DOI:
10.1039/C8AY02472C
(Critical Review)
Anal. Methods, 2019,
11, 247-261
Review: new insights into optimizing chemical and 3D surface structures of carbon electrodes for neurotransmitter detection
Received
12th November 2018
, Accepted 18th December 2018
First published on 21st December 2018
Abstract
The carbon-fiber microelectrode has been used for decades as a neurotransmitter sensor. Recently, new strategies have been developed for making carbon electrodes, including using carbon nanomaterials or pyrolyzing a photoresist etched by nanolithography or 3D printing. This review summarizes how chemical and 3D surface structures of new carbon electrodes are optimized for neurotransmitter detection. There are effects of the chemical structure that are advantageous, and nanomaterials are used ranging from carbon nanotubes (CNTs) to graphene to nanodiamond. Functionalization of these materials promotes surface oxide groups that adsorb dopamine, and dopants introduce defect sites good for electron transfer. Polymer coatings such as poly(3,4-ethylenedioxythiophene) (PEDOT) or Nafion also enhance the selectivity, particularly for dopamine over ascorbic acid. Changing the 3D surface structure of an electrode increases the current by adding more surface area. If the surface structure has roughness or pores on the micron scale, the electrode also acts as a thin layer cell, momentarily trapping the analyte for redox cycling. Vertically aligned CNTs as well as lithographically made or 3D printed pillar arrays act as thin layer cells, producing more reversible cyclic voltammograms. A better understanding of how the chemical and surface structure affects the electrochemistry enables rational design of electrodes. New carbon electrodes are being tested in vivo and strategies to reduce biofouling are being developed. Future studies should test the robustness for long term implantation, explore electrochemical properties of neurotransmitters beyond dopamine, and combine optimized chemical and physical structures for real-time monitoring of neurotransmitters.
1. Introduction
What is the perfect electrode material for in vivo electrochemical monitoring of neurotransmitters? For direct voltammetric measurements of catecholamines such as dopamine, decades of experience suggests it is carbon.1 Common carbon electrodes include glassy carbon electrodes (GCEs), carbon paste electrodes (CPEs), and screen-printed carbon electrodes (SPCEs). The most popular electrode for neurochemical measurements has been the carbon-fiber microelectrode (CFME) because of its excellent biocompatibility, small size, and good electron transfer for neurotransmitters.2 Carbon fibers are made of carbon precursors that have different orientations of carbon.2 Dopamine, the prototypical neurotransmitter studied, adsorbs to CFMEs, primarily to defect sites and oxygen-containing functional groups.3 This adsorption preconcentrates dopamine on the surface and facilitates rapid electron transfer. Fast-scan cyclic voltammetry (FSCV) is often used for real-time in vivo detection of neurotransmitters and works well at CFMEs.4,5 While carbon fibers are an excellent sensor for neurotransmitters, they have a relatively smooth surface which limits surface roughness and area,6 and the geometries of probes are limited to disk, cylindrical, or conical shapes.7
New fabrication methods for carbon electrodes allow electrodes with customizable geometries as well as optimized chemical and surface structures. Many forms of carbon nanomaterials have been used for neurotransmitter electrodes because they promote dopamine adsorption and provide a rougher surface structure without physically increasing the size of the electrode. In addition, carbon nanomaterials have apparent electrocatalytic effects due to their electronic structure.8 Research started with carbon nanotubes (CNTs), but the field has now ballooned to many different forms of graphene.8 In addition, carbon nanostructures are now made by nanolithography and 3D printing techniques.9,10 Structures are made from photoresists and then pyrolyzed to form carbon, resulting in customized geometries such as arrays.
While most research uses new carbon electrodes to increase sensitivity or to exploit their electrocatalytic effects, there are other advantages of carbon electrodes that are emerging from the literature. First, there are effects of the chemical structure that are advantageous, such as functionalization methods to add oxide groups to promote adsorption, dopants that introduce defect sites good for electron transfer, and polymer coatings combined with nanomaterials that increase selectivity. Second, there are effects of 3D surface structure that go beyond increasing the active surface area. Array-like structures are used as collector–generators and structures with pores, cavities, or long arrays act as thin layer cells to momentarily trap dopamine. This review concentrates on these advantageous chemical and structural properties, overviewing the methods to fabricate and optimize structures and how they affect the electrochemistry of neurotransmitters. The last section outlines future challenges of how this knowledge could lead to rational design of better carbon electrodes, with a focus on biological applications. The knowledge gained from fundamental electrochemical studies will lead to better, practical sensors for in vivo neurotransmitter sensing.
2. Effects of the chemical structure of carbon electrodes
Most studies exploring the effects of the chemical structure of carbon for neurotransmitter detection have aimed to increase the adsorption of neurotransmitters and promote electrocatalytic effects. This section outlines the different carbon allotropes, functionalization methods, and polymer coatings used to modify the chemical structure of carbon electrodes and reviews how these enhance electrochemical properties for neurotransmitter detection.
2.1 Carbon allotropes
2.1.1 Structure and properties of carbon electrode materials.
There are two planes of graphitic carbon when it is stacked: a basal plane parallel to the graphite sheet, and an edge plane at the edge of the graphite layers that is perpendicular to the graphite sheet (Fig. 1A).11 The edge plane is traditionally considered to have more electronic density of states and better electron transfer for surface sensitive probes (Fig. 1B).1,11,12 The amount of defects is measured from the D/G ratio by Raman spectroscopy, where a higher ratio of the graphitic D peak area to the graphitic G peak area indicates more defects and edge plane sites in the structure.1 Some recent publications have challenged the traditional ideas about the activity of edge vs. basal planes. Using scanning electrochemical cell microscopy (SECCM) to probe localized electrochemical activities, Unwin's group showed higher activity for neurotransmitter oxidation at the basal plane and no correlation between edge planes and adsorption.13 For CFMEs, electrochemical oxidation of the electrode surface enhanced dopamine anodic current and electron transfer kinetics, but decreased the Raman D/G ratio (Fig. 1C).14 These studies are in contrast to decades of work by McCreery, Compton, and others that show edge planes have faster electron transfer and better sensitivity for dopamine.1,15,16 There is still much work to be done to examine neurotransmitter reactivity at different carbon planes. However, most studies concentrate on making defect-rich electrodes.
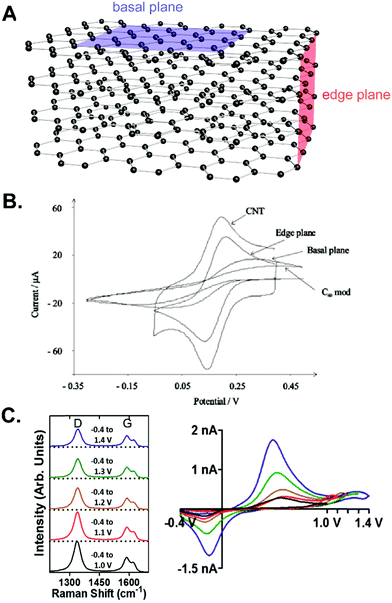 |
| Fig. 1 Electrochemistry at the edge plane and basal plane of graphitic carbon electrodes. (A) Schematic diagram of the edge and basal plane from a stack of graphene layers. (B) CVs of 1 mM [Fe(CN)6]3− at different carbon electrodes show the better electrocatalytic effects of the edge plane than the basal plane. Reproduced from ref. 15 with permission from The Royal Society of Chemistry. (C) Raman spectra and FSCVs of 2 μM dopamine at CFME with different switching potentials from +1.0 to +1.4 V. The extended waveform enhanced the dopamine signal but decreased the D/G ratio. Adapted with permission from ref. 14. Copyright 2017 American Chemical Society. | |
2.1.2 sp2-hybridized carbon nanomaterials.
sp2-hybridized carbon nanomaterials are the largest family of carbon nanomaterials for electrochemistry.17 These nanomaterials have extended conjugation, electron delocalization, and high electronic conductivity. CNTs are one of the most investigated nanomaterials for microelectrode fabrication and they enhance the electrochemical signals for dopamine and ascorbic acid, a common interferent found in the brain.8 CNT-modified CFMEs have been prepared by dip coating,18 electrophoretic deposition,19 and direct growth of CNTs.6 CNT ends have a high density of edge planes that strongly enhance dopamine adsorption in FSCV. Thus, CNTs are often fabricated in arrays, either by self-assembly20 or direct growth with chemical vapor deposition (CVD)6 to control the CNT orientation (Fig. 2A). Another method to prepare aligned CNT microelectrodes is to use a CNT fiber or yarn.21–23 Both CNT yarns and CNT fibers had better electron transfer kinetics and analytical performance for FSCV detection of cationic neurotransmitters.21
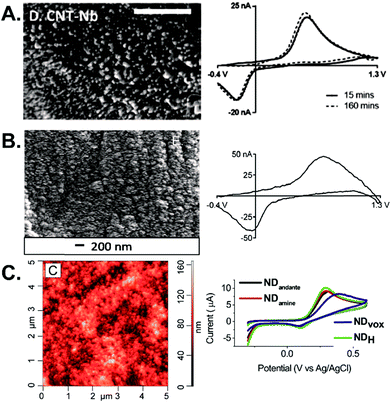 |
| Fig. 2 Carbon nanomaterial electrodes. (A) SEM image and FSCV of 1 μM dopamine at CNTs grown on Nb wires after 15 min (solid) and 160 min (dashed) of equilibration. Scale bar: 500 nm. Adapted with permission from ref. 6. Copyright 2016 American Chemical Society. (B) SEM image and FSCV of 1 μM dopamine at CNSs grown on Nb wires. Reproduced from ref. 28 with permission from The Royal Society of Chemistry. (C) AFM image of ND-coated taC electrodes and CVs of 1 mM dopamine at different types of nanodiamond. Reprinted from ref. 37 with permission from Elsevier. | |
Graphene is a sheet of sp2 carbon and has high conductivity from its extended conjugated system.17 However, a perfect sheet is mainly the basal plane without defect sites and has limited adsorption capability, so graphene oxide is often used.1 Graphene oxide synthesized via the Hummers method was dropcast on GCE to increase dopamine anodic current because of the increased conductivity and π–π stacking interaction.24 Graphene-modified CFMEs were utilized in mice hippocampal tissue to improve the current for dopamine detection.25 Working with a single layer of graphene is difficult, so electrochemical sensors are usually built out of 3D forms of graphene. 3D graphene was fabricated on an indium tin oxide planar substrate by direct CO2 laser writing of spin-coated polyimide, and had good electrochemical activity toward surface sensitive probes.26 3D graphene electrodes can also be synthesized via electrospray deposition on structured substrates.27 Carbon nanospikes (CNSs) are a form of graphene that have a spike morphology and are grown without a catalyst on metal wires by plasma-enhanced CVD, thus there is no potential for metal contamination. CNS microelectrodes are defect-rich and contain many oxides, so dopamine adsorption is enhanced (Fig. 2B).28 Carbon nanohorns (CNHs) are graphene sheets rolled in a conical structure. CNHs are synthesized via laser ablation of graphite without a metal catalyst, so they have high purity and less toxicity from metals.17 CNH deposition increased the dopamine signal at a screen-printed carbon electrode.29 CNH-modified CFMEs had high dopamine adsorption due to a high surface area and enhanced electric field at the CNH tip, and could be oxidatively etched, improving the LOD for dopamine.30
2.1.3 sp3-hybridized carbon nanomaterials.
Diamond, the sp3-hybridized carbon allotrope, is an electrical insulator. However, thin film and nanostructure fabrication, as well as doping, give it conductivity and electrochemical activity.31 For example, boron-doped diamond (BDD) electrodes have been widely used because of their stability and wide potential window.31,32 Nanodiamond (ND), a nanoparticle diamond, is synthesized via detonation of explosive carbon materials33 and has an sp3-hybridized carbon core with defects including sp2 carbons and functional groups on its surface.34 A thin film of ND has better electron transfer kinetics and lower overpotential than BDD for several redox probes,35,36 and a ND-modified electrode improved the electrocatalytic effect and limit of detection for dopamine detection (Fig. 2C).37 ND particles on the electrode surface also improve cell viability, demonstrating the potential of the electrode to be used long-term without damaging the cells.37 Tetrahedral amorphous carbon (taC) is an amorphous sp3-hybridized carbon structure with no crystallinity. It is easy to synthesize via ion deposition, sputtering, or cathodic vacuum arc,38 which makes it compatible with nanofabrication. taC was fabricated as a thin film on a silicon substrate and had a wide window potential (−1.6 to +1.7 V vs. Ag/AgCl). The oxygen content correlated with the faradaic current of the electroactive species,39 and the material was responsive to dopamine.40 Carbon quantum dots, or carbon dots, are spherical nanoparticles consisting of an sp3-carbon amorphous network.17 Carbon dots are synthesized by several methods, such as electrochemical exfoliation of graphite rods in basic solution41 or hydrothermal treatment of citrate aqueous solution.42 They are a promising nanomaterial for neurotransmitter detection because of their high surface area and high oxygen content. GCEs, SPCEs, or CFMEs modified by carbon dots had improved sensitivity and electrocatalytic properties toward dopamine detection.41,42 More work on sp3-hybridized graphene is needed to evaluate its performance toward neurotransmitter sensing in vivo.
2.2 Chemical functionalization and doping
2.2.1 Functionalization.
Many strategies have been used to introduce surface groups on the carbon electrode surface, particularly surface oxide groups which are known to adsorb dopamine. Chemical treatments can increase oxides, such as NaOH soaking, or the carbon surface functionalized via diazonium ion reduction, thermal reactions, photochemical reactions, or click chemistry.1 Electrochemical treatment, by using a waveform with an extended anodic limit, is also used to increase the oxygen content.43 For carbon nanomaterials, there are different strategies, such as acid pretreatment or electrophilic addition.17 The electrochemical properties of different functionalized CNTs were compared, and carboxylated and amide functionalized CNTs had better dopamine anodic current while functionalization with a long chain alkane, octadecylamine, caused low conductivity and steric hindrance for analyte adsorption.44 For graphene, functionalization with carboxylic acid and sulfonate aryl groups enhanced the dopamine anodic current and decreased the ascorbic acid anodic current. However, the steric hindrance from these aryl groups limited the access to the graphene electroactive area.45 Surface treatments also change the oxide functionalization; for example, oxygen plasma etching or laser treatment of CNT yarns increased the oxygen content.46,47 Too much functionalization on the sp2-hybridized carbon nanomaterials disrupts the conjugation and decreases the conductivity of the electrode.48 Therefore, the extent of functionalization and orientation of groups should be carefully controlled.
2.2.2 Doping.
Doping is the introduction of defects or impurities in materials to decrease the band gap energy and increase their conductivity. In electrochemistry, doping an ordered material increases the density of electronic states and enhances its electrochemical activity.49 Doping has been applied to carbon materials, both electrical insulators such as diamond and conducting materials such as graphene or CNTs, to enhance their properties for electrochemical sensing.1,49
Boron is electron-deficient compared to carbon and is used to dope diamond and enhance its electrochemical activity.50 A BDD microfiber was fabricated and used with FSCV for dopamine detection. The electrode has a wide potential window and low noise, but the FSCV signal is small, even for high dopamine concentrations such as 20 μM.51 The Lee group demonstrated that BDD electrodes are favorable for human studies, showing that they had less fouling than CFMEs.32 Thus, BDD exhibits antifouling properties similar to other sp3-hybridized carbon nanomaterials.32,52 For CNTs, boron-doped CNTs had a higher D/G ratio, increased peak current, and enhanced electrocatalytic properties. However, too much boron destroyed CNT conductivity and the overall signal.53 Boron-doped graphene also catalyzed dopamine oxidation on GCE.54
Nitrogen doping also improves material conductivity and electrochemistry. Nitrogen-doped carbon materials are synthesized by CVD with nitrogen-containing acetonitrile or ammonia,55 or are made by pyrolyzing nitrogen-based polymer fibers such as polypyrrole.56 Nitrogen atoms substitute for a carbon atom in the carbon layer, creating a defect. A computational model, along with dopamine cyclic voltammetry, revealed that adding some nitrogen enhanced the dopamine adsorption by stabilizing the surface energy, but too much nitrogen doping disrupts the conductivity and adsorption by disrupting dopamine pi stacking with the basal plane.57,58 Nitrogen-doped graphene fibers enhanced dopamine sensitivity 10 fold over pristine fibers.56 Doping CNT fibers with nitrogen also displayed good ascorbic acid detection,59 and doping a three-dimensional graphene porous electrode improved the sensitivity for H2O2 detection.60
2.3 Polymer coatings
2.3.1 Charged polymers.
Charged polymers have ionizable groups that attract oppositely charged molecules and repel similarly charged molecules via electrostatic interactions; thus, they are used as electrode materials to introduce selectivity. Nafion is a negatively charged, perfluorosulfonate polymer that has been extensively used in neurotransmitter applications to enhance the signal for cationic neurotransmitters. Nafion is drop-cast or electrodeposited to increase the sensitivity toward dopamine and eliminate the sensitivity to anionic interferents such as ascorbate,61 DOPAC,61 or 5-HIAA, a serotonin metabolite.62 However, polymer coatings slow down diffusion and response times,63,64 which convolutes the temporal response for in vivo measurements. Nafion has been combined with carbon nanomaterials to increase the sensitivity of electrodes. For example, Nafion/CNT-modified CFMEs had enhanced dopamine signal and reduced interference from ascorbic acid.18 Nafion-CNTs enhanced adsorption and did not slow down the response time for real-time measurements of cationic neurotransmitters (Fig. 3A).64 Over-oxidized polypyrrole (oPPy) is another polymer that has a negative charge and is selective toward cationic molecules.63,65 CFMEs have been coated with oPPy to increase the selectivity for dopamine, and incorporating carbon nanotubes further improves the sensitivity of the signal.64 In addition, oPPy coatings on interdigitated gold electrode arrays allowed amperometric measurements of dopamine release from PC12 cells without interference.66 oPPy was also coated on a BDD electrode and eliminated anionic interferences as well.67
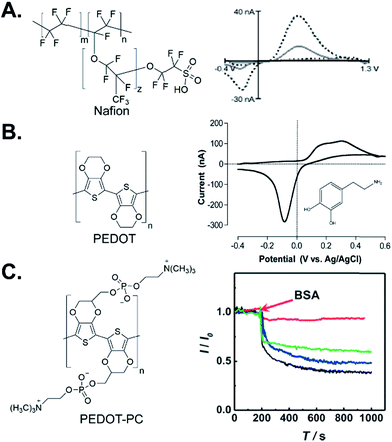 |
| Fig. 3 Polymer-coated electrodes. (A) Structure of Nafion and FSCV of 1 μM dopamine at Nafion/CNTs (dashed) compared to unmodified CFME (solid). Reproduced from ref. 64 with permission from The Royal Society of Chemistry. (B) Structure of PEDOT and FSCV of 100 μM dopamine at a PEDOT:tosylate-modified Au microelectrode chip. Adapted with permission from ref. 72. Copyright 2017 American Chemical Society. (C) Structure of PEDOT-PC and amperometric response toward 20 μM dopamine with 10 mg mL−1 injection of BSA at PEDOT-PC/CFME (red), CFME (black), PEDOT/CFME (blue), and PEDOT-OH/CFME (green). Adapted from ref. 80 with permission from Wiley. | |
2.3.2 Conducting polymers.
Conducting polymers have electronic properties similar to those of metals or semiconductors. Their conjugated structure, alternating single and double bonds, cause the electron delocalization in the structure and hence electronic conductivity.68 Conducting polymers are also inexpensive,69 have good flexibility, and are biocompatible.70 Therefore, they are fabricated as electrode materials themselves without a metal or carbon fiber support, or they are deposited on CFMEs to enhance the signal and sensitivity for neurotransmitter measurements.
Poly(3,4-ethylenedioxythiophene) (PEDOT) is one of the most popular conducting polymers used for electrochemical sensors. PEDOT was electropolymerized on a Pt or Au microelectrode chip, and the electrode had good peak resolution for dopamine, ascorbic acid, and uric acid detection.71 A composite PEDOT:tosylate film microelectrode chip was fabricated to be compatible with microfluidic systems. The film had a fast response toward dopamine and low background current with FSCV, but further studies could improve the sensitivity (Fig. 3B).72 PEDOT is combined with nanomaterials, such as graphene oxide, to increase the surface area and electrocatalytic properties for dopamine. However, the thick coating of PEDOT/graphene oxide slowed down the response and clearance time.73 PEDOT/CNT was electrodeposited on Au microwires and doubled the sensitivity of just PEDOT coating.74 Polypyrrole (PPy) is another conducting polymer and PPy-modified gold microelectrode arrays increased the current for ascorbic acid and decreased its oxidation overpotential.75 PPy derivatives such as poly(N-methylpyrrole) also improved the electrochemical detection of dopamine at the glassy carbon electrode76 and polyaniline polymers, particularly when gold nanoparticles or graphene oxide are included, also increase the signal for dopamine.77,78
Conducting polymers can be functionalized as well to enhance their chemical properties. Carboxylated PEDOT adsorbed more dopamine than unmodified PEDOT and had higher sensitivity and selectivity over ascorbic acid than other functional groups.79 Zwitterionic functional groups containing separate positive and negative charges increase the hydrophilicity of the electrode surface to eliminate protein and biofilm adsorption.80 The Mao group synthesized PEDOT-phosphorylcholine (PEDOT-PC) polymer on a CFME surface to prevent protein adsorption and decrease biofouling (Fig. 3C).80 While traditionally signals drop by 50% after tissue implantation, the PEDOT-PC electrode did not lose current after implantation in the rat brain for 2 hours. However, its sensitivity should be increased to make it more effective in vivo. A similar antifouling effect also was observed at PEDOT-PC-coated gold to incorporate Nafion into the PEDOT structure.81 PEDOT:Nafion-modified CFMEs had higher sensitivity and selectivity towards cationic dopamine than anionic DOPAC and ascorbic acid, and the coating prevented protein and biomolecule biofouling.81
2.4 Effect of the chemical structure of carbon on electrochemistry
2.4.1 Chemical structure modifications promote adsorption and enhance sensitivity.
In FSCV, the popular technique for measuring neurotransmitters in vivo, the dopamine redox reaction is adsorption-controlled via electrostatic attraction between cationic dopamine and negative oxide groups on CFMEs.3,4 Enhancing adsorption solely by increasing the surface area is not always effective because noise is proportional to capacitance, which also increases with surface area. Instead, specifically adding defect sites for adsorption or making the electrode charge more negative can improve the sensitivity and limit of detection. Using edge-plane rich carbon nanomaterials such as CNTs and CNSs to modify a microelectrode enhances dopamine adsorption.1,6,20,28 Chemical treatment such as oxidative etching of carbon nanomaterials,30,82 and treatments such as laser,47 oxygen plasma etching and antistatic gun treatment46 also generate defects and surface oxide groups to promote adsorption. Chemical functionalization of negatively charged functional groups, such as carboxylate and sulfonate groups, enhances dopamine adsorption as well.44,45,79 Overall, most studies find that a balance of edge plane and oxide sites increases adsorption, although too much of either can decrease the conductivity of the material. Table 1 summarizes the advantages and limitations of the different chemical structures of carbon electrodes.
Table 1 Summary of electrode modification to enhance the chemical structural effects for neurotransmitter detection
Modification |
Goals |
Advantages |
Limitations |
Ref. |
sp2-hybridized carbon nanomaterials |
Enhance surface area, adsorption, sensitivity, limit of detection |
Some nanomaterials are commercially available, several simple methods to fabricate, low or nontoxic compared to metals |
Impurities can affect properties, aggregation in solution, some nanomaterials are difficult to synthesize |
1, 6, 17, 18, 21 and 30
|
sp3-hybridized carbon nanomaterials |
Increase adsorption, improve stability, limit the background noise, prevent electrode fouling |
Biocompatible, good mechanical strength and stability |
Inherently insulators so size and surface functional groups must be carefully controlled |
31, 32 and 37
|
Functionalization |
Increase surface electron transfer properties and control selectivity |
Several strategies are available, easy to functionalize after electrode fabrication |
Extent and orientation of functional groups are hard to control |
1, 17, 43, 45, 47 and 48
|
Doping |
Increase conductivity and electrocatalytic effects |
Can combine with other nanomaterials, creates defect sites for electron transfer |
Too much doping can destroy conductivity, some doping methods require complicated fabrication, e.g. CVD |
49, 50, 53, 57 and 58
|
Charged polymers |
Control selectivity and prevent electrode fouling |
Easy fabrication, inexpensive, biocompatible, can combine with nanomaterials |
Slow temporal response, sensitivity decreased or not improved |
61, 63 and 65
|
Conducting polymers |
Fabricate alternative, non-metal electrode materials |
Easy fabrication, inexpensive, biocompatible, can functionalize and combine with nanomaterials |
Slow temporal response and sensitivity, depending on the coating |
68–70, 72 and 79
|
2.4.2 Chemical structure modifications that enhance electron transfer and cause electrocatalytic effects.
The structure of carbon also promotes electron transfer, causing electrocatalytic effects that help resolve peaks from different analytes.1,11,15 Defect-rich carbon nanomaterials such as CNTs, CNSs, and NDs exhibit electrocatalytic effects because they have more electronic density of states similar to the energy levels of electroactive neurotransmitters and thus allow faster electron transfer.1,17,34 Electrocatalytic effects are particularly pronounced with slower methods such as cyclic voltammetry (CV) and differential pulse voltammetry (DPV), but are even observed in FSCV as the distance between the peaks (ΔEp) in the CV decreases. In particular, electrocatalytic effects help separate dopamine, ascorbic acid, and uric acid using DPV.56,83 Electrocatalytic effects are helpful to discriminate electroactive species when using amperometry, especially if analytes are oxidized or reduced at similar potentials. Boron or nitrogen doping also enhances the electrocatalytic effect of carbon nanomaterial electrodes because they reduce the band gap energy of the material and enhance the conductivity and electron transfer rate.32,53,55,59
2.4.3 Chemical structure modifications that promote selectivity.
Selectivity is an important problem for in vivo detection because of the complex biological environment. The most investigated selectivity problem is the interference between dopamine and ascorbic acid. Dopamine is cationic at physiological pH, but ascorbic acid is anionic, therefore charge manipulation discriminates between these two species. Negatively charged polymers, such as Nafion and oPPy coating an electrode, repel the negatively charged ascorbate from the electrode and eliminate its interference.65,84 This strategy is also useful for preventing the adsorption of redox products and biomolecules, which causes electrode fouling.62 Unfortunately, polymer coatings usually slow down response times, so other modification strategies such as using carbon nanomaterials or atomic doping must be implemented to preserve the fast temporal resolution.64 Functionalization with carboxylate and sulfonate groups, either on the carbon surface or of a conducting polymer, also favorably attracts cationic dopamine.44,79 However, there are other challenges for selectivity, such as discriminating the catecholamines norepinephrine, dopamine, and epinephrine that cannot simply rely on charge. Future studies are needed to understand the properties of carbon nanomaterials to adsorb other cationic molecules and prevent biofouling as well.
3. Effects of 3D surface structures of carbon electrodes
While chemical properties of carbon are important for its detection of neurotransmitters, the physical 3D surface structure is also manipulated to enhance neurotransmitter electrochemistry.85,86 In this section, we focus on microscale surface morphologies from sub-micron to tens of microns, typical of array and nanomaterial surface structures. Different types of surface morphological structures are classified, their fabrication methods are discussed, and the electrochemical consequences of structural properties are reviewed. Advantages and limitations of these methods are also summarized in Table 2.
Table 2 Summary of fabrication methods for 3D surface structure electrodes
Fabrication method |
Type of structure |
Growth conditions |
Advantages |
Limitations |
Resolution |
Ref. |
Nanomaterial direct growth |
Arrays, porous, and cavity |
Depends on the nanomaterials and substrates |
Increased surface area/roughness, better chemical properties |
Geometries depend on nanomaterials themselves |
Depends on the material, ∼1–100 nm |
82 and 97
|
Direct laser writing (3D printing) |
Any geometry |
Atmosphere |
Customizable size and geometry |
Time consuming for large structures |
∼100 nm |
10 and 111
|
Photolithography |
Patterns, arrays |
Atmosphere |
Batch fabrication, easy process |
Clean and flat surface needed |
∼1 μm |
9
|
Reactive ion etching |
Patterns, arrays |
Vacuum |
High resolution |
No selectivity, low throughput |
∼100 nm |
112 and 117
|
Template synthesis |
Porous |
Atmosphere |
Size-controlled |
Limited geometry |
<2 nm to >50 nm |
92
|
3.1 Types of surface structures
We broadly separate microscale surface morphologies into three subdivisions: array structures (Fig. 4A), pore structures (Fig. 4B), and cavity structures (Fig. 4C). Three-dimensional arrays are a common morphology of the carbon surface, with pillar arrays made by mask-based patterning being the most common (Fig. 4D).87,88 Some well-aligned nanomaterials, such as vertically aligned carbon nanotubes (VACNTs), are also considered as a dense carbon array structure.89,90 Porous carbon materials, such as activated charcoal and carbon foam, have large surface areas without much control over pore size. To control the pore size, template-based synthesis is used (Fig. 4E),91,92 and nanomaterial composites, such as CNT composites, are also porous carbon materials.93 Cavity structures are mostly fabricated in carbon pipes and carbon pipettes (Fig. 4F).94–97 Some nanomaterials are considered to be mixtures of these structures as well.
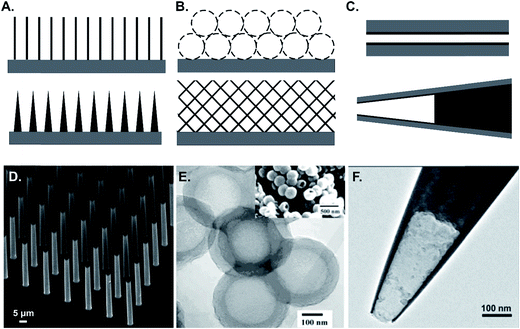 |
| Fig. 4 Illustration and examples of different types of surface 3D structures. (A) Array structures; (B) porous structures; (C) cavity structures. (D) Carbon pillar microarrays as an example of the array structure. Adapted from ref. 87 with permission from Wiley; (E) porous carbon as an example of the porous structure. Adapted from ref. 126 with permission from Wiley; (F) carbon pipette as an example of the cavity structure. Reprinted with permission from ref. 97. Copyright 2014 American Chemical Society. | |
3.2 Fabrication of surface micro/nano structures
3.2.1 Controlled growth of carbon nanomaterials.
While CNTs are often used for their chemical properties and rapid electron transfer, there are also structural advantages of using vertically aligned carbon nanomaterials as electrodes. VACNTs, sometimes referred to as CNT arrays or CNT forests, are grown with CNT lengths from 0.1 to 50 μm.98 Vertically aligned carbon nanofibers (VACNFs) are cylindrical or conical structures, with diameters from 1 to hundreds of nanometers, which differ from CNTs in the stacking of graphene sheets.90 Both VACNTs and VACNFs can be produced with a high degree of control by CVD or plasma-enhanced chemical-vapor deposition (PECVD).99 The structural advantage of vertically aligned nanomaterials is that they act as thin layer cells and trap the analyte of interest. Mao and collaborators used VACNT grown on carbon fibers for real-time, in vivo monitoring of ascorbate in rat brain82 and our group grew CNTs on metal wires and CFMEs for enhanced dopamine detection.6
CNTs can be spun into continuous fibers or yarns, often resulting in aligned CNT structures.100–103 Sombers' group constructed microelectrodes from CNT yarns and demonstrated highly reversible CVs for dopamine compared to carbon fibers.21 Safina and collaborators demonstrated that CNT fiber microelectrodes showed higher resistance to dopamine fouling,104 and our group proved that CNT yarn and CNT-fiber electrodes are useful to improve the time resolution of FSCV measurements.22,23,105 At CNT yarn electrodes, dopamine was measured at frequencies up to 500 Hz without significant loss of current.105
Carbon nanopipettes are produced by CVD deposition of a thin carbon layer on the inner surface of a quartz pipette, with a tip as small as tens to hundreds of nanometers (Fig. 4F).96 Carbon nanopipettes exhibit thin layer behavior, as diffusion distances are confined inside the pipette, leading to complete oxidation of all of the redox species.97 Carbon nanopipettes are used as nano-samplers,97 nanoelectrodes,96,97 and tips for scanning probe microscopy. The nano-scaled tip of carbon nanopipettes enables high spatial resolution measurements of neurotransmitters. Our group first used carbon nanopipette electrodes coupled with FSCV to measure dopamine changes in Drosophila, where the neuropil is only a few microns in diameter.96
3.2.2 3D-printing/direct laser writing.
3D-printing is another bottom-up approach exhibiting unique advantages in designing complex structures. Resolution has been a limitation to the 3D printing technique, as traditional 3D printing has a resolution range from tens of microns to millimeters, depending on the printing material.106 A novel 3D-printing strategy, direct laser writing, has improved the resolution to 100 nm scale while maintaining the structural stability (Fig. 5A–D).107–109 A laser cross-links a photoresist, but using two-photon absorption allows polymerization to happen in a specific place and not only on the surface (Fig. 5B).110 The 3D-printed structure (Fig. 5C) is pyrolyzed at high temperature, shrinking the architecture and producing a carbon surface (Fig. 5D).109,111 The pyrolyzed carbon surface is glassy-carbon like,112 exhibiting similar behavior to CFMEs for electrochemical detection of neurotransmitters.10 Sasso's group successfully fabricated 3D microelectrodes using direct laser writing followed by thin layer gold deposition.113 Our group fabricated free-standing, implantable electrodes using 3D printing to create the desired structures directly on small, 10–25 μm metal wires.10Fig. 5E and F show the printed, spiked structures before and after pyrolysis, and features less than 1 μm diameter were successfully patterned (Fig. 5F). The 3D-printed electrodes were implanted into rat brains where stimulated dopamine release as low as 92 nM was detected in vivo. Future work could include printing on smaller substrates, to decrease the overall size, and optimizing pyrolysis to make sure small features are maintained.
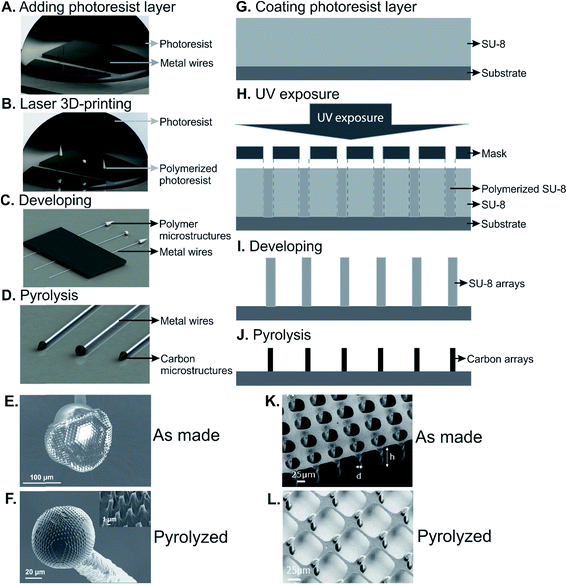 |
| Fig. 5 A schematic drawing of direct laser writing 3D printing and C-MEMS to fabricate 3D carbon structures. (A)–(D) Process flow of direct laser writing 3D printing; (E) and (F) an example of 3D-printed microstructure before and after pyrolysis. (G)–(J) Process flow of C-MEMS; (K) and (L) an example of carbon microarray fabricated by C-MEMS before and after pyrolysis. (A)–(F) Adapted from ref. 10 with permission from Wiley. (K) and (L) Reprinted from ref. 88 with permission from Elsevier. | |
3.2.3 Photolithography.
Photolithography is commonly used to pattern surface micro/nano structures on a substrate. Photolithography uses light to pattern a light-sensitive photoresist covered with a photo-mask.114,115 In cases when a patterned photoresist is pyrolyzed into carbon at high temperature, the process is often referred to as carbon-microelectromechanical systems (C-MEMS, Fig. 5G–J).116Fig. 5K and L are examples of arrays patterned by photolithography before and after pyrolysis. Pillar arrays are the standard morphology, but other geometries are made by doping nanoparticles, self-assembly, or multistep lithography.9,88,117 Carbon array microelectrodes have a large surface area and restricted mass transfer. For example, Emnéus and collaborators fabricated pyrolyzed carbon 3D scaffolds by photolithography, and cultured human neural stem cells at the bottom or between pillars. The scaffold electrode traps dopamine, and a larger fraction of released dopamine was detected than on a 2D substrate.87 Microarray structures can also be integrated into CMOS applications,118 enabling multichannel, simultaneous detection of different neurochemicals119 or from different regions.120,121 For example, Ewing group used lithography to fabricate microelectrode arrays on a chip, monitoring the neurotransmitter release in different regions of single-cell surfaces.122,123
3.2.4 Reactive ion etching.
Reactive-ion etching (RIE) is a type of dry etching which uses high-energy ions generated by plasma to attack the wafer surface and remove the materials. RIE reaches a resolution of sub-100 nm.124 For example, a nanostructured carbon electrode array was fabricated using RIE, and coupled with FSCV to detect low concentrations of dopamine.112
3.2.5 Template synthesis of porous carbon.
Template synthesis is widely used to fabricate porous carbon structures. A variety of materials are used as templates, including silica,125,126 silica oxide,127 polymer,128,129 and metal–organic frameworks.130 The templates are removed after deposition of carbon or polymer that is carbonized to form porous carbon structures. Porous carbon structures are classified into three subdivisions: microporous (<2 nm), mesoporous (2 nm to 50 nm), and macroporous (>50 nm).92 Thin layer diffusion has been observed at GCEs modified with porous film.131 The Chen group reported simultaneous detection of ascorbic acid, dopamine, and uric acid using nitrogen doped porous carbon nanopolyhedra (N–PCNP) modified electrodes. The N-PCNPs/GC electrode showed better peak separation due to the narrow pore-size distribution, high surface area, and nitrogen-doping.132
3.3 Electrochemical implications of changing surface morphology
3.3.1 Increased surface area for enhanced sensitivity.
A main advantage of 3D-structured microelectrodes is that the larger electrode surface area provides higher sensitivity for electroanalytical sensing, and a majority of papers highlight this feature.133 For substrate-based electrode sensors, 3D structures significantly increase the active surface area without increasing much volume. 3D carbon arrays offer significant increases in surface area. For example, Hemanth et al. fabricated suspended 3D pyrolytic carbon microelectrodes from an SU-8 template.88 Using square wave voltammetry for dopamine detection, 3D electrodes showed peak current response twice as high as 2D electrodes. 3D carbon arrays also increase the sensitivity in FSCV detection of dopamine,112 which are similar to the nanostructured carbon electrode arrays made using reactive ion etching. Porous carbon-modified electrodes are also sensitive and selective sensors of dopamine, with a limit of detection (LOD) that surpasses GCEs and other modified electrodes.134 Therefore, 3D structures are an effective method for increasing the surface area.
3.3.2 Collector/generator for increased sensitivity.
Surface geometries are also an important factor in collector/generator systems. Compton et al. summarized the theory of different geometries of the collector/generator electrodes.135 Interdigitated electrode arrays (IDEAs) are advantageous due to the very close inter-electrode spacing which causes the diffusion layers of generator and collector electrodes to overlap. Thus, when the species is formed at the generator electrode, it is already in the depletion zone around the generator electrode and quickly diffuses back for a feedback response. Kamath's group fabricated IDEAs using photolithography and evaluated the relationship of how different parameters such as height and width/gap ratio led to signal amplification.133 The Amemiya group reported a double carbon-fiber microelectrode system, with a nanogap in between them, for the detection of dopamine selectively over ascorbic acid, because the irreversible ascorbic acid is redox-inactive at the collector electrode.136 This electrode has not been used in vivo, but may offer advantages of limited fouling and high selectivity.137 Scan rate is an important factor in collector/generator systems. At slower scan rates, when the reaction rate to consume the species is smaller than the diffusion rate from bulk solution, a steady state is formed and the voltammogram shows a diffusion plateau.133 At faster scan rates, the plateau gradually changes into peaks.136 Collector–generator systems are useful for fluidic devices, but have not been implemented in vivo yet.
3.3.3 Thin layer effects and analyte confinement enhance sensitivity and selectivity.
One of the main effects of making a nanostructured or cavity geometry is that the analyte is confined in the material on the time scale of a short electrochemical measurement. When the surface structure scale is of the same magnitude as the diffusion layer, mass transfer is limited to a small volume of solution confined to a thin layer, estimated to be 1–10 μm for experiments on the millisecond to hundred millisecond time scale. However, in practice even surface microstructures down to the sub-micron scale can exhibit the thin layer effects.105,138 The tortuosity of surface structures increases the diffusion pathlength, and interactions between electroactive species decrease the diffusion rate. Using FSCV, a rough electrode surface significantly improves temporal resolution due to the thin layer effect.139 Surface roughness also promotes selectivity, as some reactions such as the cyclization of dopamine and subsequent leucodopaminechrome peaks are more distinct in long-length CNT electrodes compared with short-length CNT electrodes, due to trapping effects.83
The thin layer effect has been studied at several carbon electrode materials, including multiwalled carbon nanotubes,138,140–143 single walled carbon nanotubes,131,141,144 micropillar array electrodes,145 porous electrodes,143,146,147 and carbon pipettes.97 At slow scan rates, surface-structured electrodes behave similar to planar electrodes, because the mass transport towards the electrode is dominated by flux from the bulk and the contribution of species initially located within the electrode surface layer can be neglected. At higher scan rates, most current is due to thin-layer diffusion, which results in a decrease of peak-to-peak separation (ΔEp).138 For example, Compton's group found that the ΔEp decreased as the scan rate increased using a MWCNT-modified GCE (Fig. 6A–C).138 Similar results were observed at carbon pipette-based electrodes, where the thin layer effect was observed at scan rates higher than 50 mV s−1.97
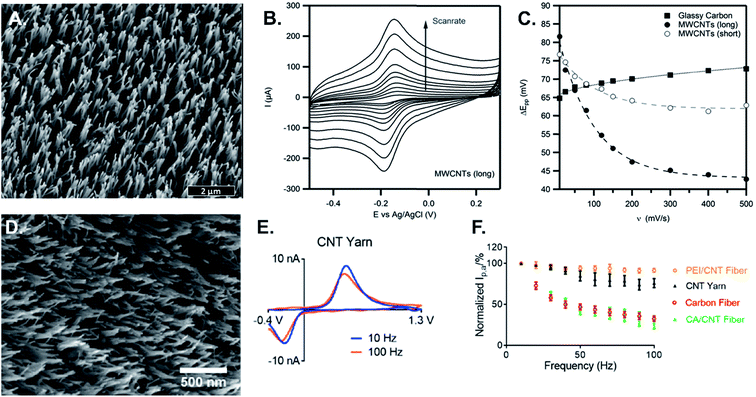 |
| Fig. 6 Thin layer effect studies in CV and FSCV. (A) 3 μm long MWCNT modified GCE; (B) scan rate study of 1 mM Ru(NH3)6Cl3; (C) peak-to-peak separation vs. scan rate. The distance between the peaks is much smaller with long MWCNTs because the analyte is trapped at time scales of fast experiments. (D) CNT-yarn microelectrode; (E) effect of scan repetition frequency for 1 μM dopamine detection at the scan repetition frequency of 10 Hz (blue) and 100 Hz (orange); (F) peak oxidation current vs. frequency at different microelectrodes. Polyethyleneimine (PEI)-CNT and CNT yarn electrodes act as thin layer cells, which make them nearly frequency independent, while carbon fibers and chlorosulfonic acid (CA)-CNT fibers are not thin-layer cells and are frequency independent. (A)–(C) Reprinted from ref. 138 with permission from Elsevier. (D)–(F) Reprinted from ref. 22 with permission from Elsevier. | |
In FSCV, measurements are typically limited to scan repetition frequencies of 10 Hz and increasing the scan repetition frequency from 10 to 90 Hz dramatically decreases the electrode sensitivity.3 In addition, CVs of dopamine at CFMEs typically have much larger oxidation currents than reduction currents. In contrast, the oxidation current of dopamine at CNT-yarn electrodes and CNT-fiber electrodes is nearly independent of scan repetition frequency, and the reduction peak is of similar magnitude to the oxidation peak (Fig. 6D–F).22,105 At CFMEs, the oxidized species dopamine-o-quinone (DOQ) desorbs easily and diffuses away from the electrode surface, resulting in the smaller magnitude reduction peak. At rough surfaces such as CNT-yarn electrodes, where the surface acts as a thin layer cell, DOQ remains close to the electrode surface and easily adsorbs again if it desorbs.22 Thus, CNT yarns and CNT fibers with micron scale surface roughness have more reversible behavior and are attractive for high-speed measurements of neurotransmitters.
4. Future directions: designing carbon electrodes for neurotransmitter sensing
4.1 Rational design of neurotransmitter sensors
Carbon-fiber electrodes have been a mainstay of neurotransmitter sensing for decades, and many new strategies are evolving for using carbon nanomaterials, lithography, and 3D-printing techniques to fabricate carbon microelectrodes. The enhancement of electrode performance is explained by chemical or surface structures using electrochemistry principles, but many research articles lack a solid foundation in fundamental electrochemistry. Therefore, rational design and optimization of chemical and 3D-surface structures is needed in order to better tune the electrochemical performance.
For dopamine, the optimal electrode provides abundant adsorption sites while maintaining electrode conductivity. New nanomaterials that have more edge plane sites and oxide functional groups will be better for electrode sensitivity. In addition, while laser or plasma treatments increase active sites and oxygen containing functional groups,46,47 they have been demonstrated only on a limited number of nanomaterials and could be more widely adopted. However, nanomaterials with 100 nanometer scale roughness will only provide sensitivity increases by promoting adsorption. To take advantage of the trapping effects and sensitivity increases of thin layer cells, the material must have surface roughness or pores that approach the micron scale. Therefore, electrodes that have rougher surface structures may have more benefits for electrochemistry than increasing active sites alone. VACNTs and VACNFs are promising for promoting thin layer cell effects, and they are combined with nanomaterial doping,148 surface treatments,46,47 or polymer coatings64,74,149 to promote selectivity as well. In addition, 3D printing and lithography are used to rationally design structures that will act as thin layer cells and trap dopamine. If the pyrolyzed carbon in these electrodes does not provide optimal electroactivity, it could be coated by a thin layer of carbon nanotubes20,44 or graphene nanomaterials28,132 to improve dopamine detection.
Many papers currently attack the problem of ascorbic acid interference of dopamine detection, and carbon nanomaterials and polymer coatings are good for promoting selectivity based on charge. One strategy is to use the electrocatalytic effects of nanomaterials to separate the peaks of dopamine and ascorbic acid and many nanomaterials do this effectively, including CNTs,18 N-doped porous carbon nanopolyhedra,132 and N-doped graphene.58 The Laurila group showed that diamond-like carbon (DLC)–MWCNT hybrid electrodes could determine dopamine in the presence of ascorbic acid.150 Future research will likely explore more hybrid nanomaterials to explore how their chemical and surface structures help discriminate dopamine and ascorbic acid. Polymer-based approaches are also common for reducing ascorbate interference. PEDOT-based electrodes discriminate dopamine from ascorbic acid and uric acid by separating the peaks.71 PEDOT functionalized with carboxylic acid groups rejects ascorbic acid and increases the signal for dopamine.79 Nafion, which can be combined with CNTs, rejects cations such as ascorbic acid.64 Future studies should also take advantage of the redox cycling of dopamine in thin layer cells, which should also promote dopamine detection over ascorbic acid, which is less reversible.136,137
One area of promise for the rational design of electrodes is to examine other electroactive neurochemicals besides dopamine. Some of them, such as the catecholamines epinephrine and norepinephrine, may undergo redox cycling, but their adsorption properties may differ from those of dopamine. Others, such as serotonin, are also cationic, but have different structures and problems with fouling as the electroactive product polymerizes and builds up on the electrode. CNT fibers have been shown to resist fouling by serotonin, so it is possible to optimize carbon surfaces for serotonin detection.23 BDD and CNT network electrodes were also favorable for trace serotonin detection because of their low background currents. While CNT networks were the most sensitive, BDD electrodes were more resistant to fouling.52 Some neurochemicals have irreversible electrochemistry, such as adenosine,151 and these neurochemicals are expected to have less sensitivity enhancement at thin-layer cell electrodes, since they would not undergo redox cycling. Thus, surface structured electrodes might not have equal enhancements for all neurochemicals, and these effects need to be better studied. Electrodes on the nanoscale, particularly carbon nanopipettes, might also have enhanced electric fields at their small tips, and electrostatic effects might also be used to preferentially detect certain analytes by charge. While dopamine is a good test compound for fundamental studies, other compounds should be examined as some nanomaterials may provide enhanced selectivity or adsorption for different classes of compounds.
4.2 Enhanced detection of neurotransmitters in vivo
While research has concentrated on the fundamental electrochemical properties of new carbon electrodes, translating those properties into electrodes that are used for cellular or tissue measurements is difficult. One of the biggest concerns in real tissue is biocompatibility and fouling and many studies claim that carbon nanomaterials have antifouling properties. For example, CNTs grown on microwires have only slightly lower current density in vivo than during calibration.6 The high sensitivity of carbon nanomaterials also means that they retain large currents even if there is some biofouling in tissue.47 The charge of the electrode material affects its hydrophilicity, and more hydrophilic surfaces typically experience less adsorption of proteins and biomolecules.152 Polymer coatings also lessen fouling,153 and the recent work by the Mao group shows that the PEDOT-PC coated electrode exhibited almost no biofouling.80 The downside to zwitterionic phosphorylcholine coatings is that they decrease the sensitivity for dopamine, compared to carboxylic acid functionalized PEDOT.79 Recently, Sombers' group reported a different strategy for rejecting larger molecular interferents: using a size exclusion polymer coating. Electrodeposition of 1,3-phenylenediamine onto CFMEs creates a size-exclusion membrane to reject larger molecular interfering agents for FSCV detection of H2O2.154 Similar effects might be obtained by nanolithography or 3D printing to print pores to exclude proteins. Solving biofouling issues is key to improving the functionality of new carbon electrodes and future studies should also focus on testing electrodes for long term implantation.
New carbon electrodes are suitable for cellular studies and in vivo measurements. Scaffolds of 3D pillars have been used to grow and differentiate stem cells and study the dynamics of exocytotic release.87 Nanodiamond coatings increases cell viability for applications of growing cells on electrodes.37 Arrays are also useful for measuring neurotransmitter release at many cells simultaneously.155 Many of the current array configurations are not individually addressable and thus future work should focus on making more parallel sensors for neurotransmitter detection. In brain slices, graphene-modified electrodes have been used with slow scan CV25 and CNT yarn microelectrodes with FSCV to detect dopamine.21In vivo, CNT yarn microelectrodes produce higher currents at faster temporal resolution than CFMEs.47 CNT-CFMEs and VACNTs have been used to detect ascorbic acid levels in the brain.82,156 Smaller electrodes are useful for detecting dopamine in smaller model organisms, such as carbon nanopipettes used in Drosophila,96 and carbon-fiber nanotip electrodes used in living PC12 cells.157 Most of these studies are short demonstrations that the electrodes can be used in vivo, but very few neuroscience studies have extensively demonstrated the long term in vivo applications of these new carbon electrodes. Therefore, more work is needed to understand the advantages of new carbon electrodes in vivo and efforts are needed to make them in a robust, reproducible manner that is amenable to mass fabrication and eventual distribution to neuroscientists.
5. Conclusions
In this article, we have shown that both chemical structure and 3D surface structure affect the electrochemistry of neurotransmitter detection. Carbon nanotube and graphene modified electrodes have electrocatalytic effects, enhance the amount of edge plane sites, and can be functionalized to promote surface oxide groups that adsorb dopamine. Polymers or polymer–nanomaterial coatings increase selectivity and decrease fouling. Making carbon nanomaterial electrodes with surface roughness on the micron scale provides additional enhancements because the electrodes act as thin layer cells and trap dopamine, increasing the sensitivity and selectivity. Nanolithography and 3D printing are also used to make arrays that act like thin layer cells or collector–generators. Research is now transitioning into using our understanding of electrode properties to rationally design electrodes, for dopamine and also other neurotransmitters. In vivo measurements are just beginning, but treatments to reduce biofouling and increase biocompatibility show great promise in making these electrodes advantageous for tissue measurements.
Conflicts of interest
There are no conflicts to declare.
Acknowledgements
Work in the Venton lab is supported by NIH R01EB026497 and R01MH085159.
References
- R. L. McCreery, Chem. Rev., 2008, 108, 2646–2687 CrossRef CAS PubMed.
- M. L. Huffman and B. J. Venton, Analyst, 2009, 134, 18–24 RSC.
- B. D. Bath, D. J. Michael, B. J. Trafton, J. D. Joseph, P. L. Runnels and R. M. Wightman, Anal. Chem., 2000, 72, 5994–6002 CrossRef CAS PubMed.
- D. L. Robinson, A. Hermans, A. T. Seipel and R. M. Wightman, Chem. Rev., 2008, 108, 2554–2584 CrossRef CAS PubMed.
- M. Ganesana, S. T. Lee, Y. Wang and B. J. Venton, Anal. Chem., 2017, 89, 314–341 CrossRef CAS PubMed.
- C. Yang, C. B. Jacobs, M. D. Nguyen, M. Ganesana, A. G. Zestos, I. N. Ivanov, A. A. Puretzky, C. M. Rouleau, D. B. Geohegan and B. J. Venton, Anal. Chem., 2016, 88, 645–652 CrossRef CAS PubMed.
- A. Oleinick, I. Álvarez-Martos, I. Svir, E. E. Ferapontova and C. Amatore, J. Electrochem. Soc., 2018, 165, G3057–G3065 CrossRef CAS.
- C. Yang, M. E. Denno, P. Pyakurel and B. J. Venton, Anal. Chim. Acta, 2015, 887, 17–37 CrossRef CAS PubMed.
- C. Wang and M. Madou, Biosens. Bioelectron., 2005, 20, 2181–2187 CrossRef CAS PubMed.
- C. Yang, Q. Cao, P. Puthongkham, S. T. Lee, M. Ganesana, N. V. Lavrik and B. J. Venton, Angew. Chem., Int. Ed., 2018, 57, 14255–14259 CrossRef CAS PubMed.
- C. E. Banks and R. G. Compton, Analyst, 2006, 131, 15–21 RSC.
- C. E. Banks, R. R. Moore, T. J. Davies and R. G. Compton, Chem. Commun., 2004, 16, 1804–1805 RSC.
- P. R. Unwin, A. G. Güell and G. Zhang, Acc. Chem. Res., 2016, 49, 2041–2048 CrossRef CAS PubMed.
- E. C. Mitchell, L. E. Dunaway, G. S. McCarty and L. A. Sombers, Langmuir, 2017, 33, 7838–7846 CrossRef CAS PubMed.
- C. E. Banks, T. J. Davies, G. G. Wildgoose and R. G. Compton, Chem. Commun., 2005, 829–841 RSC.
- W. Yuan, Y. Zhou, Y. Li, C. Li, H. Peng, J. Zhang, Z. Liu, L. Dai and G. Shi, Sci. Rep., 2013, 3, 2248 CrossRef PubMed.
- V. Georgakilas, J. A. Perman, J. Tucek and R. Zboril, Chem. Rev., 2015, 115, 4744–4822 CrossRef CAS PubMed.
- S. B. Hočevar, J. Wang, R. P. Deo, M. Musameh and B. Ogorevc, Electroanalysis, 2005, 17, 417–422 CrossRef.
- T. Xiao, Y. Jiang, W. Ji and L. Mao, Anal. Chem., 2018, 90, 4840–4846 CrossRef CAS PubMed.
- N. Xiao and B. J. Venton, Anal. Chem., 2012, 84, 7816–7822 CrossRef CAS PubMed.
- A. C. Schmidt, X. Wang, Y. Zhu and L. A. Sombers, ACS Nano, 2013, 7, 7864–7873 CrossRef CAS PubMed.
- C. Yang, E. Trikantzopoulos, C. B. Jacobs and B. J. Venton, Anal. Chim. Acta, 2017, 965, 1–8 CrossRef CAS PubMed.
- A. G. Zestos, C. B. Jacobs, E. Trikantzopoulos, A. E. Ross and B. J. Venton, Anal. Chem., 2014, 86, 8568–8575 CrossRef CAS PubMed.
- Y. Wang, Y. Li, L. Tang, J. Lu and J. Li, Electrochem. Commun., 2009, 11, 889–892 CrossRef CAS.
- M. Zhu, C. Zeng and J. Ye, Electroanalysis, 2011, 23, 907–914 CrossRef CAS.
- Q. Hong, L. Yang, L. Ge, Z. Liu and F. Li, Analyst, 2018, 143, 3327–3334 RSC.
- V. Penmatsa, T. Kim, M. Beidaghi, H. Kawarada, L. Gu, Z. Wang and C. Wang, Nanoscale, 2012, 4, 3673–3678 RSC.
- A. G. Zestos, C. Yang, C. B. Jacobs, D. Hensley and B. J. Venton, Analyst, 2015, 140, 7283–7292 RSC.
- F. Valentini, E. Ciambella, A. Boaretto, G. Rizzitelli, M. Carbone, V. Conte, F. Cataldo, V. Russo, C. S. Casari, D. F. Chillura-Martino, E. Caponetti, M. Bonchio, F. Giacalone, Z. Syrgiannis and M. Prato, Electroanalysis, 2016, 28, 2489–2499 CrossRef CAS.
- P. Puthongkham, C. Yang and B. J. Venton, Electroanalysis, 2018, 30, 1073–1081 CrossRef CAS PubMed.
- N. Yang, J. S. Foord and X. Jiang, Carbon, 2016, 99, 90–110 CrossRef CAS.
- K. E. Bennet, J. R. Tomshine, H.-K. Min, F. S. Manciu, M. P. Marsh, S. B. Paek, M. L. Settell, E. N. Nicolai, C. D. Blaha, A. Z. Kouzani, S.-Y. Chang and K. H. Lee, Front. Hum. Neurosci., 2016, 10, 102 Search PubMed.
- V. N. Mochalin, O. Shenderova, D. Ho and Y. Gogotsi, Nat. Nanotechnol., 2011, 7, 11–23 CrossRef PubMed.
- K. B. Holt, C. Ziegler, D. J. Caruana, J. Zang, E. J. Millán-Barrios, J. Hu and J. S. Foord, Phys. Chem. Chem. Phys., 2008, 10, 303–310 RSC.
- L. C. Hian, K. J. Grehan, R. G. Compton, J. S. Foord and F. Marken, Diamond Relat. Mater., 2003, 12, 590–595 CrossRef CAS.
- J. B. Zang, Y. H. Wang, S. Z. Zhao, L. Y. Bian and J. Lu, Diamond Relat. Mater., 2007, 16, 16–20 CrossRef CAS.
- E. Peltola, N. Wester, K. B. Holt, L. S. Johansson, J. Koskinen, V. Myllymäki and T. Laurila, Biosens. Bioelectron., 2017, 88, 273–282 CrossRef CAS PubMed.
- J. Robertson, Mater. Sci. Eng., R, 2002, 37, 129–281 CrossRef.
- T. Laurila, A. Rautiainen, S. Sintonen, H. Jiang, E. Kaivosoja and J. Koskinen, Mater. Sci. Eng., C, 2014, 34, 446–454 CrossRef CAS PubMed.
- T. Laurila, V. Protopopova, S. Rhode, S. Sainio, T. Palomäki, M. Moram, J. M. Feliu and J. Koskinen, Diamond Relat. Mater., 2014, 49, 62–71 CrossRef CAS.
- N. R. Devi, T. H. V. Kumar and A. K. Sundramoorthy, J. Electrochem. Soc., 2018, 165, G3112–G3119 CrossRef.
- J. Fang, Z. Xie, G. Wallace and X. Wang, Appl. Surf. Sci., 2017, 412, 131–137 CrossRef CAS.
- P. Takmakov, M. K. Zachek, R. B. Keithley, P. L. Walsh, C. Donley, G. S. McCarty and R. M. Wightman, Anal. Chem., 2010, 82, 2020–2028 CrossRef CAS PubMed.
- C. B. Jacobs, T. L. Vickrey and B. J. Venton, Analyst, 2011, 136, 3557–3565 RSC.
- C. S. R. Vusa, M. Venkatesan, A. K. Mehta, S. Berchmans and P. Arumugam, Sci. Rep., 2017, 7, 8354 CrossRef PubMed.
- C. Yang, Y. Wang, C. B. Jacobs, I. Ivanov and B. J. Venton, Anal. Chem., 2017, 5605–5611 CrossRef CAS PubMed.
- C. Yang, E. Trikantzopoulos, M. D. Nguyen, C. B. Jacobs, Y. Wang, M. Mahjouri-Samani, I. N. Ivanov and B. J. Venton, ACS Sens., 2016, 1, 508–515 CrossRef CAS PubMed.
- A. Kaplan, Z. Yuan, J. D. Benck, A. Govind Rajan, X. S. Chu, Q. H. Wang and M. S. Strano, Chem. Soc. Rev., 2017, 46, 4530–4571 RSC.
- A. Ambrosi, C. K. Chua, A. Bonanni and M. Pumera, Chem. Rev., 2014, 114, 7150–7188 CrossRef CAS PubMed.
- A. E. Fischer and G. M. Swain, J. Electrochem. Soc., 2005, 152, B369 CrossRef CAS.
- C. A. Rusinek, Y. Guo, R. Rechenberg, M. F. Becker, E. Purcell, M. Verber, C. McKinney and W. Li, J. Electrochem. Soc., 2018, 165, G3087–G3092 CrossRef CAS.
- A. G. Güell, K. E. Meadows, P. R. Unwin and J. V. MacPherson, Phys. Chem. Chem. Phys., 2010, 12, 10108–10114 RSC.
- T. J. Li, M. H. Yeh, W. H. Chiang, Y. S. Li, G. L. Chen, Y. A. Leu, T. C. Tien, S. C. Lo, L. Y. Lin, J. J. Lin and K. C. Ho, Sens. Actuators, B, 2017, 248, 288–297 CrossRef CAS.
- X. Bo, M. Li, C. Han and L. Guo, Electrochim. Acta, 2013, 114, 582–589 CrossRef CAS.
- M. Inagaki, M. Toyoda, Y. Soneda and T. Morishita, Carbon, 2018, 132, 104–140 CrossRef CAS.
- X. Ding, J. Bai, T. Xu, C. Li, H.-M. Zhang and L. Qu, Electrochem. Commun., 2016, 72, 122–125 CrossRef CAS.
- J. A. Behan, M. K. Hoque, S. N. Stamatin, T. S. Perova, L. Vilella-Arribas, M. García-Melchor and P. E. Colavita, J. Phys. Chem. C, 2018, 122, 20763–20773 CrossRef CAS.
- B. J. Matsoso, B. K. Mutuma, C. Billing, K. Ranganathan, T. Lerotholi, G. Jones and N. J. Coville, Electrochim. Acta, 2018, 286, 29–38 CrossRef CAS.
- F. Cao, L. Zhang and Y. Tian, J. Electroanal. Chem., 2016, 781, 278–283 CrossRef CAS.
- Y. Qi, J. Bai, X. Ding and H. M. Zhang, Electroanalysis, 2017, 29, 2083–2089 CrossRef CAS.
- K. T. Kawagoe, J. A. Jankowski and R. M. Wightman, Anal.
Chem., 1991, 63, 1589–1594 CrossRef CAS PubMed.
- P. Hashemi, E. C. Dankoski, J. Petrovic, R. B. Keithley and R. M. Wightman, Anal. Chem., 2009, 81, 9462–9471 CrossRef CAS PubMed.
- C. C. Hsueh and A. Brajter-Toth, Anal. Chem., 1994, 66, 2458–2464 CrossRef CAS.
- M. J. Peairs, A. E. Ross and B. J. Venton, Anal. Methods, 2011, 3, 2379–2386 RSC.
- K. Pihel, Q. D. Walker and R. M. Wightman, Anal. Chem., 1996, 68, 2084–2089 CrossRef CAS PubMed.
- L. Sasso, A. Heiskanen, F. Diazzi, M. Dimaki, J. Castillo-León, M. Vergani, E. Landini, R. Raiteri, G. Ferrari, M. Carminati, M. Sampietro, W. E. Svendsen and J. Emnéus, Analyst, 2013, 138, 3651–3659 RSC.
- H. Olivia, B. V. Sarada, D. Shin, T. N. Rao and A. Fujishima, Analyst, 2002, 127, 1572–1575 RSC.
- M. Ates, Mater. Sci. Eng., C, 2013, 33, 1853–1859 CrossRef CAS PubMed.
- W. Lei, W. Si, Y. Xu, Z. Gu and Q. Hao, Microchim. Acta, 2014, 181, 707–722 CrossRef CAS.
- S. Nambiar and J. T. W. Yeow, Biosens. Bioelectron., 2011, 26, 1825–1832 CrossRef CAS PubMed.
- F. S. Belaidi, A. Civélas, V. Castagnola, A. Tsopela, L. Mazenq, P. Gros, J. Launay and P. Temple-Boyer, Sens. Actuators, B, 2015, 214, 1–9 CrossRef CAS.
- A. R. Meier, M. Matteucci, R. F. Vreeland, R. Taboryski and M. L. Heien, Langmuir, 2016, 32, 8009–8017 CrossRef CAS PubMed.
- I. M. Taylor, E. M. Robbins, K. A. Catt, P. A. Cody, C. L. Happe and X. T. Cui, Biosens. Bioelectron., 2015, 89, 400–410 CrossRef PubMed.
- R. Samba, K. Fuchsberger, I. Matiychyn, S. Epple, L. Kiesel, A. Stett, W. Schuhmann and M. Stelzle, Electroanalysis, 2014, 26, 548–555 CrossRef CAS.
- J. Samseya, R. Srinivasan, Y. T. Chang, C. W. Tsao and V. S. Vasantha, Anal. Chim. Acta, 2013, 793, 11–18 CrossRef CAS PubMed.
- G. Fabregat, E. Córdova-Mateo, E. Armelin, O. Bertran and C. Alemán, J. Phys. Chem. C, 2011, 115, 14933–14941 CrossRef CAS.
- P. Manivel, M. Dhakshnamoorthy, A. Balamurugan, N. Ponpandian, D. Mangalaraj and C. Viswanathan, RSC Adv., 2013, 3, 14428–14437 RSC.
- Y. Zhang, L. Lin, Z. Feng, J. Zhou and Z. Lin, Electrochim. Acta, 2009, 55, 265–270 CrossRef CAS.
- C.-H. Chen and S.-C. Luo, ACS Appl. Mater. Interfaces, 2015, 7, 21931–21938 CrossRef CAS PubMed.
- X. Liu, T. Xiao, F. Wu, M.-Y. Shen, M. Zhang, H. Yu and L. Mao, Angew. Chem., Int. Ed., 2017, 56, 11802–11806 CrossRef CAS PubMed.
- R. F. Vreeland, C. W. Atcherley, W. S. Russell, J. Y. Xie, D. Lu, N. D. Laude, F. Porreca and M. L. Heien, Anal. Chem., 2015, 87, 2600–2607 CrossRef CAS PubMed.
- L. Xiang, P. Yu, J. Hao, M. Zhang, L. Zhu, L. Dai and L. Mao, Anal. Chem., 2014, 86, 3909–3914 CrossRef CAS PubMed.
- H. Muguruma, Y. Inoue, H. Inoue and T. Ohsawa, J. Phys. Chem. C, 2016, 120, 12284–12292 CrossRef CAS.
- G. A. Gerhardt, A. F. Oke, G. Nagy, B. Moghaddam and R. N. Adams, Brain Res., 1984, 290, 390–395 CrossRef CAS PubMed.
- Y. Shao, J. Wang, H. Wu, J. Liu, I. A. Aksay and Y. Lin, Electroanalysis, 2010, 22, 1027–1036 CrossRef CAS.
- M. Trojanowicz, TrAC, Trends Anal. Chem., 2006, 25, 482–489 CrossRef.
- L. Amato, A. Heiskanen, C. Caviglia, F. Shah, K. Zór, M. Skolimowski, M. Madou, L. Gammelgaard, R. Hansen, E. G. Seiz, M. Ramos, T. R. Moreno, A. Martínez-Serrano, S. S. Keller and J. Emnéus, Adv. Funct. Mater., 2014, 24, 7042–7052 CrossRef CAS.
- S. Hemanth, C. Caviglia and S. S. Keller, Carbon, 2017, 121, 226–234 CrossRef CAS.
- Y. C. Choi, Y. M. Shin, Y. H. Lee, B. S. Lee, G. S. Park, W. B. Choi, N. S. Lee and J. M. Kim, Appl. Phys. Lett., 2000, 76, 2367–2369 CrossRef CAS.
- A. V. Melechko, V. I. Merkulov, T. E. McKnight, M. A. Guillorn, K. L. Klein, D. H. Lowndes and M. L. Simpson, J. Appl. Phys., 2005, 97, 041301 CrossRef.
- C. Liang, Z. Li and S. Dai, Angew. Chem., Int. Ed., 2008, 47, 3696–3717 CrossRef CAS PubMed.
- J. Lee, J. Kim and T. Hyeon, Adv. Mater., 2006, 18, 2073–2094 CrossRef CAS.
- L. F. Chen, X. D. Zhang, H. W. Liang, M. Kong, Q. F. Guan, P. Chen, Z. Y. Wu and S. H. Yu, ACS Nano, 2012, 6, 7092–7102 CrossRef CAS PubMed.
- R. C. Mani, X. Li, M. K. Sunkara and K. Rajan, Nano Lett., 2003, 3, 671–673 CrossRef CAS.
- B. M. Kim, T. Murray and H. H. Bau, Nanotechnology, 2005, 16, 1317–1320 CrossRef CAS.
- H. R. Rees, S. E. Anderson, E. Privman, H. H. Bau and B. J. Venton, Anal. Chem., 2015, 87, 3849–3855 CrossRef CAS PubMed.
- Y. Yu, M. V Mirkin, Y. Gao, O. Mashtalir, G. Friedman and Y. Gogotsi, Anal. Chem., 2014, 86, 3365–3372 CrossRef CAS PubMed.
- Z. F. Ren, Z. P. Huang, J. W. Xu, J. H. Wang, P. Bush, M. P. Siegal and P. N. Provencio, Science, 1998, 282, 1105–1107 CrossRef CAS PubMed.
- M. Meyyappan, L. Delzeit, A. Cassell and D. Hash, Plasma Sources Sci. Technol., 2003, 12, 205–216 CrossRef CAS.
- M. Zhang, K. R. Atkinson and R. H. Baughman, Science, 2004, 306, 1358–1362 CrossRef CAS PubMed.
- Y. L. Li, I. A. Kinloch and A. H. Windle, Science, 2004, 304, 276–278 CrossRef CAS PubMed.
- L. M. Ericson, H. Fan, H. Peng, V. A. Davis, W. Zhou, J. Sulpizio, Y. Wang, R. Booker, J. Vavro, C. Guthy, A. N. G. Parra-Vasquez, M. J. Kim, S. Ramesh, R. K. Saini, C. Kittrell, G. Lavin, H. Schmidt, W. W. Adams, W. E. Billups, M. Pasquali, W. F. Hwang, R. H. Hauge, J. E. Fischer and R. E. Smalley, Science, 2004, 305, 1447–1450 CrossRef CAS PubMed.
- X. Zhang, Q. Li, T. G. Holesinger, P. N. Arendt, J. Huang, P. D. Kirven, T. G. Clapp, R. F. DePaula, X. Liao, Y. Zhao, L. Zheng, D. E. Peterson and Y. Zhu, Adv. Mater., 2007, 19, 4198–4201 CrossRef CAS.
- W. Harreither, R. Trouillon, P. Poulin, W. Neri, A. G. Ewing and G. Safina, Anal. Chem., 2013, 85, 7447–7453 CrossRef CAS PubMed.
- C. B. Jacobs, I. N. Ivanov, M. D. Nguyen, A. G. Zestos and B. J. Venton, Anal. Chem., 2014, 86, 5721–5727 CrossRef CAS PubMed.
- H. N. Chia and B. M. Wu, J. Biol. Eng., 2015, 9, 1–14 CrossRef CAS PubMed.
- M. Deubel, G. Von Freymann, M. Wegener, S. Pereira, K. Busch and C. M. Soukoulis, Nat. Mater., 2004, 3, 444–447 CrossRef CAS PubMed.
- M. S. Rill, C. Plet, M. Thiel, I. Staude, G. Von Freymann, S. Linden and M. Wegener, Nat. Mater., 2008, 7, 543–546 CrossRef CAS.
- G. Seniutinas, A. Weber, C. Padeste, I. Sakellari, M. Farsari and C. David, Microelectron. Eng., 2018, 191, 25–31 CrossRef CAS.
- M. Malinauskas, M. Farsari, A. Piskarskas and S. Juodkazis, Phys. Rep., 2013, 533, 1–31 CrossRef CAS.
- J. Bauer, A. Schroer, R. Schwaiger and O. Kraft, Nat. Mater., 2016, 15, 438–443 CrossRef CAS.
- S. Demuru, L. Nela, N. Marchack, S. J. Holmes, D. B. Farmer, G. S. Tulevski, Q. Lin and H. Deligianni, ACS Sens., 2018, 3, 799–805 CrossRef CAS PubMed.
- M. Al Abaddi, L. Sasso, M. Dimaki and W. E. Svendsen, Microelectron. Eng., 2012, 98, 378–381 CrossRef CAS.
- R. P. Seisyan, Tech. Phys., 2011, 56, 1061–1073 CrossRef CAS.
- Y. Fuchs, O. Soppera and K. Haupt, Anal. Chim. Acta, 2012, 717, 7–20 CrossRef CAS PubMed.
- C. Wang, L. Taherabadi, G. Jia, M. Madou, Y. Yeh and B. Dunn, Electrochem. Solid-State Lett., 2004, 7, A435 CrossRef CAS.
- P. Abgrall, V. Conedera, H. Camon, A. M. Gue and N. T. Nguyen, Electrophoresis, 2007, 28, 4539–4551 CrossRef CAS PubMed.
- K. L. Parent, D. F. Hill, L. M. Crown, J. P. Wiegand, K. F. Gies, M. A. Miller, C. W. Atcherley, M. L. Heien and S. L. Cowen, Anal. Chem., 2017, 89, 2790–2799 CrossRef CAS PubMed.
- M. K. Zachek, P. Takmakov, B. Moody, R. M. Wightman and G. S. McCarty, Anal. Chem., 2009, 81, 6258–6265 CrossRef CAS PubMed.
- M. K. Zachek, J. Park, P. Takmakov, R. M. Wightman and G. S. McCarty, Analyst, 2010, 135, 1556–1563 RSC.
- J. Wang and A. G. Ewing, Analyst, 2014, 139, 3290–3295 RSC.
- J. Wang, J. Dunevall and A. G. Ewing, Anal. Chem., 2014, 86, 8–13 Search PubMed.
- J. Wigstro, J. Dunevall, N. Naja, J. Lovric, J. Wang and A. G. Ewing, Anal. Chem., 2016, 88, 2080–2087 CrossRef PubMed.
- A. V Whitney, B. D. Myers and R. P. Van Duyne, Nano Lett., 2004, 4, 1507–1511 CrossRef.
- M. Zhou, J. Guo, L. Guo and J. Bai, Anal. Chem., 2008, 80, 4642–4650 CrossRef CAS.
- S. B. Yoon, K. Sohn, J. Y. Kim, C. H. Shin, J. S. Yu and T. Hyeon, Adv. Mater., 2002, 14, 19–21 CrossRef CAS.
- R. Liu, S. M. Mahurin, C. Li, R. R. Unocic, J. C. Idrobo, H. Gao, S. J. Pennycook and S. Dai, Angew. Chem., Int. Ed., 2011, 50, 6799–6802 CrossRef CAS PubMed.
- K. T. Lee, J. C. Lytle, N. S. Ergang, S. M. Oh and A. Stein, Adv. Funct. Mater., 2005, 15, 547–556 CrossRef CAS.
- A. H. Lu, W. C. Li, G. P. Hao, B. Spliethoff, H. J. Bongard, B. B. Schaack and F. Schüth, Angew. Chem., Int. Ed., 2010, 49, 1615–1618 CrossRef CAS PubMed.
- B. Liu, H. Shioyama, T. Akita and Q. Xu, J. Am. Chem. Soc., 2008, 130, 5390–5391 CrossRef CAS PubMed.
- G. P. Keeley and M. E. G. Lyons, Int. J. Electrochem. Sci., 2009, 4, 794–809 CAS.
- P. Gai, H. Zhang, Y. Zhang, W. Liu, G. Zhu, X. Zhang and J. Chen, J. Mater. Chem. B, 2013, 1, 2742–2749 RSC.
- R. R. Kamath and M. J. Madou, Anal. Chem., 2014, 86, 2963–2971 CrossRef CAS PubMed.
- P. Veerakumar, R. Madhu, S. Chen, C. Hung, P. Tang, C. Wang and S. Liu, Analyst, 2014, 139, 4994–5000 RSC.
- E. O. Barnes, G. E. M. Lewis, S. E. C. Dale, F. Marken and R. G. Compton, Analyst, 2012, 137, 1068–1081 RSC.
- P. Pathirathna, R. J. Balla and S. Amemiya, Anal. Chem., 2018, 90, 11746–11750 CrossRef CAS.
- P. Pathirathna, R. J. Balla and S. Amemiya, J. Electrochem. Soc., 2018, 165, 3026–3032 CrossRef.
- E. M. Akinoglu, E. Kätelhön, J. Pampel, Z. Ban, M. Antonietti, R. G. Compton and M. Giersig, Carbon, 2018, 130, 768–774 CrossRef CAS.
- A. G. Zestos and B. J. Venton, J. Electrochem. Soc., 2018, 165, G3071–G3073 CrossRef CAS PubMed.
- C. P. Jones, K. Jurkschat, A. Crossley, R. G. Compton, B. L. Riehl and C. E. Banks, Langmuir, 2007, 23, 9501–9504 CrossRef CAS PubMed.
- R. N. Goyal, V. K. Gupta and S. Chatterjee, Sens. Actuators, B, 2010, 149, 252–258 CrossRef CAS.
- M. J. Sims, N. V Rees, E. J. F. Dickinson and R. G. Compton, Sens. Actuators, B, 2010, 144, 153–158 CrossRef CAS.
- M. C. Henstridge, E. J. F. Dickinson, M. Aslanoglu, C. Batchelor-mcauley and R. G. Compton, Sens. Actuators, B, 2010, 145, 417–427 CrossRef CAS.
- I. Streeter, G. G. Wildgoose, L. Shao and R. G. Compton, Sens. Actuators, B, 2008, 133, 462–466 CrossRef CAS.
- R. Prehn, L. Abad, D. Sánchez-molas, M. Duch, N. Sabaté, F. Javier, F. Xavier and R. G. Compton, J. Electroanal. Chem., 2011, 662, 361–370 CrossRef CAS.
- E. O. Barnes, X. Chen, P. Li and R. G. Compton, J. Electroanal. Chem., 2014, 720–721, 92–100 CrossRef CAS.
- Z. Ban, E. Kätelhön and R. G. Compton, J. Electroanal. Chem., 2016, 776, 25–33 CrossRef CAS.
- J. M. Goran, E. N. H. Phan, C. A. Favela and K. J. Stevenson, Anal. Chem., 2015, 87, 5989–5996 CrossRef CAS PubMed.
- A. E. Ross and B. J. Venton, Analyst, 2012, 137, 3045–3051 RSC.
- S. Sainio, T. Palomäki, S. Rhode, M. Kauppila, O. Pitkänen, T. Selkälä, G. Toth, M. Moram, K. Kordas, J. Koskinen and T. Laurila, Sens. Actuators, B, 2015, 211, 177–186 CrossRef CAS.
- B. E. K. Swamy and B. J. Venton, Anal. Chem., 2007, 79, 744–750 CrossRef CAS PubMed.
- J. Clausmeyer, W. Schuhmann and N. Plumeré, TrAC, Trends Anal. Chem., 2014, 58, 23–30 CrossRef CAS.
- Y. S. Singh, L. E. Sawarynski, P. D. Dabiri, W. R. Choi and A. M. Andrews, Anal. Chem., 2011, 83, 6658–6666 CrossRef CAS PubMed.
- L. R. Wilson, S. Panda, A. C. Schmidt and L. A. Sombers, Anal. Chem., 2018, 90, 888–895 CrossRef CAS PubMed.
- J. Wang, R. Trouillon, Y. Lin, M. I. Svensson and A. G. Ewing, Anal. Chem., 2013, 85, 5600–5608 CrossRef CAS PubMed.
- M. Zhang, K. Liu, L. Xiang, Y. Lin, L. Su and L. Mao, Anal. Chem., 2007, 79, 6559–6665 CrossRef CAS PubMed.
- X. Li, S. Majdi, J. Dunevall, H. Fathali and A. G. Ewing, Angew. Chem., Int. Ed., 2015, 54, 11978–11982 CrossRef CAS.
Footnote |
† These authors contributed equally to this work. |
|
This journal is © The Royal Society of Chemistry 2019 |