DOI:
10.1039/C8SC01611A
(Edge Article)
Chem. Sci., 2018,
9, 5372-5382
An ultrasensitive polydopamine bi-functionalized SERS immunoassay for exosome-based diagnosis and classification of pancreatic cancer†
Received
9th April 2018
, Accepted 21st May 2018
First published on 23rd May 2018
Abstract
Early diagnosis and metastasis monitoring for pancreatic cancer are extremely difficult due to a lack of sensitive liquid biopsy methods and reliable biomarkers. Herein, we developed easy-to-prepare and effective polydopamine-modified immunocapture substrates and an ultrathin polydopamine-encapsulated antibody-reporter-Ag(shell)–Au(core) multilayer (PEARL) Surface-Enhanced Raman Scattering (SERS) nano-tag with a quantitative signal of the Raman reporter at 1072 cm−1, which achieved ultrasensitive and specific detection of pancreatic cancer-derived exosomes with a detection limit of only one exosome in 2 μL of sample solution (approximately 9 × 10−19 mol L−1). Furthermore, by analyzing a 2 μL clinical serum sample, the migration inhibitory factor (MIF) antibody-based SERS immunoassay could not only discriminate pancreatic cancer patients (n = 71) from healthy individuals (n = 32), but also distinguish metastasized tumors from metastasis-free tumors, and Tumor Node Metastasis (TNM) P1–2 stages from the P3 stage (the discriminatory sensitivity was 95.7%). Thus, this novel immunoassay provides a powerful tool for the early diagnosis, classification and metastasis monitoring of pancreatic cancer patients.
Introduction
Pancreatic cancer is one of the most life-threatening malignancies worldwide, with a five-year survival rate of lower than 5% due to difficulties in early diagnosis and metastasis monitoring because the pancreas is relatively hidden and lacks specific biomarkers.1 Traditional biomarkers such as carcinoembryonic antigen (CEA) and cancer antigen 19-9 (CA19-9) have improved the diagnostic accuracy of pancreatic cancer,2 but their specificity for pancreatic cancer is low because of high CA19-9 expression in benign pancreatic diseases and increased CEA expression in colorectal cancer.3,4 Therefore, it is urgently required to establish new methods that improve the specificity and sensitivity of pancreatic cancer diagnosis.
As a “fingerprint” of their parental cells, exosomes, which are secreted vesicles 40–200 nm in diameter that are usually formed via the endosomal pathway and contain proteins, microRNAs and other non-coding RNAs, can reveal information about the metabolic state and degree of malignancy of parental cells.5,6 Therefore, research on exosomes has increased with the aim of using these extracellular vesicles for the diagnosis, therapy and mechanistic study of cancers and other diseases.7,8 Recent studies have reported two new biomarkers, glypican-1 (GPC-1)9 and ephrin type-A receptor 2 (EphA2), that are expressed on exosome surfaces.10 They then developed exosome-based nanotechnologies (nano-plasmonic nanohole arrays11 and multichannel nanofluidic systems12) and applied a new data analysis method (Machine Learning Algorithm12) for sensitive and specific diagnosis, classification and metastasis monitoring of pancreatic cancer. However, for the clinical application of these technologies, there are still some remaining challenges to solve: (1) more specific and reliable exosomes or extracellular vesicle biomarkers need to be screened; (2) a sensitive detection method that requires only a small volume of bio-samples should be developed to replace traditional methods such as flow cytometry or enzyme-linked immunosorbent assay (ELISA); and (3) a simple, fast and effective pretreatment method for clinical bio-samples should be developed to avoid the current time-consuming high-speed ultracentrifugation steps for exosome enrichment.
Based on our previous work on Surface-Enhanced Raman Scattering (SERS),13–15 in this study we developed an ultrasensitive SERS immunoassay that uses an ultra-small volume of serum for the exosome-based diagnosis, classification and metastasis monitoring of pancreatic cancer. As shown in Scheme 1, polydopamine (PDA) was self-polymerized16,17 on glass slides and specific antibodies (anti-MIF, anti-GPC1, anti-CD63, or anti-epidermal growth factor receptor (EGFR)) on the exosome surface were simultaneously encapsulated into the porous hydrophilic PDA layer. Then, exosomes derived from pancreatic cancer or healthy control samples were captured and enriched on the chip surface, followed by incubation with PDA encapsulated antibody-reporter-Ag(shell)–Au(core) multilayer (PEARL) SERS tags to form a “chip-exosome-PEARL tag” sandwich structure. The Raman spectrum was then scanned and the intensity of the Raman reporter at 1072 cm−1 was chosen as the quantitative signal. To our knowledge, this is the first time that the self-polymerization of dopamine has been used to capture antibodies on a substrate in combination with PEARL SERS nano-tags to construct an immunoassay. Based on this ingenious design and synthesis, this approach provided strong SERS signals for the ultrasensitive detection of exosomes in an ultra-small volume (2 μL) of clinical pancreatic serum samples, avoiding the time-consuming high-speed ultracentrifugation process. Furthermore, motivated by clinical needs, this liquid biopsy method distinguished metastatic tumors from non-metastatic tumors, and P1–2 stages from P3 stage tumors, without the need of histopathological examinations.
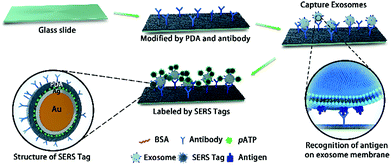 |
| Scheme 1 A schematic view of the PDA chip and PEARL SERS tag-based exosome sensors. | |
Results and discussion
Creating SERS sensors with PDA chips and PEARL tags
To develop sensitive and reliable SERS immunosensors for clinical pancreatic cancer diagnostics, we first employed a self-polymerizing PDA layer to simultaneously encapsulate and capture antibodies to increase the number of captured antibodies and maintain their bioactivity. The average thickness of the PDA layer is about 50–100 nm and the rough structure of the PDA surface (Fig. 1A and B) provided enough space for capturing antibodies. The PDA density (black dots) increased when the dopamine concentration increased from 16.5 to 66 mM (Fig. S1a†), resulting in an increased antibody capture efficiency (Fig. 1B and S1b†). The activity of captured antibodies was evaluated using Horseradish Peroxidase (HRP) as a model protein for capture due to its wide usage in commercial ELISA. As shown in Fig. S1c,† the activity of HRP decreased with increasing dopamine concentration, which suggested that more active sites of HRP were buried in the denser PDA layer. Finally, Raman spectroscopy was used to characterize the PDA surface. The optimum dopamine concentration was found to be 33 mM, as it generated the smallest interference Raman signal from PDA (Fig. S1d†). As one of the major concerns for this assay was quantitative accuracy, the reproducibility of the Raman signal was directly influenced by the homogeneity of the film area. Glass slides with and without PDA modification both displayed significant “coffee ring effects”, which showed the non-uniform adsorption of the SERS tag. In contrast, slides modified with antibodies had no “coffee ring effect” (Fig. 1C), which indicated that the modification of both PDA and antibodies synergistically improved the homogeneity of modified films due to the homogeneous capture of exosomes and good distribution of SERS tags. Compared with other antibody capture methods, such as physical adsorption on polystyrene 96-well plates or chemical covalent modification on magnetic beads,18,19 PDA encapsulation provided more biocompatible, mild and uniform surface modifications for high antibody capture efficiency and a high sensitivity for detecting cancer derived exosomes, which is the first essential factor for immunoassays.
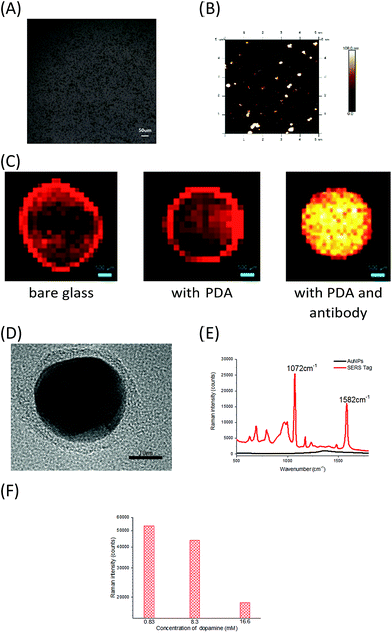 |
| Fig. 1 (A) Microscopy images of PDA chips formed with 33 mM dopamine. (B) AFM images of glass slides modified with PDA polymerized with 33 mM dopamine. (C) Raman images of sample points on slides with different modification steps (bare glass, with PDA, with PDA and antibodies) using anti-MIF SERS tags (785 nm excitation, scan area: 5 mm × 5 mm with a 100 μm scan step, and 0.1 s acquisition time for each scan point). (D) High-magnification TEM images of the final SERS tag. (E) SERS spectra of an Au nanoparticle solution (black line) and SERS tag solution (red line). (F) The influence of dopamine concentration on SERS intensity. | |
Secondly, the high sensitivity and stability of SERS tags play essential roles in the clinical application of SERS immunosensors.20,21 A SERS tag with high brightness, stability, and targeting capability is typically composed of four parts, including SERS nanostructures with a high enhancement factor, signal molecules that provide Raman signals, a signal protective layer with nanostructures, and a functional layer having a recognizable ability at the outermost layer of the material.22–24 Therefore, we designed and prepared PEARL SERS tags. Gold nanoparticles were chosen as the core, and silver, the Raman reporter molecule, BSA, PDA and antibodies were consecutively assembled onto the gold nanoparticle surface using the self-polymerization reaction of dopamine25–30 under a weak alkaline environment to form an ultra-thin (nanometer-thickness) protective and antibody encapsulating layer. The SERS tag has a distinct core–shell structure, with an approximately 1 nm-thick Ag shell and an approximately 3 nm-thick PDA shell, giving a total diameter of approximately 40 nm (Fig. 1D). As shown in Fig. 1E, the PEARL SERS tag had a very strong signal for the Raman reporter 4-aminobenzenethiol (pATP), with the peak at 1072 cm−1 contributed by the breathing vibration of the benzene ring and that at 1582 cm−1 arising from the C–N symmetric stretching vibration, while the gold nanoparticles showed no signal except for the capillary scattering background signal. To show the brightness of this SERS tag, extreme Raman excitation conditions of 0.05 mW laser power and 10 ms acquisition time (averaged 100 times) were set, and the spectrum was recorded (Fig. S1f†). Although the laser power and acquisition time were very low and short compared with normal test conditions (8 mW and 1 s), these test results also showed a distinct SERS spectrum of pATP, which indicated that the SERS tag had excellent Raman intensity and great potential for detecting trace biomarkers.
Importantly, PDA was not only used in the PEARL SERS tag as a protective shell to prevent oxidation of the Ag layer and the resulting decrease of the SERS signal, but also as an effective encapsulating reagent for detecting antibodies. The PDA thickness strongly influenced the stability and Raman intensity of the tags.31 The Raman intensity of the tags dropped significantly when the dopamine concentration increased (Fig. 1F), and the SERS tags grew too large, resulting in the sedimentation of nanoparticles when the solutions were rested for a few minutes. Based on these observations, the optimal dopamine concentration for forming the encapsulation layer of the SERS tags was set at 0.83 mM and the optimal thickness of the PDA layer was 3 nm, which is thinner than the 6 nm PDA-SERS Au tag for bone cracks.31 Our SERS tag with an ultra-thin PDA layer maintained the strong enhancement effect of Au–Ag nanomaterials and resulted in high SERS signal intensity. The PEARL tags were extremely stable and showed no decrease in the Raman signal for at least 6 months when stored at 4 °C.
Identification of candidate marker proteins on exosomes derived from pancreatic cells
To realize the clinical potential of this immunoassay for serum samples from pancreatic cancer patients, we qualitatively characterized exosomes derived from pancreatic cancer (PANC-01) and healthy cells (HPDE6-C7) by TEM. Exosomes derived from pancreatic cells showed a typical phospholipid bilayer structure (Fig. 2A and B). The diameters of exosomes from HPDE6-C7 cells were approximately 100 nm, and they were smaller than that of PANC-01-derived exosomes (140 nm). The secretory ability of adenocarcinoma cells was stronger than that of healthy cells.32–35 Owing to polymorphisms and irregularities in cancer cells,36,37 PANC-01 exosomes were less uniform than those from HPDE6-C7 cells. We further performed Nanoparticle Tracking Analysis (NTA) to quantify the number of exosomes. For NTA processing, exosomes were suspended in solution to prevent them from losing their biological functions and molecular structures. The distribution of particles smaller than 200 nm in diameter is shown in Fig. 2C. The concentration of exosomes was thus calculated to be 2.72 × 1010 ± 2.05 × 109 particles per mL, and the average size was 123.46 ± 26.93 nm, which was in agreement with the exosome sizes previously reported using TEM.38,39
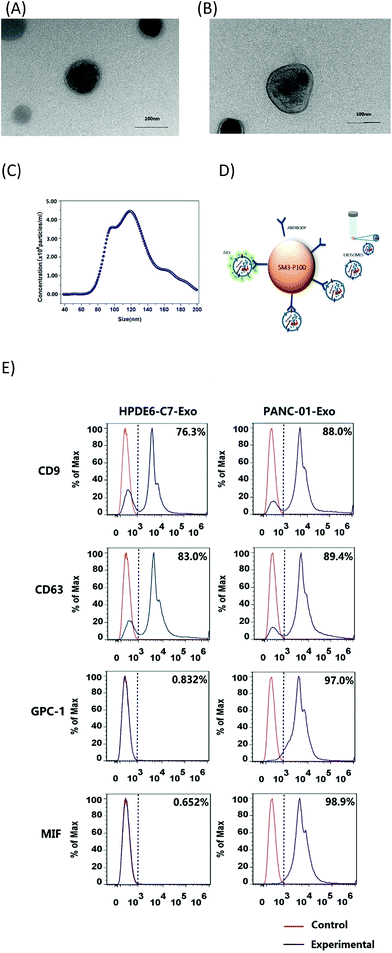 |
| Fig. 2 (A) TEM image of HPDE6-C7-derived exosomes. (B) TEM image of PANC-01-derived exosomes. (C) The distribution of the original exosome solution from PANC-01 cells by NTA. (D) Structure and formation of DIO-dyed SM3-P100-antibody-exosome complexes. (E) Relative CD9, CD63, GPC1 and MIF expression from PANC-01- (right side) and HPDE6-C7- (left side) derived exosomes. | |
To identify proteins commonly expressed on exosome membranes (such as CD9 and CD63) and specific pancreatic cancer-derived exosome proteins (such as GPC1 and MIF), supermagnetic beads with the corresponding antibodies were used to capture exosomes (Fig. 2D), and then the exosome membranes were dyed with 3,3′-dioctadecyloxacarbocyanine perchlorate (DIO) and analyzed by flow cytometry. Goat anti-mouse IgG was used as the control sample. As shown in Fig. 2E, CD9 was expressed on 88.0% and 76.3% of exosomes from PANC-01 and HPDE6-C7 cells, respectively, CD63 was expressed on 89.4% and 83.0%, respectively, GPC1 was found on 97.0% and 0.832%, respectively, and MIF was found on 98.9% and 0.652%, respectively, indicating that there was a significant increase in exosomal GPC1 and MIF expression in PANC-01 cells compared with the healthy HPDE6-C7 cells. Meanwhile, CD9 and CD63 expressions were similar in the two groups. These results suggested that MIF and/or GPC1 expression might distinguish exosomes from pancreatic cancer cells and normal pancreas cells. This conclusion was consistent with previous studies that showed that GPC1 and MIF were dramatically overexpressed in the serum from pancreatic cancer patients, and thus could be used as biomarkers to distinguish early-stage cancer from benign disease and/or predict tumor metastasis or tumor burden.9,40
Ultrasensitive exosome detection based on the chip-exosome-PEARL tag immunoassay
Based on our developed PDA chips, PEARL tags and the identified pancreatic cancer exosome-specific surface proteins mentioned above, we designed an exosome assay for pancreatic cancer (Scheme 1). Typically, 2 μL of PANC-01- or HPDE6-C7-derived exosome solutions of different concentrations were dropped onto the PDA chips, followed by adding the PEARL SERS tag solution. The homogeneous encapsulation of antibodies on the PDA chip was found to contribute to the uniformity of the sample points on the chip. We designed four experimental groups using four different antibodies: anti-CD9, anti-CD63, anti-MIF and anti-GPC1. For each group, the antibodies on the PDA chips and the PEARL SERS tag were the same. For different antibody-based platforms, we dropped exosome solution onto different spots on the PDA modified glass slide, not onto a single spot for all antibodies. The Raman peak at 1072 cm−1 was chosen as the quantitative signal, because it was one of the three strongest peaks in the spectrum and there was almost no interference from other impure peaks near the 1072 cm−1 peak. In PANC-01 exosomes, the intensities of the anti-CD9, anti-CD63, anti-GPC1 and anti-MIF groups were 1233, 3597, 2659 and 4455, respectively, while for HPDE6-C7 exosomes the respective intensities were 1240, 3414, 1024, and 648 (Fig. 3A and B). Interestingly, the CD63 intensity was higher than that of GPC1, which was not in accordance with the flow cytometry results. The reason for this discrepancy was that in flow cytometry, the membranes of captured exosomes were DIO-stained to facilitate counting the number of exosomes, while in the PDA chip, the exosomes were labeled with the PEARL tag, which was recognized by antigen epitopes on the exosomes. The number of CD63 antigens on each exosome membrane was larger than that of GPC-1, which resulted in stronger Raman intensity. Regardless, we observed that the intensities of anti-CD9 and anti-CD63 groups were similar, while there were significant differences between HPDE6-C7-exosomes and PANC-01-exosomes in the anti-GPC1 and anti-MIF groups (Fig. 3B). Compared with flow cytometry, which requires large amounts of expensive antibodies, our PDA-SERS method only requires about one fortieth of the amount of antibody. Using our PDA-SERS method, a higher SERS signal and a larger signal difference between PANC-01- and HPDE6-C7-derived exosomes were obtained using the MIF antibody than the GPC1 antibody, which was consistent with the finding that MIF is more highly expressed on the exosomes from pancreatic cancer patients than those from healthy individuals. Moreover, MIF is markedly higher in exosomes from stage I pancreatic ductal adenocarcinoma patients who later developed liver metastases than from patients whose pancreatic tumors did not progress.34,36,40,41 Exosomal MIF primes the liver for metastasis and may be a prognostic marker for the development of pancreatic ductal adenocarcinoma (PDAC) liver metastases. MIF is a well-known mediator of liver inflammation and fibrosis,42 bone marrow cell recruitment to the liver, and liver metastasis. MIF tissue and plasma levels correlate with PDAC aggressiveness.43,44 To our knowledge, this is the first time that a sensitive and stable PDA-SERS methodology has been used in exosome research. Additionally, MIF-based exosome detection was performed for the first time, except for using the conventional ELISA method. A recent study reported that exosomal GPC1 was a potential biomarker for diagnosing pancreatic cancer.9 Unfortunately, the previously used GPC1 antibody is no longer commercially available. Thus, we suspect there are some differences between the GPC1 antibodies from the two different companies.
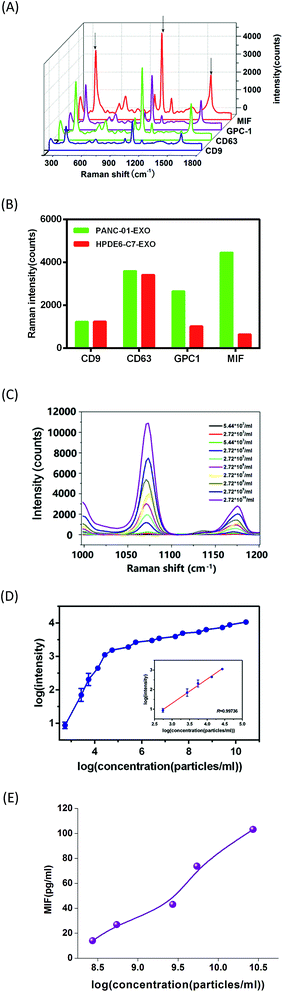 |
| Fig. 3 (A) SERS spectra of the anti-CD9, CD63, MIF and GPC1 groups for PANC-01-derived exosomes. (B) Comparison of the intensities at 1072 cm−1 of the anti-CD9, CD63, MIF and GPC1 groups for PANC-01- and HPDE6-C7-derived exosomes. (C) The Raman spectra (from 1000–1200 cm−1) for different PANC-01 exosome concentrations (5.44 × 102 to 2.72 × 1010 particles per mL). (D) Variation of the SERS intensity of MIF at 1072 cm−1 with PANC-01 exosome concentration. The inset shows the linear relationship between SERS intensity and PANC-01 exosome concentration (5.44 × 102 to 2.72 × 104 particles per mL). For each concentration, the experiments were repeated 3 times. (E) Correlation curve between MIF and PANC-01 exosome concentration using a commercial ELISA kit. | |
We further used anti-MIF to capture PANC-01-derived exosomes at different concentrations (5.44 × 102 to 2.72 × 1010 particles per mL), while the control sample was PBS without exosomes. The results showed that the SERS signal intensity increased with increasing exosome concentration (Fig. 3C and D). There was a good linear fit for log(intensity) and log(exosome concentration) between 5.44 × 102 and 2.72 × 104 particles per mL, with the limit of detection (LOD) being approximately 9 × 10−19 mol L−1 (S/N = 3). There was only one exosome in a 2 μL exosome sample of 5.44 × 102 particles per mL. The LOD is three orders of magnitude lower than that of the most sensitive exosome detection methods currently reported, such as Au–Ag nanorods with an SERS reporter (LOD: 1200 exosomes),18 super-hydrophobic surfaces decorated with nano-geometry-based photonic structures to detect exosomes on SERS (0.2 ng mL−1),45 electrochemical impedance spectroscopy (9500 exosome particles per 50 μL)46 and size exclusion chromatography with fluorescence detection (2.9 × 107 exosome particles per mL).47 The MIF concentration of the PANC-01 exosome solution was also detected using a commercial Human MIF ELISA kit (argb1294; Arigo Biolaboratories, Hsinchu City, Taiwan). As shown in Fig. 4E, the detection limit of our PDA SERS tag method was almost 6-fold lower than that of the commercial ELISA kit, which was about 2.72 × 108 particles per mL. The excellent sensitivity of this PDA-SERS method undoubtedly results from the PDA on glass slides and the core–shell Au–Ag SERS nano-tags and has enough hydrophilic antibody binding sites and optimal protective function for Ag shells. Compared with the ultra-stable silica shell protection method, which is one of the finest protective modification methods,48,49 the PDA shell has the advantages of being easily modified, environmentally friendly, and having great biocompatibility. This supersensitive MIF SERS platform can analyze individual exosomes and distinguish pancreatic cancer derived exosomes from those of healthy cells, which is valuable for subcellular mechanistic research and for clinical supervision or therapy in pancreatic cancer.
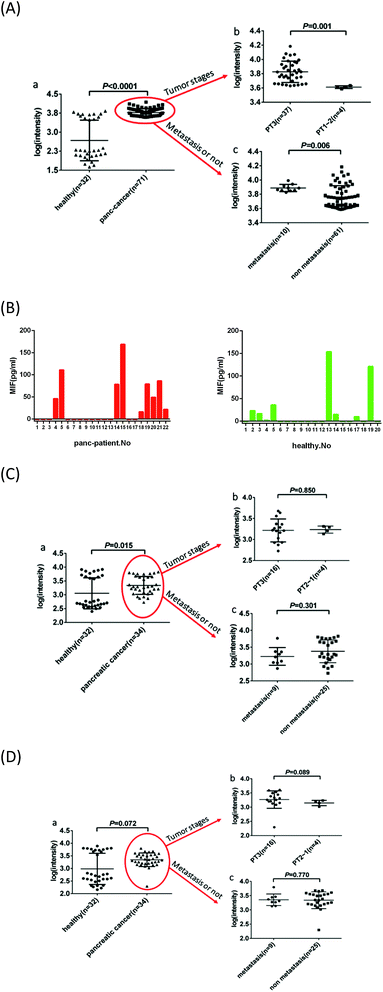 |
| Fig. 4 (A) Shapiro–Wilk analysis plots of the SERS results of serum samples of pancreatic cancer patients (n = 71) and healthy controls (n = 32) using the anti-MIF platform. The ordinate represents log values of Raman intensity; we subtracted the intensity of control samples from experimental samples. (B) Waterfall plots of pancreatic cancer patients (n = 22) and healthy controls (n = 20) using commercial ELISA kit results. The ordinate represents MIF concentrations in pancreatic cancer patients or healthy individuals, and the abscissa represents the number of subjects involved in the study. Shapiro–Wilk analysis plots of the SERS results from 32 healthy individuals and 34 pancreatic cancer patients tested with (C) anti-GPC1 and (D) anti-EGFR platforms. | |
To compare the detection sensitivity with other antibodies, anti-GPC1, EGFR, CD63 and EpCAM SERS assays were also applied to test exosomes derived from PANC-01 cells at various concentrations (5.44 × 102 to 2.72 × 104 particles per mL). The results of these assays are summarized in Table S1.† The immunosensors based on anti-GPC1, EGFR and EpCAM all showed good linear fitting with R values (multiple correlation coefficient) of >0.99, P values (probability) <0.05 and a similar LOD as the anti-MIF immunosensor (Fig. S6a, b and d†). In contrast, the anti-CD63 immunosensor displayed slightly poorer linear fitting (R < 0.92 and P > 0.05) and had a higher LOD (Fig. S6c†).
Validation of chip-exosome-PEARL SERS immunosensors in clinical serum samples from pancreatic cancer patients
71 serum samples from histologically diagnosed pancreatic cancer patients and 32 samples from healthy individuals were assayed using this immunosensor. Serum samples were diluted 3-fold with PBS, followed by filtration with a 0.22 μm filter. Then, 2 μL of diluted sample was added to the PDA chip encapsulated with the anti-MIF antibody and detected with PEARL SERS tags. For the control group 2 μL of PBS without serum was used, and the intensity acquired was subtracted from the intensity of the experimental groups. The results are shown as log(intensity) in Fig. 4A. The Shapiro–Wilk test showed that Wcontrol = 0.806, P < 0.0001 and Wexperiment = 0.916, P < 0.0001, indicating a non-normal distribution in both groups. A test of homogeneity of variances showed F = 314.177, P < 0.0001. The comparison of the pancreatic cancer and healthy control groups was measured by two independent samples' non-parametric Mann–Whitney test, Z = −6.257, P < 0.0001, which showed that there was a statistical difference. The intensities of the pancreatic cancer and healthy control groups (mean ± SD) were 3.77 ± 0.15 and 2.67 ± 0.80, respectively. In the pancreatic cancer group, the median was 3.7542 and the interquartile range was 0.25, while in the healthy control group, the median was 2.2785 and the interquartile range was 1.54. These results indicated that the MIF SERS-PEARL immunosensor distinguished the serum of pancreatic cancer patients from that of healthy individuals, and also provided proof for the clinical reference range, which makes it a promising method with sufficient basis for clinical application.
Furthermore, we employed statistical methods to obtain more diagnostic information from the anti-MIF SERS-exosome immunosensor results, such as distinguishing different Tumor Node Metastasis (TNM) classification stages (if the patients' cancers had TNM staging), and metastasis from non-metastasis according to their histopathological reports (Table S2†). We divided the 41 pancreatic cancer samples with defined TNM stages (omitting those without TNM staging) into P3 and P1–2 subgroups and further compared their log(intensity). We classified the pancreatic cancer samples into “metastasis” and “non-metastasis” groups; the former included metastases to the liver, hilum of the spleen, adrenal glands and lymph nodes, while the latter contained tumors that had infiltrated into tissues around the pancreas, such as adipose tissue, nerves, and extra-pancreatic tissues, such as the duodenal submucosal layer and bile duct. These statistical results are shown in Table S3.† All Mann–Whitney test values for P1–2 and P3, and metastasis and non-metastasis groups were statistically significant and matched the histopathological reports. Surprisingly, this method could also discriminate between patients with P3-stage tumors and those with P1–2-stage tumors, meaning that it can supplement tumor staging to further realize accurate diagnoses. For comparison, serum samples of pancreatic cancer patients (n = 22) and healthy controls (n = 20) were also tested using the commercial Human MIF ELISA kit. As shown in Fig. 4B, only nine and eight serum samples gave positive results from patients and healthy controls, respectively. Thus, the comparison between our anti-MIF SERS immunosensor and a commercially available ELISA kit indicated that our analytical platform had significant advantages for analyzing small-volume serum samples.
Along with MIF, GPC1 and EGFR were also highly expressed on exosome surfaces.50,51 Therefore, to determine the most powerful antibody for accurate and sensitive diagnosis, anti-GPC1- and anti-EGFR-based chip-exosome-PEARL SERS immunosensors were also applied to the same serum samples of pancreatic cancer patients (n = 34) and healthy controls (n = 32). The Shapiro–Wilk test of GPC1 (Fig. 4C) and EGFR (Fig. 4D) and W/P values (Tables S4 and S5,† respectively) showed that the distribution of both experimental and control groups was non-normal, similar to anti-MIF. The anti-GPC1 platform could distinguish healthy individuals from pancreatic cancer patients, but anti-EGFR could not. Furthermore, neither test could distinguish P3 from P1–2 tumors, nor could they distinguish the “metastasis” and “non-metastasis” subgroups.
To estimate whether MIF, GPC1 and EGFR could constitute a more discriminatory panel for the clinical diagnosis of pancreatic cancer, TNM staging and metastasis, we performed a receiver operating characteristic (ROC) logistic regression (Fig. 5A) to determine the sensitivity, specificity and accuracy (Table 1) of using exosome markers individually. The ability of the MIF-based immunosensor for discriminating between pancreatic cancer and healthy controls, metastasis and non-metastasis, and P1–2 and P3 was much higher than that of GPC-1- and EGFR-based immunosensors. Notably, the MIF discriminatory sensitivity was 95.7% for early-stage pancreatic cancer (P1–2) versus P3, which further demonstrated the potential of MIF as a promising exosome marker for pancreatic cancer.
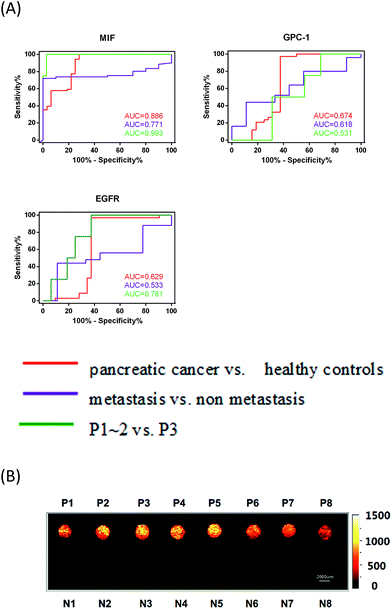 |
| Fig. 5 (A) Receiver operating characteristic (ROC) curves were calculated for single exosome markers (MIF, GPC-1 and EGFR) (red: pancreatic cancer vs. healthy controls; purple: metastasis vs. non-metastasis; and green: P1–2 vs. P3). AUC stands for the area under the curve. (B) Raman imaging scanning of the 7.2 × 1.8 cm chip containing serum samples from pancreatic cancer patients (P1–8) and healthy individuals (N1–8) tested using the anti-MIF platform. | |
Table 1 Statistical analyses of exosome markers (MIF/GPC1/EGFR) for pancreatic and healthy serum samples
Biomarkers |
Groups |
Cutoffa |
AUC(95% CI) |
Sensitivity(95% CI) |
Specificity(95% CI) |
Presented in log(Raman intensity) formation. Panc: pancreatic cancer, H: healthy, Px: patient with pancreatic cancer in stage x(x = 1–3), M: metastasis, NM: non-metastasis, AUC: the area under the ROC curve, and CI: confidence interval.
|
MIF |
Panc(n = 71) vs. H(n = 32) |
3.546 |
0.886(0.811–0.962) |
0.625(0.563–0.687) |
0.762(0.710–0.813) |
P3(n = 37) vs. P1–2(n = 4) |
3.854 |
0.993(0.973–1.014) |
0.957(0.911–1.004) |
0.536(0.436–0.636) |
M(n = 10) vs. NM(n = 61) |
3.757 |
0.771(0.668–0.875) |
0.533(0.464–0.601) |
0.739(0.648–0.831) |
GPC1 |
Panc(n = 34) vs. H(n = 32) |
3.848 |
0.674(0.524–0.823) |
0.592(0.501–0.683) |
0.582(0.525–0.639) |
P3(n = 16) vs. P1–2(n = 4) |
2.726 |
0.531(0.270–0.792) |
0.550(0.366–0.734) |
0.481(0.352–0.610) |
M(n = 9) vs. NM(n = 25) |
3.266 |
0.618(0.415–0.821) |
0.546(0.447–0.645) |
0.572(0.457–0.687) |
EGFR |
Panc(n = 34) vs. H(n = 32) |
3.657 |
0.629(0.469–0.789) |
0.570(0.476–0.664) |
0.559(0.503–0.615) |
P3(n = 16) vs. P1–2(n = 4) |
3.385 |
0.781(0.578–0.984) |
0.750(0.593–0.907) |
0.531(0.392–0.671) |
M(n = 9) vs. NM(n = 25) |
3.589 |
0.533(0.319–0.747) |
0.524(0.425–0.622) |
0.510(0.391–0.629) |
The differential performances of the PDA-SERS (combined MIF-, GPC1- and EFGR-based platforms), and CEA- and CA19-9-based ELISA assays (Fig. S8†) are summarized in Table 2. As tumors develop, cancer cells can infiltrate into the surrounding tissues, disrupting tissue homeostasis and causing organ dysfunction. As the tumor architecture deteriorates, cells can enter the circulatory system and relocate to remote organs, which is the primary cause of cancer-related deaths. Our MIF SERS-PEARL liquid biopsy platform was also able to distinguish metastasized tumors from non-metastasized ones without the need of tissue biopsy or MRI imaging. Thus, patients with and without metastases could be identified and monitored throughout the following treatments, and this information could be incorporated when making treatment decisions. To our knowledge, this is the first time that MIF-based liquid biopsy was used to differentiate tumors by stage and metastatic activity. Furthermore, only 2 μL of serum sample was required for SERS analysis, demonstrating that micro-volume detection can be realized.
Table 2 The diagnosis results of different platforms
Analytical platform |
Cancer vs. healthy |
PT1–2 vs. PT3 |
Metastasis vs. non-metastasis |
Our work.
The clinical test.
Commercial kit; “○”means successfully classified; “X” means unclassified.
|
Anti-MIF SERSa |
○ |
○ |
○ |
Anti-GPC1 SERSa |
○ |
X |
X |
Anti-EGFR SERSa |
X |
X |
X |
CA19-9b |
X |
X |
X |
CEAb |
X |
X |
X |
MIF ELISA kitc |
X |
X |
X |
Additionally, our chip-based exosome-PEARL SERS immunosensor offered more intuitionistic ways to discriminate cancer patients from healthy individuals by Raman imaging techniques with tremendous progress in spectral acquisition speed, detection sensitivity, spatial resolution, and penetration depth.52–56 Briefly, the exosomes captured and SERS tag-labeled chip was fully scanned on its entire surface (7.2 × 1.8 cm2) with a 200 μm step between each point. The anti-MIF-SERS chip containing eight pancreatic cancer samples and eight healthy samples was scanned in about 1 h. Then, the scanning results were analyzed by calculating the peak area of every point in the peak range (1045–1100 cm−1) and a color gradient was given to reveal the intensities. In this work, a brighter color in Raman imaging means more SERS tags, representing more antigens and exosomes. From the SERS images (Fig. 5B), we directly distinguished pancreatic cancer patients (bright spots) from healthy individuals (dark spots). Furthermore, the thresholds provided herein can serve as references for clinical applications.
Conclusions
PDA-modified glass slides and an ultra-thin PDA layer encapsulated Au(core)–Ag(shell) SERS tag with a quantitative signal of the Raman reporter at 1072 cm−1 were constructed for the sensitive and specific detection of pancreatic cancer-derived exosomes to clinically diagnose tumors and metastases. The MIF antibody-based PDA-SERS platform can detect exosomes in trace samples with the lowest detection limit down to one exosome, making it much more sensitive than previously reported methods. Clinical serum samples from pancreatic cancer patients and healthy individuals could be clearly differentiated using MIF-, GPC1-, and EGFR-based PDA-SERS methods, with the requirement of only 2 μL serum samples. Furthermore, the MIF-based method could distinguish metastatic tumors from those without metastases, and P1–2-stage tumors from those in the P3 stage, which could previously only be accomplished by surgical biopsy. Thus, this immune-based SERS analytical platform might be able to detect early cancerous lesions to improve therapeutic outcomes and patient lives.
This study was designed to show the feasibility of the PDA-SERS method for diagnosing cancer. This method can be further expanded to simultaneous and multiplex target assays with high diagnostic accuracy by Raman imaging. Protein microarray and microfluidic chip technologies are also compatible with this PDA-SERS method for high throughput and fast liquid biopsy of exosomes, tumor-derived extracellular vesicles, circulating DNAs, and most other biologically relevant molecules. We believe that the clinical application of these liquid biopsy methods will greatly relieve the distress caused by histopathological tests, and will provide a promising future for early diagnosis and efficient therapy for cancer patients.
Experimental
Materials and methods
Materials.
Dopamine hydrochloride, N-(3-dimethylaminopropyl)-N′-ethylcarbodiimide hydrochloride, N-hydroxysuccinimide, MES monohydrate, bovine serum albumin, Tween® 20, 4-aminobenzenethiol (pATP), trisodium citrate, hydrogen tetrachloroaurate (HAuCl4·3H2O), dopamine, silver nitrate, and other chemical reagents were from Sigma-Aldrich, United States. Sodium hydroxide and hydrogen peroxide solution were from Macklin Biochemical Co. Ltd., Shanghai, China. Concentrated sulfuric acid (98%) was from Sinopharm Chemical Reagent Limited Corporation, China. 3,3′-Dioctadecyloxacarbocyanine perchlorate was from Beyotime, Shanghai, China. RPMI 1640 Medium, DMEM, Fetal Bovine Serum (FBS), Phosphate-Buffered Saline (PBS), pH 7.4, Tris–HCl, pH 8.0, and trypsin–EDTA (0.05%) phenol red were from Thermo Fisher Scientific, United States. Ethanol was from AoRui Biotechnology Company, Shanghai, China. Anti-CD9 antibody, anti-CD63 antibody, anti-MIF antibody, anti-GPC1 antibody, and goat anti-mouse IgG H&L (FITC) were purchased from ABCAM company, United States. AllMag® SM3-P100 superparamagnetic nanoparticles were from Shanghai Allrun Nano Science & Technology Co., Ltd, China. The PANC-01 cell line was from the cell bank of the University of Chinese Academy of Sciences. HPDE6-C7 was obtained from the American Type Culture Collection.
Synthesis of SERS-labelled nanomaterials.
The 18 nm gold nanoparticles were synthesized using Frens' protocol.57 The Au–Ag core–shell nanocomposites were synthesized by the following steps: 600 μL of gold nanoparticle solution was put in a clean round flask with stirring; then 20 μL of 0.1 M ascorbic acid, 5 μL of silver nitrate (appropriate concentration), 100 μL of Tris–HCl buffer (50 mM, pH = 8.5), 100 μL of pATP aqueous solution (appropriate concentration), and 200 μL of 1% BSA were added stepwise. After the mixture had reacted for 30 min, the solution was centrifuged at 6000 rpm for 15 min; then the supernatant was removed, and the precipitate was re-dispersed in 500 μL of Tris–HCl buffer. The re-dispersed solution was combined with 100 μL of 15 μg mL−1 antibody and 100 μL of dopamine solution (appropriate concentration), and the reaction lasted 1 h. After the reaction completed, the solution was centrifuged, and the precipitate was re-dispersed. The final solution was stored at 4 °C until use.
Modification of the polydopamine chip.
Glass slides (24.5 × 76.2 mm2, 1–2 mm thick) were soaked in Piranha solutions for 2 h, and then washed with deionized water. Then, several glass slides were put into 20 mL of a dopamine hydrochloride solution of the appropriate concentration for 1.5 h, and then 20 mL of 1 M Tris–HCl (pH = 8.0) with 15 μg antibody was added and reacted for approximately 1.5 h. The polydopamine chips were washed with PBS for further exosome detection.
Cell culture.
PANC-01 and HPDE6-C7 cells were cultured in RPMI 1640 and DMEM, respectively, with 10% FBS, at 5% CO2 in culture bottles. The FBS used in this study was filtered through a 0.22 μm filter (Merck Millipore, Burlington, MA, USA) and then centrifuged for 16 h twice to make it exosome-free.
Exosome separation.
Culture medium from the two cell lines was collected and the ultracentrifugation process was performed in an ultracentrifuge (CS150FNX; Hitachi, Tokyo, Japan): the medium was centrifuged at 800 × g for 5 min and 2000 × g for 10 min to remove cellular debris; after filtering through a 0.22 μm filter to acquire the exosomes, the medium was centrifuged at 120
000 × g for 4 h, and finally the exosomes were diluted in PBS and centrifuged at 120
000 × g for 4 h twice. The separated exosomes were then suspended in PBS to the desired concentration. For serum samples for transmission electron microscopy (TEM) characterization, the exosomes were diluted to the appropriate concentration, filtered through the 0.22 μm filter, and then ultracentrifuged at 120
000 × g for 4 h and washed twice with PBS.
Exosome detection using the SERS method.
Before capture, the polydopamine chip was blocked with 0.05% BSA at 37 °C for 30 min, and then it was washed with PBS and PBS-Tween20 (PBST). The original exosome solution from PANC-01 and HPDE6-C7 cells was diluted to the appropriate concentration, and then 2 μL of the exosome solution was dropped on the polydopamine chip, followed by incubation for 1 h at 37 °C. After incubation, the chip was washed with PBS and PBST; then, 3 μL of PEARL was dropped on the sample to cover it, and the chip was incubated for 1 h at 37 °C, and then washed with PBS and PBST. Raman signals were collected on a Horiba Jobin Yvon XploRA confocal micro-Raman system, and the excitation laser wavelength was 785 nm. Labspec software (version 6) was used to obtain the average Raman intensity of the samples and mapping images. The signal intensities of the different samples were obtained by averaging approximately 196 test point signals in a 250 × 250 μm2 square region (testing step: approximately 19.2 μm, and 1 s for each point) with a laser power of 8 mW. The Raman mapping images of PDA chips were obtained by plotting the Raman peak areas in a 7.2 × 1.8 cm2 oblong region (mapping step: 200 μm, and 0.1 s for each point) with a laser power of 80 mW. The Raman peak area was used to set the false color mapping scale and the scale value was set from 20 to 100.
Patient samples.
The serum samples of 71 patients diagnosed with pancreatic ductal adenocarcinoma and 32 samples from healthy volunteers were collected between December 2012 and August 2016 at Changhai Hospital, Shanghai, China, with written informed consent. All experiments on clinical samples were performed in accordance with the Guidelines for Care and Use of Laboratory Clinical Blood Samples of Changhai Hospital, Shanghai, China and were approved by the Medical Research Ethics Committee, Changhai Hospital, Shanghai, China. The average age of the pancreatic ductal adenocarcinoma patients was 60.08 ± 9.81 years, and there were 33 women and 38 men; for the healthy group, the average age was 50.25 ± 13.55 years, and it comprised 10 women and 22 men. Samples with complex tumors, including pancreatic cancer with other cancers were excluded from this study.
Statistical analysis.
Comparisons of measurement data among more than three groups were made by an LSD-t test, if the data met the homogeneity of variance (P > 0.05) and normality distribution (Shapiro–Wilk test P > 0.1) requirements. Comparisons between the pancreatic cancer group and the healthy group were made by two independent samples' non-parametric Mann–Whitney test. The test level for LSD-t and M–W was 0.05, while for the Shapiro–Wilk test it was 0.1. Receiver operating characteristic curves were obtained using Graph Prism 6.0. Sensitivity and specificity results were calculated using IBM SPSS Statistics 21.0; the cut-off value was log(Raman intensity) where sensitivity − (1 − specificity) was the max. The combined values of MIF, GPC1, and EGFR were calculated by the logistic regression method. Other statistical results or graphs were also from Graph Prism 6.0, IBM SPSS Statistics 21.0 and Origin 7.5.
Conflicts of interest
There are no conflicts to declare.
Acknowledgements
We thank our clinical colleagues involved in the clinical care of the patients reported here. We are thankful for the financial support from the National Natural Science Foundation of China (21375027, 21335002, and 21427806), Shanghai Pujiang Program (17PJD001) and Natural Science Foundation of Shanghai (12ZR1401700).
Notes and references
- W. Chen, R. Zheng, P. D. Baade, S. Zhang, H. Zeng, F. Bray, A. Jemal, X. Q. Yu and J. He, Ca-Cancer J. Clin., 2016, 66, 115–132 CrossRef PubMed
.
- J. Kim, W. R. Bamlet, A. L. Oberg, K. G. Chaffee, G. Donahue, X. J. Cao, S. Chari, B. A. Garcia, G. M. Petersen and K. S. Zaret, Sci. Transl. Med., 2017, 9, eaah5583 CrossRef PubMed
.
- J. Datta and C. M. Vollmer Jr, South. Med. J., 2014, 107, 256–263 CrossRef PubMed
.
- K. S. Goonetilleke and A. K. Siriwardena, Eur. J. Surg. Oncol., 2007, 33, 266–270 CrossRef PubMed
.
- Z. Nuzhat, V. Kinhal, S. Sharma, G. E. Rice, V. Joshi and C. Salomon, Oncotarget, 2017, 8, 17279–17291 CrossRef PubMed
.
- H. L. Shao, J. Chung, L. Balaj, A. Charest, D. D. Bigner, B. S. Carter, F. H. Hochberg, X. O. Breakefield, R. Weissleder and H. Lee, Nat. Med., 2012, 18, 1835–1840 CrossRef PubMed
.
- J. M. Hu, Y. Sheng, K. J. Kwak, J. F. Shi, B. H. Yu and L. J. Lee, Nat. Commun., 2017, 8, 1683 CrossRef PubMed
.
- Y. Yoshioka, N. Kosaka, Y. Konishi, H. Ohta, H. Okamoto, H. Sonoda, R. Nonaka, H. Yamamoto, H. Ishii, M. Mori, K. Furuta, T. Nakajima, H. Hayashi, H. Sugisaki, H. Higashimoto, T. Kato, F. Takeshita and T. Ochiya, Nat. Commun., 2014, 5, 3591 CrossRef PubMed
.
- S. A. Melo, L. B. Luecke, C. Kahlert, A. F. Fernandez, S. T. Gammon, J. Kaye, V. S. LeBleu, E. A. Mittendorf, J. Weitz, N. Rahbari, C. Reissfelder, C. Pilarsky, M. F. Fraga, D. Piwnica-Worms and R. Kalluri, Nature, 2015, 523, 177–182 CrossRef PubMed
.
- K. Liang, F. Liu, J. Fan, D. Sun, C. Liu, C. J. Lyon, D. W. Bernard, Y. Li, K. Yokoi, M. H. Katz, E. J. Koay, Z. Zhao and Y. Hu, Nature Biomedical Engineering, 2017, 1, 0021 CrossRef PubMed
.
- K. S. Yang, H. Im, S. Hong, I. Pergolini, A. F. del Castillo, R. Wang, S. Clardy, C.-H. Huang, C. Pille, S. Ferrone, R. Yang, C. M. Castro, H. Lee, C. F. del Castillo and R. Weissleder, Sci. Transl. Med., 2017, 9, eaal3226 CrossRef PubMed
.
- J. Ko, N. Bhagwat, S. S. Yee, N. Ortiz, A. Sahmoud, T. Black, N. M. Aiello, L. McKenzie, M. O'Hara, C. Redlinger, J. Romeo, E. L. Carpenter, B. Z. Stanger and D. Issadore, ACS Nano, 2017, 11, 11182–11193 CrossRef PubMed
.
- X. Gao, Q. Yue, Z. Liu, M. Ke, X. Zhou, S. Li, J. Zhang, R. Zhang, L. Chen, Y. Mao and C. Li, Adv. Mater., 2017, 29(21), 1603917 CrossRef PubMed
.
- C. L. Sun, R. Zhang, M. X. Gao and X. M. Zhang, Anal. Bioanal. Chem., 2015, 407, 8883–8892 CrossRef PubMed
.
- Y. F. Zhan, H. R. Liu, J. L. Tang, Z. Y. Li, X. Y. Zhou, R. Zhang, L. Chen, Y. Mao and C. Li, ACS Appl. Mater. Interfaces, 2017, 9, 17769–17776 Search PubMed
.
- P. Zhang, M. He and Y. Zeng, Lab Chip, 2016, 16, 3033–3042 RSC
.
- J. Ryu, S. H. Ku, H. Lee and C. B. Park, Adv. Funct. Mater., 2010, 20, 2132–2139 CrossRef
.
- S. F. Zong, L. Wang, C. Chen, J. Lu, D. Zhu, Y. Z. Zhang, Z. Y. Wang and Y. P. Cui, Anal. Methods, 2016, 8, 5001–5008 RSC
.
- K. Yang, Y. J. Hu and N. Dong, Biosens. Bioelectron., 2016, 80, 373–377 CrossRef PubMed
.
- M. Li, J. W. Kang, S. Sukumar, R. R. Dasari and I. Barman, Chem. Sci., 2015, 6, 3906–3914 RSC
.
- M. Li, S. R. Banerjee, C. Zheng, M. G. Pomper and I. Barman, Chem. Sci., 2016, 7, 6779–6785 RSC
.
- Y. Q. Wang, B. Yan and L. X. Chen, Chem. Rev., 2013, 113, 1391–1428 CrossRef PubMed
.
- H. Zhang, C. Wang, H. L. Sun, G. Fu, S. Chen, Y. J. Zhang, B. H. Chen, J. R. Anema, Z. L. Yang, J. F. Li and Z. Q. Tian, Nat. Commun., 2017, 8, 15447 CrossRef PubMed
.
- S. Pal, S. Harmsen, A. Oseledchyk, H. T. Hsu and M. F. Kircher, Adv. Funct. Mater., 2017, 27, 1606632 CrossRef PubMed
.
- D. J. Miller, D. R. Dreyer, C. W. Bielawski, D. R. Paul and B. D. Freeman, Angew. Chem., Int. Ed., 2017, 56, 4662–4711 CrossRef PubMed
.
- M. Y. Liu, G. J. Zeng, K. Wang, Q. Wan, L. Tao, X. Y. Zhang and Y. Wei, Nanoscale, 2016, 8, 16819–16840 RSC
.
- S. K. Madhurakkat Perikamana, J. Lee, Y. B. Lee, Y. M. Shin, E. J. Lee, A. G. Mikos and H. Shin, Biomacromolecules, 2015, 16, 2541–2555 CrossRef PubMed
.
- M. E. Lynge, P. Schattling and B. Stadler, Nanomedicine, 2015, 10, 2725–2742 CrossRef PubMed
.
- X. Y. Zhong, K. Yang, Z. L. Dong, X. Yi, Y. Wang, C. C. Ge, Y. L. Zhao and Z. Liu, Adv. Funct. Mater., 2015, 25, 7327–7336 CrossRef
.
- L. Han, K. Z. Liu, M. H. Wang, K. F. Wang, L. M. Fang, H. T. Chen, J. Zhou and X. Lu, Adv. Funct. Mater., 2018, 28, 1704195 CrossRef
.
- C. Jiang, Y. Wang, J. Wang, W. Song and L. Lu, Biomaterials, 2017, 114, 54–61 CrossRef PubMed
.
- T. Koike, S. Ohara, Y. Inomata, Y. Abe, K. Iijima and T. Shimosegawa, Inflammopharmacology, 2007, 15, 61–64 CrossRef PubMed
.
- Y. Mori, T. Ohtsuka, H. Kono, Y. Nagayoshi, N. Ideno, T. Aso, S. Kozono, K. Ohuchida, S. Takahata, M. Nakamura, K. Mizumoto and M. Tanaka, Pancreas, 2013, 42, 187–192 CrossRef PubMed
.
- M. Tada, M. Omata, S. Kawai, H. Saisho, M. Ohto, R. K. Saiki and J. J. Sninsky, Cancer Res., 1993, 53, 2472–2474 Search PubMed
.
- M. Ueki, M. Ueda, S. Okamura and T. Yamada, J. Med., 1995, 26, 17–30 Search PubMed
.
- I. G. Macara and L. McCaffrey, Philos. Trans. R. Soc., B, 2013, 368, 20130012 CrossRef PubMed
.
- A. D. Garg, S. Martin, J. Golab and P. Agostinis, Cell Death Differ., 2014, 21, 26–38 CrossRef PubMed
.
- D. W. Greening, S. K. Gopal, R. Xu, R. J. Simpson and W. Chen, Semin. Cell Dev. Biol., 2015, 40, 72–81 CrossRef PubMed
.
- L. Milane, A. Singh, G. Mattheolabakis, M. Suresh and M. M. Amiji, J. Controlled Release, 2015, 219, 278–294 CrossRef PubMed
.
- B. Costa-Silva, N. M. Aiello, A. J. Ocean, S. Singh, H. Zhang, B. K. Thakur, A. Becker, A. Hoshino, M. T. Mark, H. Molina, J. Xiang, T. Zhang, T. M. Theilen, G. Garcia-Santos, C. Williams, Y. Ararso, Y. Huang, G. Rodrigues, T. L. Shen, K. J. Labori, I. M. Lothe, E. H. Kure, J. Hernandez, A. Doussot, S. H. Ebbesen, P. M. Grandgenett, M. A. Hollingsworth, M. Jain, K. Mallya, S. K. Batra, W. R. Jarnagin, R. E. Schwartz, I. Matei, H. Peinado, B. Z. Stanger, J. Bromberg and D. Lyden, Nat. Cell Biol., 2015, 17, 816–826 CrossRef PubMed
.
- N. Funamizu, C. Hu, C. Lacy, A. Schetter, G. Zhang, P. He, J. Gaedcke, M. B. Ghadimi, T. Ried, H. G. Yfantis, D. H. Lee, J. Subleski, T. Chan, J. M. Weiss, T. C. Back, K. Yanaga, N. Hanna, H. R. Alexander, A. Maitra and S. P. Hussain, Int. J. Cancer, 2013, 132, 785–794 CrossRef PubMed
.
- J. Bernhagen, R. Krohn, H. Lue, J. L. Gregory, A. Zernecke, R. R. Koenen, M. Dewor, I. Georgiev, A. Schober, L. Leng, T. Kooistra, G. Fingerle-Rowson, P. Ghezzi, R. Kleemann, S. R. McColl, R. Bucala, M. J. Hickey and C. Weber, Nat. Med., 2007, 13, 587–596 CrossRef PubMed
.
- D. Heinrichs, M. Knauel, C. Offermanns, M. L. Berres, A. Nellen, L. Leng, P. Schmitz, R. Bucala, C. Trautwein, C. Weber, J. Bernhagen and H. E. Wasmuth, Proc.
Natl. Acad. Sci. U. S. A., 2011, 108, 17444–17449 CrossRef PubMed
.
- N. Funamizu, C. X. Hu, C. Lacy, A. Schetter, G. Zhang, P. J. He, J. Gaedcke, M. B. Ghadimi, T. Ried, H. G. Yfantis, D. H. Lee, J. Subleski, T. Chan, J. M. Weiss, T. C. Back, K. Yanaga, N. Hanna, H. R. Alexander, A. Maitra and S. P. Hussain, Int. J. Cancer, 2013, 132, 785–794 CrossRef PubMed
.
- L. Tirinato, F. Gentile, D. Di Mascolo, M. L. Coluccio, G. Das, C. Liberale, S. A. Pullano, G. Perozziello, M. Francardi, A. Accardo, F. De Angelis, P. Candeloro and E. Di Fabrizio, Microelectron. Eng., 2012, 97, 337–340 CrossRef
.
- Q. Li, G. K. Tofaris and J. J. Davis, Anal. Chem., 2017, 89, 3184–3190 CrossRef PubMed
.
- R. Xu, A. Fitts, X. T. Li, J. Fernandes, R. Pochampally, J. H. Mao and Y. M. Liu, Anal. Chem., 2016, 88, 10390–10394 CrossRef PubMed
.
- J. F. Li, X. D. Tian, S. B. Li, J. R. Anema, Z. L. Yang, Y. Ding, Y. F. Wu, Y. M. Zeng, Q. Z. Chen, B. Ren, Z. L. Wang and Z. Q. Tian, Nat. Protoc., 2013, 8, 52–65 CrossRef PubMed
.
- L. Ming, J. W. Kang, S. Sukumar, R. R. Dasari and I. Barman, Chem. Sci., 2015, 6, 3906–3914 RSC
.
- J. Li, Y. X. Chen, X. Guo, L. Zhou, Z. M. Jia, Z. Peng, Y. P. Tang, W. D. Liu, B. Zhu, L. Wang and C. P. Ren, J. Cell Mol. Med., 2017, 21, 838–847 CrossRef PubMed
.
- H. Y. Zhang, T. Deng, R. Liu, M. Bai, L. K. Zhou, X. Wang, S. Li, X. Y. Wang, H. Yang, J. L. Li, T. Ning, D. Z. Huang, H. L. Li, L. Zhang, G. G. Ying and Y. Ba, Nat. Commun., 2017, 8, 15016 CrossRef PubMed
.
- M. F. Kircher, A. de la Zerda, J. V. Jokerst, C. L. Zavaleta, P. J. Kempen, E. Mittra, K. Pitter, R. M. Huang, C. Campos, F. Habte, R. Sinclair, C. W. Brennan, I. K. Mellinghoff, E. C. Holland and S. S. Gambhir, Nat. Med., 2012, 18, 829–834 CrossRef PubMed
.
- J. X. Cheng and X. S. Xie, Science, 2015, 350, aaa8870 CrossRef PubMed
.
- B. A. Kairdolf, X. M. Qian and S. M. Nie, Anal. Chem., 2017, 89, 1015–1031 CrossRef PubMed
.
- Y. Chen, L. Ding, W. Song, M. Yang and H. Ju, Chem. Sci., 2016, 7, 569–574 RSC
.
- Y. Zou, S. Huang, Y. Liao, X. Zhu, Y. Chen, L. Chen, F. Liu, X. Hu, H. Tu and L. Zhang, Chem. Sci., 2018, 9, 2842–2849 RSC
.
- G. Frens, Nat.
Phys. Sci., 1973, 241, 20–22 Search PubMed
.
Footnotes |
† Electronic supplementary information (ESI) available. See DOI: 10.1039/c8sc01611a |
‡ These authors contributed equally to this work. |
|
This journal is © The Royal Society of Chemistry 2018 |
Click here to see how this site uses Cookies. View our privacy policy here.