrated that the mostly used membrane for MCs, glass fiber filters (GF/F), was not showing optimal results when analyzing a higher number of multi-class cyanotoxins due to their diverse range of physicochemical properties.38 Therefore, two types of materials have been reported as optimal for environmental waters, depending on the targeted cyanotoxins: nylon and polypropylene (GH polypro-GHP).38,41 Nylon and GHP membranes are both hydrophilic, implying that lower interactions would occur with less hydrophilic compounds such as MCs. GHP filters are made of hydrophilic polypropylene, which is supposed to reduce unwanted interactions making them potentially universal filters compared with polypropylene whose surface is known to adsorb MCs.49 Considering the broad range of cyanotoxins in the present study, analyte recoveries were evaluated using these two materials (Fig. 3). Membranes with a pore size of 0.2 μm can remove the majority of cyanobacterial cells while reducing the amount of particles entering the chromatographic columns to ensure a longer lifetime. Although nylon offers good recoveries for a number of compounds, there are some exceptions and mean recoveries are from 8 to 100%. GHP yielded better recoveries for all targeted compounds with mean recoveries varying between 82 and 108%, without exceptions. These results are in accordance with previous studies showing virtually no analyte losses using this membrane type.41,50
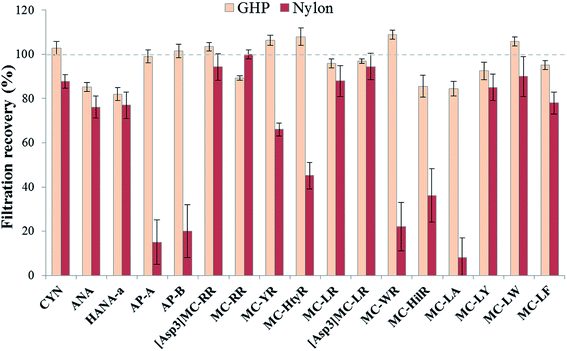 |
| Fig. 3 Comparison of recovery (%) values (mean ± SD, n = 5, 200 ng L−1) of two filter materials, GHP (GH polypropylene) and nylon, spiked in matrix-matched lake water. GHP showed virtually no loss of the targeted compounds and yielded more consistent recoveries. | |
3.2. Optimization of the on-line SPE procedure
On-line SPE conditions were optimized according to previous work that detected six MCs, ANA-a and CYN using an SPE-UHPLC coupled to a triple quadrupole mass spectrometer.38 The injected volume was set at 1 mL for high-throughput convenience while limiting analyte breakthrough. Additionally to the optimized parameters, a wash step was added consecutively to sample loading. The on-line aqueous mobile phase is maintained after sample enrichment, but before the elution step. The wash volume passing through the column should be optimized so that satisfactory matrix removal is attained while limiting analyte breakthrough. The influence of wash volume (no wash applied vs. 0.5 or 1 mL) for selected cyanotoxins is illustrated in Fig. 4. Between 0 mL and 0.5 mL, the analyte signal improved significantly (e.g., 2.5× for MC-YR, 2.5× for [Asp3]MC-LR, 3× for MC-LF, 1.5× for CYN). The improvement between 0 mL and 0.5 mL is modest for more hydrophilic compounds such as ANA-a and HANA-a, presumably due to some breakthrough losses. Between 0.5 mL and 1 mL, the analyte signal either improved by a small margin (e.g., MC-YR), or decreased significantly, especially for more hydrophilic compounds (e.g., CYN, ANA-a). In view of the above, we selected a 0.5 mL wash volume.
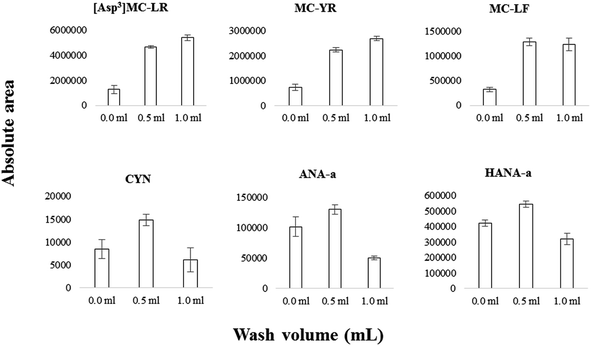 |
| Fig. 4 Optimization of the on-line SPE wash step consecutive to sample loading using matrix-matched lake water spiked at 250 ng L−1 with native cyanotoxins, evaluated at three different on-line SPE wash volumes as shown on the x-axis: 0, 0.5 and 1 mL. The y-axis represents the signal intensity. The error bars correspond to the standard deviation of five replicates (n = 5). | |
Extraction recoveries depend on organic modifiers and pH (which was maintained the same) and injection volume, which was lowered compared to our previous method.38 According to this study, on-line SPE recoveries evaluated with spiked matrix-matched water were acceptable and ranged from 72 to 102% for the range of targeted cyanotoxins.
Historically, the most challenging chromatography step was the adjustment of the elution gradient conditions for analysis of ANA-a when the detection is conducted with standard resolution mass spectrometry.24,51–53 Phe is an isobaric compound to ANA-a, detected within algal blooms, and with the two most abundant fragments of ANA-a in common. If not well-separated from ANA-a, Phe could thus result in false positives during MS detection. In our previous study using liquid chromatography coupled to tandem mass spectrometry, two different organic eluents were included in the chromatographic gradient to achieve resolution of ANA-a from its isobaric interference.38 However, HRMS has adequate resolving power to distinguish both compounds.45 In view of this, the choice of organic solvents and the elution gradient program was simplified without compromising the integrity of the analysis (Section 2.3).
3.3. HRMS conditions
The evaluation of MS scan modes used for quantification purposes is essential to ensure reliable data, depending on the compounds and matrices. Solliec et al. (2015) demonstrated that the PRM mode can provide better sensitivity and selectivity for a majority of targeted veterinary antibiotics in swine manure compared to the FS mode using a Q-Orbitrap mass spectrometer.48 Moreover, the PRM mode is commonly used with triple quadrupole mass spectrometers for the determination of MCs using the common product ion m/z 135 for quantification.17 However, using such a mode does not allow acquisition of expanded data on the samples in order to obtain a retrospective analysis of suspect MCs. Nonetheless, the FS mode can be efficient for quantitative purposes and this has been confirmed in several studies.54–56 As mentioned above, the use of high-resolution FS presents other advantages, for instance the retrospective analysis of suspect-target analytes in environmental samples. To determine which of the two scan modes is favored for better identification, the extracted chromatograms from unspiked surface water were compared to the same water spiked at 200 ng L−1 in both scan modes. ANA-a, HANA-a and CYN are early-eluting, low mass compounds and, unsurprisingly, undesirable peaks can be observed in their mass range. For this reason, these compounds were detected using the PRM mode to improve the confidence in their identification. MC-LW and MC-LA were two other difficult-to-measure compounds for which the PRM mode proved useful. The illustrative chromatograms in Fig. S1† demonstrate the improvement of identification using the PRM mode. The other targeted compounds were satisfactorily extracted from the FS analysis. Both scan modes were operated in parallel between 3 and 4.7 min, but the data acquired for each chromatographic peak were sufficient to be quantitative despite the increased duty cycle. Using the combined PRM-FS approach, suitable chromatographic performances were obtained in the surface water matrix for all the targeted compounds (Fig. 5). The full scan MS mode was also useful for conducting a fast retrospective screening of additional cyanotoxins not included in the list of quantitatively targeted analytes.
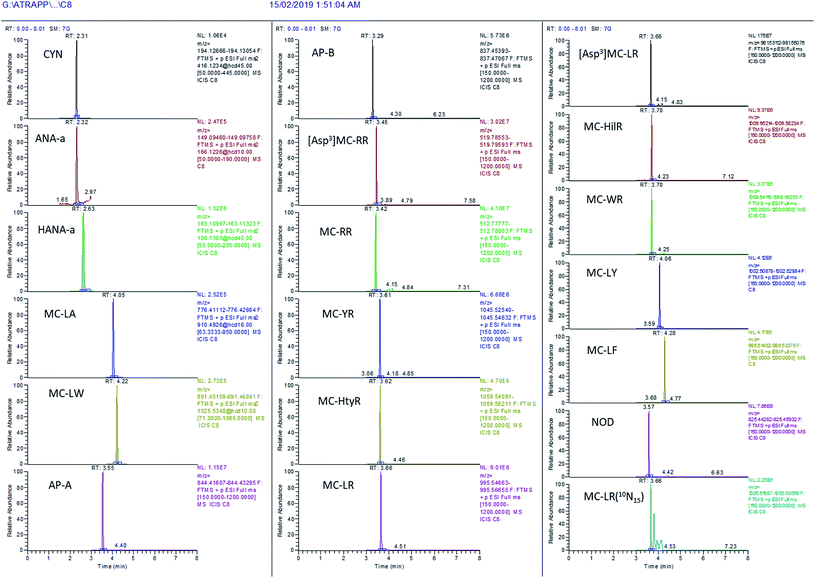 |
| Fig. 5 Illustration of the chromatographic performance of the present on-line SPE – UHPLC-HRMS method in surface water spiked at 1000 ng L−1 with the targeted cyanotoxins. | |
3.4. Analytical method validation
Matrix-matched calibration curves were used to limit the impact of matrix effects on the reported results. The matrix-matched water consisted of composite surface water sampled in the lakes under study in spring, before the HAB season to ensure that it is analyte-free. Whether this composite matrix would correctly compensate matrix effects for individual lake samples should, however, be validated. These lakes are often more loaded with organic matter and nutrients during the summer season in addition to the potential increase in biomass caused by the appearance of cyanobacterial cells. Therefore, the matrix effects caused by these potentially more complex summer water samples were assessed by comparison with the matrix-matched water sample. For this purpose, relative matrix effects were evaluated by comparing the slope of the matrix-matched calibration curve (used for quantification in the present study) vs. the slopes of standard additions to selected lake samples from this study: Lac Mimi, Lac à la Tortue, and Lac Juneau. Positive matrix effect values (%) indicate signal enhancement, while negative values indicate signal suppression. Matrix effects remained in most instances within ±20% (Table 3), an acceptable range for complex environmental samples, given the limited number of internal standard types available. Significant matrix effects were observed for CYN when using NOD-R as the internal standard (unpublished data), but the matrix effect was lowered within ±30% when using isotopically labelled MC-LR (Table 3). Finding appropriate ISs for the analysis of emerging contaminants is often a challenge, and cyanotoxins are no exception since only a few isotopically labelled compounds are available in the market.
Table 3 Summary of R2, method detection limits (MDL), method quantification limits (MQL), and matrix effects (%)
Cyanotoxins |
R
2
|
MDL (ng L−1) |
MQL (ng L−1) |
Matrix effects (%) |
Lac Mimi |
Lac à la Tortue |
Lac Juneau |
CYN |
0.9987 |
53 |
176 |
29 |
13 |
22 |
ANA-a |
0.9997 |
15 |
49 |
12 |
9 |
12 |
HANA-a |
0.9996 |
11 |
36 |
1 |
−4 |
−13 |
AP-A |
0.9998 |
20 |
65 |
−2 |
7 |
3 |
AP-B |
0.9995 |
12 |
39 |
−14 |
−14 |
−8 |
[Asp3]MC-RR |
0.9996 |
16 |
52 |
5 |
−12 |
12 |
MC-RR |
0.9996 |
8 |
27 |
5 |
−0.2 |
13 |
MC-YR |
0.9998 |
24 |
80 |
−4 |
−6 |
−7 |
MC-HtyR |
0.9995 |
30 |
98 |
1 |
9 |
3 |
MC-LR |
0.9998 |
20 |
67 |
−12 |
−18 |
−13 |
[Asp3]MC-LR |
0.9998 |
14 |
46 |
−5 |
−9 |
−6 |
MC-HilR |
0.9995 |
21 |
71 |
−7 |
−8 |
−10 |
MC-WR |
0.9994 |
36 |
120 |
−3 |
8 |
4 |
MC-LA |
0.9998 |
20 |
67 |
−8 |
8 |
3 |
MC-LY |
0.9991 |
35 |
116 |
6 |
8 |
−12 |
MC-LW |
0.9997 |
38 |
126 |
−5 |
6 |
18 |
MC-LF |
0.9996 |
27 |
89 |
−9 |
−11 |
−8 |
Matrix-matched calibration curves showed suitable linearity, with R2 ≥ 0.998 (Table 3). Residuals for the different calibration levels were within ±20% of the linear fit, except for the first calibration level close to the LOQ. The precision (%RSD) and accuracy (%) were also suitable as shown in the ESI (ESI Table S1†). Intra-day precision ranged from 1.2 to 17%, with the exception of MC-LY with a RSD of 48% at the low spike level (120 ng L−1). Inter-day precision varied from 3.1 to 19% with the exception of MC-LY with a RSD of 31% at the low spike level (120 ng L−1). The MDL and MQL values ranged from 8 to 53 ng L−1 and from 27 to 176 ng L−1, respectively (Table 3). These values are substantially lower than the established and proposed water quality guidelines from different jurisdictions. Water quality guidelines in drinking water can vary from 0.1 to 1.6 μg L−1 for MC-LR or total MCs (WHO, USA states depending of age groups), 0.7 to 3 μg L−1 for CYN (USA states, depending on age groups), and 0.7 to 6 μg L−1 for ANA-a (USA states depending on age groups, Canada and New Zealand).25,26 The method appears as a valid and sensitive approach for the screening of targeted MCs, CYN and ANA-a for risk management purposes and likely for other non-targeted cyanotoxins.
For internal standardization purposes, the isotopically labelled analog of the targeted compound is generally the best choice for an efficient correction. In our previous work, native NOD-R was, however, used as the internal standard for microcystins, the compound having a structure analogous to that of cyanopeptides.38 The reasons for this choice were the low probability of finding this cyanotoxin in targeted freshwater lake samples and the fact that commercially available isotopically labelled compounds for cyanotoxins were not readily available or costly. In this work, MC-LR(15N10) was evaluated and compared to NOD-R. For most of the targeted cyanotoxins, MC-LR(15N10) offered better quantitative correction, but ANA-a, HANA-a, MC-LA MC-HtyR and MC-LY showed better results with NOD-R. In the present work focusing on freshwaters, we did not detect any samples containing NOD-R that could have contributed to the measured spiked NOD-R concentration used for internal standardization.
3.5. Method application to the fast screening of surface water
The SPE-LC-HESI-HRMS method was used to analyze multi-class cyanotoxins in surface water samples from lakes monitored across Québec and Ontario (Canada). The samples were collected through the Adopt a Lake crowd-sourcing campaign, an initiative conducted to raise awareness about the protection of natural resources affected by cyanobacteria.46 Citizens interested in knowing the state of health of nearby lakes participated by monetary donation or by helping to sample a lake. The volunteers received a sampling box including protocols and necessary equipment.
From the lakes that were sampled between May and the end of September 2018, all were reported to contain cyanotoxins with concentrations varying between 9 and 10
000 ng L−1 (Table 4). Higher variability, shown with higher RSD on replicate field samples, was observed in some instances, more particularly for the lower-range concentrations close to the limit of detection. This variability may also be attributed to the sample nature and the sampling procedures, independently of the analytical method. Citizens were individually in charge of collecting the samples, which could be locally inhomogeneous, thus increasing the probability of error during the sampling of field replicates. Concentrations could also be underestimated if degradation occurred between sampling and analysis in the laboratory. The presence of concentration levels well above target criteria for drinking water, confirmed by the value of citizen-initiated sampling, allowed us to identify contaminated lakes that are not systematically tracked for toxic blue-green algae. The results of three samples analysed for taxonomy at the genus level by the 16S rRNA methodology also revealed that the Microcystis genus was present in these samples.
Table 4 Cyanotoxin detection in lakes from the Adopt a Lake campaign (Québec and Ontario, Canada). Concentrations are reported in ng L−1 with standard variation of duplicate analysis (ND: analyte not detected)
Sampling date |
Sampling site |
ANA-a |
[Asp3]MC-RR |
MC-RR |
MC-LR |
MC-HilR |
MC-LA |
AP-A |
AP-B |
Some reported concentrations are between LDM and LQM and should be considered indicative and not quantitative. Only the analytes with results > LDM are presented.
|
2018-05-26 |
Lac Juneau |
ND |
ND |
ND |
89 ± 61 |
ND |
ND |
ND |
ND |
2018-06-03 |
Lac aux Bouleaux |
32 ± 45a |
ND |
ND |
47 ± 67a |
52 ± 38 |
ND |
ND |
ND |
2018-06-25 |
Lac Moffatt |
ND |
ND |
18 ± 26a |
85 ± 60 |
ND |
ND |
ND |
ND |
2018-07-14 |
Lac Roxton |
ND |
ND |
ND |
ND |
111 ± 58 |
23 ± 10a |
ND |
1160 ± 870 |
2018-07-28 |
Réservoir Choinière |
38 ± 53a |
ND |
ND |
1240 ± 460 |
ND |
ND |
ND |
107 ± 4 |
2018-07-28 |
Lac Brome |
35 ± 49a |
ND |
49 ± 1 |
ND |
ND |
ND |
ND |
ND |
2018-07-28 |
Lac Memphrémagog |
ND |
252 ± 142 |
278 ± 27 |
3500 ± 920 |
ND |
80 ± 6a |
8280 ± 170 |
10 000 ± 3200 |
2018-07-28 |
Lac Waterloo |
ND |
ND |
ND |
ND |
70 ± 21 |
ND |
ND |
162 ± 14 |
2018-08-05 |
Lac à la Tortue |
ND |
24 ± 33 |
ND |
37 ± 23a |
ND |
ND |
ND |
ND |
2018-08-22 |
Lac McKay |
54 ± 33 |
ND |
ND |
29 ± 10a |
65 ± 18 |
690 ± 200 |
ND |
ND |
2018-09-01 |
Lac Pohénégamook |
23 ± 33a |
ND |
ND |
48 ± 16a |
30 ± 4a |
ND |
85 ± 17 |
53 ± 10 |
2018-09-03 |
Lac aux Cygnes |
79 ± 12 |
ND |
ND |
ND |
ND |
ND |
ND |
ND |
2018-09-03 |
Lac René |
59 ± 38 |
ND |
ND |
138 ± 58 |
ND |
ND |
ND |
ND |
2018-09-17 |
Lac des Îles |
54 ± 1 |
ND |
ND |
64 ± 33 |
ND |
ND |
ND |
ND |
2018-09-19 |
Muskrat lake, ON |
58 ± 2 |
ND |
17 ± 12a |
306 ± 28 |
32 ± 25a |
ND |
88 ± 20 |
83 ± 30 |
2018-09-30 |
Lac Mimi |
ND |
32 ± 5 |
ND |
108 ± 9 |
ND |
ND |
ND |
ND |
Nine lakes were positive to ANA-a, with concentrations ranging between 23 and 79 ng L−1. These concentrations are relatively low and would not pose an immediate threat to human health. Nevertheless, the detection of ANA-a suggests that ANA-a cyanobacteria producers are present, and higher concentrations could be observed when conditions are favorable or if a larger number of sites were sampled.
Out of the 12 quantitatively targeted microcystins, 5 were recurrently detected in these lake samples (Table 4). MC-LR was the most frequently detected microcystin (12/16 samples) and it was also found at the highest concentrations (range in positive samples: 29–3476 ng L−1). MC-HilR was the second most often detected microcystin (6/16 samples), while other detected MCs were less recurrent. When 2 or more MCs were detected, MC-LR was often predominant, except for Lac McKay where MC-LA dominated the MC profile (Table 4). Two particular samples, Réservoir Choinière and Lac Memphrémagog, had summed MC levels higher than the proposed recommendations for MC-LR equivalents by the World Health Organization (WHO) for drinking water (1 ug L−1). For recreational water, guidance and action levels vary between 0.8 μg L−1 and 20 μg L−1 in different American states and are set at 20 μg L−1 in Canada. The targeted MCs in Lac Memphrémagog and Réservoir Choinière had totalized concentrations of 4.1 and 1.4 μg L−1, respectively, which are lower than the Canadian guidance level for recreational water. These values are still a potential concern for more vulnerable targets such as children and domestic animals that are exposed to such lakes.
In addition to providing quantitative data for the targeted cyanotoxins, the on-line SPE-UHPLC-HRMS method can be useful in the retrospective analysis of extracted full scan MS chromatograms (Section 2.4). The acquired data can be revisited to screen previously reported cyanotoxins not currently included in monitoring programs.17 A summary of the tentative candidates qualitatively identified in samples from the present survey is provided in Table 5. A semi-quantification was performed using MC-LR as the reference compound, except for [Gly1, Asp3, ADMAdda5, Dhb7]MC-RR for which MC-RR was used (see also ESI Table S2† for detailed concentrations per sample). [M(O)1, Glu(OCH3)6]MC-LR was detected with an exact mass accuracy of −2.3 ppm and a retention time of 3.49 min in the sample from Lac Memphrémagog. The latter value is in reasonable agreement with the retention time of MC-LR in the same sample (3.55 min), and the observed monoisotopic profile of the pseudomolecular ion (ESI Fig. S2†) is also in agreement with the elemental composition. The MC variant was previously reported in the discovery paper of Qi et al. (2015) and is also included in the list of 246 microcystins published by Meriluoto et al. (2017).17,57 The fact that [M(O)1, Glu(OCH3)6]MC-LR was detected at a relatively high level (∼7500 ng L−1) in the particular sample where MC-LR also displayed the highest concentration may provide additional confidence, although unambiguous confirmation was not attained here and would require high-resolution MS/MS experiments on sufficiently concentrated extracts.17,57
Table 5 Qualitatively identified microcystins in samples from the present survey, including retention time (RT, min), theoretical and observed exact m/z and corresponding mass accuracy (δ (ppm)), occurrence frequency in lake samples (n out of 16), and semi-quantifieda concentration levels (ng L−1)
Tentative candidate |
RT (min) |
Theoretical m/z |
Observed m/z |
δ (ppm) |
Occurrence |
Semi-quantified levels (ng L−1) |
MC-LR was used as the reference compound for semi-quantification, except for [Gly1, Asp3, ADMAdda5, Dhb7]MC-RR for which MC-RR was used.
Or other isomers (Meriluoto et al. 2017).17
|
[M(O)1, Glu(OMe)6]MC-LR |
3.49 |
1085.5700 |
1085.5675 |
−2.30 |
3/16 |
ND-7500 |
[ADMAdda5, Dha7]MC-LRb |
3.46 |
1009.5353 |
1009.5364 |
1.07 |
2/16 |
ND-1700 |
MC-HphHty |
3.46 |
1064.5339 |
1064.5314 |
−2.38 |
2/16 |
ND-1500 |
Two less studied cyanopeptides were also monitored in the present study. Anabaenopeptins AP-A and AP-B were detected at relatively high concentrations in Lac Roxton and Lac Memphrémagog (at 1160 and 8280–10
000 ng L−1, respectively). AP-A was detected in three lake samples and AP-B in six lake samples; overall, 38% of the surveyed samples contained one or both congeners. AP-A is reported to show a toxicity similar to that of MCs and AP-B has the potential to induce cell lysis, thus increasing the bioavailability of cell-bound cyanotoxins. APs are not yet included in cyanotoxin monitoring programs, but some studies showed their systematic presence in toxic cyanobacterial blooms when MCs are observed.10,13,58 About 96 AP congeners are known to date and little information is available on their occurrence and impact on public health and ecosystems. In light of these results, it is also likely that other AP congeners are regularly present in algal blooms. More in-depth characterization studies could help underline the most abundant congeners present in contaminated waters following HABs, which would be useful for improved management and health safety of drinking water sources.
4. Conclusions
A simple and high-throughput methodology based on on-line SPE-UHPLC-HRMS has been developed for the analysis of 17 multi-class cyanotoxins in lake water. Two high-resolution scan modes, FS and PRM, were combined into a single acquisition method to improve the quantification of difficult-to-measure compounds. HRMS detection presented the further advantage of resolving ANA-a from its isobaric interference Phe. Suitable method performance was obtained, with low relative matrix effects and suitable accuracy and precision. This is a fast (8 min), high-throughput, and robust method that includes, to our knowledge, the widest scope of cyanotoxins in a single automated workflow. Method detection limits in the range of 8–53 ng L−1 were obtained, which is relevant for environmental analysis of field samples. The applicability of the method was verified on a range of lake samples collected in the summer 2018 through a citizen-science collaborative project (Adopt a Lake campaign). Cyanotoxins were found in sixteen samples with individual concentrations varying between 9 and 10
000 ng L−1. ANA-a was found in more than half of the samples albeit at low concentrations (compliant with guidelines), which suggests a high occurrence of this toxin and its producers. Two particular samples, Réservoir Choinière and Lac Memphrémagog, contained summed microcystin levels higher than proposed recommendations for MC-LR equivalents by the WHO for drinking water (1 μg L−1) but lower than the Canadian guidance level for recreational waters (20 μg L−1), or the threshold proposed by the USEPA for recreational use (8 μg L−1). A retrospective analysis of extracted full scan MS chromatograms suggested the presence of some additional MC variants, at semi quantified concentrations in the μg L−1 range in the sample from Lac Memphrémagog. In addition, about 38% of the samples contained either anabaenopeptins AP-A, AP-B, or both, with concentrations of up to 10
000 ng L−1, higher than the reported MCs. Little information is available about the environmental fate and effects on public health of these infrequently monitored cyanopeptides. In view of the systematic co-occurrence of APs and MC congeners in some of these samples, complementary research is needed to characterize their toxicological properties (individually and from mixtures with known compounds) and their occurrence at a broader spatial scale and properly evaluate their associated risks.
Conflicts of interest
There are no conflicts of interest to declare.
Acknowledgements
This work was made possible through the financial support from Génome Québec and Genome Canada to the ATRAPP (Algal Blooms, Treatment, Risk Assessment, Prediction and Prevention Through Genomics) project. The authors also thank B. Husk (BlueLeaf), the citizen contributors and volunteers of Adopt a Lake for their help in the sampling campaign, and T. Andriananjamanantsoa for the 16S rRNA analysis.
References
-
W. Carmichael, in Cyanobacterial harmful algal blooms: state of the science and research needs, Springer, 2008, pp. 105–125 Search PubMed.
- S. Merel, D. Walker, R. Chicana, S. Snyder, E. Baurès and O. Thomas, Environ. Int., 2013, 59, 303–327 CrossRef CAS PubMed.
- J. O'Neil, T. Davis, M. Burford and C. Gobler, Harmful Algae, 2012, 14, 313–334 CrossRef.
- X. Yan, X. Xu, M. Wang, G. Wang, S. Wu, Z. Li, H. Sun, A. Shi and Y. Yang, Water Res., 2017, 125, 449–457 CrossRef CAS PubMed.
- B. Moss, S. Kosten, M. Meerhoff, R. W. Battarbee, E. Jeppesen, N. Mazzeo, K. Havens, G. Lacerot, Z. Liu and L. De Meester, Inland Waters, 2011, 1, 101–105 CrossRef.
- M. Y. Cheung, S. Liang and J. Lee, J. Microbiol., 2013, 51, 1–10 CrossRef CAS PubMed.
- R. Wood, Environ. Int., 2016, 91, 276–282 CrossRef CAS PubMed.
- C. C. Carey, B. W. Ibelings, E. P. Hoffmann, D. P. Hamilton and J. D. Brookes, Water Res., 2012, 46, 1394–1407 CrossRef CAS PubMed.
- H. W. Paerl and V. J. Paul, Water Res., 2012, 46, 1349–1363 CrossRef CAS PubMed.
- E. M.-L. Janssen, Water Res., 2019, 151, 488–499 CrossRef CAS PubMed.
- Z. Svirčev, D. Drobac, N. Tokodi, B. Mijović, G. A. Codd and J. Meriluoto, Arch. Toxicol., 2017, 91, 621–650 CrossRef PubMed.
- A. Campos and V. Vasconcelos, Int. J. Mol. Sci., 2010, 11, 268–287 CrossRef CAS PubMed.
- L. Beversdorf, C. Weirich, S. Bartlett and T. Miller, Toxins, 2017, 9, 62 CrossRef PubMed.
- L. Spoof, A. Błaszczyk, J. Meriluoto, M. Cegłowska and H. Mazur-Marzec, Mar. Drugs, 2016, 14, 8 CrossRef PubMed.
- B. Sedmak, S. Carmeli and T. Eleršek, Microb. Ecol., 2008, 56, 201–209 CrossRef CAS PubMed.
- M. Welker and H. Von Döhren, FEMS Microbiol. Rev., 2006, 30, 530–563 CrossRef CAS PubMed.
-
J. Meriluoto, L. Spoof and G. A. Codd, Handbook of cyanobacterial monitoring and cyanotoxin analysis, John Wiley & Sons, 2017 Search PubMed.
- K. Bouma-Gregson, R. M. Kudela and M. E. Power, PLoS One, 2018, 13, e0197669 CrossRef PubMed.
- E. Mantzouki, M. Lürling, J. Fastner, L. de Senerpont Domis, E. Wilk-Woźniak, J. Koreivienė, L. Seelen, S. Teurlincx, Y. Verstijnen and W. Krztoń, Toxins, 2018, 10, 156 CrossRef PubMed.
-
M. Bruno, O. Ploux, J. S. Metcalf, A. Mejean, B. Pawlik-Skowronska and A. Furey, in Handbook of Cyanobacterial Monitoring and Cyanotoxin Analysis, Wiley & Sons, 2016 Search PubMed.
- J. Fawell, R. Mitchell, R. Hill and D. Everett, Hum. Exp. Toxicol., 1999, 18, 168–173 CrossRef CAS PubMed.
- J. T. Antunes, P. N. Leão and V. M. Vasconcelos, Front. Microbiol., 2015, 6, 473 Search PubMed.
- M. A. Burford, J. Beardall, A. Willis, P. T. Orr, V. F. Magalhaes, L. M. Rangel, S. M. Azevedo and B. A. Neilan, Harmful Algae, 2016, 54, 44–53 CrossRef PubMed.
- I. R. Falconer and A. R. Humpage, Environ. Toxicol., 2006, 21, 299–304 CrossRef CAS PubMed.
-
World Health Organization, Guidelines for drinking-water quality, World Health Organization, Geneva, 2017 Search PubMed.
- A. Zamyadi, L. Ho, G. Newcombe, H. Bustamante and M. Prévost, Water Res., 2012, 46, 1524–1535 CrossRef CAS PubMed.
-
A. Soltani, P. Hess, M. B. Dixon, S. F. Boerlage, D. M. Anderson, G. Newcombe, J. House, L. Ho, P. Baker and M. Burch, World Health Organization and International Guidelines for toxin control, Harmful Algal Boom management, and response planning, Intergovernmental Oceanographic Commission of UNESCO, Paris, 2017 Search PubMed.
-
U. S. EPA, Guidelines and Recommendations, https://www.epa.gov/nutrient-policy-data/guidelines-and-recommendations#what2, accessed February 2nd, 2019 Search PubMed.
-
U. S. EPA, Drinking water health advisories for two cyanobacterial toxins, 2015 Search PubMed.
-
U. S. EPA, Contaminant candidate list (CCL) and regulatory determination-Contaminant candidate list 3-CCLvol. 3, 2018 Search PubMed.
-
R. Baum, J. Bartram and S. Hrudey, in The Flint water crisis confirms that US drinking water needs improved risk management. ACS Publications, 2016 Search PubMed.
-
Gouvernement du Québec, Règlement sur la qualité de l’eau potable. Loi sur la qualité de l’environnement. Q-2, r. 40, 2017 Search PubMed.
- C. Wagner and R. Adrian, Limnol. Oceanogr., 2009, 54, 2460–2468 CrossRef.
- B. Greer, S. E. McNamee, B. Boots, L. Cimarelli, D. Guillebault, K. Helmi, S. Marcheggiani, S. Panaiotov, U. Breitenbach and R. Akçaalan, Harmful Algae, 2016, 55, 31–40 CrossRef CAS PubMed.
- D. S. Palagama, R. E. West III and D. Isailovic, Anal. Methods, 2017, 9, 2021–2030 RSC.
- S.-K. Zervou, C. Christophoridis, T. Kaloudis, T. M. Triantis and A. Hiskia, J. Hazard. Mater., 2017, 323, 56–66 CrossRef CAS PubMed.
- E. Beltrán, M. Ibáñez, J. V. Sancho and F. Hernández, J. Chromatogr. A, 2012, 1266, 61–68 CrossRef PubMed.
- P. B. Fayad, A. Roy-Lachapelle, S. V. Duy, M. Prévost and S. Sauvé, Toxicon, 2015, 108, 167–175 CrossRef CAS PubMed.
- L. Balest, S. Murgolo, L. Sciancalepore, P. Montemurro, P. P. Abis, C. Pastore and G. Mascolo, Anal. Bioanal. Chem., 2016, 408, 4063–4071 CrossRef CAS PubMed.
-
J. Choi, J.-H. Jang, J. Massi and J. Beck, Fast and Accurate Determination of Algal Toxins in Water Using Online Preconcentration and UHPLC-Orbitrap Mass Spectrometry, 2019, accessed October 2nd, http://https://tools.thermofisher.com/content/sfs/brochures/AN-64186-LC-MS-Microcystin-Nodularin-Drinking-Water-AN64186-EN-HR.pdf Search PubMed.
- X. Ortiz, E. Korenkova, K. J. Jobst, K. A. MacPherson and E. J. Reiner, Anal. Bioanal. Chem., 2017, 409, 4959–4969 CrossRef CAS PubMed.
- J. Birbeck, J. Westrick, G. O'Neill, B. Spies and D. Szlag, Toxins, 2019, 11, 13 CrossRef CAS PubMed.
- G. Munoz, S. Vo Duy, A. Roy-Lachapelle, B. Husk and S. Sauvé, J. Chromatogr. A, 2017, 1516, 9–20 CrossRef CAS PubMed.
- C. Flores and J. Caixach, J. Chromatogr. A, 2015, 1407, 76–89 CrossRef CAS PubMed.
- A. Roy-Lachapelle, M. Solliec, M. Sinotte, C. Deblois and S. Sauvé, Talanta, 2015, 132, 836–844 CrossRef CAS PubMed.
-
Faculté des arts et des sciences, Adopt a lake, https://fas.umontreal.ca/adopte-un-lac/adopt-a-lake/, accessed May 16th, 2019 Search PubMed.
- G. Munoz, S. V. Duy, A. Roy-Lachapelle, B. Husk and S. Sauvé, J. Chromatogr. A, 2017, 1516, 9–20 CrossRef CAS PubMed.
- M. Solliec, A. Roy-Lachapelle and S. Sauvé, Anal. Chim. Acta, 2015, 853, 415–424 CrossRef CAS PubMed.
- L. Kamp, J. L. Church, J. Carpino, E. Faltin-Mara and F. Rubio, Chem.-Biol. Interact., 2016, 246, 45–51 CrossRef CAS PubMed.
- J. Mankiewicz-Boczek, J. Palus, I. Gągała, K. Izydorczyk, T. Jurczak, E. Dziubałtowska, M. Stępnik, J. Arkusz, M. Komorowska and A. Skowron, Harmful Algae, 2011, 10, 356–365 CrossRef CAS.
- A. A. de la Cruz, A. Hiskia, T. Kaloudis, N. Chernoff, D. Hill, M. G. Antoniou, X. X. He, K. Loftin, K. O'Shea, C. Zhao, M. Pelaez, C. Han, T. J. Lynch and D. D. Dionysiou, Environ. Sci.: Processes Impacts, 2013, 15, 1979–2003 RSC.
- L. Pearson, T. Mihali, M. Moffitt, R. Kellmann and B. Neilan, Mar. Drugs, 2010, 8, 1650–1680 CrossRef CAS PubMed.
- J. K. Fawell, R. E. Mitchell, R. E. Hill and D. J. Everett, Hum. Exp. Toxicol., 1999, 18, 168–173 CrossRef CAS PubMed.
- M. Kellmann, H. Muenster, P. Zomer and H. Mol, J. Am. Soc. Mass Spectrom., 2009, 20, 1464–1476 CrossRef CAS.
- C. L. Chitescu, G. Kaklamanos, A. I. Nicolau and A. A. M. L. Stolker, Sci. Total Environ., 2015, 532, 501–511 CrossRef CAS PubMed.
- S. Mejia-Avendaño, G. Munoz, S. Vo Duy, M. l. Desrosiers, P. Benoît, S. b. Sauvé and J. Liu, Environ. Sci. Technol., 2017, 51, 8313–8323 CrossRef PubMed.
- Y. Qi, L. Rosso, D. Sedan, L. Giannuzzi, D. Andrinolo and D. A. Volmer, Rapid Commun. Mass Spectrom., 2015, 29, 220–224 CrossRef CAS PubMed.
- S. Gkelis, T. Lanaras and K. Sivonen, Mar. Drugs, 2015, 13, 6319–6335 CrossRef CAS PubMed.
Footnote |
† Electronic supplementary information (ESI) available. See DOI: 10.1039/c9ay01132c |
|
This journal is © The Royal Society of Chemistry 2019 |
Click here to see how this site uses Cookies. View our privacy policy here.