DOI:
10.1039/C7SC03236F
(Edge Article)
Chem. Sci., 2018,
9, 2340-2347
Cholesterol enhances influenza binding avidity by controlling nanoscale receptor clustering†
Received
25th July 2017
, Accepted 23rd January 2018
First published on 24th January 2018
Abstract
Influenza virus infects cells by binding to sialylated glycans on the cell surface. While the chemical structure of these glycans determines hemagglutinin–glycan binding affinity, bimolecular affinities are weak, so binding is avidity-dominated and driven by multivalent interactions. Here, we show that membrane spatial organization can control viral binding. Using single-virus fluorescence microscopy, we demonstrate that the sterol composition of the target membrane enhances viral binding avidity in a dose-dependent manner. Binding shows a cooperative dependence on concentration of receptors for influenza virus, as would be expected for a multivalent interaction. Surprisingly, the ability of sterols to promote viral binding is independent of their ability to support liquid–liquid phase separation in model systems. We develop a molecular explanation for this observation via molecular dynamics simulations, where we find that cholesterol promotes small-scale clusters of glycosphingolipid receptors. We propose a model whereby cholesterol orders the monomeric state of glycosphingolipid receptors, reducing the entropic penalty of receptor association and thus favoring multimeric complexes without phase separation. This model explains how cholesterol and other sterols control the spatial organization of membrane receptors for influenza and increase viral binding avidity. A natural consequence of this finding is that local cholesterol concentration in the plasma membrane of cells may alter the binding avidity of influenza virions. Furthermore, our results demonstrate a form of cholesterol-dependent membrane organization that does not involve lipid rafts, suggesting that cholesterol's effect on cell membrane heterogeneity is likely the interplay of several different factors.
Introduction
Influenza virus enters and infects host cells by binding to sialylated glycoproteins and glycolipids located on the surface of epithelial cells. Specifically, viral hemagglutinin binds terminal sialic acids on cell-surface glycans.1,2 The remainder of the glycan chemical structure, in particular the linkage between sialic acid and the penultimate galactose, modulates the overall virus-receptor binding affinity and thus receptor specificity. Because glycan chemical structures vary between human and bird upper respiratory tracts, viruses tend to bind glycans of their “host” species with greater affinity, and changes to influenza glycan receptor specificity are believed critical for human-to-human transmission of avian influenza viruses.3–7
In addition to these determinants of monomeric affinity, it is possible that lateral organization of the host membrane plays an important role in controlling overall binding avidity. Single glycan–hemagglutinin affinities are relatively weak, with dissociation constants in the millimolar range in solution.8,9 Both viral and cellular surfaces have many copies of hemagglutinin and glycan receptors, respectively, with approximately 300 hemagglutinin trimers on the viral surface.10 This suggests that binding is avidity driven and thus lateral organization of target receptors could be an important determinant of viral binding and infectivity.
To test the influence of target receptor nanoscale organization, we measured influenza binding avidity to planar lipid bilayers containing glycosphingolipid GD1a model receptors. Synthetic lipid bilayers provide a platform for defined manipulations of membrane composition and glycan chemical structure that are challenging to achieve in cellular membranes due to complex composition and cellular homeostatic mechanisms. Although influenza binds several different glycoproteins and glycolipids, GD1a has been the model receptor of choice in studying the interactions between virus and synthetic membranes.11–15 We hypothesized that membrane composition changes that affect the lateral organization of GD1a receptors (or indeed any other glycan receptors for influenza) would alter influenza binding avidity.
Cell plasma membranes display heterogeneous spatial distribution of lipids and proteins.16–18 Cholesterol has been extensively studied as one factor that may induce this spatial organization, particularly cholesterol–lipid interactions that are correlated with liquid–liquid phase separation in model systems.19–23 Although model systems involving cholesterol, phospholipids, and sphingolipids do undergo such phase separation at higher sphingolipid mole fractions than used here,24–26 it remains unclear whether phase separation would be an organizing principle for glycosphingolipids at physiological concentrations of <5 mol%. Glycosphingolipids have been described to self-associate in membranes in the absence of cholesterol,27,28 and they may also co-associate with cholesterol.29 While it is thus reasonable to hypothesize that cholesterol may modulate glycosphingolipid–glycosphingolipid association, neither the effect of cholesterol on nanoscale assemblies of glycosphingolipids nor the resulting effects on influenza binding and infection are yet well understood.
To test how membrane spatial organization could impact viral binding, we varied sterol mol% and chemical composition in synthetic bilayers containing low mol% GD1a, hypothesizing that sterol composition would affect GD1a lateral organization. We first measured influenza binding avidity to these bilayers using single-virus fluorescence microscopy and showed that the presence of cholesterol enhances binding and that binding occurs in a cooperative fashion. We then employed molecular dynamics simulations to develop a model for how cholesterol alters membrane ordering to increase GD1a multimers. We conclude that cholesterol promotes the formation of GD1a-rich regions. These regions with a high local concentration of GD1a thus efficiently bind and retain virus, so an increase in the number of such regions explains the higher binding avidity of influenza to membranes with higher sterol.
Experimental methods
Fluorescence labeling of influenza virus.
Influenza A virus (strain X-31, A/Aichi/68, H3N2) was purchased from Charles River Laboratories (Wilmington, MA). For viral binding measurements, virus was labeled with the lipophilic dye TR-DHPE by incubation in a solution of dye-containing buffer (20 mM HEPES, 150 mM NaCl, pH 7.2, 17 μg ml−1 TR-DHPE) at room temperature for 2 hours. Dye incorporation rate into viral membranes is unknown but we estimate final concentrations of less than 3 mol% because viruses were not labeled at self-quenched concentrations and TR-DHPE self-quenching occurs at 3–5 mol% in model membranes.30 Following labeling, virus was pelleted by centrifugation and then re-suspended in dye-free HEPES buffer. Amount of labeled virus recovered was estimated by measuring viral protein concentration in the final sample as previously described.31 Labeled virus was left to sit overnight at 4 °C and subsequently used in viral binding experiments or stored at −20 °C.
Lipid vesicle preparation.
Lipid mixtures (140 nmol total lipid) containing 20 mol% DOPE, 0–40 mol% sterol, 0–5 mol% GD1a, 0.5 mol% Oregon Green-DHPE and remaining mol% POPC were prepared in chloroform and dried under house vacuum for 3 hours to overnight. Dried lipid films were re-suspended in phosphate buffered saline (10 mM NaH2PO4, 90 mM sodium citrate, 150 mM NaCl, pH 7.4), and large unilamellar vesicles with a nominal diameter of 100 nm were prepared by extrusion. Unless indicated otherwise, GD1a concentration was fixed at 1% and sterol concentration was fixed at 10%. Vesicle mixtures were stored at 4 °C and used within one week of extrusion.
Single virus binding assay.
Viral binding to supported lipid bilayers inside a microfluidic device was quantified by fluorescence microscopy and subsequent spot detection of bound Texas-Red-labeled virions. The full procedure of viral binding experiments is as schematized in ESI Fig. 1:† 2-chamber microfluidic devices were assembled by bonding etched polydimethylsiloxane to glass coverslips, similar to those described previously;31 the overall assay setup is also similar to what has been described previously for single-virus binding studies.32 Supported planar lipid bilayers were then formed inside the microfluidic device by vesicle fusion. GD1a concentrations are reported as the average mol% across all lipids, although prior experiments have shown that similar glycosphingolipids are preferentially oriented away from the solid support, so the mol% available for virus binding may be up to 2× the global average.33 Flow-cells were then mounted on the microscope stage and centered 5 mm from the inlet hole. Bilayers were quality-tested prior to viral binding for homogeneity using visualization by Oregon Green and fluidity using a fluorescence recovery after photobleaching (FRAP) assay (see ESI†). A HEPES buffer solution containing ∼10–100 pM of fluorescently labeled virus particles was flowed through the microfluidic device for 2.5 minutes. Viral binding was monitored by fluorescence image acquisition every 3–5 seconds and increased linearly during this time (ESI Fig. 2†). At the end of the flow period, the solution inside the microfluidic device was immediately exchanged for virus free vesicle buffer to remove excess and unbound virions. Stably bound virions were imaged by fluorescence microscopy at nine separate locations spaced 0.5 mm apart around the center of the microfluidic device.
Membrane GD1a concentrations and viral concentrations were optimized in pilot experiments to ensure stable binding and moderate density of bound virus. In final epifluorescence images, individual viral particles were fully distinguishable and a total of 100–400 particles were bound per field of view. Supported bilayers with a minimum of 0.25 mol% GD1a showed linear binding with time and binding was not saturated by the end of the constant flow time period (ESI Fig. 2†). At a concentration of 1 mol% GD1a virions diffused slowly once bound, similar to previous reports,11 and remained attached to the membrane over time. Complete dissociation events were rare (ESI Fig. 2†). Membranes with 1 mol% GD1a were selected to measure binding avidity changes with sterol variant and sterol concentration. Membranes containing either 10 mol% cholesterol or cholestenone demonstrated linear binding capacities over a 5-fold range of virus concentration (ESI Fig. 2†). Final experiments utilized virus concentrations within the lower region of this linear range.
FRAP measurements.
To measure membrane fluidity, supported lipid bilayers containing 0.5 mol% Oregon Green DHPE were subjected to FRAP analysis. A localized circular region of the membrane with radius 10.2 μm was photobleached using maximum light intensity for 20 s. Recovery images of the entire FOV were then taken every 3 second for a minimum of 4 minutes. Average image intensity within the photobleached region over the recovery period was normalized to pre-bleach intensity and then fitted to the FRAP equations developed by Kang et al. that correct for fluorophore diffusion during bleaching to determine the diffusion coefficient.34
Microscopy and epifluorescence micrograph analysis.
Binding of Texas-Red labeled virons was monitored using a Nikon Ti-U microscope with a 100 oil immersion objective, NA 1/4 1.49 (Nikon Instruments, Melville, NY) and Spectra-X LED Light Engine (Lumencor, Beaverton, OR) as an excitation light source. Epifluorescence micrographs of stably bound virions were captured via an Andor iXon 897 EMCCD camera (Andor Technologies, Belfast, UK) using 16-bit image settings. The number of bound virions per field of view was quantified using the spot detection plugin of Icy.35 The particle detection threshold was manually set. For data pooled from multiple days of experiments, the average number of bound virions per biological replicate was normalized to a single standardized set of conditions to correct for day-to-day variation. Analyses of significance used Student's t-test unless otherwise stated.
Simulation analysis.
Self-association behavior of GD1a molecules was measured in simulations via analysis of contact lifetimes, calculated as follows. GD1a–GD1a contacts were measured using a distance cutoff of 8 Å between the backbone AM1/AM2 beads or any of the headgroup beads of two molecules. This contact cut-off distance corresponds approximately to the first solvation shell. Distances between beads of adjacent GD1a molecules were calculated at 0.01 μs intervals after discarding an equilibration interval of 2.5 μs for each simulation. To avoid hard-cutoff artifacts, contact counts were smoothed using a 5-point moving average. Pairwise contact lifetimes were used to calculate dissociation rate constants using a previously developed Bayesian formulation based on a Poisson event model43 as described below. Cholesterol effects on dissociation rates were robust to changes in cutoff distance (6–11 Å) and beads used to define a contact.
GD1a lipid tail order was calculated according to the formula
, where θ is the angle between the bilayer normal and the bond vector of two consecutive tail beads.
Results and discussion
Sterol composition alters membrane avidity for influenza virus
We used a single influenza virus-binding assay performed in a microfluidic device to measure the effect of target membrane composition on influenza virus binding (ESI Fig. 1†). Texas-Red-labeled virions were allowed to bind to planar bilayers containing GD1a receptors, and membrane-binding affinity was quantified via spot detection of stably bound virions by fluorescence microscopy. For all experiments reported below, viral binding was linear with exposure time and viral concentration (ESI Fig. 2†).
To test whether sterols can affect viral binding by altering the local environment of GD1a receptors in the target membrane, we measured single virion binding to membranes containing 10 mol% of each of seven different sterols (Fig. 1). The concentration of GD1a receptors was fixed at 1 mol% for these experiments. Influenza binding was sensitive to the presence of sterol but largely independent of sterol chemical identity. Membranes containing 10 mol% cholesterol showed significantly higher binding avidity than sterol-free membranes (p < 0.005). Essentially no binding was observed in the absence of GD1a receptors either in the presence or the absence of cholesterol (ESI Fig. 5†). The cholesterol-dependence of binding was striking because even at cholesterol concentrations much lower than those typically found in the plasma membrane, binding was enhanced by 30%. Cholestenone, an oxidized version of cholesterol (ESI Fig. 6†), further increased influenza virus binding by 30% compared to cholesterol-containing membranes (p < 0.0005). Other sterol variants tested, including lanosterol, 7-DHC, desmosterol, stigmasterol and ergosterol, did not statistically differ from cholesterol at 10 mol%. Binding enhancement was consistent across at least eight biological replicates in each case. These results demonstrate the surprising conclusion that even low mol fractions of sterols can greatly alter influenza binding avidity.
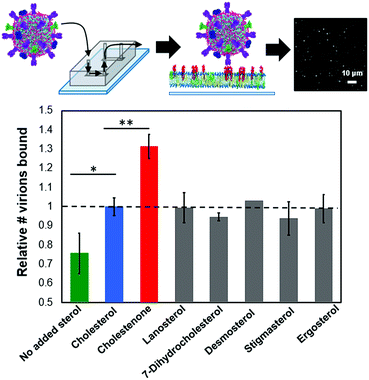 |
| Fig. 1 Sterols enhance influenza binding avidity to target membranes. Relative influenza binding avidity to planar bilayers containing 10 mol% of the indicated sterol was measured using a single-virus binding assay (ESI Fig. 1†); GD1A is shown as entirely in the upper leaflet in supported bilayers as suggested for GM1.33 Cholesterol-containing membranes bound significantly more influenza particles than membranes lacking sterol (p < 0.005). Of the six cholesterol analogues tested, only cholestenone increased binding significantly compared to cholesterol (p < 0.0005). In addition to sterol, target bilayers contained 1 mol% GD1a, 20 mol% DOPE, 0.5 mol% OG-DHPE and remaining mol% POPC. | |
Membrane binding avidity is sterol-dose-dependent and viral binding is cooperative with target receptor concentration
To gain mechanistic insight into how sterols alter viral binding avidity, we measured the dependence of influenza binding with respect to either sterol or GD1a concentration in the target membrane. Both cholesterol and cholestenone showed similar monotonic increases in binding with mol fraction sterol (Fig. 2A). Over intermediate sterol concentrations (5–20 mol%), both sterols behaved similarly with linear increases in binding with respect to mol% cholesterol and nearly identical slope. At high concentrations approximating the mol% cholesterol in the plasma membrane, influenza binding was nearly twice that of cholesterol-free bilayers. Combined with the cooperativity analysis discussed below, the most parsimonious explanation for these data is that cholesterol and cholestenone have a common mechanism in promoting viral binding, with an additional enhancement of monomeric binding affinity by cholestenone.
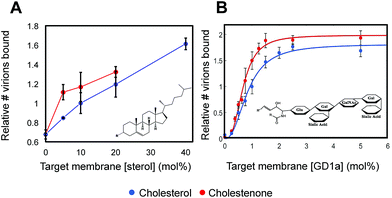 |
| Fig. 2 Viral binding is dose-dependent on sterol concentration and cooperative in receptor concentration. (A) Dose-dependence of viral binding as a function of mol% sterol. Both cholesterol and cholestenone enhanced membrane binding avidity in a dose-dependent manner but cholestenone demonstrated a more marked effect at low concentrations. Above 10 mol%, both sterols showed a roughly linear dose dependence. Membranes containing 40 mol% cholestenone did not form homogenous supported bilayers and could not be measured. All values are normalized to 10 mol% cholesterol. (B) Cooperativity analyzed by fitting binding versus GD1a receptor concentration using the Hill equation. Hill coefficients of 2.36 ± 0.85 and 2.83 ± 0.61 for cholesterol and cholestenone respectively were not significantly different (p = 0.15). | |
Viral binding avidity showed a sigmoidal relationship with receptor concentration as would be expected for a multivalent binding interaction between viral hemagglutinin and sialic acids presented on GD1a (Fig. 2B). Cooperativity was measured by fitting binding curves to the Hill equation, yielding fit equations of
and
. The Hill coefficients extracted from this fit were thus 2.36 ± 0.85 and 2.83 ± 0.61 for cholesterol and cholestenone, respectively, and differences were not statistically significant (p = 0.15 via comparison of bootstrap-resampled cumulative distribution functions). However, both maximum binding and half-maximum concentration coefficients were significantly different between sterols with p-values of 0.04 and <0.002, respectively. Together with the cholestenone-concentration dependence of binding, this suggests that cholestenone may increase monomeric hemagglutinin–GD1a affinity, but that this effect saturates at a low sterol mol fraction and does not change cooperativity. Viral dissociation events were only observed at GD1a mol fraction less than 0.25%, again consistent with a multivalent binding interaction. The cooperativity we observe makes viral binding highly sensitive to local receptor concentration and the number of available GD1a within a virus-bilayer encounter complex. Because binding showed the same cooperativity with respect to mol% GD1a in bilayers with cholestenone and with cholesterol, we concluded that this effect is unrelated to liquid–liquid phase coexistence because cholestenone does not support such coexistence.44–46 We therefore hypothesized that cholesterol promotes GD1a receptor nanoscale clustering in some other manner. This clustering in turn increases the number of GD1a present at a virus-bilayer contact and would explain cholesterol's ability to increase viral binding avidity. Glycosphingolipid clustering and membrane environment has also been shown to alter multimeric binding of GM1 by cholera toxin, although rigorous analyses of cooperativity have not been performed and the effect is complicated by an additional cholesterol-dependent effect on receptor conformation.47–49
GD1a molecules self-associate in molecular dynamics simulations of lipid bilayers
To develop a molecular model for the potential cholesterol-mediated nanoscale clustering of GD1a, we performed coarse-grained molecular dynamics simulations of GD1a self-association in lipid membranes. In our simulated bilayers, the phospholipid and cholesterol composition were identical to those used in binding experiments (ESI Table 1†). For GD1a, a range of concentrations was tested spanning the global mean concentration used experimentally (1 mol% GD1a), the maximum concentration available for viral binding if GD1a were preferentially on the top leaflet (2 mol%),33 and any locally increased concentration within the simulated 15 × 15 nm patch that could result from large-scale compositional fluctuations and generate regions that could bind virus more readily (4–10 mol%). Simulations of cholestenone were not performed because the MARTINI coarse-grained representation, while producing results of acceptable fidelity for cholesterol, does not easily allow encoding of the hydrogen-bonding differences between cholesterol and cholestenone.50
Under all simulation conditions, GD1a molecules formed multimers within 2.5 μs that reversibly dissolved and re-formed over the course of each >18 μs simulation. Representative simulation snapshots depicting GD1a clusters in membranes containing 4 mol% or 10 mol% GD1a and 20 mol% cholesterol are rendered in Fig. 3A and B. In analyzing the simulations, we defined GD1a multimers as two or more GD1a molecules separated by fewer than 8 Å, chosen as the distance to the first major peak in the radial distribution function of simulated lipids. These transient GD1a clusters are highly consistent with previous experimental thermodynamic data on ganglioside self-aggregation in lipid membranes51,52 as well as data on transient GM1 nanoclusters.53,54 In these simulations cholesterol was not more likely to be in contact with GD1a than other phospholipids, indicating a lack of preferential association. Although the coarse-grained model with polarizable solvent employed here may quantitatively overestimate GD1a–GD1a association free energies,39 it should be well powered to detect relative changes in GD1a self-association, as we describe below.
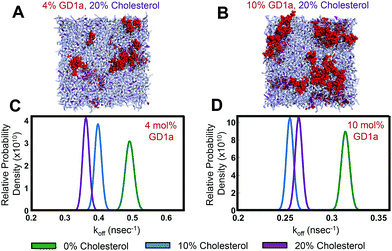 |
| Fig. 3 Cholesterol slows GD1a–GD1a dissociation rates in molecular dynamics simulations. Simulation snapshots for membranes containing 20 mol% cholesterol and (A) 4 mol% GD1a or (B) 10 mol% GD1a. GD1a molecules showed reversible clustering in simulations containing 0, 10 or 20 mol% cholesterol. Cholesterol is rendered in purple, GD1a in red, and phospholipids in gray. (C and D) Probability density functions for GD1a–GD1a dissociation rate constants estimated from simulations. These compare rates over the range 0–20 mol% cholesterol at either 4 mol% or 10 mol% GD1a. Cholesterol significantly reduced koff compared to sterol-free membranes under all of these conditions (p < 10−6). | |
Simulated bilayers containing cholesterol show reduced GD1a multimer dissociation rates
To quantify the effect of cholesterol on GD1a self-association, we estimated the rate of dissociation from clusters of two or more GD1a molecules (Fig. 3C and D). Rather than estimating the association/dissociation energy between isolated glycosphingolipids as previously performed for GM1 and GM3,39 we wished to measure the effect of cholesterol on GD1a nanoclusters within the heterogeneous membrane environment tested experimentally. We therefore estimated spontaneous dissociation rates of multimers in our simulations using a Bayesian formulation based on a Poisson event model that we developed previously.43 This calculation was designed to calculate dissociation rates averaged over the population of multimer sizes spontaneously formed in the mixed membranes to which we measured viral binding.
Based on 18 μs of simulation per condition, GD1a dissociation rates were significantly lowered by 10 or 20 mol% cholesterol in membranes containing 4 mol% and 10 mol% GD1a (Fig. 3C and D). The number of dissociation events in simulations of 2 mol% GD1a were too low to be powered for statistical significance but also demonstrated increased mean contact lifetime (ESI Fig. 4†) and decreased dissociation rate constant with cholesterol. Multimer association rates were not measured due to the relative paucity of diffusional encounters between GD1a monomers and long lifetimes of the resulting multimers. The asymmetry between statistical power in estimating association and dissociation events is explained as follows: in a dilute two-dimensional fluid of GD1a, the number of statistically independent time windows when a dissociation event could occur (where each window is defined as the time expected for a monomeric lipid to diffuse the characteristic lipid–lipid separation distance) is much greater than the number of statistically independent encounters between monomers. We therefore performed fluorescence recovery after photobleaching experiments to estimate diffusion rates in phospholipid
:
cholesterol
:
GD1a mixtures (ESI Fig. 7†). Estimated diffusion rates did not vary significantly between 0 and 20 mol% cholesterol and thus do not suggest a difference in encounter complex formation rates. We argue that measuring off-rates in simulations is therefore most appropriate to examine the mechanism of cholesterol-promoted viral binding since, in kinetically controlled processes, off-rates are considered usually more influential in determining overall binding stability.55 Thus, these data suggest that cholesterol decreases GD1a–GD1a off rates, driving enhanced GD1a clustering and multimer stability and helping to explain increased viral binding avidity.
Cholesterol reduces the entropic penalty of multimer formation to favor GD1a multimers
We propose the following model for cholesterol enhancement of viral binding avidity (Fig. 4A): GD1a multimer formation is enthalpically favorable due to glycan–glycan interactions52 but entropically disfavored due to steric confinement of lipid tails within multimers. Cholesterol lowers this entropic penalty of association by ordering lipid tails of a monomeric ganglioside and allows the enthalpy of multimerization to dominate the free energy of association. Under the assumption that association enthalpy remains largely unchanged by inclusion of cholesterol in the membrane, a decrease in association free energy (ΔΔGassociation < 0) shifts the monomer–multimer equilibrium in favor of GD1a clustering. Cholesterol thus leads to nanoscale clustering of the target receptor in a lipid bilayer and increases the number of available GD1a within the virus-membrane encounter complex, enhancing the membrane binding avidity of influenza.
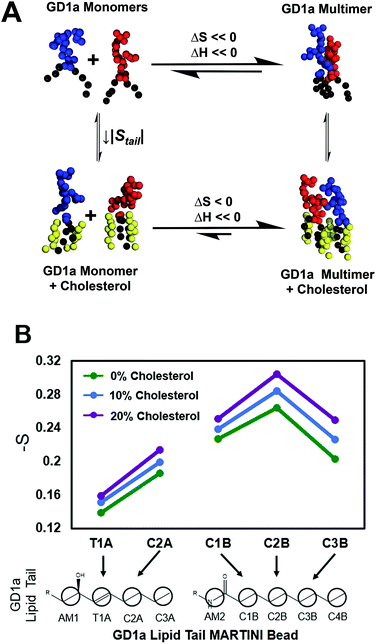 |
| Fig. 4 Thermodynamic model for cholesterol-mediated increase in GD1a nanoclusters based on simulations. (A) Thermodynamic model for GD1a multimerization and association with cholesterol. Multimerization has a favorable enthalpy but unfavorable entropy. We postulate that cholesterol has a differential ordering effect on GD1a in the monomeric reference state as compared to the multimer. This decreases the entropic penalty of multimerization, increasing the overall fraction of multimers at equilibrium and thus viral binding avidity. Snapshots are rendered of monomers and multimers in simulated membranes containing 4 mol% GD1a. The monomer–dimer equilibrium is shown for simplicity, but the same model applies to more complex multimers. (B) Orientational order parameters for GD1a lipid tails in simulated membranes containing 1 mol% GD1a. Increasing concentrations of cholesterol progressively order GD1a tails, suggestive of an overall decrease in entropy. | |
Since a key feature of this model is ordering of monomeric GD1a tails, we measured cholesterol-induced changes in GD1a tail order in our simulations (Fig. 4B). Membranes with 1 mol% GD1a were chosen for this measurement to maximally approximate the GD1a monomeric state. Order parameters were calculated by analogy to SCD; while not truly measuring carbon–deuterium order parameters in coarse-grained simulations, this method nonetheless measures lipid orientation with regard to the membrane normal and serves as a partial readout of lipid entropy. For all five coarse grained beads in the GD1a lipid tails, calculated order parameters increased monotonically with mol% cholesterol (Fig. 4B). Simulation results therefore support the hypothesis that cholesterol orders the monomeric state of GD1a, reducing the entropic penalty of association and thus enhancing clustering.
Conclusions
Our results show that influenza viral binding avidity for membranes is highly sensitive to sterol composition in the membrane, even at constant receptor density. We suggest that cholesterol drives the formation of GD1a multimers in the target membrane by lowering the entropic penalty of GD1a association and thus increasing the local concentration of other GD1a molecules near an initial virus-GD1a binding event. Due to the multivalent nature of viral binding, these GD1a nanoclusters will enhance retention of bound virus. It is of course also possible that hemagglutinin–GD1a binding events serve to further retain GD1a at the site of viral binding and increase GD1a cluster size beyond that in the absence of virus. Although many examples of cholesterol-driven membrane nanoclusters are linked to cholesterol's ability to support liquid–liquid phase coexistence, our data suggest this is not the case here. First, mol fractions of cholesterol required to increase viral binding are much lower than those needed for phase coexistence in similar mixtures. Second, cholestenone does not support phase separation in model systems44 but demonstrates similar receptor cooperativity and binding enhancement behavior to cholesterol. Our analyses show that, while cholestenone slightly increases monomeric receptor affinity for virus compared to cholesterol, both cholesterol and cholestenone have a similar ability to enhance viral binding, which we propose is due to promotion of GD1a nanoclusters. Thus, sterols promote viral binding in a fashion that is completely logical from a chemical perspective but not typically considered in the physiology of viral binding, which focuses on mainly glycan structure. From a physiological perspective, the surprising sensitivity of influenza viral binding to sterol concentration may have implications in disease susceptibility due to differences in membrane composition and suggests the potential for new research into epidemiologic factors and host-directed therapies for influenza.
Conflicts of interest
There are no conflicts of interest to declare.
Acknowledgements
C. Broshkevitch designed the GD1a topology and helped prototype simulations. The authors thank F. Moss and E. Webster for many helpful discussions. This work was funded by National Institutes of Health grants R01 GM098304 to P. M. K. and R35 GM118044 to S. G. B. as well as a Wallenberg Academy Fellowship to P. M. K. and a Stanford Bio-X fellowship to I. N. G. Simulations were performed using the Stanford XStream cluster, NSF Blue Waters, and the PDC Center for High Performance Computing supported by the Swedish National Infrastructure for Computing.
Notes and references
- J. J. Skehel and D. C. Wiley, Annu. Rev. Biochem., 2000, 69, 531–569 CrossRef CAS PubMed.
- J. C. Paulson, J. E. Sadler and R. L. Hill, J. Biol. Chem., 1979, 254, 2120–2124 CAS.
- K. Shinya, M. Ebina, S. Yamada, M. Ono, N. Kasai and Y. Kawaoka, Nature, 2006, 440, 435–436 CrossRef CAS PubMed.
- D. van Riel, V. J. Munster, E. de Wit, G. F. Rimmelzwaan, R. A. Fouchier, A. D. Osterhaus and T. Kuiken, Science, 2006, 312, 399 CrossRef CAS PubMed.
- M. Imai, T. Watanabe, M. Hatta, S. C. Das, M. Ozawa, K. Shinya, G. Zhong, A. Hanson, H. Katsura, S. Watanabe, C. Li, E. Kawakami, S. Yamada, M. Kiso, Y. Suzuki, E. A. Maher, G. Neumann and Y. Kawaoka, Nature, 2012, 486, 420–428 CAS.
- S. Herfst, E. J. Schrauwen, M. Linster, S. Chutinimitkul, E. de Wit, V. J. Munster, E. M. Sorrell, T. M. Bestebroer, D. F. Burke, D. J. Smith, G. F. Rimmelzwaan, A. D. Osterhaus and R. A. Fouchier, Science, 2012, 336, 1534–1541 CrossRef CAS PubMed.
- C. A. Russell, P. M. Kasson, R. O. Donis, S. Riley, J. Dunbar, A. Rambaut, J. Asher, S. Burke, C. T. Davis, R. J. Garten, S. Gnanakaran, S. I. Hay, S. Herfst, N. S. Lewis, J. O. Lloyd-Smith, C. A. Macken, S. Maurer-Stroh, E. Neuhaus, C. R. Parrish, K. M. Pepin, S. S. Shepard, D. L. Smith, D. L. Suarez, S. C. Trock, M. A. Widdowson, D. B. George, M. Lipsitch and J. D. Bloom, eLife, 2014, 3, e03883 Search PubMed.
- N. K. Sauter, J. E. Hanson, G. D. Glick, J. H. Brown, R. L. Crowther, S. J. Park, J. J. Skehel and D. C. Wiley, Biochemistry, 1992, 31, 9609–9621 CrossRef CAS PubMed.
- N. K. Sauter, M. D. Bednarski, B. A. Wurzburg, J. E. Hanson, G. M. Whitesides, J. J. Skehel and D. C. Wiley, Biochemistry, 1989, 28, 8388–8396 CrossRef CAS PubMed.
- E. C. Hutchinson, P. D. Charles, S. S. Hester, B. Thomas, D. Trudgian, M. Martinez-Alonso and E. Fodor, Nat. Commun., 2014, 5, 4816 CrossRef CAS PubMed.
- T. Ivanovic, J. L. Choi, S. P. Whelan, A. M. van Oijen and S. C. Harrison, eLife, 2013, 2, e00333 Search PubMed.
- D. Floyd, J. R. Ragains, J. J. Skehel, S. C. Harrison and A. M. van Oijen, Proc. Natl. Acad. Sci. U. S. A., 2008, 105, 15382–15387 CrossRef CAS PubMed.
- L. Wessels, M. W. Elting, D. Scimeca and K. Weninger, Biophys. J., 2007, 93, 526–538 CrossRef CAS PubMed.
- A. M. Haywood and B. P. Boyer, Proc. Natl. Acad. Sci. U. S. A., 1985, 82, 4611–4615 CrossRef CAS.
- D. Alford, H. Ellens and J. Bentz, Biochemistry, 1994, 33, 1977–1987 CrossRef CAS PubMed.
- B. F. Lillemeier, J. R. Pfeiffer, Z. Surviladze, B. S. Wilson and M. M. Davis, Proc. Natl. Acad. Sci. U. S. A., 2006, 103, 18992–18997 CrossRef CAS PubMed.
- P. Schwille, J. Korlach and W. W. Webb, Cytometry, 1999, 36, 176–182 CrossRef CAS PubMed.
- D. Lingwood and K. Simons, Science, 2010, 327, 46–50 CrossRef CAS PubMed.
- M. Edidin, Annu. Rev. Biophys. Biomol. Struct., 2003, 32, 257–283 CrossRef CAS PubMed.
- S. L. Veatch and S. L. Keller, Biophys. J., 2003, 85, 3074–3083 CrossRef CAS PubMed.
- I. Levental, F. J. Byfield, P. Chowdhury, F. Gai, T. Baumgart and P. A. Janmey, Biochem. J., 2009, 424, 163–167 CrossRef CAS PubMed.
- T. Baumgart, A. T. Hammond, P. Sengupta, S. T. Hess, D. A. Holowka, B. A. Baird and W. W. Webb, Proc. Natl. Acad. Sci. U. S. A., 2007, 104, 3165–3170 CrossRef CAS PubMed.
- D. Lingwood, H. J. Kaiser, I. Levental and K. Simons, Biochem. Soc. Trans., 2009, 37, 955–960 CrossRef CAS PubMed.
- R. S. Petruzielo, F. A. Heberle, P. Drazba, J. Katsaras and G. W. Feigenson, Biochim. Biophys. Acta, 2013, 1828, 1302–1313 CrossRef CAS PubMed.
- R. F. de Almeida, A. Fedorov and M. Prieto, Biophys. J., 2003, 85, 2406–2416 CrossRef CAS PubMed.
- S. L. Veatch and S. L. Keller, Phys. Rev. Lett., 2005, 94, 148101 CrossRef PubMed.
- E. Bertoli, M. Masserini, S. Sonnino, R. Ghidoni, B. Cestaro and G. Tettamanti, Biochim. Biophys. Acta, 1981, 647, 196–202 CrossRef CAS.
- T. W. Tillack, M. Wong, M. Allietta and T. E. Thompson, Biochim. Biophys. Acta, 1982, 691, 261–273 CrossRef CAS.
- M. M. Lozano, J. S. Hovis, F. R. Moss III and S. G. Boxer, J. Am. Chem. Soc., 2016, 138, 9996–10001 CrossRef CAS PubMed.
- G. Pahler, C. Panse, U. Diederichsen and A. Janshoff, Biophys. J., 2012, 103, 2295–2303 CrossRef PubMed.
- R. J. Rawle, S. G. Boxer and P. M. Kasson, Biophys. J., 2016, 111, 123–131 CrossRef CAS PubMed.
- D. W. Lee, H. L. Hsu, K. B. Bacon and S. Daniel, PLoS One, 2016, 11, e0163437 Search PubMed.
- I. Carton, L. Malinina and R. P. Richter, Biophys. J., 2010, 99, 2947–2956 CrossRef CAS PubMed.
- M. Kang, C. A. Day, A. K. Kenworthy and E. DiBenedetto, Traffic, 2012, 13, 1589–1600 CrossRef CAS PubMed.
- J.-C. Olivo-Marin, Pattern Recogn., 2002, 35, 1989–1996 CrossRef.
- X. Periole and S. J. Marrink, Methods Mol. Biol., 2013, 924, 533–565 CAS.
- M. N. Melo, H. I. Ingolfsson and S. J. Marrink, J. Chem. Phys., 2015, 143, 243152 CAS.
- C. A. Lopez, Z. Sovova, F. J. van Eerden, A. H. de Vries and S. J. Marrink, J. Chem. Theory Comput., 2013, 9, 1694–1708 CrossRef CAS PubMed.
- R. X. Gu, H. I. Ingolfsson, A. H. de Vries, S. J. Marrink and D. P. Tieleman, J. Phys. Chem. B, 2017, 121(15), 3262–3275 CrossRef CAS PubMed.
- S. Pronk, S. Pall, R. Schulz, P. Larsson, P. Bjelkmar, R. Apostolov, M. R. Shirts, J. C. Smith, P. M. Kasson, D. van der Spoel, B. Hess and E. Lindahl, Bioinformatics, 2013, 29, 845–854 CrossRef CAS PubMed.
- M. Parrinello and A. Rahman, J. Chem. Phys., 1982, 76, 2662–2666 CrossRef CAS.
- G. Bussi, D. Donadio and M. Parrinello, J. Chem. Phys., 2007, 126, 014101 CrossRef PubMed.
- P. M. Kasson, D. L. Ensign and V. S. Pande, J. Am. Chem. Soc., 2009, 131, 1338–1340 CrossRef PubMed.
- M. E. Beattie, S. L. Veatch, B. L. Stottrup and S. L. Keller, Biophys. J., 2005, 89, 1760–1768 CrossRef CAS PubMed.
- B. L. Stottrup and S. L. Keller, Biophys. J., 2006, 90, 3176–3183 CrossRef CAS PubMed.
- X. Xu and E. London, Biochemistry, 2000, 39, 843–849 CrossRef CAS PubMed.
- D. Lingwood, B. Binnington, T. Rog, I. Vattulainen, M. Grzybek, U. Coskun, C. A. Lingwood and K. Simons, Nat. Chem. Biol., 2011, 7, 260–262 CrossRef CAS PubMed.
- D. A. Bricarello, E. J. Mills, J. Petrlova, J. C. Voss and A. N. Parikh, J. Lipid Res., 2010, 51, 2731–2738 CrossRef CAS PubMed.
- S. Rissanen, M. Grzybek, A. Orlowski, T. Rog, O. Cramariuc, I. Levental, C. Eggeling, E. Sezgin and I. Vattulainen, Front. Physiol., 2017, 8, 252 CrossRef PubMed.
- J. D. Perlmutter and J. N. Sachs, J. Am. Chem. Soc., 2009, 131, 16362–16363 CrossRef CAS PubMed.
- S. L. Frey, E. Y. Chi, C. Arratia, J. Majewski, K. Kjaer and K. Y. Lee, Biophys. J., 2008, 94, 3047–3064 CrossRef CAS PubMed.
- S. L. Frey and K. Y. Lee, Biophys. J., 2013, 105, 1421–1431 CrossRef CAS PubMed.
- M. Amaro, R. Sachl, G. Aydogan, I. I. Mikhalyov, R. Vacha and M. Hof, Angew. Chem., Int. Ed., 2016, 55, 9411–9415 CrossRef CAS PubMed.
- R. Sachl, M. Amaro, G. Aydogan, A. Koukalova, I. I. Mikhalyov, I. A. Boldyrev, J. Humpolickova and M. Hof, Biochim. Biophys. Acta, 2015, 1853, 850–857 CrossRef CAS PubMed.
- J. J. Hopfield, Proc. Natl. Acad. Sci. U. S. A., 1974, 71, 4135–4139 CrossRef CAS.
Footnote |
† Electronic supplementary information (ESI) available: ESI methods and figures. See DOI: 10.1039/c7sc03236f |
|
This journal is © The Royal Society of Chemistry 2018 |
Click here to see how this site uses Cookies. View our privacy policy here.