Progressions in reasoning about structure–property relationships
Received
28th September 2017
, Accepted 11th October 2017
First published on 13th October 2017
Abstract
In this essay, findings from research in science and chemistry education are used to describe and discuss progression in students' structure–property reasoning through schooling. This work provides insights into the challenges that students face to master this important component of chemical thinking. The analysis reveals that student reasoning is often guided by nonnormative implicit schemas that are little affected by traditional instruction. These schemas prioritize chemical composition over molecular structure, and centralized causality over emergence in the explanation and prediction of the properties of substances. The types of components that students invoke to make sense of properties and phenomena may change with schooling, but the underlying reasoning persists. In general, learners assume that observed properties and behaviors are directly related to the types of atoms present in a system and determined by these individual atoms' inherent characteristics.
Introduction
Educational practice and research in different scientific disciplines are often guided by topical views of the subject matter. Traditional physics courses are organized around topics such as mechanics and electromagnetism, while biology courses have units on reproduction and genetics. In chemistry, our view of the discipline is segmented at several levels (Talanquer and Pollard, 2010; Sevian and Talanquer, 2014). We draw sharp boundaries between organic chemistry, inorganic chemistry, and physical chemistry, and we subdivide the content in each of these areas into multiple subtopics (e.g., chemical bonding, carbonyl chemistry). This segmentation is also characteristic of science education research, where studies often focus on the learning and teaching of specific topics.
Topical characterizations of school disciplines create challenges for students, teachers, and researchers as these ways of organizing and representing content tend to conceal connections between concepts, ideas, and ways of reasoning within and across different fields of study (Talanquer, 2013a). In an effort to break these boundaries and make conceptual relationships more explicit, science education standards in the US have moved away from topical representations of school disciplines, using constructs such as big ideas, disciplinary practices, and crosscutting concepts to outline and organize what is to be learned (NRC, 2012, 2013). “Structure and Property” is an example of these types of overarching constructs, and it certainly encapsulates one of the most characteristic and productive ways of reasoning in all areas of chemistry (Hoffmann, 1995; Goodwin, 2003, 2008; NRC, 2003).
Structure–property reasoning in chemistry relies on the fundamental assumption that the physical and chemical properties of substances in our world are determined by the composition and structure of submicroscopic components. This assumption is at the center of most chemical models of matter, guiding diverse chemistry practices, from explanation and prediction, to chemical analysis, synthesis, and control. Surprisingly, despite its centrality in chemical thinking, there has been little explicit discussion about how to best help students develop structure–property reasoning, and existing educational research provides mostly indirect clues about the challenges that students face to master this way of thinking.
In this paper, I use existing findings in the research literature in science and chemistry education to describe and discuss what we know about student progressions in reasoning about structure–property relationships. I relied on findings about students' understanding of chemical concepts and ideas published in major journals in the field, or summarized in review and perspective papers and books (Nakhleh, 1992; Taber, 2002; Kind, 2004; Barke et al., 2009; Gilbert and Treagust, 2009; Tsaparlis and Sevian, 2013). The central goals of this contribution are to provide insights into the types of understandings that students need to develop to advance in their structure–property reasoning, and to make more explicit the cognitive difficulties that they are likely to encounter in their learning. Many of the claims made in this essay are conjectures based on both existing research and the theoretical framework that guides my interpretation of available research findings. This work is motivated by the conviction that school chemistry at all educational levels should focus more strongly on the development of crosscutting disciplinary ways of thinking that can be productively applied in diverse contexts.
Connection to learning progressions
The last decade in science education research has seen a surge of interest in the development and testing of learning progressions of central ideas in various disciplines (NRC, 2007; Corcoran et al., 2009; Duschl et al., 2011). A learning progression is a proposal about how students' understanding of a targeted idea can evolve with appropriate instruction. The learning progression is a conjectural model of how to best orchestrate the meaningful assimilation of a central idea based on current understandings of how people learn in the domain (Duncan and Rivet, 2013; Duncan and Gotwals, 2015). Such a learning progression is often represented as a set of levels corresponding to different stages in students' knowledge, starting with initial ideas (lower anchor) and ending with the desired scientific conceptualization (upper anchor). Intermediate stages are carefully defined to represent from less to more sophisticated ways of thinking that enable the desired progression. A learning progression is also expected to define the learning experiences that can best lead to the desired outcomes at different stages, as well as the learning performances that can be used to assess student progress (Duschl et al., 2011).
There are actually few published works that fully characterize a learning progression of a central idea as described in the previous paragraph (Rogat et al., 2011). Some studies present descriptions of conjectured stages in a progression, without definition of the learning experiences and performances that can bring about the proposed progression (Duncan et al., 2009; Stevens et al., 2010; Cooper et al., 2012a). Other researchers have focused on developing the cognitive bases on which learning progressions can be built, by investigating how students' ideas actually evolve under current instruction in different domains (Talanquer, 2009; Hadenfeldt et al., 2016). These types of studies identify and describe the different conceptualizations and ways of thinking that students commonly express as they engage with a given content at different moments in their schooling. The present paper falls into this latter category. This clarification is important because, over the years, the term “learning progression” has become somewhat polysemous in the research literature. This essay does not present a conjectured curricular or instructional model of how to develop structure–property reasoning. Rather, its goal is to organize and highlight what we have learned about student thinking in this area, with the intent of providing a more explicit and cohesive cognitive foundation on which productive chemistry curriculum, instruction, and assessment could be developed.
Theoretical framework
Analyses of progression in student understanding are constrained by the nature of the mental constructs that one assumes are subject to progress and are thought to enable learning. For example, one may consider that people have rather stable alternative conceptions or mental models in a given area and that progress is only possible when those erroneous cognitive constructs are replaced by normative understandings. In this case, analysis of progression may focus on characterizing the alternative conceptions or mental models that students most commonly express at different educational stages. This particular view of conceptual progression has been questioned by diverse authors (Vosniadou, 2008; Brown, 2014). The analysis presented in this essay was carried out under a different perspective, which was informed by existing research in the fields of conceptual change and human reasoning.
Findings from psychological research in multiple areas suggest that the human mind relies on a wide variety of knowledge constructs and reasoning strategies when engaged in any given task (diSessa, 1993; Gigerenzer et al., 1999; Redish, 2004; Brown and Hammer, 2008; Chi, 2008; Taber, 2008; Vosniadou et al., 2008; Kahneman, 2011; Brown, 2014). These diverse cognitive resources support and guide, but also constrain our thinking. Some of these resources are implicit and operate without conscious awareness (Taber, 2014), while others are explicit and can be commanded and verbalized. Some of them seem to be organized in rather cohesive knowledge structures and reasoning schemas, while others may be loosely connected or adapted to perform specific tasks. Formal and informal learning experiences affect these cognitive resources and their deployment in different manners (diSessa and Sherin, 1998; Chi, 2008; Vosniadou et al., 2008). Learning may expand the number of resources available or increase the easiness with which some of them are deployed. Learning may create new connections between resources or reinforce some existing connections and weaken others. Learning may change the conditions under which some resources are triggered, helping automatize or control their use in particular contexts.
A large proportion of our cognitive resources, both knowledge constructs and reasoning strategies, seem to be implicit (Dienes and Perner, 1999). Implicit knowledge includes representations of the properties of different types of entities, events, processes, and states without attributing them to any particular system. This implicit knowledge creates tacit assumptions or expectations that support explanation and prediction of the properties and behaviors of the systems and situations we encounter. For example, we implicitly know that solid objects are cohesive, persist over time and space, and fall down when unsupported (Spelke and Kinzler, 2007). Consequently, when watching a ball rolling off a table, we automatically predict its falling to the ground if no one stops it. We possess implicit knowledge about the shared characteristics of members of each of many kinds of entities, events, processes, and states (e.g., liquid entities, pulling events, mixing processes, resting states). Humans also seem to have implicit causal schemas that guide the identification of plausible causes for given effects (Grotzer, 2003; Keil, 2006). These schemas tend to be general cognitive constructs that are applied to build explanations in different domains (Talanquer, 2006; diSessa, 2014; Coley et al., 2017). We often, for example, explain changes by looking for an agent acting on or within a system in linear and direct ways (linear causality), explain behaviors in terms of the wills or desires of certain agents (teleology), or explain observable properties in terms of hidden components or essences with inherent characteristics (essentialism). These causal schemas allow us to generate tentative hypotheses of why things happen.
Implicit cognitive resources also include shortcut reasoning strategies (heuristics) for judgment and decision making (Gigerenzer et al., 1999; Kahneman, 2011). These heuristics simplify reasoning by reducing the number of factors used in making a decision or by providing rules of thumb for how and where to look for relevant selection cues. For example, the “recognition” heuristic leads us to assign more weight in our decisions to options that we recognize versus those that are unfamiliar. These types of cognitive tools are content independent but task specific. They make efficient use of information readily available and take advantage of regularities in the structure of our surroundings. Implicit heuristics allow us to make adaptive decisions under conditions of limited time, knowledge, and computational power. They can, however, lead us astray by biasing our attention towards particular cues.
The human mind seems to automatically deploy a variety of cognitive resources when confronting a task. Which specific resources are triggered depends on, among other factors, the actual resources available to each individual, the fluency with which these resources are triggered based on prior experiences, the nature of the particular task at hand, the context in which the task is presented, and the individual's personal goals and levels of attention and motivation in the moment (Morewedge and Kahneman, 2010; Evans and Stanovich, 2013). Many of the resources automatically deployed are implicit, but learning and repeated practice may facilitate the quick application of relevant explicit resources. Learning in a given area also strengthens people's ability to monitor and control their automatic responses, and substitute them if deemed necessary.
When we interact with an object or event, available perceptual information and language cues are used by the mind to recognize the entity or phenomenon and to assign it to specific categories via associative thinking and analogical reasoning (Vosniadou and Ortony, 1989; Gelman, 2009). For example, if we hold a rigid, flat, and transparent entity in one hand we may recognize it as a piece of glass, and automatically assign it the attributes of solid objects. In the absence of specific prior knowledge about the system under analysis, our categorizations are likely to be guided by the surface features that are most salient to us. The categorizations that we make have important repercussions on how we think about entities and phenomena because categories guide and constrain our reasoning about what is possible (e.g., glass has sharp edges and thus we may get cut) (Chi, 2008). Categorization decisions depend on prior knowledge and are sensitive to the features that are most noticed in a particular context.
When confronted with questions about an object or event, we search for answers using the cognitive resources at our disposal. For example, if we were asked to explain why the piece of glass in our hand is transparent, we would likely be perplexed. Forced to come up with some explanation, our mind would engage in a search for known systems with similar qualities. What first comes to mind may trigger a particular causal schema. If we associate transparency with water, we may claim that the glass contains such a liquid or has some water-like properties. If we associate transparency with lack of color or with purity, we may propose that the glass does not contain any colored stuff or that it is a pure substance. We dynamically build explanations seeking for an answer that is locally satisficing (Sloman, 1996). This is, the explanation is satisfactory in our minds and suffices to complete the task at hand. We approach explanation in pragmatic ways, looking for simple explanatory factors, deploying knowledge constructs triggered by recognition or similarity judgments, and testing ideas until satisfaction (Sherin et al., 2012; Lombrozo, 2016). The dynamic explanations that we build are not necessarily stable; thus, they may easily change when challenged, additional information is provided, or other alternatives come to mind. But they may become more stable if they are intuitively appealing and successfully applied to make sense of properties or phenomena in different contexts.
From this perspective, many of the nonnormative explanations that students express in classrooms or during research interviews could be better characterized as dynamic alternative “constructions” than as stable alternative conceptions (Brown and Hammer, 2008; Sherin et al., 2012; Brown, 2014). Given the labile nature of many of these constructions, using them as direct measures of student understanding is likely to be less productive than analyzing them to infer the cognitive resources from which they emerge. This type of analysis can provide insights into the underlying knowledge constructs and reasoning strategies that most commonly support or constrain students' thinking as learners advance in their understanding of central ideas in a discipline. This was the approach followed in the elaboration of this work.
Structure–property reasoning
Chemistry educators often refer to “structure–property relationships” as a central understanding that students need to develop without much specification of what such an understanding entails. Analysis of student learning in this area, however, demands the unpacking of this type of reasoning to make explicit its core characteristics.
Chemical thinking relies on a wide variety of models that connect the properties of systems of interest to the structure of a set of components. The term “properties” refers to observable qualities or measurable quantities that provide information about how a system responds to different types of interactions (e.g., mechanical, thermal, chemical) with its surroundings. The term “structure” typically encompasses information about the types of components (composition) that make up the system, their spatial distribution (geometry), and the nature of their interactions (connectivity). The structure of chemical systems is commonly depicted using symbolic–iconic representations containing both explicit and implicit information that needs to be decoded (Hoffmann and Laszlo, 1991; Cooper et al., 2012b).
Different models are built to establish structure–property relationships for systems defined at different scales spanning from the multi-entity macroscopic scale to the electronic scale (Fig. 1) (Jensen, 1998; Talanquer, 2011). In general, these models relate the properties of a system at one scale with the properties and behaviors of components at a subscale. For example, the properties of a water filter (multi-entity macroscopic) may be explained in terms of the properties of pure water, activated carbon, and common solutes (single-entity macroscopic). In turn, the physical properties of liquid water may be attributed to the dynamic behavior of submicroscopic particles (multi-particle submicroscopic), and so on.
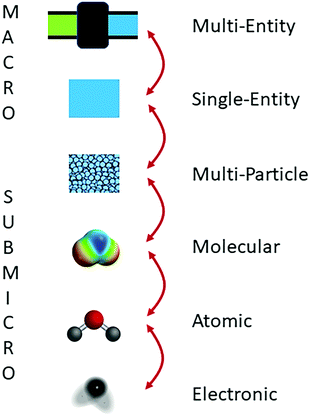 |
| Fig. 1 Different scales at which structure–property relationships are commonly built in chemical thinking. Additional scales of analysis (e.g., meso-scale) may be needed in the modeling of particular systems (e.g., polymeric materials) (Meijer et al., 2009). | |
In chemical thinking, structure–property relationships can be built using associative reasoning or mechanistic reasoning depending on the nature and goals of a task (Fig. 2). Structure–property relationships can be expressed in a quite automatic manner by using direct associations between the properties of a system and the composition and structure of its components. For example, stronger intermolecular forces between particles (multi-particle) are automatically associated with higher boiling points for liquids (single-entity macroscopic); larger differences in electronegativity between atoms in a bond (atomic) are associated with larger bond polarities (molecular); and lower valence electron energy states (electronic) are linked to higher electronegativities (atomic). These quick associations facilitate the making of predictions and simplify the construction of explanations. However, the justification of such structure–property relationships demands the application of mechanistic reasoning in which causal links are built between the structure of components and the properties of the system of interest (Russ et al., 2008). These latter properties are assumed to emerge from the dynamic interactions between components, and thus cannot be inferred by direct transference of properties from one scale to another (Luisi, 2002; Talanquer, 2015; Tümay, 2016). The properties of a system are seen as statistical averages over the many configurations that components can dynamically adopt during the time that an observation or measurement lasts.
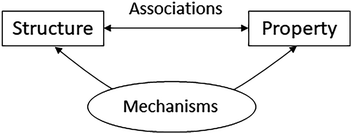 |
| Fig. 2 Structure–property reasoning in chemistry relies on both associative and mechanistic reasonings. | |
The types of mechanisms invoked in the construction and justification of structure–property relationships for different types of properties have distinctive characteristics. Mechanisms used to make sense of a system's physical properties (e.g., boiling point, viscosity, polarity) are often based on the analysis of interactions between components and their effects on their relative positions and motions. We explain, for example, differences in boiling points as resulting from differences in the strength of the intermolecular forces that need to be overcome to separate particles. On the other hand, mechanisms used to make sense of chemical properties (chemical reactivity) often focus on the effect of interactions on the structure and relative energy of the components involved (Goodwin, 2003, 2008). For instance, we explain reaction directionality by making claims about potential energy differences between the molecules of reactants and products.
The above discussion suggests that the development of structure–property reasoning is likely to be challenging as it requires recognizing, decoding, and interpreting multiple explicit and implicit features (composition, geometry, connectivity) in the representations of chemical systems, building conceptual bridges between different scales of analysis, applying appropriate associative and mechanistic reasoning as needed depending on the task, and making use of the different explanatory schemes that are most productive in particular contexts. A description of progression in structure–property reasoning should provide insights into how students confront these challenges in their path to understanding.
From a chemical perspective, all the properties of a system are the manifestation of processes in which some of the system's components are involved. However, some of these processes are imperceptible to our senses, such as the photon–electron interactions assumed to be responsible for the color of a material. Some other processes are directly perceptible, like the dissolution of common salt in water denotative of its solubility, or are made explicit through chemical representations, like molecular resonance which is symbolically depicted using structural formulas. Analysis of existing findings on student thinking suggests that distinctive types of reasoning are triggered when students seek to explain properties that seem to be intrinsic to a system and when they explain properties that involve perceptible or explicit changes. Thus, in the following sections I use the differentiation between “making sense of intrinsic properties” and “making sense of explicit behaviors” to organize the discussion about progressions in structure–property reasoning.
Making sense of intrinsic properties
Results from existing research suggest that student reasoning about the origins or causes of the properties of substances undergoes major changes through schooling. Nevertheless, such reasoning seems often anchored on an implicit schema that is little affected by instruction. In this schema (Fig. 3), the properties of substances are associated with the presence of particular components with inherent characteristics responsible for the observed properties of materials in linear additive manners (i.e., they result from the weighted average of the properties of the components) (Talanquer, 2006, 2008, 2013b; Taber and García-Franco, 2010). The nature of the invoked components changes as students advance in their studies, but the underlying reasoning persists. This is a composition-based reasoning schema in which structural features are commonly ignored and the need for mechanistic explanations is often bypassed.
 |
| Fig. 3 Dominant schema guiding and constraining student structure–property reasoning through schooling. | |
In the following paragraphs, I describe common patterns in student reasoning, from less to more sophisticated, about the properties of substances. The level of sophistication has been assigned based on personal judgements about the extent to which a given form of reasoning would allow individuals to propose generalizable mechanisms to describe, explain, and predict properties and behaviors (i.e., their explanatory power). I have chosen to use the suffix “ism” to label each major pattern of reasoning to suggest that each of them represents an implicit stance in the analysis of structure–property relationships. Any particular stance is not assumed to fully constrain student reasoning, but rather serve as a blueprint that guides the elaboration of dynamic cognitive constructions as students engage in explanation and prediction. As represented in Fig. 4, advanced students can be expected to more frequently express and apply the more sophisticated forms of reasoning. However, the stance that is dominant in a student's explanation might depend on the system under analysis. Students frequently apply more sophisticated reasoning in the analysis of known cases, but revert back to less sophisticated thinking when facing unfamiliar situations. Thus, different stances may be adopted by the same learner under distinct conditions.
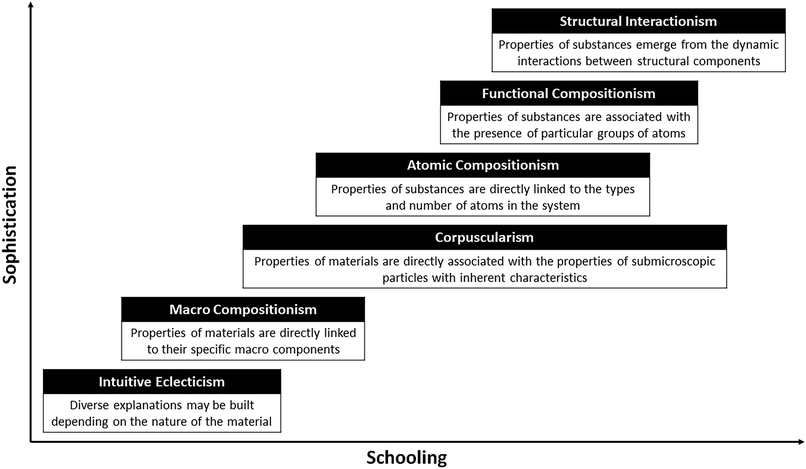 |
| Fig. 4 Dominant stances in the progression of students' thinking about the intrinsic properties of materials. | |
Intuitive eclecticism
Novice students without much formal schooling do not seem to adhere to a single common explanation about the properties of materials. Some substances are assumed to have inherent characteristics (i.e., that is how they naturally are). For example, common salt is salty, sugar is sweet, and water is wet and liquidy (Krnel et al., 2005). The properties of other materials could be attributed to the presence of components that give them such characteristics. So, alcohol may be liquid because it has water in it and copper may be reddish because it contains a substance of that color (Ngai et al., 2014). Novice students often substantialize properties by attributing them to a material or quasi-material component (Sanmartí et al., 1995; Reiner et al., 2000). The imagined component serves as a placeholder for the property without the need for further explanation. Some properties may also be explained as resulting from external actions on the material, so metals could be shiny because they got polished and glass may be transparent because it was cleaned thoroughly (Solominodou and Stavridou, 2000).
Macro compositionism
Education seems to solidify the assumption that the properties of materials are directly linked to their specific macro components (their chemical makeup). As learners develop, they gain experience with and receive information about different types of materials and their characteristics. This knowledge is often reduced to simple associations between a material and specific properties inferred from the material's source, history, behavior, or uses. For example, soap produces bubbles, glue is sticky, and alcohol is flammable (Krnel et al., 2005; Bretz and Emenike, 2012). In school, students also learn about elemental substances such as oxygen which is needed to produce fire, silver and aluminium which are shiny metals, and carbon which is a black solid related to burning. This explicit knowledge is used in combination with an additive compositional schema to make sense and predict properties of materials. For instance, students may claim that silver chloride should be a shiny substance because it has silver in it or that ethanol is flammable because it contains oxygen (Talanquer, 2013b).
Corpuscularism
Students' learning about the particulate (or corpuscular) nature of matter brings about a change in the nature of the components that are invoked to explain and predict properties of materials. Arguments shift from referring to macro components to talking about the particles present in a system and their inherent properties. Particle properties, however, are typically inferred from the macroscopic properties of the materials. For instance, particles of copper are assumed to be reddish and malleable as a macroscopic sample of that metal (Ben-Zvi et al., 1986), and particles of gas are assumed to expand when the temperature is increased (Griffiths and Preston, 1992). From this perspective, materials have certain properties because those are the properties that their particles have. The actual nature of the particles that comprise a given substance is likely rather undefined in a novice's mind. These particles may be simply conceived as small pieces of the substance at hand (Talanquer, 2006, 2009).
The particulate model of matter assigns major roles to the relative positions, motions, and interactions of submicroscopic particles in determining the physical properties of substances. Typically, students use these ideas to explain properties in rather associative ways. The may learn to link, for example, high temperatures and pressures with fast moving particles, or strong particle interactions with high boiling points. However, many learners are unable to build detailed mechanistic explanations of how measurable properties emerge from the particles' random movements and interactions (Talanquer, 2015; Tümay, 2016).
Atomic compositionism
Emphasis on the atomic nature of matter in introductory science courses seems to give pre-eminence in students' minds to atomic composition as the ultimate cause of all observed properties (Taber, 2003). The number and characteristics of the individual atoms present in a system become critical cues in making sense of and predicting a substance's properties, regardless of structural considerations (Talanquer, 2008). Consequently, chemical formulas and not molecular structures are the focus of analysis. Students pay particular attention to object-like attributes of the atomic components, including atomic mass and size, and the number of atoms of each type (Maeyer and Talanquer, 2010, 2013; Broman and Parchmann, 2014). Atomic components are often thought of as macro-objects with inherent properties (e.g., oxygen atoms are flammable or very reactive, iron atoms can rust, hydrogen atoms are acidic). This pattern of reasoning may lead students to think, for example, that substances comprised of heavier atoms will have higher boiling points (because more energy is needed to displace them) or that substances with more hydrogen atoms in their chemical formulas are stronger acids (Cooper et al., 2013; Heisterkamp and Talanquer, 2015). As students advance in their studies, the types of atomic properties under consideration may be expanded to include, for example, electronegativity and types of bonds (Cullipher and Sevian, 2015). Notwithstanding, the focus on the atomic scale rather than on the molecular scale seems to persist in the reasoning of a significant fraction of students even in advance science courses (DeFever et al., 2015).
The implicit reliance on atomic composition to reason about the properties of chemical substances is likely a major contributor to the difficulties that many students have to differentiate between both intra and intermolecular interactions, and the processes these interactions regulate (e.g., chemical reactions versus phase changes). “Atomic compositionism” relies on building direct links between the atomic and the single-entity macroscopic scales, without much consideration for the molecular and multi-particle scales. Students may recognize molecules as aggregates of atoms with particular chemical compositions, but the importance of atomic connectivity and geometry in determining the identify and properties of substances is undervalued (Ngai et al., 2014). Thus, it may not be surprising to encounter students who create new chemical bonds between atoms when representing a substance's transition from liquid to solid or who break chemical bonds when representing the change from liquid to gas (Cooper et al., 2015). Structural changes may have little significance to students who implicitly conceive substances as mixtures of different types of atoms.
The productive use of both the particulate and atomic models of matter to build structure–property relationships demands the identification of the relevant particles in a system (e.g., atoms, molecules, ions) and the characterization of their structure and interactions. Students who hold an atomic compositional view of substances can be expected to struggle with these tasks. One of the major challenges that students face in developing structure–property reasoning is properly connecting the atomic and the corpuscular models of matter. This demands the construction of bridges between the atomic, molecular, and multi-particle scales by learning to use information about atomic composition to infer molecular structure and, from there, deduce potential interactions. However, meaningful learning in this area demands a shift from an implicit compositional schema to a structural one.
Functional compositionism
There is evidence that students in more advanced chemistry courses can shift their attention from single atoms to particular groups of connected atoms when building structure–property relationships (Graulich, 2015; Weinrich and Talanquer, 2015). The presence of these atomic groups is often associated with physical and chemical properties in normative and nonnormative ways. For instance, a student may use the presence of –OH groups in the molecules alcohols as indicative of hydrogen bonding interactions and use it to predict a higher boiling point. Another student may also notice the –OH group and infer that these substances are chemical bases (McClary and Talanquer, 2011). Attention to “functional groups” is an important step towards the recognition of structural factors that affect the properties of chemical compounds. However, many students treat these groups as compositional elements, thinking of them as isolated components and failing to recognize the importance of their structure, and the relevance of their interactions with other structural elements (Graulich and Bhattacharyya, 2017).
Structural interactionism
A large fraction of students who take traditional chemistry courses finish their studies without developing an expert stance towards structure–property reasoning (labeled here as structural interactionism). Progression seems hampered by various issues: (i) analyses constrained to the atomic scale with little thought about the molecular and multi-particle scales; (ii) attention directed at compositional rather than structural features; (iii) application of linear additive versus emergent thinking to infer properties at one scale from information about components at a subscale; (iv) conceptualization of components as having intrinsic rather than extrinsic properties that emerge from interactions with surroundings entities. As described in the following section, a rather static, deterministic, and homogeneous view of the atomic and molecular worlds also limits students' ability to comprehend how constant properties and behaviors can emerge from dynamic, probabilistic, and heterogeneous submicroscopic systems and processes.
Making sense of explicit behaviors
Some properties of substances, like their solubility and chemical reactivity, manifest through behaviors that are perceptible or commonly represented using chemical symbolism. Students' explanations of these behaviors progress through schooling but, as was the case for the explanation of intrinsic properties, reasoning in this area is often guided and constrained by an underlying schema that persists despite instruction. This is a causal schema in which events or processes are seen as involving a causal agent with certain dispositions or intentions. This agent undergoes changes to meet some goal or acts on another entity that may passively respond or could offer resistance to the changes being imposed. Effects are assumed to correlate in linear ways with perceived properties of the entities involved, such as their size, strength, and proximity in time and space. Larger molecules, for example, may be seen as having more casual agency or as more resistant to change. Typically, a single causal agent and a single causal force are invoked (Chi et al., 2012; Talanquer, 2006). This way of thinking has been analyzed and discussed by a variety of authors interested in different aspects of human reasoning; thus, it has been described in different manners, including force-dynamics reasoning (Talmy, 1988), experiential gestalt of causation (Andersson, 1986), and centralized mindset (Resnick, 1996). I use the term “centralized causalism” in the following sections to refer to this implicit stance in students' explanations of the properties of material systems based on the characteristics of their components.
Major implicit stances identified in the analyses of students' reasoning about the behaviors of substances are summarized in Fig. 5 arranged from less to more sophisticated. These stances overlap as students advance in their schooling. Some of them coexist as they may be selectively applied to explain different types of behaviors. In general, learning affects the nature of the components assumed to have causal agency, but has little effect on the causal schema that is commonly deployed.
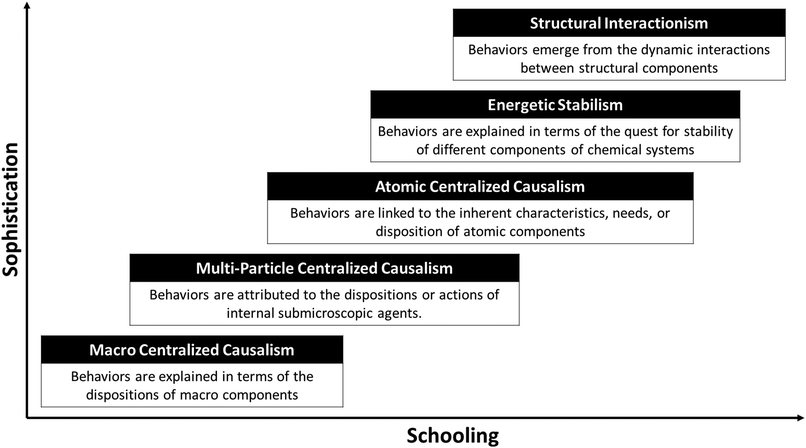 |
| Fig. 5 Dominant stances in the progression of students' thinking about the explicit behaviors of materials. | |
Macro centralized causalism
In the absence of basic chemical knowledge, explanations for the behavior of substances are likely to depend on the specific nature of the process under analysis and on the conditions in which it takes place. If the behavior occurs spontaneously, without the action of an easily recognizable causal agent, explanations are likely to be teleological in nature referring to a natural disposition, need, or desire that must be satisfied (Talanquer, 2006). For instance, a hot substance may be thought to cool down because it seeks to be in equilibrium with its environment (diSessa, 2014). If a causal agent can be identified, then a substance's behavior can be linked to the agent's actions, with effects determined by some measure of the agent's strength and of the substance's disposition to change. For example, a student could think that salt dissolves in water because this liquid melts the solid, while a stone does not dissolve in water because rocks are harder and more difficult to melt (Hatzinikita et al., 2005). From this perspective, observed properties are determined by the natural dispositions of some macro components acting either independently or in conjunction with an external agent.
Multi-particle centralized causalism
Students' recognition of the particulate nature of matter causes a shift in the nature of the components invoked to justify physical behaviors. Observed properties become now attributed to the dispositions or actions of internal submicroscopic agents. For instance, osmotic behavior may be explained by claiming that particles of the solvent move across a membrane seeking to homogeneize concentrations in both sides of an osmosis chamber (teleological argument) (Talanquer, 2010). On the other hand, the solubility of salt in water may now be linked to the action of water particles colliding with, attracting, and separating salt particles. Under this stance, one can expect an increase in the number of arguments based on mechanical interactions and generic attractions and repulsions between particles. However, students are likely to attribute more causal agency to particles that are larger, heavier, more charged, or moving faster (Yan and Talanquer, 2015).
Atomic centralized causalism
Discussions about the atomic nature of matter in science courses often lead students to think that the properties and dispositions of individual atoms determine a substance's chemical properties. Diverse research studies have shown that students frequently assert that the needs or wants of atoms are responsible for these particles' behaviors (Taber, 1998; Taber and Watts, 2000; Talanquer, 2013c). These types of claims are made at different scales of analysis. For instance, atoms are assumed to gain, lose, and share electrons seeking to get full valence electron shells (electronic scale). Atoms are thought to arrange in particular positions in molecules to minimize repulsions (molecular scale). Some atoms are also conceived as more active than others, based on properties such as electronegativity and size. Consequently, judgments about the chemical reactivity of substances are likely to focus on the known characteristics and dispositions of component atoms, disregarding structural information. An explanation based on this logic would state, for example, that oxygen gas is highly reactive because it is made up of highly electronegative atoms that seek to get two electrons to fill their valence shell.
Energetic stabilism
Expert explanations of a substance's chemical properties are commonly based on a comparative analysis of molecular structural features in different states that are then used to build inferences about relative energetic stabilities. Through schooling, students are exposed to these types of explanations but they often reduce them to teleological arguments in which the quest for stability is assumed to drive a substance's behavior (Taber, 2009). These types of explanations are quite appealing to students from a wide range of chemistry backgrounds. For example, when given a choice, a majority of college chemistry students prefer teleological explanations of proton exchange (acid–base behavior) and electron exchange (redox behavior) over explanations based on relative probabilities of available states (Talanquer, 2013c). Many students find the claim that substances undergo particular changes in order to become more stable a satisfactory and sufficient explanation, and do not recognize the need for mechanistic accounts.
Through their schooling, students build different associations between compositional and structural features of submicroscopic entities and their chemical stability. For example, charged atoms may be seen as more stable than neutral atoms because the latter are assumed to not have a full valence shell, while charged molecules may be judged to be less stable than neutral ones because the former can more readily interact with other species. The presence of multiple bonds between atoms may be taken as a sign of molecular stability, and the recognition of resonance in molecules or ions may trigger ideas about extra stabilization. Over time, advanced chemistry students acquire a large collection of associative rules (e.g., tertiary carbocations are most stable, polar bonds are stronger than nonpolar bonds) that support the development of inferences about the relative energetic stability of different submicroscopic entities or chemical states (Weinrich and Talanquer, 2015). Student understanding of the mechanisms responsible for stability differences, however, is often quite limited (Bhattacharyya, 2014). Consequently, explanations of behavior are commonly constrained to descriptions of noticed differences between compared species or states (e.g., bromide ions are larger than chloride ions), followed by restatements of related associative rules (e.g., larger anions are more stable than smaller ones).
Many students end their chemistry training thinking of chemical stability (or reactivity) as an absolute property of a chemical species that depends on the composition and structure of rather static and isolated subcomponents. Consequently, analyses of a species' behaviors often ignore the effects of interactions among subcomponents and with surrounding entities (e.g., molecules of the same or different reactants, molecules of solvent). These analyses also tend to disregard the role that configurational effects at the molecular and multiparticle levels (i.e., entropic effects) may have on the stability of a system or state (Weinrich and Talanquer, 2015; Yan and Talanquer, 2015). Additionally, learners often fail to recognize the existence of variability in the distribution and configurations of the particles that make up a substance, and in the events in which these particles participate at any given time. Figuratively speaking, it is as if students' focus was on the physical traits, needs, moods, and tribulations of a single entity assumed to be alone or taken as representative of a homogeneous population.
Structural interactionism
Major characteristics of expert structure–property reasoning about the behaviors of substances have been described in previous sections. Results from existing research suggest that a minority of the students who finish their required chemistry courses at the college level satisfactorily masters this type of thinking.
Conclusion and implications
The analysis presented in this paper suggests that progression towards expert structure–property reasoning happens slowly and gradually as students accumulate knowledge and assimilate different models of matter. Student thinking is often guided by nonnormative implicit schemas that are little affected by traditional instruction. These schemas prioritize chemical composition over molecular structure, and centralized causality over emergence in the explanation and prediction of the properties of substances. The types of components that students invoke to make sense of properties and phenomena may change with schooling, but the underlying reasoning persists. In general, learners assume that observed properties and behaviors are directly related to the types of atoms present in a system and determined by these atoms' inherent dispositions and tendencies. A large number of the alternative conceptions and conceptual difficulties reported in this area seem to stem from the different reasoning stances described in this paper.
When students engage in structure–property reasoning they tend to do it in associative manners, stating relationships but failing to properly justify them using mechanistic arguments. Few learners, even those in advanced courses, seem to develop the ability to build explanations that mechanistically connect the electronic, atomic, molecular, multi-particle, and macroscopic scales. The construction of these types of multi-layered explanations is complex as it demands the integration of vast amounts of knowledge, the clear differentiation between multiple concepts defined at diverse scales, and the ability to recognize and properly evaluate the effects of the various variables affecting the behavior of the targeted chemical system.
Based on existing findings, progression in structure–property reasoning does not seem to proceed in well-defined stages. Through schooling, students' chemical knowledge increases and there are major changes in the types of components most frequently invoked to explain properties and behaviors. Advanced students are likely to generate more sophisticated explanations with higher frequency than novice learners. However, the types of explanations constructed by students are sensitive to diverse factors, such as familiarity with the system or properties under analysis, and recognition of relevant cues during a task. For example, a student may generate a mechanistic explanation based on generic attractions between particles to explain the insolubility of a salt, and then use teleological arguments to justify the decomposition of a chemical compound (Yan and Talanquer, 2015). Students often switch between the stances summarized in Fig. 4 and 5 depending on the nature of the task.
Given the complexities of structure–property reasoning, it is possible that slow and gradual progress in this area is inevitable. Nevertheless, analyses of student learning in traditional versus alternative chemistry curricula suggest that purposeful interventions can have a significant positive impact in the development of this type of reasoning. Curricular projects which actively engage students in the development, application, and evaluation of models have shown to promote learning and accelerate the development of structure–property reasoning (Copper et al., 2017). Similarly, educational projects that focus on helping students to develop crosscutting disciplinary practices and ways of thinking (Sevian and Talanquer, 2014) have positive effects on students' abilities to build mechanistic explanations of diverse phenomena (Talanquer and Pollard, 2010, 2017).
Analyses of the types of instructional tasks used in alternative curricula that foster structure–property reasoning indicate that students benefit from consistent and continued work on activities that ask them to analyze data, identify patterns, build and apply models to make sense of properties and behaviors, represent their ideas in multiple forms (e.g., sketches, graphs, diagrams), engage in argument with their peers, and generate and communicate explanations. These curricula also seem to approach the analysis of chemical systems from the molecular and multi-particle perspectives, building bridges to the atomic scale when necessary. The findings summarized in this essay suggest that atoms-first approaches to the teaching of chemistry may actually reinforce students' biases towards atom-centered explanations of the properties and behaviors of substances. The study of chemical models for their own sake, without an anchor in real systems or phenomena to be explained, it is likely to hinder rather than foster the development of structure–property reasoning as students will not be challenged to connect the real and modeled worlds.
Conflicts of interest
There are no conflicts to declare.
References
- Andersson B., (1986), The experimental gestalt of causation: a common core to pupils' preconceptions in science, Eur. J. Sci. Educ., 8(2), 155–171.
- Barke H. D., Hazri A. and Yitbarek S., (2009), Misconceptions in chemistry: addressing perceptions in chemical education, Berlin: Springer Verlag.
- Ben-Zvi R., Eylon B.-S. and Silberstein J., (1986), Is an atom of copper malleable? J. Chem. Educ., 63, 64–66.
- Bhattacharyya G., (2014), Trials and tribulations: student approaches and difficulties with proposing mechanisms using the electron-pushing formalism, Chem. Educ. Res. Pract., 15, 594–609.
- Bretz S. L. and Emenike M. E., (2012), Hannah's prior knowledge about chemicals: a case study of one Fourth Grade child, Sch. Sci. Math., 112(2), 99–108.
- Broman K. and Parchmann I., (2014), Students' application of chemical concepts when solving chemistry problems in different contexts, Chem. Educ. Res. Pract., 15, 516–529.
- Brown D. E., (2014), Students' conceptions as dynamically emergent structures, Sci. Educ., 23, 1463–1483.
- Brown D. E. and Hammer D., (2008), Conceptual change in physics, in Vosniadou S. (ed.), International handbook of research on conceptual change, New York: Routledge, pp. 127–154.
- Chi M. T. H., (2008), Three kinds of conceptual change: belief revision, mental model transformation, and ontological shift, in Vosniadou S. (ed.), International handbook of research on conceptual change, New York: Routledge, pp. 61–82.
- Chi M. T., Roscoe R. D., Slotta J. D., Roy M. and Chase C. C., (2012), Misconceived causal explanations for emergent processes, Cognit. Sci., 36, 1–61.
- Coley J. D., Arenson M., Xu Y. and Tanner K. D., (2017), Intuitive biological thought: developmental changes and effects of biology education in late adolescence, Cognit. Psychol., 92, 1–21.
- Cooper M. M., Underwood S. M., Hilley C. Z. and Klymkowsky M. W., (2012a), Development and assessment of a molecular structure and properties learning progression, J. Chem. Educ., 89, 1351–1357.
- Cooper M. M., Underwood S. M. and Hilley C. Z., (2012b), Development and validation of the Implicit Information from Lewis Structures Instrument (IILSI): Do students connect structures with properties? Chem. Educ. Res. Pract., 13(1), 195–200.
- Cooper M. M., Corley L. M. and Underwood S. M., (2013), An investigation of college chemistry students’ understanding of structure–property relationships, J. Res. Sci. Teach., 50(6), 699–721.
- Cooper M. M., Williams L. C. and Underwood S. M., (2015), Student understanding of intermolecular forces: a multimodal study, J. Chem. Educ., 92(8), 1288–1298.
- Cooper M. M., Stieff M. and De Sutter D., (2017), Sketching the invisible to predict the visible: from drawing to modeling in chemistry, Top. Cognit. Sci., DOI: http://10.1111/tops.12285.
- Corcoran T., Mosher F. A. and Rogat A., (2009), Learning progressions in science: an evidence-based approach to reform, Consortium for Policy Research in Education Report #RR-63, Philadelphia, PA: Consortium for Policy Research in Education.
- Cullipher S. and Sevian H., (2015), Atoms versus bonds: how students look at spectra, J. Chem. Educ., 92, 1996–2005.
- DeFever R. S., Bruce H. and Bhattacharyya G., (2015), Mental rolodexing: senior chemistry majors’ understanding of chemical and physical properties, J. Chem. Educ., 92, 415–426.
- Dienes Z. and Perner J., (1999), A theory of implicit and explicit knowledge, Behav. Brain Sci., 22, 735–808.
- diSessa A. A., (1993), Toward an epistemology of physics, Cognit. Instruct., 10, 165–255.
- diSessa A. A., (2014), The construction of causal schemes: learning mechanisms at the knowledge level, Cognit. Sci., 38, 795–850.
- diSessa A. A., and Sherin B. L., (1998). What changes in conceptual change? Int. J. Sci. Educ., 20(10), 1155–1191.
- Duncan R. G. and Gotwals A. W., (2015), A tale of two progressions: on the benefits of careful comparisons, Sci. Educ., 99, 410–416.
- Duncan R. G. and Rivet A. E., (2013), Science learning progressions, Science, 339(6118), 396–297.
- Duncan R. G., Rogat A. and Yarden A., (2009), A learning progression for deepening students' understandings of modern genetics across the 5th–10th grades, J. Res. Sci. Teach., 46, 655–674.
- Duschl R., Maeng S. and Sezen A., (2011), Learning progressions and teaching sequences: a review and analysis, Stud. Sci. Educ., 47(2), 123–182.
- Evans J. S. and Stanovich K. E., (2013), Dual-process theories of higher cognition: advancing the debate, Perspect. Psychol. Sci., 8(3), 223–241.
- Gelman S. A. (2009). Learning from others: Children's construction of concepts, Ann. Rev. Psychol., 60, 115–140.
- Gigerenzer G., Todd P.M. and the ABC Research Group, (1999), Simple heuristics that make us smart, New York: Oxford University Press.
- Gilbert J. K. and Treagust D. F., (2009), Multiple representations in chemical education, Dordrecht: Springer.
- Goodwin W. M., (2003), Explanations in organic chemistry, Ann. N. Y. Acad. Sci., 988, 141–153.
- Goodwin W. M., (2008), Structural formulas and explanation in organic chemistry, Found. Chem., 10, 117–127.
- Graulich N., (2015), Intuitive judgments govern students’ answering patterns in multiple-choice exercises in organic chemistry, J. Chem. Educ., 92, 205–211.
- Graulich N, and Bhattacharyya G., (2017), Investigating students’ similarity judgments in organic chemistry, Chem. Educ. Res. Pract., 18, 774–784.
- Griffiths A. K. and Preston K. R., (1992), Grade-12 students' misconceptions relating to fundamental characteristics of atoms and molecules, J. Res. Sci. Teach., 29, 611–628.
- Grotzer T. A., (2003), Learning to understand the forms of causality implicit in scientifically accepted explanations, Stud. Sci. Educ., 39, 1–74.
- Hadenfeldt J. C., Neumann K., Bernholt S., Liu X. and Parchmann I., (2016), Students’ progression in understanding the matter concept, J. Res. Sci. Teach., 53, 683–708.
- Hatzinikita V., Koulaidis V. and Hatzinikitas A., (2005), Modeling pupils’ understanding and explanations concerning changes in matter, Res. Sci. Educ., 35, 471–495.
- Heisterkamp K. and Talanquer V., (2015), Interpreting data: the hybrid mind, J. Chem. Educ., 92, 1988–1995.
- Hoffmann R., (1995), The Same and Not the Same, Columbia New York: University Press.
- Hoffmann R. and Laszlo P., (1991), Representation in chemistry, Angew. Chem., Int. Ed., 20, 1–16.
- Jensen W., (1998), Logic, history, and the chemistry textbook I. Does chemistry have a logical structure? J. Chem. Educ., 75, 679–687.
- Kahneman D., (2011), Thinking, fast and slow, New York: Farrar, Straus and Giroux.
- Keil F., (2006), Explanation and understanding, Annu. Rev. Psychol., 57, 227–254.
- Kind V., (2004), Beyond appearances: students’ misconceptions about basic chemical ideas, 2nd edn, London: Royal Society of Chemistry.
- Krnel D., Watson R. and Glažar S. A., (2005), The development of the concept of ‘matter’: a cross-age study of how children describe materials, Int. J. Sci. Educ., 27, 367–383.
- Lombrozo T., (2016), Explanatory preferences shape learning and inference, Trends Cognit. Sci., 20, 748–759.
- Luisi P. L., (2002), Emergence in chemistry: chemistry as the embodiment of emergence, Found. Chem., 4, 183–200.
- Maeyer J. and Talanquer V., (2010), The role of heuristics in students thinking: ranking of chemical substances, Sci. Educ., 94(6), 963–984.
- Maeyer J. and Talanquer V., (2013), Making predictions about chemical reactivity: assumptions and heuristics, J. Res. Sci. Teach., 50(6), 748–767.
- McClary L. and Talanquer V., (2011), Heuristic reasoning in chemistry: Making decisions about acid strength, Int. J. Sci. Educ., 33, 1433–1454.
- Meijer M., Bulte A.M. W. and Pilot A., (2009), Structure–property relations between macro and micro representations: relevant meso-level in authentic tasks, in Gilbert J. K. and Treagust D. (ed.), Multiple representations in chemical education, Dordrecht: Springer, pp. 195–213.
- Morewedge C. K. and Kahneman D., (2010), Associative processes in intuitive judgment, Trends Cognit. Sci., 14(10), 435–440.
- Nakhleh M. B., (1992), Why some students don't learn chemistry, J. Chem. Educ., 69, 191–196.
- National Research Council (NRC), (2003), Beyond the molecular frontier: challenges for chemistry and chemical engineering, Washington, DC: National Academy Press.
- National Research Council (NRC), (2007), Taking science to school: learning and teaching science in grades K-8, Washington, DC: National Academies Press.
- National Research Council (NRC), (2012), A framework for K-12 science education: practices, crosscutting concepts, and core ideas, Washington, DC: The National Academies Press.
- National Research Council (NRC), (2013), The next generation science standards, Washington, DC: The National Academies Press.
- Ngai C., Sevian H. and Talanquer V., (2014), What is this substance? What makes it different? Mapping progression in students’ assumptions about chemical identity, Int. J. Sci. Educ., 36, 2438–2461.
- Redish E. F., (2004), A theoretical framework for physics education research: modeling student thinking, in Redish E. F. and Vicentini M. (ed.), Proceedings of the International School of Physics, “Enrico Fermi” Course CLVI, Amsterdam: IOS Press.
- Reiner M., Slotta J.D., Chi M.T.H. and Resnick L.B., (2000), Naive physics reasoning: a commitments to substance-based conceptions, Cognit. Instruct., 18(1), 1–34.
- Resnick M., (1996), Beyond the centralized mindset, J. Learn. Sci., 5, 1–22.
- Rogat A., Anderson A., Foster J., Goldberg F., Hicks J., Kanter D., et al., (2011), Developing learning progressions in support of the new science standards: a RAPID workshop series, New York: Consortium for Policy Research in Education, Teachers College, Columbia University.
- Russ R. S., Scherr R. E., Hammer D. and Mikeska J., (2008), Recognizing mechanistic reasoning in student scientific inquiry: a framework for discourse analysis developed from philosophy of science, Sci. Educ., 92(3), 499–525.
- Sanmartí N., Izquierdo M and Watson R., (1995), The substantialisation of properties, Sci. Educ., 4(4) 349–369.
- Sevian H. and Talanquer V., (2014), Rethinking chemistry: a learning progression on chemical thinking, Chem. Educ. Res. Pract., 15(1), 10–23.
- Sherin B. L., Krakowski M. and Lee V. R., (2012), Some assembly required: how scientific explanations are constructed during clinical interviews, J. Res. Sci. Teach., 49, 166–198.
- Sloman S. A., (1996), The empirical case for two systems of reasoning, Psychol. Bull., 119(1), 3–22.
- Solominodou C. and Stavridou H., (2000), From inert object to chemical substance: students’ initial conceptions and conceptual development during an introductory experimental chemistry sequence, Sci. Educ., 84, 382–400.
- Spelke E. S. and Kinzler K. D., (2007), Core knowledge, Dev. Sci., 10, 89–96.
- Stevens S., Delgado C. and Krajcik J. S., (2010), Developing a hypothetical multi-dimensional learning progression for the nature of matter, J. Res. Sci. Teach., 47, 687–715.
- Taber K. S., (1998), An alternative conceptual framework from chemistry education, Int. J. Sci. Educ., 20, 597–608.
- Taber K. S., (2002), Chemical misconceptions—Prevention, diagnosis and cure: vol. I: theoretical background, London: Royal Society of Chemistry.
- Taber K. S., (2003), The atom in the chemistry curriculum: fundamental concept, teaching model or epistemological obstacle? Found. Chem., 5 (1), 43–84.
- Taber K. S., (2008), Conceptual resources for learning science: issues of transience and grain-size in cognition and cognitive structure, Int. J. Sci. Educ., 30(8), 1027–1053.
- Taber K. S., (2009), College students' conceptions of chemical stability: the widespread adoption of a heuristic rule out of context and beyond its range of application, Int. J. Sci. Educ., 31(10), 1333–1358.
- Taber K. S., (2014), The significance of implicit knowledge in teaching and learning chemistry, Chem. Educ. Res. Pract., 15(4), 447–461.
- Taber K. S. and García-Franco A., (2010), Learning processes in chemistry: drawing upon cognitive resources to learn about the particulate structure of matter, J. Learn. Sci., 19(1), 99–142.
- Taber K. S. and Watts M., (2000), Learners’ explanations for chemical phenomena, Chem. Educ. Res. Pract., 1(3), 329–353.
- Talanquer V., (2006), Common sense chemistry: a model for understanding students’ alternative conceptions, J. Chem. Educ., 83(5), 811–816.
- Talanquer V., (2008), Students’ predictions about the sensory properties of chemical compounds: additive versus emergent frameworks, Sci. Educ., 92(1), 96–114.
- Talanquer V., (2009), On cognitive constraints and learning progressions: the case of structure of matter, Int. J. Sci. Educ., 31 (15), 2123–2136.
- Talanquer V., (2010), Exploring dominant types of explanations built by
general chemistry students, Int. J. Sci. Educ., 32 (18), 2393–2412.
- Talanquer V., (2011), Macro, submicro, and symbolic: the many faces of the chemistry “triplet”, Int. J. Sci. Educ., 33(2), 179–195.
- Talanquer V., (2013a), School chemistry: the need for transgression, Sci. Educ., 22, 1757–1773.
- Talanquer V., (2013b), How do students reason about chemical substances and reactions? in Tsaparlis G. and Sevian H. (ed.), Concepts of matter in science education, Dordrecht: Springer, pp. 331–346.
- Talanquer V. (2013c), When atoms want, J. Chem. Educ., 90 (11), 1419–1424.
- Talanquer V., (2015), Threshold concepts in chemistry: the critical role of implicit schemas, J. Chem. Educ., 92(1), 3–9.
- Talanquer V. and Pollard J., (2010), Let's teach how we think instead of what we know, Chem. Educ. Res. Pract., 11, 74–83.
- Talanquer V. and Pollard J., (2017), Reforming a large foundational course: Successes and challenges, J. Chem. Educ., DOI: http://10.1021/acs.jchemed.7b00397.
- Talmy L., (1988), Force dynamics in language and cognition, Cognit. Sci., 12(1), 49–100.
- Tsaparlis G. and Sevian H. (ed.), (2013), Concepts of matter in science education, Dordrecht: Springer.
- Tümay H., (2016), Reconsidering learning difficulties and misconceptions in chemistry: emergence in chemistry and its implications for chemical education, Chem. Educ. Res. Pract., 17, 229–245.
- Vosniadou S. (ed.), (2008), International handbook of research on conceptual change, New York: Routledge.
- Vosniadou S. and Ortony A. (ed.), (1989), Similarity and analogical reasoning, New York: Cambridge University Press.
- Vosniadou S., Vamvakoussi X. and Skopeliti I., (2008), The framework theory approach to the problem of conceptual change, in Vosniadou S. (ed.), International handbook of research on conceptual change, New York: Routledge, pp. 3–34.
- Weinrich M. and Talanquer V., (2015), Mapping students’ conceptual modes when thinking about chemical reactions used to make a desired product, Chem. Educ. Res. Pract., 16, 561–577.
- Yan F. and Talanquer V., (2015), Students’ Ideas about how and why chemical reactions happen: mapping the conceptual landscape, Int. J. Sci. Educ., 37, 3066–3092.
|
This journal is © The Royal Society of Chemistry 2018 |
Click here to see how this site uses Cookies. View our privacy policy here.