DOI:
10.1039/C8SC02533A
(Edge Article)
Chem. Sci., 2018,
9, 7241-7246
Probing cytoplasmic and nuclear microRNAs in single living cells via plasmonic affinity sandwich assay†
Received
8th June 2018
, Accepted 25th July 2018
First published on 25th July 2018
Abstract
MicroRNAs (miRNAs) regulate the expression of mRNAs in cells. The determination of subcellular miRNAs in single living cells is essential for understanding the subcellular localizations and functions of miRNAs as well as the microheterogeneity of cells. However, current approaches fail either to keep the cells alive or maintain their original cellular composition or are unable to provide subcellular resolution. Herein, we presented a new approach, called plasmonic affinity sandwich assay (PASA), for rapid and ultrasensitive probing of trace cytoplasmic and nuclear miRNAs in single living cells. It combined efficient in vivo subcellular extraction with ultrasensitive plasmon-enhanced Raman scattering (PERS) detection. By virtue of a micromanipulator, target miRNAs in the cytoplasm and nucleus were first specifically extracted from single living cells by gold thinlayer-coated glass microprobes modified with a half complementary sequence to the target miRNA and then, they were labelled with silver nanotags modified with a Raman reporter and the other half complement. Sandwich-like complexes of extraction sequence-target miRNA-labelling sequence were formed on the extraction microprobe, which were subjected to PERS detection. The subcellular resolution of this approach was confirmed with miR-29b (predominantly localized in the nuclei) and miR-29a (mainly located in the cytoplasm), whereas the quantitative capability was verified with three cytoplasmic miRNAs including miR-21, miR-155 and miR-203. This approach obviated tedious steps such as subcellular fractionation and enzymatic amplification, and it required only 10 min. It could be a promising tool to provide insights into subcellular localizations, functions and microheterogeneity of miRNAs.
Introduction
MicroRNAs (miRNAs), which are endogenous ∼22 nt non-coding RNAs, play important regulatory roles in animals and plants by targeting mRNAs for cleavage or translational repression.1–4 The expression levels of miRNAs are critical to their biological functions. Particularly, dysregulated miRNA expression patterns have been found to be associated with the states of various diseases and therefore, miRNAs have been suggested as biomarkers for the diagnosis of diseases and as targets for therapy.5–9 Thus, the determination of miRNAs in tissues, cells and biosamples is essential for understanding their biological functions as well as miRNA-based disease diagnosis and therapy. To this end, a variety of tools have been established to determine miRNAs such as quantitative reverse transcription polymerase chain reaction (RT-qPCR),10,11 Northern blotting,12,13 microarray,14,15in situ hybridization (ISH),16–18 molecular imaging,19–22 and flow cytometry.23,24 While the prevailing view indicates that miRNAs function to regulate mRNA stability and translation only in the cytoplasm, increasing evidences have suggested that nuclear miRNAs can also be involved in transcriptional and post-transcriptional regulatory processes.25–28 In addition, it is highly desirable to keep the cells under testing alive after analysis for further investigation. Therefore, analytical tools permitting the determination of subcellular miRNAs in single living cells can be important assets for better understanding the subcellular localizations and functions of miRNAs as well as the microheterogeneity of cells. However, the above-mentioned analytical techniques are inadequate for probing subcellular miRNAs in single living cells. RT-qPCR, Northern blotting and microarray require subcellular fractionation and enzymatic amplification, which are tedious and time-consuming and also require destruction of the cells. Both ISH and molecular imaging can provide satisfactory subcellular resolution and keep the cells alive, but they need introduction of signal reporter molecules into the cells, which may perturb the cells' normal composition or state. Flow cytometry delivers high throughput but fails to provide subcellular localization information. Although a number of new methods have been developed for determining miRNAs in single cells, they also fail to provide subcellular resolution.29–33
Herein, we presented a new approach, called plasmonic affinity sandwich assay (PASA), for rapid and ultrasensitive determination of trace cytoplasmic and nuclear miRNAs in single living cells. The principle of this approach is illustrated in Fig. 1. It combined efficient in vivo subcellular extraction with ultrasensitive plasmon-enhanced Raman scattering (PERS) detection. A gold thinlayer-coated glass microprobe modified with a half complementary sequence to the target miRNA was used for the extraction. By virtue of a micromanipulator, the extraction microprobe was precisely penetrated through the cytomembrane of a single living cell and the nuclear membrane for extraction for a short duration (typically 3 min). After the extraction, target miRNA molecules on the extraction microprobe were labelled with Raman nanotags, which were silver nanoparticles (AgNPs) modified with a Raman reporter and the other half complementary sequence. After the removal of unwanted species, especially excessive Raman nanotags, affinity sandwiches, i.e., extraction sequence/target miRNA/labelling sequence were formed on the extraction microprobe due to hybridization between the target miRNA and its half complementary sequences. Then, the extraction probe was scanned under a micro Raman spectroscope from the tip. Under radiation of a laser beam, the Raman nanotags generated a strong surface-enhanced Raman scattering (SERS) signal, whereas the gold-coated extraction microprobe generated surface plasmon, which further greatly enhanced the SERS signal of the Raman nanotags. Such PERS detection has been confirmed to be able to provide ultrahigh sensitivity at the single-molecule level.34 The PASA approach allowed for PCR-free determination of trace miRNAs in the cytoplasm and nucleus of single living cells. Because the extraction microprobe is small (1–2 μm at the tip) and the extraction can be completed within a short period, the in vivo subcellular extraction is minimally invasive due to which the cells can be still alive after extraction. The subcellular resolution of this approach was confirmed with miR-29b (predominantly localized in the nuclei) and miR-29a (mainly located in the cytoplasm), whereas the quantitative capability was verified with three cytoplasmic miRNAs including miR-21, miR-155 and miR-203. This approach avoided tedious steps such as subcellular fractionation and enzymatic amplification, and it required only 10 min. Therefore, this approach can be a promising tool to provide insights into subcellular localizations, functions and microheterogeneity of miRNAs.
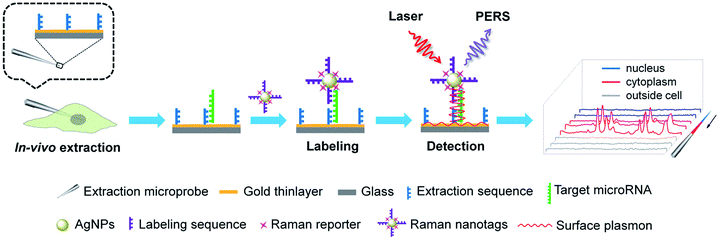 |
| Fig. 1 Schematic of the determination of cytoplasmic and nuclear miRNAs in a single living cell via PASA. | |
Results and discussion
Comparison of PERS and SERS
The mechanism of PERS detection was verified through computer simulation using the finite-difference time-domain (FDTD) method. As shown in Fig. 2A and B, under radiation with a laser beam, an AgNP on a gold thinlayer (PERS mode) generated a much stronger electric field around the nanoparticle when compared with an AgNP on a glass substrate (SERS mode). The effectiveness in the signal enhancement of the PERS detection was experimentally verified. As shown in Fig. 2C and D, the signal intensity generated under the PERS mode was 16.6 times higher than that for the SERS mode. Since SERS is a highly sensitive detection scheme, further enhanced sensitivity in the PERS mode was highly favourable for the detection of trace miRNAs in single cells.
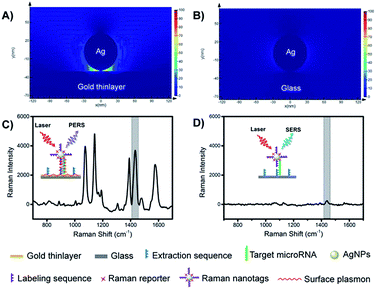 |
| Fig. 2 Electric-field intensity simulated by FDTD under (A) PERS mode and (B) SERS mode. Representative Raman spectra for (C) PERS mode and (D) SERS mode. Insertions show schematic of the principle of PERS and SERS modes. | |
Characterization of the microprobes and nanotags
The preparation procedures of the extraction microprobes and Raman nanotags are illustrated in Fig. S1.† Since Au–N and Ag–S bonds were formed while attaching the extraction and labelling sequences onto the extraction microprobes and the Raman nanotags, respectively, it was necessary to make sure that the presence of these bonds would not interfere with the Raman signal of the Raman reporter.
Fig. 3A shows an image of a representative bare basic microprobe and a gold-coated extraction sequence-modified microprobe. The extraction microprobe did not show any apparent Raman signal (Fig. 3B). The core size of the Raman nanotags was ∼60 nm (Fig. 3C). Fig. 3D shows that only the Raman nanotags exhibited significantly enhanced Raman signal of the reporter 4-aminothiophenol (PATP). A characteristic peak at 1435 cm−1 was employed for detection.
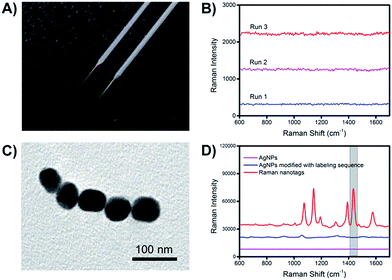 |
| Fig. 3 (A) Photo of a bare starting microprobe (grey) and a final extraction microprobe (gold). (B) Raman spectra of the extraction sequence-functionalized microprobe. (C) Transmission electron microscopic (TEM) image of a Raman nanotag. (D) Raman spectra of bare AgNPs, AgNPs modified with only labelling sequence and Raman nanotags (AgNPs modified with labelling sequence and Raman reporter). | |
The composition of the Raman nanotags, in particular the ratio between the labelling sequence and PATP, plays a critical role in plasmonic affinity sandwich assay. To optimize the ratio, miR-21 was used as the target; the concentrations of the labelling sequence for miR-21 and PATP were set at 10 μM and 100 μM, respectively, and the volume ratios between the solution of the labelling sequence and the solution of PATP were set at 1
:
1, 1
:
2, 1
:
3 and 1
:
4. The dependences of the Raman intensity of the prepared Raman nanotags under the two states, i.e., unlabelling state (PERS detection mode, on a bare gold-coated substrate) and labelling state (PASA detection mode, on a target miRNA-bond extraction sequence-modified gold-coated substrate, with the absence of free Raman nanotags) are shown in Fig. 4A. Clearly, the dependences under the two states were different. Under the unlabelling state, the Raman signal increased as the ratio between the labelling sequence and PATP decreased. This can be assigned to the fact that the total amount of PATP in the Raman nanotags increased as the ratio was reduced. However, under the labelling state, there was an optimal ratio (1
:
2) at which the Raman signal was the strongest. This indicated that there was a compromise between the amount of PATP and that of the labelling sequence when the Raman nanotags were used for PASA detection. The ratio 1
:
2 was selected for later investigations. Fig. 4B shows the UV-visible absorption spectra for PATP and the labelling sequence as well as the UV-visible extinction spectra for AgNPs, AgNPs modified with the labelling sequence and the Raman nanotags. PATP exhibited a maximum adsorption at 252 nm, whereas the labelling sequence exhibited a maximum adsorption at 259 nm. AgNPs, AgNPs modified with the labelling sequence and the Raman nanotags exhibited a maximum adsorption at 415 nm, which was assigned to the localized surface-plasmon resonance (LSPR) characteristic of AgNPs.
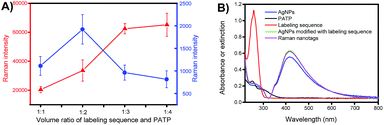 |
| Fig. 4 (A) Comparison of signals for Raman nanotags prepared with labelling sequence solution (10 μM) and PATP solution (100 μM) at different volume ratios on a gold-coated substrate (red) and on a target miRNA-bond extraction sequence-modified gold-coated substrate (blue). Error bars represent standard deviations. (B) UV-visible absorption spectra for PATP and the labelling sequence and UV-visible extinction spectra for AgNPs, AgNPs modified with labelling sequence (without a Raman reporter) and Raman nanotags. | |
The extraction equilibrium on the microprobes was investigated using miR-21 at different concentrations as the samples. As shown in Fig. S2,† when the concentration was as low as 10 pM, the equilibrium was achieved quickly, needing only 3 min. When the concentration of miR-21 in the sample was increased, more time was required to reach equilibrium. Since miRNAs are present in cells in very low concentrations, the extraction time was set at 3 min. Such a short extraction time was favourable to keep the cells alive after extraction.
The probe-to-probe signal variation was investigated with 8 miR-21-capturing microprobes prepared under the same conditions (Fig. S3†). The relative standard deviation (RSD) values in the signal intensities for the detection of miR-21 from standard solutions at concentrations of 100 pM and 10 nM were found to be 10% and 14%, respectively. Considering that the concentrations used were very low, the RSD values are satisfactory.
Performance of PASA
Selectivity is a key criterion for the evaluation of the performance of the developed PASA approach. The selectivity of the PASA approach was investigated using miR-21 as a test sequence and several other miRNAs with similar sequences as interferents. For two-base mismatched and four-base mismatched sequences, the cross-reactivity was low (4.3 to 6.3%), whereas for single-base mismatched sequences, the cross-reactivity was relatively high (19.5 to 25.5%) (Fig. 5A). Since the single-base mismatched sequences of miRNAs are not very abundant, such cross-reactivity levels are acceptable.
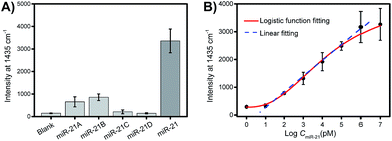 |
| Fig. 5 (A) Interference test. Sample: 10 μM miR-21 or miR-21A (single-base mismatch), miR-21B (single-base mismatch), miR-21C (two-base mismatch), miR-21D (four-base mismatch) dissolved in 20 mM Tris buffer containing 100 mM NaCl, pH 7.6. (B) Dependence of the intensity of the Raman signal on the concentration of miR-21. Error bars represent the standard deviations for three parallel measurements. | |
Sensitivity is another key criterion for the evaluation of the performance of the developed PASA approach. To investigate the sensitivity of the PASA approach, miR-21 was used as the target. The binding isotherm (Fig. 5B) obeyed a typical logarithmic function; the signal intensity increased linearly with the logarithm of the miR-21 concentration within a wide range, spanning 6 logs of concentration (from 10 pM to 1 μM). The limit of quantification (LOQ) was 10 pM. Through logistic function fitting, the apparent dissociation constant (Kd) for the extraction was estimated to be 3.9 nM. Such high affinity ensured that low-abundance intracellular miRNAs could be efficiently extracted from single cells.
Subcellular resolution
To investigate the subcellular resolution of the PASA approach, miR-29b and miR-29a were chosen as test sequences; miR-29b was reported to be predominantly localized in the nuclei, whereas miR-29a was reported to be mainly located in the cytoplasm.25,35 The extraction microprobes were precisely penetrated through the membrane of single living cells of interest and the nuclear membrane for extraction. After the extraction, the extraction microprobes were taken out, followed by washing and labelling. Finally, the extraction microprobes were scanned from the tip with a step of 1 μm. Fig. 6 shows the expression levels of miR-29b and miR-29a in the nuclei and cytoplasm of single HeLa cells measured by PASA, which are in good agreement with the subcellular localization reported in literature.25,35
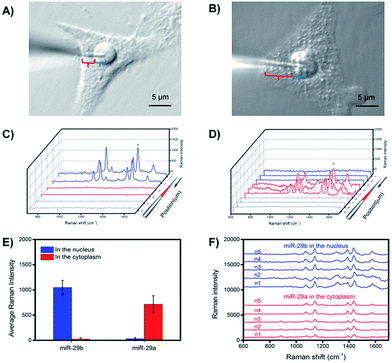 |
| Fig. 6 An image showing the position of an extraction microprobe for probing (A) miR-29b and (B) miR-29a within a single HeLa cell. Raman spectra for (C) miR-29b and (D) miR-29a obtained along the extraction microprobe. (E) Average Raman intensity of miR-29b and miR-29a in single living HeLa cells with subcellular resolution. (F) Representative Raman spectra of multiple probe measurements of miR-29b and miR-29a in the nucleus and cytoplasm, respectively. | |
Quantitative analysis of cytoplasmic miRNAs
To demonstrate the quantitative capability of the PASA approach, three cytoplasmic miRNAs were further tested including miR-21, miR-155 and miR-203. Fig. 7A–C show the Raman intensities of captured cytoplasmic miR-21, miR-155 and miR-203 in individual cells of different cell lines (please see Fig. S4–S6† for corresponding Raman spectra). Clearly, the expression level of these cytoplasmic miRNAs was significantly different between normal and cancerous cells. Both miR-21 and miR-155 were up-regulated in cancerous cells (HeLa and MCF-7 cells), whereas they were limitedly expressed in normal cells (MCF-10A cells). In comparison, miR-203 was down-regulated in cancerous cells (HeLa and MCF-7 cells) and slightly expressed in normal cells (MCF-10A cells). These results were in good agreement with the expression levels of these miRNAs reported in literature.36–38 In addition, these miRNAs showed clearly different expressions in individual cells of the same cell lines, suggesting apparent cell microheterogeneity. With the linear calibration curve established (Fig. 5B), the concentration of miR-21 was measured, and the results are shown in Fig. 7D. Based on the cell size estimated under a microscope, the copy number of miR-21 within the individual cells under testing (Fig. 7D) could be roughly determined. For example, the values were calculated to be 110 ± 82, 36 ± 1 and 111 ± 11 for HeLa cell #1, MCF-10A cell #1 and MCF-7 cell #1, respectively.
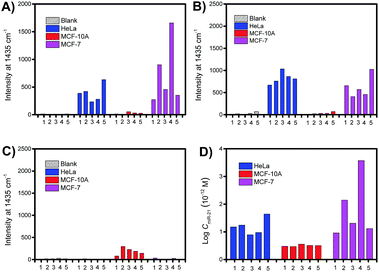 |
| Fig. 7 Comparison of the expression levels of cytoplasmic miRNAs in single living cells. Raman intensity of cytoplasmic (A) miR-21, (B) miR-155, and (C) miR-203 in individual cells of different cell lines. (D) Estimated concentrations of cytoplasmic miR-21 in individual cells according to the intensities shown in (A). | |
Finally, we further demonstrate that in vivo extraction is essential for the accurate determination of the real expression of miRNAs in single cells and microheterogeneity. The PASA approach can be also applicable to large sample volumes. However, when single cells were lysated and then measured by the PASA approach, a dramatic dilution effect was observed. Even when the dilution effect was reduced as much as possible (from a single cell to a minimal measurable volume, 0.2 μL, diluted by about 20
000 times), the concentration of the sample was still outside the linear range of the calibration curve; thereby, the obtained results failed to reflect the real expression and microheterogeneity (Fig. S6†). To compensate for the dilution effect due to cell lysis, a large population of cells is needed, e.g., 20
000 cells or more. However, this can only allow for the determination of the average concentration of target miRNAs and cannot provide the concentration at the single-cell level. Although LOQ of the PASA approach was not very low (10 pM), since there was no dilution effect during the in vivo extraction, it allowed for the determination of target miRNAs in single cells. Clearly, in-cell extraction avoided any dilution effect, thus not only making determination possible but also reducing the measurement error to a great extent.
Conclusions
To conclude, through combining efficient in vivo subcellular extraction with ultrasensitive PERS detection, a new single-cell analytical tool, termed as PASA, has been developed in this study. The PASA approach allowed for rapid and ultrasensitive determination of trace cytoplasmic and nuclear miRNAs in single living cells. This procedure is facile, obviating tedious steps such as subcellular fractionation and enzymatic amplification, and it requires only 10 min. It can be a promising tool to provide deep insights into subcellular localizations, functions and microheterogeneity of miRNAs.
Conflicts of interest
There are no conflicts to declare.
Acknowledgements
We acknowledge financial support from the National Science Fund for Distinguished Young Scholars (21425520), the key grants (21627810, 21327902) from the National Natural Science Foundation of China, the “333” Talents Project from Jiangsu Provincial Government, China (No. BRA2016351), and the Open Grant from the State Key Laboratory of Analytical Chemistry for Life Science (No. 5431ZZXM1706).
Notes and references
- V. Ambros, Nature, 2004, 431, 350–355 CrossRef PubMed
.
- D. P. Bartel, Cell, 2004, 116, 281–297 CrossRef PubMed
.
- W. Filipowicz, S. N. Bhattacharyya and N. Sonenberg, Nat. Rev. Genet., 2008, 9, 102–114 CrossRef PubMed
.
- H. Grosshans and W. Filipowica, Nature, 2008, 451, 414–416 CrossRef PubMed
.
- G. A. Calin and C. M. Croce, Nat. Rev. Cancer, 2006, 6, 857–866 CrossRef PubMed
.
- J. Lu, G. Getz, E. A. Miska, E. Alvarez-Saavedra, J. Lamb, D. Peck, A. Sweet-Cordero, B. L. Ebet, R. H. Mak, A. A. Ferrando, J. R. Downing, T. Jacks, H. R. Horvitz and T. R. Golub, Nature, 2005, 435, 834–838 CrossRef PubMed
.
- G. Di Leva and C. M. Croce, Trends Mol. Med., 2010, 16, 257–267 CrossRef PubMed
.
- C. M. Croce, Nat. Rev. Genet., 2009, 10, 704–714 CrossRef PubMed
.
- J. Kota, R. R. Chivukula, K. A. O'Donnell, E. A. Wentzel, C. L. Montgomery, H. W. Hwang, T. C. Chang, P. Vivekanandan, M. Torbenson, K. R. Clark, J. R. Mendell and J. T. Mendell, Cell, 2009, 137, 1005–1017 CrossRef PubMed
.
- A. K. White, M. Vanlnsberghe, O. L. Petriv, M. Hamidi, D. Sikorski, M. A. Marra, J. Piret, S. Aparicio and C. L. Hansen, Proc. Natl. Acad. Sci. U. S. A., 2011, 108, 13999–14004 CrossRef PubMed
.
- F. C. Tang, P. Hajkova, S. C. Barton, D. O'Carroll, C. Lee, K. Q. Lao and M. A. Surani, Nat. Protoc., 2006, 1, 1154–1159 CrossRef PubMed
.
- G. A. Calin, C. Sevignani, C. Dan Dumitru, T. Hyslop, E. Noch, S. Yendamuri, M. Shimizu, S. Rattan, F. Bullrich, M. Negrini and C. M. Croce, Proc. Natl. Acad. Sci. U. S. A., 2004, 101, 2999–3004 CrossRef PubMed
.
- E. Varallyay, J. Burgyan and Z. Havelda, Nat. Protoc., 2008, 3, 190–196 CrossRef PubMed
.
- J. F. Ji, T. Yamashita, A. Budhu, M. Forgues, H. L. Jia, C. L. Li, C. X. Deng, E. Wauthier, L. M. Reid, Q. H. Ye, L. X. Qin, W. Yang, H. Y. Wang, Z. Y. Tang, C. M. Groce and X. W. Wang, Hepatology, 2009, 50, 472–480 CrossRef PubMed
.
- C. J. Guo, Q. Pan, D. G. Li, H. Sun and B. W. Liu, J. Hepatol., 2009, 50, 766–778 CrossRef PubMed
.
- A. Raj, P. Van Den Bogaard, S. A. Rifkin, A. Van Oudenaarden and S. Tyagi, Nat. Methods, 2008, 5, 877–879 CrossRef PubMed
.
- D. Y. Vargas, A. Raj, S. A. E. Marras, F. R. Kramer and S. Tyagi, Proc. Natl. Acad. Sci. U. S. A., 2005, 102, 17008–17013 CrossRef PubMed
.
- D. K. Darnell, S. Stanislaw, S. Kaur and P. B. Antin, RNA, 2010, 16, 632–637 CrossRef PubMed
.
- B. D. Brown, M. A. Venneri, A. Zingale, L. S. Serge and L. Naldini, J. Nat. Med., 2006, 12, 585–591 CrossRef PubMed
.
- J. Lu and A. Tsourkas, Nucleic Acids Res., 2009, 37, e100 CrossRef PubMed
.
- R. J. Deng, L. H. Tang, Q. Q. Tian, Y. Wang, L. Lin and J. H. Li, Angew. Chem., Int. Ed., 2014, 53, 2389–2393 CrossRef PubMed
.
- Z. Cheglakov, T. M. Cronin, C. He and Y. Weizmann, J. Am. Chem. Soc., 2015, 137, 6116–6119 CrossRef PubMed
.
- S. Mukherji, M. S. Ebert, G. X. Y. Zheng, J. S. Tsang, P. A. Sharp and A. Van Oudenaarden, Nat. Genet., 2011, 43, 854–U60 CrossRef PubMed
.
- F. Porichis, M. G. Hart, M. Griesbeck, H. L. Everett, M. Hassan, A. E. Baxter, M. Lindqvist, S. M. Miller, D. Z. Soghoian, D. G. Kavanagh, S. Reynolds, B. Norris, S. K. Mordecai, Q. Nguyen, C. F. Lai and D. E. Kaufmann, Nat. Commun., 2014, 5, 5641 CrossRef PubMed
.
- H. W. Hwang, E. A. Wentzel and J. T. Mendell, Science, 2007, 315, 97–100 CrossRef PubMed
.
- K. T. Gagnon, L. Li, Y. Chu, B. A. Janowski and D. R. Corey, Cell Rep., 2014, 6, 211–221 CrossRef PubMed
.
- C. W. Park, Y. Zeng, X. Zhang, S. Subramanian and C. J. Steer, RNA Biol., 2010, 7, 606–614 CrossRef PubMed
.
- T. C. Roberts, Nucleic Acids, 2014, 3, e188 CrossRef PubMed
.
- A. K. Ranjan, M. V. Joglekar, A. N. Atre, M. Patole, R. R. Bhonde and A. A. Hardikar, RNA Biol., 2012, 9, 949–953 CrossRef PubMed
.
- M. Y. Wu, M. Piccini, C. Y. Koh, K. S. Lam and A. K. Singh, PLoS One, 2013, 8, e55044 CrossRef PubMed
.
- H. Koo, I. Park, Y. Lee, H. J. Kim, J. H. Jung, J. H. Lee, Y. Kim, J. H. Kim and J. W. Park, J. Am. Chem. Soc., 2016, 138, 11664–11671 CrossRef PubMed
.
- H. Tian, Y. Y. Sun, C. H. Liu, X. R. Duan, W. Tang and Z. P. Li, Anal. Chem., 2016, 88, 11384–11389 CrossRef PubMed
.
- W. H. Dai, H. F. Dong, K. K. Guo and X. J. Zhang, Chem. Sci., 2018, 9, 1753–1759 RSC
.
- J. Liu, D. Y. Yin, S. S. Wang, H. Y. Chen and Z. Liu, Angew. Chem., Int. Ed., 2016, 55, 13215–13218 CrossRef PubMed
.
- E. Van Rooij, L. B. Sutherland, J. E. Thatcher, J. M. Dimaio, R. H. Naseem, W. S. Marshall, J. A. Hill and E. N. Olson, Proc. Natl. Acad. Sci. U. S. A., 2008, 105, 13027–13032 CrossRef PubMed
.
- F. Y. Meng, R. Henson, H. Wehbe-Janek, K. Ghoshal, S. T. Jacob and T. Patel, Gastroenterology, 2007, 133, 647–658 CrossRef PubMed
.
- S. A. Jiang, H. W. Zhang, M. H. Lu, X. H. He, Y. Li, H. Gu, M. F. Liu and E. D. Wang, Cancer Res., 2010, 70, 3119–3127 CrossRef PubMed
.
- E. A. Mathe, G. H. Nguyen, E. D. bowman, Y. Q. Zhao, A. Budhu, A. J. Schetter, R. Braun, M. Reimers, K. Kumamoto, D. Hughes, N. K. Altorki, A. G. Casson, C. G. Liu, X. W. Wang, N. Yanaihara, N. Hagiwara, A. J. Dannenberg, M. Miyashita, C. M. Croce and C. C. Harris, Clin. Cancer Res., 2009, 15, 6192–6200 CrossRef PubMed
.
Footnote |
† Electronic supplementary information (ESI) available. See DOI: 10.1039/c8sc02533a |
|
This journal is © The Royal Society of Chemistry 2018 |