DOI:
10.1039/C8AN01059E
(Communication)
Analyst, 2019,
144, 130-136
Quantifying cyanide in water and foodstuff using corrin-based CyanoKit technologies and a smartphone
Received
8th June 2018
, Accepted 4th November 2018
First published on 21st November 2018
Abstract
This paper describes the detection of endogenous cyanide using corrin-based CyanoKit technologies in combination with a smartphone readout device. When applied to the detection of cyanide in water, this method demonstrates high repeatability and discriminative power with a limit of blank of 0.074 ppm and an instrument limit of detection of 0.13 ppm. Quantification of endogenous cyanide in cassava and bitter almond extracts with the smartphone readout is in excellent agreement with independent analyses using traditional spectrophotometric detection. The prototype system objectively detects levels of cyanide with a high granularity at the point-of-need and does not depend on large, heavy and expensive instrumentation. The methodology has the potential to be easily adopted in resource limited situations and low-income countries.
Introduction
Universal access to mobile information and communication technologies (ICT) through smartphones has facilitated digitalisation and easy access to data across many fields. Of particular interest are the embedded sensors and computational power of smartphones as they can be leveraged to provide low-cost and accurate data acquisition in geographical areas where access to technology and objective measurement tools were previously unavailable. Application areas for mobile sensors are manifold and include health and wellbeing.1 For example, the embedded accelerometers on a smartphone can be used for detecting device orientation, but also for tracking the owners’ physical activity.2 The smartphone camera is another powerful sensor to address medical and analytical needs. The successful examples include monitoring heart rate3 and breathing rate4 continuously over time, point-of-need food allergen detection,5 and various microscopy applications.6 The colour camera is a useful sensor to identify intensity and colorimetric samples, either as single assessments or changes over time. Hardware components have significantly improved over the past years so that lower priced phones feature cameras with high quality specifications. Therefore, smartphones are ideal mobile tools to objectively perform colorimetric readings of chemical and other analytical tests in remote and low resource settings.
Cyanogenic glycosides appear in more than 2500 plants7 where they can enzymatically release toxic cyanide. The most prominent cyanogenic glycosides are prunasin and amygdalin, found in fruit kernels such as bitter almonds which contain high levels of the latter, and linamarin found in high levels in cassava. Cassava is a major carbohydrate source for more than 2 billion people in the world. It is of particular importance in Africa, because it is drought resistant and yields well even in poor soil without fertilizer.8 Cyanide intoxication of poorly processed cassava foodstuff may lead to illnesses such as neuropathy and epidemic spastic paraparesis (“konzo”) in populations where cassava is the staple food.9 Furthermore, a high intake of bitter almonds can cause severe cyanide intoxication.10 Therefore detection, monitoring and removal of cyanide during the processing of foodstuff is of utmost importance. Commercially available analytical methods for detecting cyanide are sophisticated and complicated to perform, require processing of toxic chemicals, or depend on highly trained users.11 To avoid such situations in the future, more appropriate point-of-need tests are required that can accurately and rapidly identify foodstuff at risk.12,13
Herein, we report on a smartphone-based device for the quantitative detection of biological endogenous cyanide (CN−) in water and foodstuff using CyanoKit technologies (Cyanoguard AG, Wädenswil, CH). Thus far, CyanoKit technology has been constrained to the semi-quantitative detection of cyanide by the “naked-eye” using a colour reference chart.14,15 To overcome these limitations, we introduce an easy to process quantification system based on ubiquitous smartphones equipped with a camera, and computer vision algorithms to provide a quantitative and reproducible readout at low cost. This novel and innovative approach represents a clear step forward in analyte detection compared to most mobile optical-sensor systems that apply colorimetry to immobilised, paper-based indicators.16
Experimental
Cyanide testing
The principle of the colorimetric detection of CN− with corrin-based chemosensors has been described elsewhere17 and is summarized herein shortly. An orange coloured aqua, cyano corrinoid is immobilized on a solid-support (CyanoKit) and the rapid, selective substitution of the cobalt-coordinated H2O with CN− leads to a violet-coloured dicyano-corrinoid in the colorimetric sensing area of the cartridge (Fig. 1).18
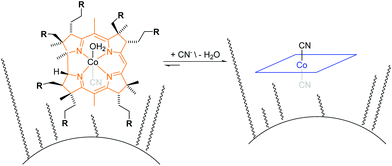 |
| Fig. 1 Schematic overview of cyanide binding with immobilized corrinoids in the detection zone of the CyanoKit. R = COOCnH2n+1 where n = [1,4]. | |
System design/device
The system presented herein consists of a smartphone including an app and an imaging attachment that holds a colour chart and the CyanoKit testing cartridge (Fig. 2). The imaging attachment has been developed following the approach of Oncescu et al. to have maximal control over the light conditions during image taking19 and to minimize possible user errors from positioning of the cartridge in front of the camera. The attachment consists of a smartphone holder, which centres the position of the smartphone, specifically the camera and LED-flash, relative to the device case (Fig. 2). The case is used to shield the cartridge and the colour chart inside from other light sources than the smartphones’ LED, and to keep the distance between the camera, cartridge, and colour chart constant. The cap is used to hold the cartridge in place with a holder in which the cartridge can be clipped in, and additionally holds the colour chart underneath the cartridge. This design ensures that the LED illumination applies similarly to both the sensing area of the cartridge and the colour chart.
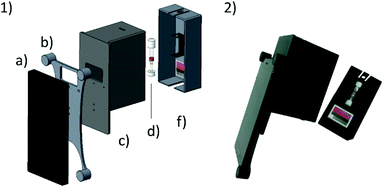 |
| Fig. 2 Illustration of the imaging system: (1) isometric view with (a) smartphone with app, (b) smartphone holder, (c) case, (d) CyanoKit sensing cartridge, (f) cap with cartridge holder and colour calibration chart of prototype used for experimentation. (2) Photograph of whole system that was used for experimentation. | |
We used a Huawei Y5-II smartphone (Android 5.1 lollipop) which has a primary camera with 8 MP, f/2.0, autofocus and LED flash. The parts of the attachment were printed from black polylactic acid (PLA), in order to remove unwanted reflections from the inside of the case, using a Prusa i3 MK2 (Prusa Research, Prague, CZ) fused deposition modelling 3D printer.
Quantitative cyanide determinations of liquid samples with the CyanoKit cartridge and the smartphone device consists of two steps. A liquid sample is passed through the cartridge containing the cyanide sensitive chromophore. The latter is subsequently analysed with the smartphone. Therefore, the cartridge is inserted into the device cap, and mounted on the device case. Afterward, the colorimetric reaction zone of the cartridge is photographed, colour-corrected with the colour chart, analysed, and displayed, stored or transmitted to a database with the smartphone app (Fig. 3).
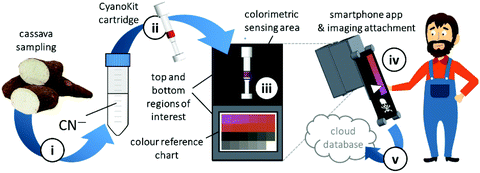 |
| Fig. 3 Envisioned use of the smartphone system for cyanide detection in foodstuff: a liquid sample derived from foodstuff (i) is passed through the CyanoKit cartridge (ii). The cartridge is placed in the smartphone accessory (iii, top region of interest) and the colorimetric reaction is photographed. The app performs an automated colour correction based on the custom colour calibration chart (bottom region of interest) and provides an analysis of the cyanide concentration which is then displayed on the smartphone screen (iv) and can be added to online databases (v). | |
Algorithm
In brief, the computer vision algorithm embedded in the custom imaging app converts the acquired image data to the CIElab colour space20 (Fig. 4). Then, it automatically detects the sensing zone and the reference zones. The mean colour obtained from the sensing zone is normalized using the normalization curve derived from the reference zone. It then estimates the CN− concentration from the obtained colour value using a calibration function.
 |
| Fig. 4 Algorithm for image acquisition and processing. The top and bottom regions of interest (ROI) are processed independently and then results combined to normalize sensing area colour and estimate cyanide (CN−) concentration in the sample and foodstuff. | |
The image acquisition in the first stage of our algorithm is performed using the native camera functions available in the Android app development framework. Although the imaging attachment limits external light and provides a controlled environment, many remaining variables can greatly influence the consistency and integrity of the obtained image. We control four additional parameters with the camera software: (1) LED-flash of the smartphone is set to torch-mode, which enables the camera to auto-focus correctly and consistently distribute light reflections; (2) white-balance is set to “fluorescent light”; (3) sensitivity of the camera sensor to ISO value 160; and (4) exposure time of the camera sensor to 200 ms.
After image acquisition, the RGB pixel data is transformed to a mat array and converted to the CIElab colour space. Because the cartridge and colour chart are mechanically fixed in known positions, the image is now divided horizontally into top and bottom regions of interest (ROI). Using the bottom ROI, the algorithm recovers the reference colour chart. This is done by applying a white colour filter, which leaves only the border of the colour chart and the white colour patch within the image. The algorithm then searches for contours by joining all the consecutive points which have the same intensity. With the assumption that only simple contours, such as rectangles, are relevant in our application, a list of all possible contours is created. From this list, the contour with the largest area is then identified as the colour reference chart, and a rectangle is formed around it. The relative position of the colour patches inside this rectangle always remains the same and are easy to identify. The mean colour values of each colour patch is then calculated. The algorithm then derives from the pre-stored colour values the correction matrix for the extracted colour patch. Finally, using cubic splines, a normalization function is built ad-hoc, which can then be used to correct the colours detected in the top ROI.
The top ROI contains the cartridge with its colorimetric sensing area. First, this ROI is analysed by applying a digital colour filter to the image. Since all possible colour values of the sensing area have a significant red component and the surrounding colours of the cartridge are controlled and clearly different from red, this operation returns the sensing area. Using this image segment, a binary mask is created and with a bitwise-and-operation used to extract the same colour segment from the L- and b-channel. Now, analogue to the process described previously, the largest contour of the image is detected and a rectangle is built around it, from which, the mean colour value is extracted.
By combining the results of the two sub algorithms, the colour values of the CyanoKit sensing area are corrected by applying the spline normalization function and the mean colour channel values computed. This colour value is then used in a calibration curve function to estimate the CN− concentration.
The algorithm was implemented in Android Studio v3.0 with the support of the Open Source Computer Vision Library v3.3 (OpenCV).21 Mat arrays, the basic image container of OpenCV, are used to store the pixel information during runtime and the CIElab colour space is used as standard colour space.
Colour reference chart
In order to correct for the colour variation from manufacturer algorithms and sensor specifications, a contextual custom colour reference chart with 4 × 6 colour patches with distinct CIElab colours relevant for the CyanoKit was developed. Wannous et al. have shown that custom colour reference charts are superior to standard colour charts, if the range of photographed scenes is known before obtaining an image.22 In our approach, we provide correction values for the b-channel while holding the L- and a-channel constant in the first two rows. The other two rows provide correction values for the L channel while keeping the a- and b-channel at zero. Additionally, the border of the colour chart has been designed to be white for good contrast and the ratios of the colour chart and the colour patches have been standardized in order to make it easy for computer vision algorithms to find the colour reference chart and the individual patches. The colour reference chart was designed using Inkscape 0.92.2 and printed on white 80 g m−2 paper using a HP Color LaserJet CP3505 printer.
Materials and instruments
KCN and Ches were obtained from Fluka (Buchs, CH). Aquacyano-cobyrinic acid heptamethylester (ACCbs) was synthesized as described elsewhere.23 CyanoKit cartridges were obtained from CyanoGuard (Wädenswil, CH). Aq. stock solns. of KCN (1000 ppm) were prepared freshly before use. The pH value of 9.5 of the aq. stock soln. of the buffer Ches (0.25 M) was adjusted by the addition of either an aq. soln. of NaOH (2 N) or HCl (1 N). All measurements were performed at a final buffer concentration of 50 mM and at T = 21 ± 1 °C. The pH values of the aq. solns. were measured with a Metrohm 827 pH lab.
Preparation of CN− spiked H2O
Aliquots of the CN− stock soln. were diluted with H2O as required.
Preparation of cassava root and potato extracts
Extracts from three different cassava roots and a potato (negative control) were prepared as described elsewhere24 and two aliquots (100 μL) were diluted for analysis with the smartphone and with UV-vis spectroscopy as desired.
Preparation of almond extracts
Three bitter almonds (Prunus Amygdalus Amara) imported from Spain and a sweet almond (Prunus dulcis) as a negative control were ground with a zest grater. The almond powder (0.4 g) was diluted with H2O (40 mL) and the aq. sample was stored in a sealed tube for 60 min at 22 °C in order to enzymatically release endogenous CN− from the cyanogenic glycoside.17 It was centrifuged for 10 min at 4000 U and an aliquot (100 μL) was diluted for analysis with the smartphone and with UV-vis spectroscopy as desired.
Calibration
To describe the relationship between the CN− concentrations and the obtained mean colour value of the smartphone camera, we modelled a quadratic regression curve using the colours measured from images obtained from CyanoKit cartridges loaded with defined quantities of CN−. These calibration cartridges were prepared by passing CN− spiked H2O (0 to 1 ppm) through the CyanoKit cartridge according to the manufacturer's instructions in triplicates.
A Perking Elmer Lambda 35 spectrophotometer using quartz cells with a path length of 1 cm was used as reference measurement as described in literature.15 The relationship between the CN− concentrations and the UV-Vis measurements were derived by a linear regression curve from the calibration titrations of ACCbs with CN− (0 to 0.8 ppm) in H2O in triplicates such as
Measurements
For evaluation of the smartphone readout, an aq. sample soln. (1 mL, pH 9.5) was passed through a CyanoKit cartridge according to the manufacturer's instructions. For quantifications by UV-Vis spectroscopy, an aq. sample soln. (1 mL, CHES [50 mM] pH 9.5, ACCbs [30 μM]) was prepared and analysed as described elsewhere.15 All evaluation cartridges and spectroscopy samples were prepared in triplicates each.
Smartphone
The cartridge was placed in the imaging attachment and an image was taken and analysed using the smartphone app containing the colour normalization and calibration curve algorithms. This step was quadruplicated for cartridges containing CN− spiked H2O, triplicated for cassava or potato extracts, and duplicated for almond extracts.
Spectroscopy
UV-vis spectra of CN− containing foodstuff extracts were measured with the spectrophotometer and the calibration function as described above. The UV-vis spectra were recorded in triplicate.
Evaluation
All results obtained from the evaluation cartridges and spectroscopy samples were imported into Matlab (Mathworks, Natick, USA) for statistical analysis and visualisation. The limit of blank (LoB) was calculated using the linearly interpolated 95th percentile of the measurements obtained from the CN− spiked H2O sample with 0 ppm. The limit of detection (LoD) was derived from the sum of the LoB and the interpolated 5th percentile of the measurements obtained from the H2O sample with 0.1 ppm. A Bland–Altman plot was created to derive bias and limit of agreements,25 and the root mean square error (RMSE) was calculated. CN− concentrations from aq. samples of processed foodstuff were calculated back to concentrations in the unprocessed foodstuff (mg kg−1) considering the protocols of sample preparation with dilution factors of 100 (cassava/potato) and 5000 (almonds). Results were then compared using Sidak's multiple comparison test at an alpha level of 0.05.
Results and discussion
We have developed a mobile system featuring a method for the quantitative detection of CN− concentration using corrin-based CyanoKit technologies in combination with a smartphone readout device. This method consists of five steps (i–v, Fig. 3). First (i), a liquid sample is prepared from the foodstuff as described in the Experimental section. Afterward, the sample is passed through the CyanoKit cartridge with the immobilized chemosensor (ii). In particular, cyanide leads to substitution of cobalt-coordinating H2O of the immobilized aqua, cyano corrinoid in the detection zone of the cartridge (Fig. 1).17 This chemical reaction is accompanied by a change of colour from orange to violet of the metal-based indicator with increasing CN− concentrations. The cartridge is then inserted into the device accessory (iii) and the colorimetric reactions is photographed, automatically colour-corrected with a reference colour chart and analysed using the smartphone app (iv), and for further aggregation, the results transmitted to a server (v). With this approach we solved two major challenges of using corrin-based CyanoKit technologies: (1) we created for the measurements a standardized and portable illumination environment without being disturbed by ambient conditions and (2) developed a repeatable and rapid readout that was independent of the subjective visual inspection by a human through providing a custom colour reference chart and a calibration algorithm. The time required for the smartphone measurement from inserting the cartridge into the holder to displaying the results on the smartphone app and store them on a server (iii–v) lasted less than 15 s. The duration of the analysis is therefore a multiple faster than a conventional, lab based UV-Vis measurement. Technical details on the construction of the imaging attachment, the programming of the app and the underlying algorithms to detect and process the colour indication are presented in detail in the Experimental section.
To make a quantitative measurement of CN− possible, a calibration between the obtained mean colour value in the sensing area, as determined by the smartphone camera, and the cyanide concentrations was necessary. Therefore, we created a set of reference images from CyanoKit cartridges with different, known concentrations of CN−, which we then analysed to establish a mathematical relationship linking colour to CN− concentration. We obtained a quadratic regression curve with a coefficient of determination (R-squared) = 96.38% and p < 0.001, such as
Therefore, for quantification of CN−, only one colour channel (b-channel of CIELab) was required to achieve high discriminative power and the calibration procedure was simple enough to be included into the smartphone app.
With this calibration curve and app at hand, we tested 11 different CN− spiked water samples. This allowed us to determine the system performance on known samples. The limit of blank (LoB) was as low as 0.074 ppm and the limit of detection (LoD) was only 0.13 ppm (Fig. 5). With increasing CN− concentrations, the discriminative power reduced. Overlapping distributions could be observed in spiked water with 0.6 and 0.7, and 0.8 and 0.9 ppm (Fig. 5). RMSE was 0.06 ppm and, as depicted on a Bland–Altman plot, the bias was 0.002 ppm and the 95% confidence interval of the detection was at 0.12 ppm (Fig. 6). Measurements were outside the confidence interval for spiked water above 0.5 ppm, and at 0.8 ppm the system had clearly a drop in discriminative power where the results overlapped with the 0.9 ppm data (Fig. 6). This behaviour can be partially attributed to the fact that at the upper end of the ppm range, most of the colorimetric reagent in the CyanoKit cartridge is saturated with CN−. Therefore, the colour changes are not as prominent as in the lower range. Furthermore, the camera sensor of the smartphone is likely saturating in the upper range of the red channel as only 256 shades can be recorded (8-bit pixel resolution). Both of these effect might have contributed to the need for a quadratic instead of a linear calibration curve.
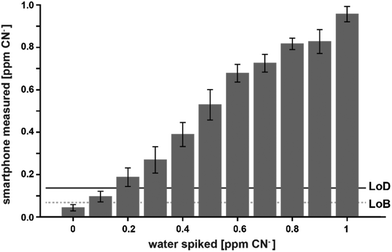 |
| Fig. 5 Results of the cyanide-spiked H2O samples that were analysed with the smartphone app (12 measurements for each concentration). The limit of blank (LoB) and the limit of detection (LoD) are represented as dotted and continuous lines, respectively. | |
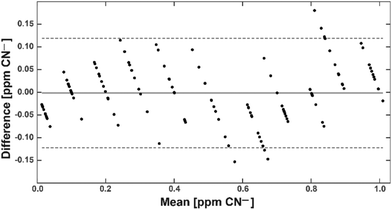 |
| Fig. 6 Bland–Altman plot comparing the ground truth of the cyanide-spiked H2O with the smartphone measurements. The continuous line depicts the mean error and the dashed lines enclose the 95% confidence interval. | |
Encouraged by the promising results of detecting cyanide in spiked water, we applied the CyanoKit technology in combination with the new smartphone readout to the detection of endogenous cyanide in foodstuff. In particular, we determined cyanide concentrations between 20 and 69 mg kg−1 in three fresh cassava samples (Fig. 7) and as high as 3197 mg kg−1 in three bitter almond samples (Fig. 8). Cyanide analysis in foodstuff with the smartphone readout device was statistically comparable to results from traditional UV-Vis spectroscopy (Fig. 7 and 8) and the measured concentrations were all within expected ranges for cassava (15–400 mg kg−1)26 and bitter almonds (900–4700 mg kg−1).27,28 Unsurprisingly, the variability in the measurements with the smartphone camera was larger than the lab based spectroscopy. Most importantly, a clear difference between CN− containing (cassava, bitter almonds) and CN− free (potato, sweet almonds) foodstuff was observed, confirming the previously obtained LoB and LoD results with spiked water in foodstuff. These observations indicate the suitability of the test to evaluate contents of CN− in a large variety of samples from different types of foodstuff.
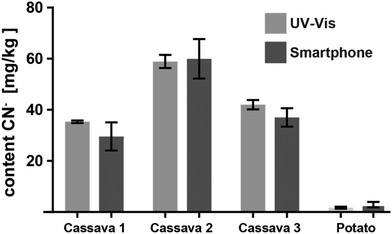 |
| Fig. 7 Cyanide content measured from three different cassava root and one potato samples with the smartphone reader and with UV-Vis spectroscopy (3 measurements each). | |
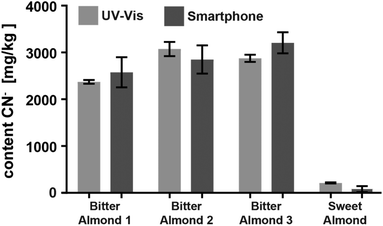 |
| Fig. 8 Comparison of the cyanide content in three bitter and one sweet almond samples analysed with either the smartphone device or with UV-Vis spectroscopy (2 measurements each). | |
In this work, we did not evaluate different smartphone models to test universal application of the approach. However, with our specific colour checker design and automated normalization algorithms, sensor based variation in colour measurements can be compensated. We used a 2 years old phone with sensors at the lower end of possible specifications. Most current phones feature improved sensor quality with higher pixel count and better quantum efficiencies which improves low light performance and reduces noise in the images. Therefore, even better colour estimation results can be expected with the majority of new phones. Furthermore, the design of the smartphone holder is modular and can accommodate a vast range of models (Fig. 2). For universal use of the proposed system, an automated quality control algorithm that evaluate camera specifications and image quality (e.g. noise levels), including integrity verification of the colour reference chart, will be necessary.
For this work, we did not optimize the sample preparation process to achieve maximal sensitivity at the recommended threshold for CN− intake (e.g. 10 ppm for cassava). A foodstuff specific, optimized and simplified preparation of samples could lead to a simple detection tool for populations that apply short-soaked cassava preparations with high risk for elevated CN− residues and the development of konzo.29
In addition to food safety control, we envisage applications of this straightforward CN− testing systems in water analysis as underscored by our pioneering studies with CN− spiked H2O. The US Environmental Protection Agency recommends the upper limit of CN− in water to be 0.2 ppm.30 Therefore, the proposed sensing system with a LoD of 0.12 ppm can safely distinguish between accepted and toxic water without additional processing or enrichment.
Conclusion
The proposed smartphone based CN− test enables an objective and repeatable assessment of water and foodstuff at a sensitivity that is relevant to the food industry and end-user alike. The measurement procedure is simple and can easily be performed at the point-of-use without sophisticated equipment. The software, colour reference chart and schematics could be easily downloaded from an internet resource and locally reproduced. With today's ubiquitous access to smartphones, the automated readout of colorimetric tests is a valid alternative to semi-quantitative, visual assessments of biochemical tests.
Conflicts of interest
WK has no conflicts to declare. FZ is inventor of the CyanoKit technology and shareholder of Cyanoguard AG who manufactures the CyanoKit. MC is now an employee of Cyanoguard AG.
Acknowledgements
The authors thank CyanoGuard AG for providing the CyanoKit at no cost. WK is supported through the Swiss National Science Foundation Grant 150640.
Notes and references
- E. J. Topol, S. R. Steinhubl and A. Torkamani, J. Am. Med. Assoc., 2015, 313, 353–354 CrossRef CAS PubMed.
- J. Kwapisz, G. Weiss and S. Moore, ACM SIGKDD Explor. Newsl., 2010, 12, 74–82 CrossRef.
- E. Jonathan and M. Leahy, Physiol. Meas., 2010, 31, 1–6 CrossRef PubMed.
- W. Karlen, A. Garde, D. Myers, C. Scheffer, J. M. Ansermino and G. A. Dumont, IEEE J. Biomed. Health Inform., 2015, 19, 1331–1338 Search PubMed.
- A. F. Coskun, J. Wong, D. Khodadadi, R. Nagi, A. Tey and A. Ozcan, Lab Chip, 2013, 13, 636–640 RSC.
-
A. F. Coskun, H. Zhu and A. Ozcan, in Mobile Point-of-Care Monitors and Diagnostic Device Design, ed. W. Karlen, CRC Press, Boca Raton, 2014, pp. 23–42 Search PubMed.
-
W. M. Haschek, Haschek and Rousseaux's Handbook of Toxicologic Pathology, Academic Press, Boston, 3rd edn, 2013 Search PubMed.
- D. Nhassico, H. Muquingue, J. Cliff, A. Cumbana and J. H. Bradbury, J. Sci. Food Agric., 2008, 88, 2043–2049 CrossRef CAS.
- M. J. Boivin, D. Okitundu, G. Makila-Mabe Bumoko, M.-T. Sombo, D. Mumba, T. Tylleskar, C. F. Page, J.-J. Tamfum Muyembe and D. Tshala-Katumbay, Pediatrics, 2013, 131, e1231–e1239 CrossRef PubMed.
- M. Boutbaoucht, M. Najib, A. G. El Adib, M. Sbihi, S. Younous, Y. Mouaffak and F. Zegzouti, Ann. Trop. Med. Public Health, 2013, 6, 679–680 CrossRef.
- Z. Xu, X. Chen, H. N. Kim and J. Yoon, Chem. Soc. Rev., 2010, 39, 127–137 RSC.
- F. Zelder, Chem. Commun., 2015, 51, 14004–14017 RSC.
- C. R. Nicoleti, L. G. Nandi and V. G. Machado, Anal. Chem., 2015, 87, 362–366 CrossRef CAS PubMed.
- F. Zelder and L. Tivana, Org. Biomol. Chem., 2015, 13, 14–17 RSC.
- L. D. Tivana, J. Da Cruz Francisco, F. Zelder, B. Bergenståhl and P. Dejmek, Food Chem., 2014, 158, 20–27 CrossRef CAS PubMed.
- A. İncel, O. Akın, A. Çağır, Ü. H. Yıldız and M. M. Demir, Sens. Actuators, B, 2017, 252, 886–893 CrossRef.
- C. Männel-Croisé and F. Zelder, ACS Appl. Mater. Interfaces, 2012, 4, 725–729 CrossRef PubMed.
- B. Aebli, C. Männel-Croisé and F. Zelder, Inorg. Chem., 2014, 53, 2516–2520 CrossRef CAS PubMed.
- V. Oncescu, D. O'Dell and D. Erickson, Lab Chip, 2013, 13, 3232–3238 RSC.
-
K. Nassau, Color for science, art and technology, Elsevier, 1997 Search PubMed.
- G. Bradski, Dr. Dobb's J. Softw. Tools, 2000, 11, e Search PubMed.
- H. Wannous, Y. Lucas, S. Treuillet, A. Mansouri and Y. Voisin, J. Electron. Imaging, 2012, 21, 023015 CrossRef.
- C. Männel-Croisé and F. Zelder, Inorg. Chem., 2009, 48, 1272–1274 CrossRef PubMed.
- C. Männel-Croisé, B. Probst and F. Zelder, Anal. Chem., 2009, 81, 9493–9498 CrossRef PubMed.
- J. M. Bland and D. G. Altman, J. Biopharm. Stat., 2007, 17, 571–582 CrossRef PubMed.
-
F. Simeonova and L. Fishbein, Hydrogen Cyanide and Cyanides: Human Health Aspects, World Health Organization, Geneva, CH, 2004 Search PubMed.
- T. A. Shragg, T. E. Albertson and C. J. Fisher, West. J. Med., 1982, 136, 65–69 CAS.
- N. Chaouali, I. Gana, A. Dorra, F. Khelifi, A. Nouioui, W. Masri, I. Belwaer, H. Ghorbel and A. Hedhili, ISRN Toxicol., 2013, 2013, 610648 Search PubMed.
- T. Tylleskär, M. Banea, N. Bikangi, R. D. Cooke, N. H. Poulter and H. Rosling, Lancet, 1992, 339, 208–211 CrossRef.
-
Agency for Toxic Substances and Disease Registry, Toxicological Profile for Cyanide, Atlanta, USA, 2006 Search PubMed.
|
This journal is © The Royal Society of Chemistry 2019 |
Click here to see how this site uses Cookies. View our privacy policy here.