DOI:
10.1039/C9AN00107G
(Communication)
Analyst, 2019,
144, 4488-4492
High-throughput screening Raman microspectroscopy for assessment of drug-induced changes in diatom cells
Received
16th January 2019
, Accepted 22nd June 2019
First published on 9th July 2019
Abstract
High-throughput screening Raman spectroscopy (HTS-RS) with automated localization algorithms offers unsurpassed speed and sensitivity to investigate the effect of dithiothreitol on the diatom Phaedactylum tricornutum. The HTS-RS capability that was demonstrated for this model system can be transferred to unmet analytical applications such as kinetic in vivo studies of microalgal assemblages.
1. Introduction
Monitoring microalgal assemblages both in their natural environment and laboratory systems has become a routine process in environmental science and pollution research as well as in biofuel and biomass production.1,2 Analytical methodologies for high-throughput screening (HTS) of microalgae often use enzyme immunoassay-based analyses of bulk samples or fluorescence detection of stained single cells in microfluidic devices.3,4 Raman microspectroscopy provides specific molecular information on single cells label-free and non-invasively such as direct, quantitative in vivo lipid profiling of oil producing microalgae.5 Contributions of carotenoid and chlorophyll (Chl) molecules dominate in Raman spectra of microalgal cells at visible excitation wavelengths due to resonance enhancement. Raman spectroscopy was applied for in vivo pigment analysis of microalgae,6 assessment of growth stages7 and their respective nutritional status.8 Wang et al. recently demonstrated Raman-activated droplet sorting of Haematococcus pluvialis in a microfluidic device.9 Their system probed astaxanthin (AXT) levels at a rate of ∼260 cells per min, and subsequently isolated AXT-hyperproducing cells. In the field of high-throughput microalgal lipidomics, Kim et al.10 established droplet microfluidic cultures of Chlamydomonas reinhardtii on a poly(dimethylsiloxane) chip. Upon varying the nitrogen concentration in the medium, Raman spectra revealed elevated lipid production in cell cultures with higher nitrogen level which was positively correlated with Nile red staining. Non-linear multiphoton modalities like coherent anti-Stokes Raman scattering (CARS) and two photon excited fluorescence (TPEF) were coupled to a laser scanning microscope to assess a large number of individual microalgal cells. The change in carotenoid content in diatoms under different light cycles was analyzed by tuning the CARS emission to 1528 cm−1 and Chl distribution was visualized by TPEF at 850 nm.11 Recently, a HTS-Raman spectroscopy (HTS-RS) platform was developed to identify more than 100
000 single eukaryotic cells.12 The current work adapted this platform to detect drug- and light-induced changes in pigment composition of individual living Phaedactylum tricornutum cells. The effect of dithiothreitol (DTT) on the photosynthetic apparatus of diatom cells served as model system. DTT inhibits the enzymatically catalyzed reaction of the intracellular photoprotective xanthophyll cycle which is the interconversion of diadinoxanthin (Ddx) into its de-epoxidized form diatoxanthin (Dtx) (Fig. 1). This interconversion is enforced in situations when the microalgal cells are exposed to high light irradiance. As a consequence the formation of Dtx leads to dissipation of excess energy by non-photochemical quenching of excited states in the photosystem as well as the capture of harmful singlet oxygen species. Several groups studied this process by high performance liquid chromatography (HPLC) on pooled cells.13,14
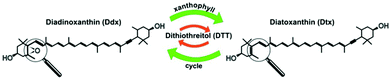 |
| Fig. 1 The xanthophyll cycle in diatoms is the interconversion of diadinoxanthin (Ddx) in its de-epoxidized analogue diatoxanthin (Dtx) which serves as an intracellular photoprotective species. In the presence of dithiothreitol (DTT) this de-epoxidation reaction is inhibited. | |
2. Experimental
2.1 Cultivation of P. tricornutum
A single batch of P. tricornutum (strain CCMP 2561) was cultivated in a 50 ml culture flask (Greiner, Germany) filled with 15 ml of sterile filtered artificial seawater medium (ASW) as described in Maier et al.15 Microalgal cells were kept in a homebuilt cultivation chamber at a constant temperature of 18 °C, a 14/10 hours light/dark cycle and an illuminance of 1000 lx by fluorescence tubes (Osram T5 36 W640). The cell density was controlled by manual counting using a Fuchs-Rosenthal hemocytometer. Spectroscopic experiments were started when the culture entered stationary phase with a cell density of 5 × 106 cells per ml. Then, the cell culture was split evenly into three batches of 5 ml. A DTT solution (Roth, Germany) in 200 μl ASW was added to one batch, resulting in a final DTT concentration of 8.64 mM. The DTT treated batch (HL+) as well as an untreated batch (HL−) were both exposed to an illuminance of 2000 lx, whereas the control batch was kept at 1000 lx.
2.2 Raman measurements
A custom-build upright Raman microscope was previously described in detail.12 Briefly, a 785 nm single mode laser (Xtra, Toptica, Germany) was used for excitation. A clean-up filter (785 ± 1.5 nm) removes background contributions generated in the single mode delivery fiber. Because the beam underfills the objective lens, the beam diameter in the sample plane is expanded, covering an area of roughly 10 μm2. The sample holder was mounted on two motorized stages for x–y translation (CONEX MFA-Series; Newport, USA) and another stage for z-positioning stage (MTS25-Z8, Thorlabs, USA). After passing a notch filter (785 ± 19 nm, Laser Components, Germany) a multi-mode fiber guides the Raman scattered light to a spectrometer (Isoplane 160, Princeton Instruments, USA) which is equipped with a grating of 400 groves per mm and a CCD detector (PIXIS-400 BR-eXcelon, Princeton Instruments, USA). For brightfield microscopy, the sample is illuminated by a white light LED and detected on a CCD camera (DCC1645C, Thorlabs, Germany). The setup is controlled by in-house written software under LabView (National Instruments, USA).
Raman spectra were measured from 18 culture conditions within a period of 8 hours by pipetting 40 ml of cell suspension on CaF2 slides immersed in distilled water. In order to avoid photo-damage the laser intensity was 30 mW at the sample plane, exposure time was 100 ms, and a 60×/1.0 water immersion objective lens (Nikon) was used. In total, Raman spectra of 16
888 individual P. tricornutum cells were recorded. The acquisition of such large number of measurements in a relatively short time would be very challenging with a traditional Raman data acquisition approach.
2.3 Data processing
Raman spectra were pre-processed and analyzed using the hyperSpec package16 in R. The spectral range was limited to 650–1800 cm−1. In order to increase the signal-to-noise ratio all spectra of the same time point were reconstructed by singular-value decomposition using the first 20 components. Baseline was corrected following an extended multiplicative scattering correction (EMSC) approach to eliminate spectral contributions from water and autofluorescence of Chl. To ensure sufficient spectral quality, spectra with high fluorescence and without pigment information were rejected by means of a respective Pearson Correlation coefficient below 0.97 related to a reference spectrum from P. tricornutum leaving 3139 cells with at least 170 cells per time point for subsequent analysis. Resulting spectra were area-normalized in the wavenumber range from 650–1800 cm−1. Subsequently, a partial least squares linear discriminant analysis (PLS-LDA) model was trained with a subset of spectra using the plslda function from the cbmodels package.17
3. Results and discussion
3.1 Automated cell localization algorithm
An overview of the cell localization algorithm is depicted for a single frame in the flowchart (Fig. 2a). A mosaic of multiple frames was collected for each condition. Due to the different shape of diatoms compared to blood cells, the algorithm needed substantial modification.12 First, the captured bright field image is converted to a grayscale image (Fig. 2b) which corresponds to the image obtained from the microscope objective lens. The grayscale image has pixels with 8 bit unsigned integer values ranging from 0 to 255. The bright field image was acquired using inhomogeneous LED illumination. The inhomogeneous background in the grayscale image was estimated by a smoothing convolution filter with a kernel defined as,
where aij is a constant and has a value of 1 generally. Here the kernel size was 50 × 50.
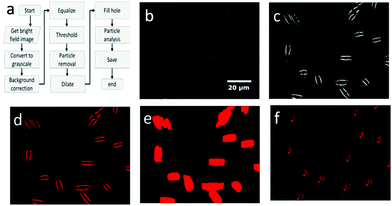 |
| Fig. 2 The image processing algorithm to detect algae (a) consists of several steps and the outcome from several steps are depicted here i.e., grayscale image (b), image after equalization (c), threshold image (d), image after dilation (e) and the image after particle analysis (f). All images correspond to a single field of view. | |
The effect of inhomogeneous illumination is removed by subtracting the estimated background from the grayscale image. This background corrected image is free from the inhomogeneous illumination contribution, but it suffers from low pixel values. To enhance the image contrast as well as intensity an equalize function is applied (Fig. 2c) which generally scales a certain pixel value to the whole pixel value range, in this case pixel values from 7 to 55 are scaled to values from 0 to 255. The values below 7 are responsible for the noise contribution and the values above 55 do not change the image quality significantly. The threshold operation is needed to detect the particles in the image which converts the equalized image to a binary image. If the equalized image pixel values are below a certain level or threshold value, in this case 55, the corresponding pixel value is assigned to zero. If the pixel values are above the threshold, it is assigned to one. The binary image resulting from the threshold operation (Fig. 2d) clearly distinguishes the particles from the background and each microalga is detected as two lines. The two lines of each alga could be avoided by changing the focal plane position. However, this was not done because the focal plane provided the strongest Raman signal. The binary image still has some small particles that are removed below a size limit by the particle removal operation. At this point, two lines originate from one cell and after particle detection step each of the line would be recognized as two individuals, resulting in double detection of single microalgal cell. So a dilation operation was employed which is defined as
where
P0 is the central pixel with a mask and the mask structuring elements have the pixel values of
Pi. After the dilation operation the two lines are combined to one line. Hence each alga now has a corresponding line in the binary image (
Fig. 2e). Small gaps might exist in the binary image. A fill-hole operation removes these voids. Then, the coordinates of the particles as well as the number of the microalgae are obtained by a particle analysis which is also known as blob analysis. The center of mass of each particle is calculated using the formula
where
XG,
YG and
N are the x-center of mass, y-center of mass and the number of cell pixels respectively. The coordinate information combined with the intensity image (
Fig. 2f) is saved for subsequent operations. The center of mass of double cells (
e.g. 1, 3 and 12) laid just between two cells. As the laser diameter was much larger than the cell width, this complication was in most cases not relevant for parallel orientation of cells (
e.g. 1 and 3) and one high quality spectrum was collected representing both cells. To minimize this effect, the cells were pipetted at low density.
3.2 Raman spectroscopy based assessment of pigment content
A Raman spectrum probes intrinsic molecular vibrations of all biomolecules in a cell and changes thereof with dozens of vibrational bands available for analysis. As evident from bands around 1160 and 1530 cm−1 in the mean Raman spectrum of P. tricornutum (Fig. 3a) most intense spectral contributions are assigned to a pool of carotenoid molecules, mainly light-harvesting fucoxanthin (Fx) as well as Ddx and Dtx.18 Less intense bands are labeled for Chl.
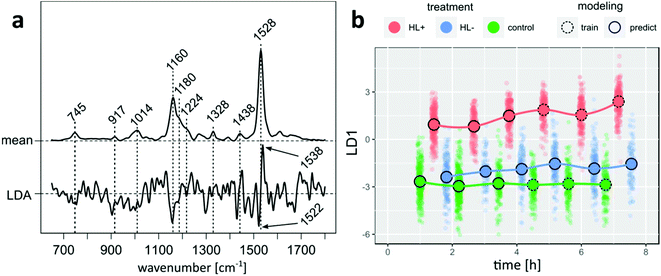 |
| Fig. 3 PLS-LDA model coefficient is compared with a mean Raman spectrum of P. tricornutum and points to lower Ddx + Dtx concentrations in the carotenoid pool upon DTT exposure (a). Projection of Raman spectra of all samples into LD1 subspace visualizes the time-resolved effect of DTT in combination with higher irradiance (red), higher irradiance without DTT (blue) and control P. tricornutum cells (green) (b). | |
Following the acquisition, a PLS-LDA model was trained with spectra from control and HL+ samples, which were acquired more than four hours after splitting the cultures. Subsequently, all spectra from HL− as well as remaining spectra from HL+ and control that were left out from modeling were projected into LD subspace as shown in Fig. 3b. HL+ spectra are already separated from HL− and control spectra without DTT addition 1.5 hours after inoculation. Following the mean center of the point clouds over time, spectral features of HL+ cells further changed after 3 hours in contrast to constant scores of control cells. Considering that the model reflects differences between DTT treated cells at higher irradiance and control cells, it is interesting to see where the projection of spectra from HL− cells at higher irradiance will fall into LD space. The projection of HL− spectra is more similar to control cells at the beginning, but over time its mean center is noticeably shifted towards higher LD scores. This result indicates that the modelled differences originally arouses from DTT treatment rather than shifting the cultures to higher irradiance alone. Underlying spectral changes can be derived from model coefficients shown in Fig. 3a. Negative model coefficients are correlated with spectra from untreated cells (HL− and control). Van Leeuwe et al. investigated the pigment composition of Phaeocystis Antarctica under similar culture conditions with HPLC.19 Three hours after addition of DTT they found that the pigment ratio (Ddx + Dtx)/Chl significantly decreased in HL+ cells by 20% relative to control cells. Supporting evidence for a qualitatively similar observation can be deduced from the coefficient signature around 1528 cm−1 which is assigned to C
C stretching modes of carotenoid molecules. It shows a negative feature at 1522 cm−1 and a positive feature at 1538 cm−1. Premvardhan et al. investigated resonance-enhanced Raman spectra of isolated Fx and Ddx complexes from Cyclotella meneghiniana in different organic solvents.18 Independent of the conditions the C
C band of Ddx was shifted towards lower wavenumbers compared to Fx. Since the observed Raman band at 1528 cm−1 in cell spectra is a linear combination of signals from Fx and Ddx + Dtx the observed LD coefficient pattern points towards reduced spectral contributions from Ddx + Dtx molecules in cells of HL+ samples. Similarly, negative LD coefficients at 1158 cm−1 arising from C–C stretching mode can be mainly assigned to the pool of Ddx + Dtx as the relative band intensity in this spectral region is higher for both pigments compared to the hypsochrome shifted band of Fx.18 Without further model validation we conclude that our findings agree well with the HPLC experiments of van Leeuwe et al.19
4. Conclusions
While for HPLC analysis thousands of pooled cells have to be lysed and the experiments take several hours to perform, the HTS-RS platform can provide comparable information from individual and living cells. Raman experiments of hundreds cells for a single time point can be performed in minutes. The introduced HTS-RS platform allows studying subtle changes in the pigment composition of microalgal cells induced by external stimuli in a label-free manner without sample preparation. The full potential of the HTS capability could not be exploited yet because only ca. 20% (3139 out of 16
888) spectra passed the quality criterion. The following approaches are planned to increase the fraction of usable Raman spectra. If the center of mass is between two cells, then the laser focus might miss the highest pigment content in the cell. Therefore, the cell localization algorithm needs further refinement. Even more relevant for a large field of view, cells outside the focal plane give weak Raman signals which will be compensated in the future by an auto focus option for each frame. The HTS-RS capability that was demonstrated for this model system can be transferred to unmet analytical applications that require high chemical specificity and sensitivity such as kinetic in vivo studies of microalgal assemblages and large scale monitoring of microplastic particles. Whereas contributions of pigments are resonance enhanced in Raman spectra of algae, Raman cross sections of plastics are several orders higher compared to non-resonance enhanced biomolecules which facilitates their Raman-based detection as another application of the HTS-RS platform.
Conflicts of interest
The authors declare no conflict of interest.
Acknowledgements
Financial support of the DFG via the project diatoms (FKZ KR4387/1-1) is highly acknowledged. We thank Prof. Pohnert (University of Jena) for kindly providing the culture medium.
References
- T. M. Mata, A. A. Martins and N. S. Caetano, Renewable Sustainable Energy Rev., 2010, 14, 217–232 CrossRef CAS.
- M. A. Torres, M. P. Barros, S. C. G. Campos, E. Pinto, S. Rajamani, R. T. Sayre and P. Colepicolo, Ecotoxicol. Environ. Saf., 2008, 71, 1–15 CrossRef CAS PubMed.
- M. Chen, T. Mertiri, T. Holland and A. S. Basu, Lab Chip, 2012, 12, 3870–3874 RSC.
- S. Bae, C. W. Kim, J. S. Choi, J.-W. Yang and T. S. Seo, Anal. Bioanal. Chem., 2013, 405, 9365–9374 CrossRef CAS PubMed.
- H. Wu, J. V. Volponi, A. E. Oliver, A. N. Parikh, B. A. Simmons and S. Singh, Proc. Natl. Acad. Sci. U. S. A., 2011, 108, 3809–3814 CrossRef CAS PubMed.
- K. Li, J. Cheng, Q. Ye, Y. He, J. Zhou and K. Cen, Bioresour. Technol., 2017, 244, 1439–1444 CrossRef CAS PubMed.
- J. Rüger, N. Unger, I. W. Schie, E. Brunner, J. Popp and C. Krafft, Algal Res., 2016, 19, 246–252 CrossRef.
- P. Heraud, J. Beardall, D. McNaughton and B. R. Wood, FEMS Microbiol. Lett., 2007, 275, 24–30 CrossRef CAS PubMed.
- X. Wang, L. Ren, Y. Su, Y. Ji, Y. Liu, C. Li, X. Li, Y. Zhang, W. Wang, Q. Hu, D. Han, J. Xu and B. Ma, Anal. Chem., 2017, 89, 12569–12577 CrossRef CAS PubMed.
- H. S. Kim, S. C. Waqued, D. T. Nodurft, T. P. Devarenne, V. V. Yakovlev and A. Han, Analyst, 2017, 142, 1054–1060 RSC.
- F. B. Legesse, J. Rüger, T. Meyer, C. Krafft, M. Schmitt and J. Popp, ChemPhysChem, 2018, 19, 1048–1055 CrossRef CAS PubMed.
- I. W. Schie, J. Rüger, A. S. Mondol, A. Ramoji, U. Neugebauer, C. Krafft and J. Popp, Anal. Chem., 2018, 90, 2023–2030 CrossRef CAS PubMed.
- M. Harker, C. Berkaloff, Y. Lemoine, G. Britton, A. J. Young, J.-C. Duval, N.-E. Rmiki and B. Rousseau, Eur. J. Phycol., 1999, 34, 35–42 CrossRef.
- M. Lohr and C. Wilhelm, Proc. Natl. Acad. Sci. U. S. A., 1999, 96, 8784–8789 CrossRef CAS PubMed.
- I. Maier and M. Calenberg, Bot. Acta, 1994, 107, 451–460 CrossRef CAS.
-
C. Beleites and V. Sergo, hyperSpec: a package to handle hyperspectral data sets in R, URL http://hyperspec.r-forge.r-project.org, R package version 0.98, 2011 Search PubMed.
-
C. Beleites, cbmodels: Collection of “combined” models: PCA-LDA, PLS-LDA, PLS-LR as well as EMSC, R package version 0.5-20150729, 2015 Search PubMed.
- L. Premvardhan, L. Bordes, A. Beer, C. Büchel and B. Robert, J. Phys. Chem. B, 2009, 113, 12565–12574 CAS.
- M. A. van Leeuwe, R. J. W. Visser and J. Stefels, J. Phycol., 2014, 50, 1070–1080 CrossRef CAS PubMed.
|
This journal is © The Royal Society of Chemistry 2019 |