DOI:
10.1039/C8AY02416B
(Paper)
Anal. Methods, 2019,
11, 58-69
Solvent-free spectroscopic method for high-throughput, quantitative screening of fatty acids in yeast biomass
Received
6th November 2018
, Accepted 24th November 2018
First published on 26th November 2018
Abstract
Sustainable biofuels and bioproducts technologies are being developed by fermentation of sugars present and released from pretreated cellulosic biomass to lipids using oleaginous yeasts. Detailed analytical characterization of lipid content through cultivation under different scenarios not only is a bottleneck that slows down development of improved strains and processes, this process also creates significant chemical waste. Since lipids exhibit a dominant, distinct, and unique fingerprint in the NIR spectrum, the use of multivariate linear regression of respective wavelengths can be used for the prediction of intracellular lipid content present in the yeast biomass. We present data on the multivariate quantitative correlation of NIR spectra with measured lipid content in different oleaginous yeast strains. This work is the first demonstration of the rapid, non-destructive, lipid quantification on as little as 10 mg of yeast biomass in a 96-well format, preventing significant chemical pollution by applying a real-time monitoring process. We demonstrate a distinct correlation of lipid content with the accumulation of select fatty acids of the lipids for 5 different yeast species, among which, for S. cerevisiae and L. starkeyi, in-depth calibration curves were developed from 65 and 154 unique samples, respectively. We demonstrate that NIR spectra can be used to accurately predict intracellular lipid content using multivariate linear regression analysis in a manner of minutes, avoiding the need for lengthy chemical analyses that are resource intensive.
Introduction
The depletion of fossil resources and concomitant increase in atmospheric CO2 concentration has stimulated research and development towards the economic feasibility of sustainable and carbon neutral biofuels and bioproducts from biological feedstocks. The adoption of sustainable biobased fuels and products by society is a growing area of interest. Oleaginous yeast fermentation and optimization of single cell lipid accumulation is a critical area of research as an alternative to plant-based oils, with potentially much higher carbon conversion efficiencies. This growing area will benefit tremendously from the technology developed here.
The genetic engineering of oleaginous fungi for the production of lipid feedstocks for conversion to “drop-in” ready biofuels is hampered by the currently laborious and lengthy (often multiple days) methods for lipid content determination and often with considerable uncertainty in the measurements, when different methods are used.1–7 In addition, in some cases the traditional lipid analysis methods also require relatively large amounts of biomass (∼0.5 g) and are thus not applicable for screening large culture collections or identifying improved strains out of thousands of potential candidates. In some literature, micro-scale lipid analysis methods have been developed to use very small quantities (2–10 mg) of biomass,8,9 however, even with those methods, the procedures still use lipid-extraction solvents and can make the rapid high-throughput screening of 100's of samples difficult. Alternative methods include fluorescence tagging of lipid bodies in the cells with BODIPY, which then lends itself well for in vivo measurements of lipid accumulation,10 however, in our experience, fluorescence-based lipid measurements can be difficult to develop as absolute quantification methods, mainly because of species-specific effects of dye uptake and stability.
As an alternative to the labor-intensive chemical analyses, infrared spectroscopy, a non-destructive and high throughput approach, has been shown to be useful for the simultaneous prediction of lipid, protein, and carbohydrate content in algal biomass.11 Near-infrared (NIR) spectra are made up of dispersive overtones and combinations of molecular vibrations that give broad peaks from solid, opaque, and liquid samples requiring minimal preparation.12 Quantitative calibration models can be developed to accurately predict the concentration of specific biochemical components based on correlations between the NIR spectra and the known composition of a select sample set. Thus, with appropriate calibration models, rapid measurements can be made on the composition of new samples using only the spectra of the new samples.13,14
We have previously demonstrated the feasibility of NIR reflectance spectroscopy for quantitative determination of exogenously added and internally accumulated lipids in microalgal biomass.11,15 It was shown that accurate calibration models can be built based on NIR spectra solely correlated with increasing concentration of lipids indicating that lipids present within algal biomass have a sufficient and unique fingerprint in the NIR spectrum. An important additional finding was that NIR and mid-IR were able to distinguish between neutral and polar lipids (triglyceride vs. phosphatidylcholine lipids).11,15,16 Further, the use of near and mid-IR on microalgal and oleaginous yeast biomass has demonstrated a relationship between changes in IR spectra with changes in the cells' biochemistry based on calibration curves from either single wavenumbers or multivariate regression of specific spectral ranges.17–19 In the case of microbial biomass, often the amount of material is not sufficient to use in existing, more traditional, spectroscopy configurations, sometimes requiring over 1 g of material. To allow for spectroscopy on much smaller biomass quantities (∼10 mg), we developed a 96-well plate configuration for NIR spectroscopy for biomass from oleaginous yeasts.
Of particular importance, the use of NIR for lipid content estimation eliminates the use of hazardous substances typically used in the quantification of internal microbial lipids. Typical methods for Soxhlet lipid or in situ fatty acid methyl ester (FAME)3 extraction use substantial volumes of chloroform, methanol, hydrochloric acid, and hexane. This NIR technique and the reduced sample size necessary for accurate lipid content estimation vastly reduces the environmental impacts of microbial lipid-based biofuels research while allowing rapid lipid content estimation for immediate production improvements.
We selected the oleaginous yeasts Trichosporon oleaginosus, Lipomyces starkeyi, Rhodosporidium toruloides, Saccharomyces cerevisiae D5A20–25 and the oleaginous filamentous fungus Mucor circinelloides.26,27 From these 5 different species grown in both nitrogen replete and deplete conditions, we expect a sufficiently wide range of lipid accumulation from 5% to 65% lipids allowing for the calibration of our predictive lipid content model. In addition, the sample sets derived from these diverse species allow us to address the following questions arising from previous work and literature; can we discern the different yeast species based on the NIR spectra? Is the quality of the spectra and resulting prediction models from data collected in a 96-well plate format adequate for high-throughput lipid content measurement? How accurately can we predict the composition of new, independent samples? To our knowledge, this is the first report of the use of NIR for high-throughput quantification of lipid content in yeast biomass using a combined species prediction model with the inclusion of an independent validation test set of predicted lipid content.
Materials and methods
Yeast strains, media, and fermentation conditions
The yeasts T. oleaginosus ATCC 20509, L. starkeyi ATCC 12659 and NRRL Y-11557, R. toruloides ATCC 17902, and S. cerevisiae D5A24,25,28–30 were maintained in yeast peptone-dextrose (YPD) media (#Y1375, Sigma-Aldrich) at 30 °C and the fungus Mucor circinelloides ATCC 1216b was maintained on potato dextrose agar (#70139, Sigma-Aldrich) at 28 °C as described.31 Seed cultures were grown at 30 °C with shaking at 225 rpms in 100 mL of media in a 500 mL baffled flask. For lipid production, cultures were grown in 300 mL of yeast nitrogen base (YNB) media (#Y1251, Sigma-Aldrich) containing 5% glucose, glycerol, or xylose and 5 mM or 35 mM ammonium provided as (NH4)2SO4 in a 1 L baffled flask at 30 °C with shaking at 225 rpms in duplicate. Lipid production media was inoculated with washed cells from an overnight culture to an initial culture density measured as optical density at 600 nm (OD600) of 1. Due to extended lag phase and slow initial growth we typically encountered with L. starkeyi, seed cultures were allowed to grow for 2 days in YPD prior to inoculation in lipid accumulation medium. Media composition and C
:
N ratios for each lipid production growth experiments are available from the author upon request.
For NIR to lipid content correlation experiments, at each time point, 40 mL of culture was harvested by centrifugation, washed with 50 mL water, and the washed pellet were frozen at −80 °C for in situ lipid content and NIR spectral analysis.
Fermentations for validation of the lipid content predictions in a corn stover hydrolyzate (presented in Fig. 8)32,33 were performed in Sartorius BioStat Q-Plus fermentors (Bohemia, NY) at a 300 mL working volume using L. starkeyi (NRRL Y-11557). All of the fermentations were performed in batch mode using L. starkeyi taken from cell stock stored at −70 °C. For the inoculum, the seed medium consisted of YP media (10 g L−1 yeast extract, 20 g L−1 peptone) supplemented with 50 g L−1 glucose at pH 5.2. We inoculated 4 mL of L. starkeyi concentrated cell stock into 250 mL of seed medium in a 500 mL shake flask. We incubated the culture at 30 °C and 250 RPM agitation for 3 days. When the culture reached an optical density (OD600) of 9.2, the culture was then used to inoculate fermentors of 300 mL working volume at an initial OD600 of 0.9. The cells were concentrated and washed before inoculation into the fermentors. The fermentors contained filtered biomass sugars from either enzymatically hydrolyzed disc-refined low severity pretreated corn stover (F1 + F2), washed solids of deacetylated pretreated corn stover (F3 + F4), or deacetylated pretreated corn stover (F5 + F6), with the previously described composition,32,33 along with pure sugar controls in YNB media (F7 + F8). All fermentors were supplemented with 1 g L−1 yeast extract and 2 g L−1 peptone and performed in duplicate. The control fermentors were containing YNB media were also supplemented with 1 g L−1 yeast extract and 2 g L−1 peptone and contained 108 g L−1 total sugar (glucose and xylose) to match the level of total sugars in the hydrolysates. The fermentations were controlled at 30 °C, pH 5.2 with 4 N NaOH, 100 ccm airflow, and 25% partial pressure of oxygen (pO2) controlled by agitation. Hamilton (Reno, NV) OxyFerm FDA 120 O2 sensors were used to measure pO2 saturation. Progress of the fermentations was monitored by measuring fermentable sugar concentration by HPLC and ammonium utilization by YSI 7100, as described before.28,31 The fermentors were run for 90 hours, with time point samples taken at different intervals during fermentation for rapid lipid content assessment with NIR spectroscopy in addition to whole cell total lipid analysis (FAME method described below).
Lipid analysis
The lipid content and composition in yeast and fungal biomass was determined using the current best methods selected. In brief, lipids were determined as total FAME content via a direct, whole biomass transesterification reaction as described before.3 The procedure consisted of dissolving 10 mg of lyophilized biomass sample in 0.2 mL of chloroform
:
methanol (2
:
1, v/v), and subsequent transesterification of the lipids in situ with 0.3 mL of HCl
:
methanol (5%, w/v) for 1 h at 80 °C in the presence of 250 μg of tridecanoic acid (C13) methyl ester as an internal standard. The resulting FAMEs were extracted with hexane at room temperature for 1 h and analyzed by gas chromatography flame ionization detection (GC-FID) (Agilent 6890 N; DB-WAX 30 m 0.25 mm i.d. and 0.25 μm film thickness; temperature program 70–300 °C over 23 min at 10 °C min−1). Data were normalized to the internal standard (C13) and expressed on a dry cell weight basis (% FAME DCW) throughout this work.
NIR spectroscopy and data analysis
NIR spectra were collected on ∼10 mg freeze-dried biomass using an ASD LabSpec Pro (ASD Inc., Boulder, CO, USA) adapted to a 96-well format. Spectra were collected in solid white 96-well plates using an ASD LabSpec Pro spectrometer where empty wells were used for collecting reference spectra (baselining). Spectra were transformed from reflectance to absorbance (ln(1/R)) prior to any mathematical and spectral transformations.
All transformed NIR spectra were processed in R version 3.0.1 (ref. 34) and statistical analyses were carried out using the following packages: “chemometrics” version 1.3.8,35 “signal” version 0.7–1 and “pls” version 2.3–0 along with functions present in base R.36,37 Principal Component Analyses (PCA) were calculated using the singular value decomposition (SVD) algorithm. Partial Least Squares (PLS) regression analysis was used for quantitative correlation. For all models, PLS regression was performed using the NIPALS algorithm, using full, leave-one-out cross validation on a centered dataset. The optimum number of principal components used for the PLS regression is shown in the text accompanying the figures and was selected based on an apparent minimum in root mean squared error of the prediction (RMSEP) of the cross-validation of the models. In order to find the best calibration model, we investigated the effect of mathematical spectral pretreatment and spectral derivatives on the quality of the prediction model for NIR spectra including or excluding the visible region of the spectra (wavelengths 350–1100 nm). The algorithms we used were multiplicative scatter correction (MSC), standard normal variate (SNV) and Savitsky–Golay smoothing/derivatization of the spectra, as described before.11,15
The data, including spectra, cultivation media and conditions, lipid content (% FAME DCW), and fatty acid profile, which shows the relationship between growth conditions, lipid content, and fatty acid profile are available from the author upon request.
Results and discussion
For the 5 fungal species, S. cerevisiae D5A, C. curvatus, M. circinelloides, L. starkeyi, and R. toruloides, we measured the lipid content and fatty acid profile over a range of different physiological conditions including those induced from different media types such as corn stover hydrolysate and defined media (YNB) containing a high (35 mM NH4) versus low (5 mM NH4) nitrogen concentration and those induced from the relatively un-controlled environment of shake flasks to that of highly controlled fermentors. A high versus low relative nitrogen concentration was required to induce lipid production as oleaginous yeasts are well known for accumulating high amounts of lipids during nitrogen stress.20,38 A total of 252 cell biomass samples were collected and analyzed for total lipid content (% FAME DCW) and lipid profile. The distribution of the lipid content for each species is shown in Fig. 1. This dataset shows that the lipid concentration of these 289 samples spans a range of sufficient breadth (4–63% FAME DCW) necessary to build robust predictive models.11,39,40
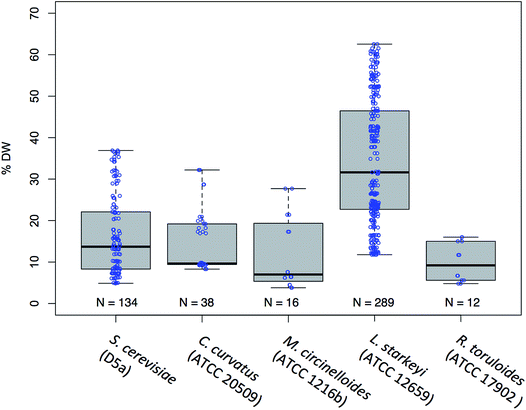 |
| Fig. 1 Range and distribution of lipid content data (% FAME DCW) obtained for 5 species of yeast used for multivariate model calibration shown as a Box-and-Whisker plot. The median value of the data sets are shown as a solid horizontal black line, the interquartile range (IQR) is shown as a box around the median value, with the ‘Whiskers’ indicating the values that fall within 1.5 IQR each point (open blue circles) represents individual measurements for S. cerevisiae (D5A); C. curvatus (ATCC 20509); M. circinelloides (ATCC 1216b); L. starkeyi (ATCC 12659); R. toruloides (ATCC 17902). | |
Profiling of fatty acids in oleaginous yeast species
In addition to the % FAME DCW, the lipid composition profiles (fatty acid profiles) were also collected from these 289 samples (Fig. 2A). Highly distinct lipid profiles were observed for each species with S. cerevisiae being the most different from the others. This is not surprising given that S. cerevisiae is not typically regarded as being oleaginous and was only recently found to accumulate greater than 20% lipids.24,30 Principal component analysis (PCA) of fatty acid profiles was carried out to check for distinctions in the fatty acid profile that underpins species-specific lipid profiles (Fig. 2B). The distinct grouping observed points to distinct profiles for all 5 species, with the biggest differences observed for S. cerevisiae D5A, explaining 75% of the variation seen in the data. The compositional and spectral variation that is found in these samples shows a highly distinct distribution of lipids between the different organisms. Fig. 2C shows impact of individual fatty acids on the distinctions seen in the PCA plot, PC1 (75%) mainly driven by C16:1n7 (negative) followed by minor contributions of C16:0, C18:2 and C18:1n9 and along PC2 (18.4%) driven by C18:2 and minor negative contributions by C16:0.
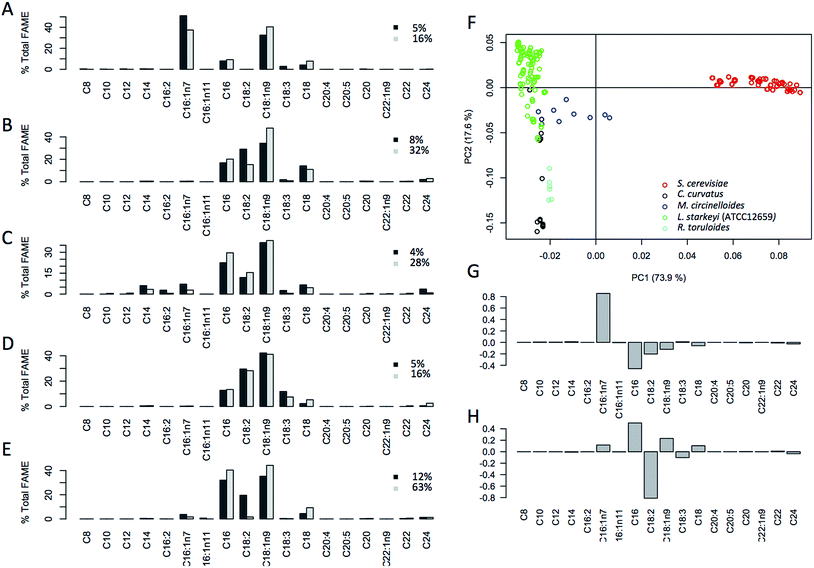 |
| Fig. 2 Summary of fatty acid profiles for 5 fungal species 5 species (a–e) illustration of fatty acid profiles for each species; (a) S. cerevisiae (D5A); (b) C. curvatus (ATCC 20509); (c) M. circinelloides (ATCC 1216b); (d) R. toruloides (ATCC 17902); (e) L. starkeyi (ATCC 12659); at two lipid accumulation levels (low and high, black and grey respectively, shown as % FAME DCW) illustrating fatty acid chain length rearrangement with lipid content increases. (f) Distribution and grouping of sample sets in a principal component analysis (PCA) based on each species fatty acid profile illustrating dominant features in the fatty acid profile specific for each species. Grouping along component 1 and component 2 driven by the fatty acids profile as shown in the loadings plot (g and h). | |
Spectroscopy in 96-well plate configuration
In addition to the in situ % FAME DCW data, we collected 4 replicate spectra from each sample in a 96-well plate with each well having ∼10 mg of biomass. We found that high-quality spectra could be obtained in the 96-well plate format with a fiberoptic probe; however, a reduction of the absorbance of the 2300–2500 nm region (and concomitant increase in the spectral noise levels) was observed due to light absorption by the fiber-optics. Visual differences in the biomass from the different strains are reflected in large spectral variation in the visible region as shown in Fig. 3, where typical spectra of a high and low lipid content biomass sample for each of the 5 species are shown. The spectra illustrate significant inter-species differences in the visible region of the spectrum (350–800 nm). When comparing the respective high and low-lipid spectra, it is clear that the same regions of the NIR spectrum are increasing with increased lipid content for all five species, with the largest changes found at 1215, 1725 and 2305 nm respectively. These observations are consistent with the spectral absorption bands associated with lipids found in the literature41 and is supported by the major absorbance from a triglyceride standard.11 The characteristic absorption bands of lipids in the NIR spectrum are (i) the first overtones of C–H stretching vibrations (1600–1900 nm), (ii) the region of second overtones of C–H stretching vibrations (1100–1250 nm) and (iii) two regions (2000–2350 nm and 1350–1500 nm) which contain bands due to combinations of C–H stretching vibrations and other vibrational modes.41 These regions are shown to vary the most in the L. starkeyi samples, in particular the relative changes observed around 1215 nm, 1725 nm, and 2305 nm between the spectra corresponding to the low and high lipid content biomass (ranging from 18.4 to 62.6% FAME DCW, Fig. 3). The spectra from M. circinelloides appears to be distinct between the high and low lipid samples, however, the difference is mostly related to the offset in absolute absorbance between the spectra, rather than wavelength-specific variation and this difference would mostly be normalized after spectral pretreatment as described above.
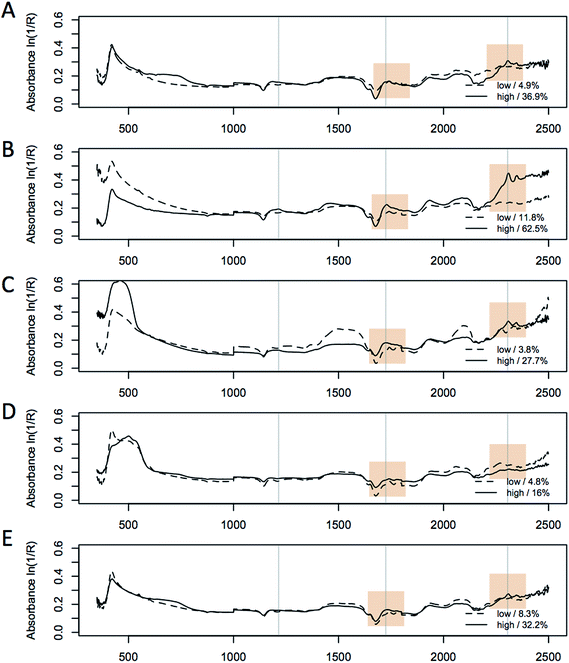 |
| Fig. 3 Overlay of spectra of high (solid line) and low (dashed line) lipid containing samples for 5 species (a–e); S. cerevisiae (D5A); L. starkeyi (ATCC 12659), M. circinelloides (ATCC 1216b); R. toruloides (ATCC 17902); C. curvatus (ATCC 20509), collected in a 96-well plate format. The spectra were normalized prior to plotting using the multiplicative scatter correction (MSC) algorithm. Selected lipid-responsive wavelengths in the spectra are highlighted that correspond to the main lipid overtones at, 1215 nm, 1725 nm, and 2305 nm. | |
Principal component analysis of spectra
To investigate structure in the data set and identify the major variation contributions, we performed PCA on the spectra. Fig. 4 shows the major spectral variation for the species investigated for both raw spectra and scatter-corrected spectra (standard normal variate, SNV). No distinct grouping by species was observed either in the raw or the pretreated spectra, supporting the potential for combining all spectra into one dataset for the potential cross-species prediction of lipid content. This indicates that the highly distinct fatty acid profiles do not necessarily translate into large spectral variation. It is likely that the similar chain lengths of the fatty acids measured (predominantly C16 and C18 fatty acids) also dominate the spectral absorbances.
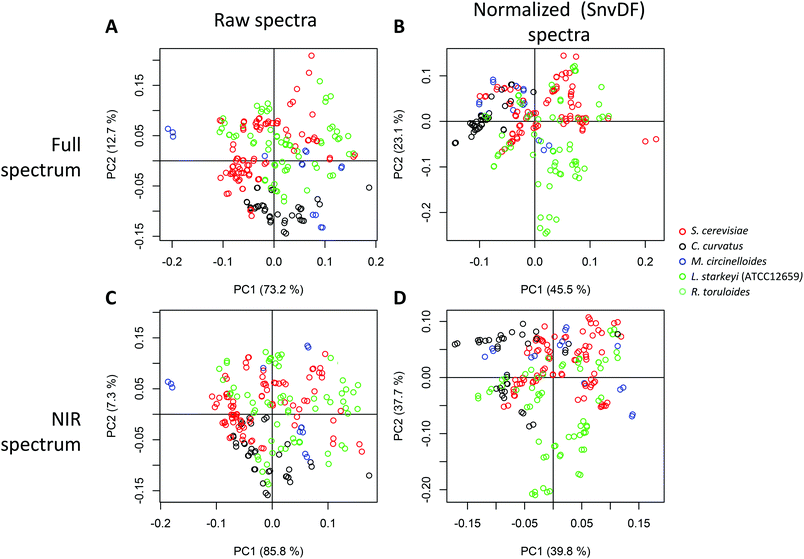 |
| Fig. 4 Analysis of variability of the spectra relating to the different species and impact of spectral normalization algorithms. Principal component analysis of 96-well plate collected full Vis-NIR spectra, colored by species, before (a–c) and after (b–d) spectral normalization and using the full (Vis-NIR) (a and b) or truncated (only NIR region, 1100–2500 nm) spectra (c and d). | |
The contribution of the spectral variation after normalization (SNV) follows a different pattern, with PC2 indicating a higher contribution from L. starkeyi biomass, which, as the highest lipid content species, could indicate the influence of composition impacting the spectral fingerprints (by at least 23%, as measured by the variability explained by PC2). This may indicate an advantage of performing mathematical pretreatment prior to multivariate analysis of spectra, in particular when large spectral variation is present and could interfere with a species-agnostic prediction model. The effect of the visible region was not noticeable in that the principal component-based groupings observed were conserved with or without the visible region, indicating that the interspecies differences in the visible region of the spectra may not significantly influence the IR region.
Partial least square regression
We used PLS multivariate regression analysis to develop quantitative predictive models of lipid content. For the purpose of demonstrating the quality of the predictions, we built general multiple-species models, as well as strain-specific prediction models. The quality of each of the three models is shown in Fig. 5 showing predicted-versus-measured plots, root mean squared error of the prediction (RMSEP) and the regression coefficients of the calibration and validation data for the lipid content of the combined 5-species model (total of 252 unique samples, 489 spectra). These models in general needed three principal components to achieve the high quality (R2 > 0.9) of model validation shown as the predicted versus measured plot of the leave-one-out full cross-validation (Fig. 5A, 6A and 7A).
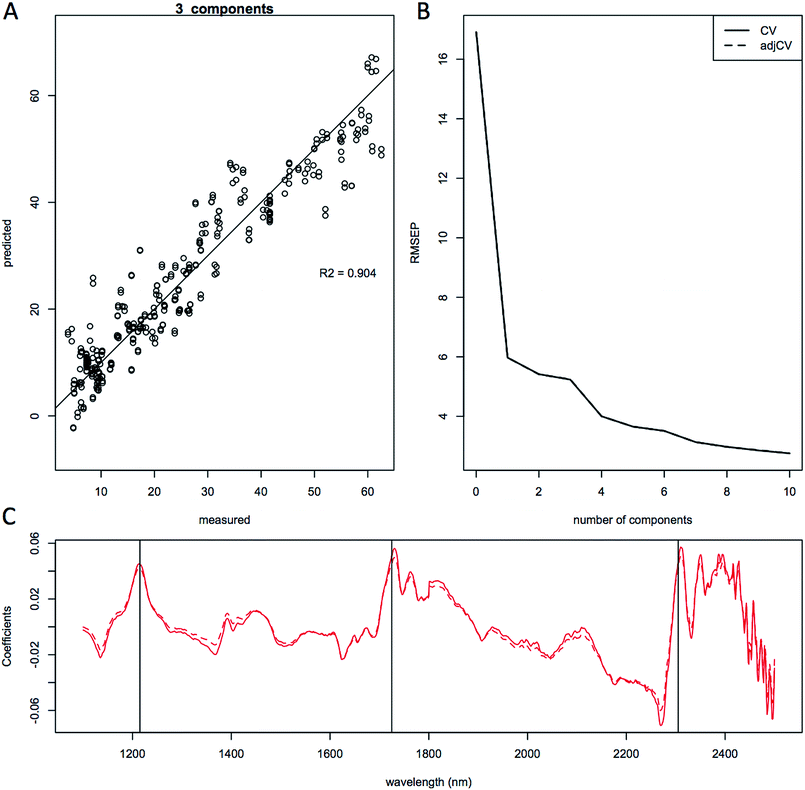 |
| Fig. 5 Quantitative prediction of lipid content using combined species model. Partial least squares modeling results using 3 principal components of lipid content for the entire complete data set (489 spectra from 5 species). Results are shown as; (a), predicted vs. measured plot showing the cross validation correlation for lipid content; (b), root mean squared error of the prediction (RMEP) plot, (c) regression coefficients plot. Spectra were smoothed and normalized using a standard normal variate correction (SNV) prior to modeling. Model quality: 3 principal components, r2 = 0.904 and RME CV = 5.23% FAME DCW. | |
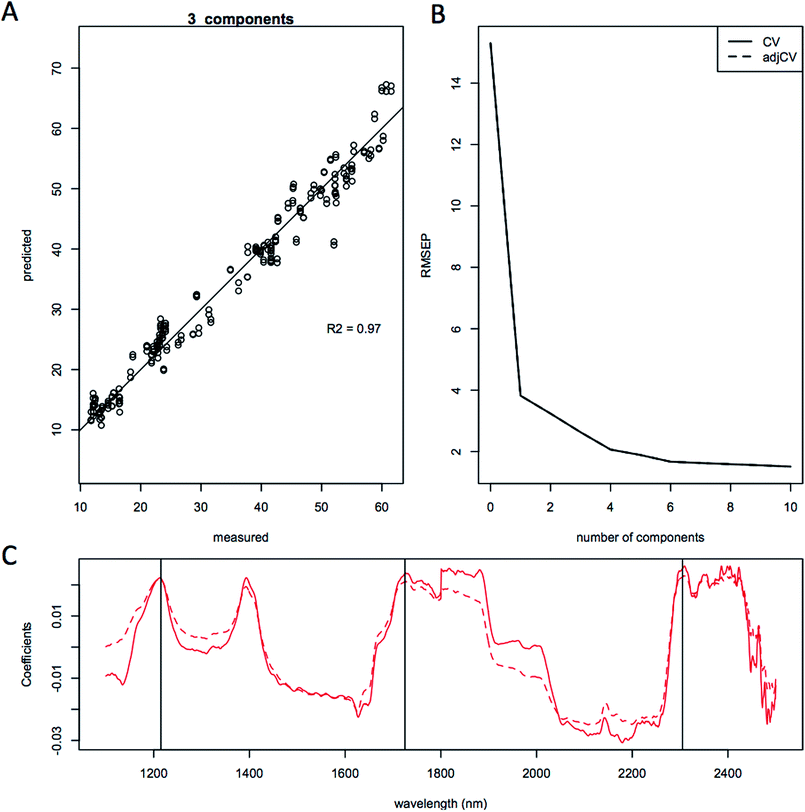 |
| Fig. 6 Quantitative prediction of lipid content using only L. starkeyi (ATCC 12659) model. Partial least squares modeling results using 3 principal components for lipid content of L. starkeyi samples (289 spectra on 154 samples). Results are shown as; (a), predicted vs. measured showing the cross validation correlation for lipid content; (b), root mean squared error of the prediction (RMEP), calculated as 2.63%; (c), regression coefficients. Spectra were smoothed and normalized using a standard normal variate correction (SNV) prior to modeling. | |
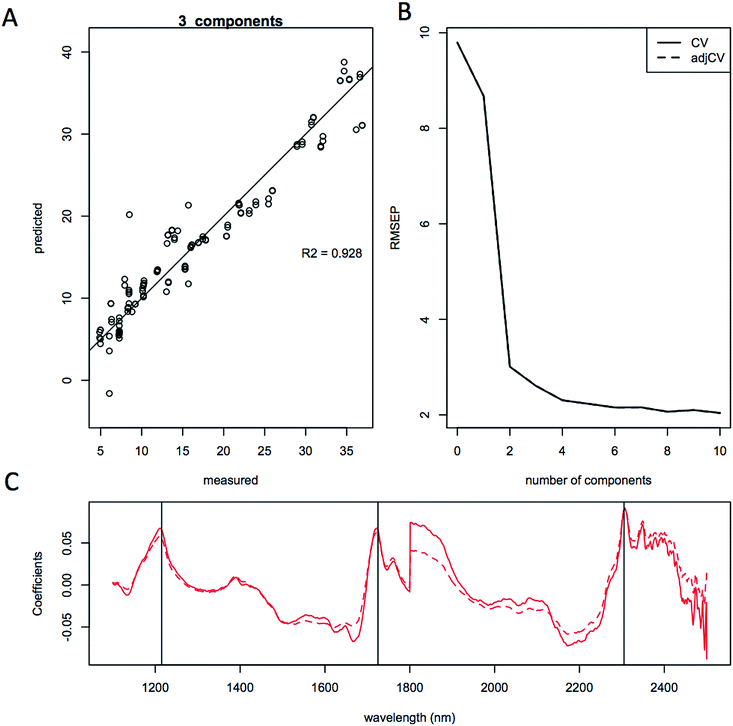 |
| Fig. 7 Quantitative prediction of lipid content using only S. cerevisiae model. Partial least squares modeling results using 3 principal components of lipid content for only S. cerevisiae (D5A) samples (134 spectra). Results are shown as; (a), predicted vs. measured showing the cross validation correlation for lipid content; (b), root mean squared error of the prediction (RMEP); (c), regression coefficients. Spectra were smoothed and normalized using a standard normal variate correction (SNV) prior to modeling. | |
For the development of the prediction model presented here we have removed the visible region from the spectra specifically for building the prediction models (i.e. only using 1100 to 2500 nm) to avoid competing interference from pigments present in some yeasts.42 We left the noisy 2400–2500 nm region in the spectra to reduce the risk of cutting out any lipid-specific information from this region, since 2300 nm is one of the major lipid-responsive wavelengths. In addition to spectral wavelength selection, we also explored mathematical transformation of NIR spectra prior to building partial least squares multivariate calibration models to help improve the predictions and subtract scatter and other spectral variations not related to the composition of the biomass. A prerequisite for the robustness of NIR models for predicting composition is that the range in compositional variability of the component of interest needs to be sufficiently large to allow for predictions across species and for regression algorithms to subtract the orthogonal variation from the spectra. With a limited concentration range of predicted components, the data set will likely not be equally distributed, the quality of the models will be reduced, and it will become more difficult to find a linear correlation in component concentrations.18 A sufficient range for building calibration models depends on both the absolute range of values for a given constituent and on the precision of the primary measurements with the ratio of the constituent concentration range to the precision of the primary measurement being a better metric then either of these parameters alone.
Plots of the RMSEP relative to the number of components or latent variables used in the models are shown in Fig. 5B, 6B and 7B and does not show a clear minimum, but a change in slope can be observed at around 3 components, which is what was used for the quantitative linear regressions. The effect of different spectral pretreatments, such as trimming the spectra to only include the NIR region or mathematical pretreatment, standard normal variate (SNV), spectral smoothing, and Savitzky–Golay derivatization43 to remove scatter due to different particle sizes and species-specific features is typically scored based on the RMSEP and R2 values39 and the number of principal components needed to build the regression model. The use of fewer principal components typically gives more robust models since less noise is being included in the fitting algorithm. We performed multiple mathematical spectral pretreatments and found that an SNV correction where the sum squared deviation over the spectrum equals unity gives the best models thanks to the removal of the species specific spectral fingerprints.
The quality of the models when selecting individual species improved significantly relative to the mixed species model, as indicated by the correlation coefficient of the predicted versus measured agreement; R2 = 0.904 for the mixed model, whereas the individual models for L. starkeyi and S. cerevisiae (D5A) have correlation coefficients of 0.970 and 0.928 respectively (Fig. 5–7). We observed the same lipid-specific spectral regions are driving the quantitative predictions as those shown in Fig. 2 (1215 nm, 1725 nm, and 2305 nm) (Fig. 5–7C). The precision of the predictions with the combined model suffers relative to a single species prediction model with the addition of variable species (reflected in Fig. 5A) but can still achieve a prediction accuracy of ±6% FAME DCW content for the validation model (root mean square error of prediction, RMSEP, Fig. 5B). For most applications of rapid high-throughput screening, this precision is adequate. For a more detailed screen for promising candidate organisms, a species-specific model may need to be developed. For the work presented here, for the species other than S. cerevisiae and L. starkeyi, the available samples and quantitative lipid data was not sufficient to develop respective single-species prediction models. We did not include the preliminary models for the additional species because they do not represent the potential accuracy of models that could be built with more samples and a larger range of lipid content.
Being able to rapidly assess the lipid content present in the biomass during an experiment or fermentation allows for the timely adjustment of conditions to further increase lipid content and can greatly help with developing improvements in fermentation technology or rapidly screen different substrates used for yeast fermentations. In a test of the accuracy and precision of the quantitative prediction models, we validated the individual L. starkeyi model by sampling during a controlled fermentation experiment. For this experiment, yeast biomass was grown in filtered liquors from different pretreatments of corn stover and the lipid content of the yeast biomass was measured both directly as % FAME DCW and predicted using the L. starkeyi species-specific model (Fig. 6). The lipid content data illustrates small differences between the measured and predicted values (Fig. 8). Of the 32 predicted values, 7 predicted measurements exceeded 10% relative percent difference with a maximum deviation from the measured values of 15% supporting the use of NIR screening as a rapid tool to track lipid accumulation in ongoing experiments. In addition, by testing our species-specific NIR lipid prediction model on a different strain of the same species grown on substrates other than substrates the model was based on, validates the use of NIR lipid prediction modeling for use as a strain and growth substrate agnostic method for rapidly measuring lipid content.
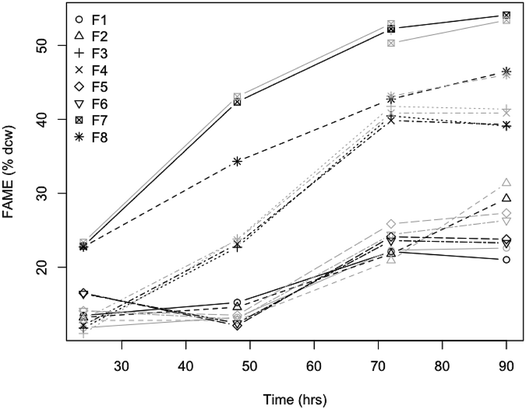 |
| Fig. 8 Validation of NIR lipid content prediction model for L. starkeyi fermentation. Pretreated corn stover liquors were fermented with L. starkeyi NRRL Y-11557 and lipid content was measured directly as % FAME DCW or predicted with the L. starkeyi species-specific NIR model (black and grey symbols respectively) over 80 h of fermentation. The designation F1 − F8 represent different media formulations; filtered liquor from enzymatically hydrolyzed material from either disc-refined low severity pretreated corn stover (F1 + F2), washed solids of deacetylated pretreated corn stover (F3 + F4), or deacetylated pretreated corn stover (F5 + F6), pure sugar controls (F7 + F8). | |
Conclusions
We demonstrated for the first time a fully quantitative correlation between NIR spectra and measured lipid content in fungal biomass. There are large influences of inter-species differences in the visible and NIR portions of the spectrum; however, spectral transformation functions could partly reduce this effect and aid with further multivariate analyses. Our work suggests that regression models can be used based on the measured lipid content found in fungal biomass. The 96-well, high-throughput, NIR approach presented here shows that we can obtain accurate independent predictions from a dataset consisting of 252 biomass samples and, together with the application of multiple linear regression analysis, allows for a much improved and increased throughput of lipid content analysis. A fully integrated high-throughput approach could involve cultivation of yeast in a 96-well plate format followed by quantitative NIR spectroscopic prediction of the composition. This technology was applied to the near real time monitoring of lipid-producing yeast fermentations and shows a prediction accuracy that is adequate for rapid, non-destructive screening useful in fermentation optimization.
In conclusion, the methodology reported here will likely have wide general appeal across biofuels and biochemical research as a solvent-free, rapid, sustainable, green methodology for the accurate estimation of microbial lipids. Our approach is an innovative application of a currently well-developed technology to establish an environmentally friendly methodology applicable to research and development as well as having industrial applications for near real time monitoring of industrial microbial lipid production. In future development, an in situ measurement during cultivation could be adapted, while optimizing the spectroscopy to be applicable in high-moisture environments, and the feasibility demonstration reported in this manuscript can become a great starting point for future work.
Conflicts of interest
The authors have no conflicts to declare.
Competing interests
The authors declare that they have no competing interests.
Authors' contribution
LL and MZ devised the strategy and came up with the experimental design of the study. LL carried out the spectroscopic modeling, data analysis, and wrote the manuscript. EK carried out a subset of the yeast fermentations. SV carried out the lipid analysis. HR and ND carried out a subset of the fermentations and supplied the samples for the final validation experiments of L. starkeyi grown on corn stover hydrolyzate. All authors read and approved the final manuscript.
Abbreviations
YPD | Yeast peptone dextrose |
YNB | Yeast nitrogen base |
OD | Optical density |
NIR | Near-infrared |
PLS | Partial least squares regression |
RMSEP | Root mean squared error of prediction |
MSC | Multiplicative scatter correction |
SNV | Standard normal variate |
PCA | Principal component analysis |
FAME | Fatty acid methyl ester |
DCW | Dry cell weight |
GC-FID | Gas chromatography flame ionization detection |
Acknowledgements
The authors would like to thank Wei Wang and Hui Wei for M. circinelloides biomass, Andrew Lowell for L. starkeyi biomass from corn stover fermentation experiments, John Yarbrough for help with initial cultivation and helpful discussions, Robert Sebag for help in collecting the spectra. The NREL authors thank the U.S. Department of Energy (DOE) Energy Efficiency and Renewable Energy (EERE) Bioenergy Technologies Office (BETO) for funding this work via Contract No. DE-AC36-08GO28308 with NREL. The U.S. Government retains and the publisher, by accepting the article for publication, acknowledges that the U.S. Government retains a nonexclusive, paid up, irrevocable, worldwide license to publish or reproduce the published form of this work, or allow others to do so, for U.S. Government purposes.
References
- J. Folch, M. Lees and G. H. Sloane-Stanley, J. Biol. Chem., 1957, 226, 497–509 CAS.
- S. J. Iverson, S. L. C. Lang and M. H. Cooper, Lipids, 2001, 36, 1283–1287 CrossRef CAS.
- L. Laurens, M. Quinn, S. Van Wychen, D. Templeton and E. J. Wolfrum, Anal. Bioanal. Chem., 2012, 403, 167–178 CrossRef CAS.
- T. Schneider, S. Graeff-Hönninger, W. T. French, R. Hernandez, N. Merkt, W. Claupein, M. Hetrick and P. Pham, Energy, 2013, 61, 34–43 CrossRef CAS.
- R. Schneiter and G. Daum, Methods Mol. Biol., 2006, 313, 75–84 CAS.
- X. L. Gual, I. Riezman, M. R. Wenk and H. Riezman, Methods Enzymol., 2010, 470, 369–391 Search PubMed.
- C. S. Ejsing, J. L. Sampaio, V. Surendranath, E. Duchoslav, K. Ekroos, R. W. Klemm, K. Simons and A. Shevchenko, Proc. Natl. Acad. Sci. U. S. A., 2009, 106, 2136–2141 CrossRef CAS.
- K. Qiao, T. M. Wasylenko, K. Zhou, P. Xu and G. Stephanopoulos, Nat. Biotechnol., 2017, 35, 173–177 CrossRef CAS PubMed.
- K. Qiao, S. Hussain, I. Abidi, H. Liu, H. Zhang, S. Chakraborty, N. Watson, P. Kumaran and G. Stephanopoulos, Metab. Eng., 2015, 29, 56–65 CrossRef CAS PubMed.
- A. Back, T. Rossignol, F. Krier, J. M. Nicaud and P. Dhulster, Microb. Cell Fact., 2016, 5, 147 CrossRef.
- L. M. L. Laurens and E. J. Wolfrum, J. Agric. Food Chem., 2013, 61, 12307–12314 CrossRef CAS.
-
D. A. Burns and E. W. Ciurczak, Handbook of near-infrared analysis, Marcel Dekker, New York, 2001 Search PubMed.
-
T. Naes, T. Isaksson, T. Fearn and T. Davies, in A user-friendly guide to multivariate calibration and classifications, NIR publications, Chichester, UK, 2002 Search PubMed.
-
H. Martens and T. Naes, Multivariate calibration, John Wiley, New York, 1989 Search PubMed.
- L. M. L. Laurens and E. J. Wolfrum, BioEnergy Res., 2010, 4, 22–35 CrossRef.
- C. J. Hirschmugl, Z. E. Bayarri, M. Bunta, J. B. Holt and M. Giordano, Infrared Phys. Technol., 2006, 49, 57–63 CrossRef CAS.
- H. Wagner, Z. Liu, U. Langner, K. Stehfest and C. Wilhelm, J. Biophotonics, 2010, 3, 557–566 CrossRef CAS.
- W. Mulbry, J. Reeves, Y. Liu, Z. Ruan and W. Liao, J. Appl. Phycol., 2012, 24, 1261–1267 CrossRef CAS.
- D. Ami, R. Posteri, P. Mereghetti, D. Porro, S. M. Doglia and P. Branduardi, Biotechnol. Biofuels, 2014, 7, 1–14 Search PubMed.
- J. M. Ageitos, J. A. Vallejo, P. Veiga-Crespo and T. G. Villa, Appl. Microbiol. Biotechnol., 2011, 90, 1219–1227 CrossRef CAS.
- I. R. Sitepu, L. a. Garay, R. Sestric, D. Levin, D. E. Block, J. Bruce German and K. L. Boundy-Mills, Biotechnol. Adv., 2014, 32, 1336–1360 CrossRef CAS.
- X. Meng, J. Yang, X. Xu, L. Zhang, Q. Nie and M. Xian, Renewable Energy, 2009, 34, 1–5 CrossRef CAS.
- C. Ratledge, Biochem. Soc. Trans., 2002, 30, 1047–1050 CrossRef CAS.
- E. P. Knoshaug, S. Van Wychen, A. Singh and M. Zhang, Biofuel Res. J., 2018, 5, 800–805 CrossRef.
- Q. He, Y. Yang, S. Yang, B. S. Donohoe, S. Van Wychen, M. Zhang, M. E. Himmel and E. P. Knoshaug, Biotechnol. Biofuels, 2018, 1–20 Search PubMed.
- H. Wei, W. Wang, J. M. Yarbrough, J. O. Baker, L. Laurens, S. Van Wychen, X. Chen, L. E. Taylor, Q. Xu, M. E. Himmel and M. Zhang, PLoS One, 2013, 8, e71068 CrossRef CAS.
- C. Xia, J. Zhang, W. Zhang and B. Hu, Biotechnol. Biofuels, 2011, 4, 15 CrossRef.
- D. D. Spindler, C. E. Wyman, A. Mohagheghi and K. Grohmann, Appl. Biochem. Biotechnol., 1988, 17, 279–293 CrossRef CAS.
- R. B. Bailey, T. Benitez and A. Woodard, Appl. Environ. Microbiol., 1982, 44, 631–639 CAS.
- Y. Kamisaka, K. Kimura, H. Uemura and M. Yamaoka, Appl. Microbiol. Biotechnol., 2013, 97, 7345–7355 CrossRef CAS.
- H. Wei, W. Wang, J. M. Yarbrough, J. O. Baker, L. Laurens, S. van Wychen, X. Chen, L. E. Taylor, Q. Xu, M. E. Himmel and M. Zhang, PLoS One, 2013, 8, 1–12 CrossRef.
- J. Shekiro III, E. M. Kuhn, N. J. Nagle, M. P. Tucker, R. T. Elander and D. J. Schell, Biotechnol. Biofuels, 2014, 7, 23 CrossRef.
- N. D. Weiss, N. J. Nagle, M. P. Tucker and R. T. Elander, Appl. Biochem. Biotechnol., 2009, 155, 418–428 CrossRef CAS.
-
R Development Core Team, R: A language and environment for statistical computing, R Foundation for Statistical Computing, Vienna, Austria, 2013, http://www.R-project.org Search PubMed.
-
P. Filzmoser and K. Varmuza, Chemometrics: Multivariate Statistical Analysis in Chemometrics, R Package version 1.3.8, 2012 Search PubMed.
-
Signal developers, Signal: Signal Processing, R package version 0.7-6, 2013 Search PubMed.
-
B. H. Mevik, R. Wehrens, K. H. Liland, Pls: Partial Least Squares and Principal Component Regression, R Package version 2.3-0, 2011 Search PubMed.
- I. R. Sitepu, L. a. Garay, R. Sestric, D. Levin, D. E. Block, J. Bruce German and K. L. Boundy-Mills, Biotechnol. Adv., 2014, 32, 1336–1360 CrossRef CAS.
-
K. H. Esbensen, Multivariate Data Analysis – in practice: an introduction to multivariate data analysis and experimental design, CAMO Process AS, Oslo, Norway, 2002 Search PubMed.
-
H. Martens and M. Martens, Multivariate analysis of quality: an introduction, John Wiley, New York, 2001 Search PubMed.
-
A. A. Ismail, A. Nicodemo, J. Sedman, F. R. van de Voort and I. E. Holzbaur, in Spectral properties of lipids, ed. R. J. Hamilton and J. Cast, CRC Press LLC, Boca Raton, FL, 1999 Search PubMed.
- L. C. Mata-Gómez, J. C. Montañez, A. Méndez-Zavala and C. N. Aguilar, Microb. Cell Fact., 2014, 13, 12 CrossRef.
- A. Savitzky and M. J. E. Golay, Anal. Chem., 1964, 36, 1627–1639 CrossRef CAS.
|
This journal is © The Royal Society of Chemistry 2019 |
Click here to see how this site uses Cookies. View our privacy policy here.