DOI:
10.1039/C8RA05640D
(Paper)
RSC Adv., 2018,
8, 29368-29377
High-resolution lipidomics reveals dysregulation of lipid metabolism in respiratory syncytial virus pneumonia mice†
Received
2nd July 2018
, Accepted 2nd August 2018
First published on 17th August 2018
Abstract
Respiratory syncytial virus (RSV) is a leading viral pathogen responsible for lower respiratory tract infections, particularly in children under five years worldwide, often resulting in hospitalization. At present, the molecular-level interactions between RSV and its host and the underlying mechanisms of RSV-induced inflammation are poorly understood. Herein, we describe an untargeted high-resolution lipidomics platform based on UHPLC-Q-Exactive-MS to assess the lipid alterations of lung tissues and plasma from a mouse model of RSV pneumonia. Untargeted lipidomics using LC-MS with multivariate analysis was applied to describe the lipidomic profiling of the lung tissues and plasma in RSV pneumonia mice. Lipid identification was conducted via an in silico MS/MS LipidBlast library using the MS-DIAL software. We observed distinct compartmental lipid signatures in the mice lung tissues and plasma and significant lipid profile changes between the systematic and localized host responses to RSV. A total of 87 and 68 differential lipids were captured in the mice lung tissue and plasma, respectively, including phospholipids, sphingolipids, acylcarnitine, and fatty acids. Some of these lipids belong to pulmonary surfactants, illustrating that RSV pneumonia-induced aberrations of the pulmonary surfactant system may play a vital role in the etiology of respiratory inflammation. Our findings reveal that the host responses to RSV and various lipid metabolic pathways were linked to disease pathology. Furthermore, our findings could provide mechanistic insights into RSV pneumonia.
1. Introduction
The respiratory syncytial virus (RSV) is the most common viral pathogen of pneumonia in children less than two years of age. It has been reported that RSV is the leading cause of infant hospitalization worldwide, and it is also a major contributor to child morbidity and mortality.1,2 To date, no effective vaccine has been licensed against the pneumovirus, and there are also very limited treatment options available for RSV.3 This implies that we only partially understand the mechanisms of RSV pathogenesis. Hence, there is an urgent need to comprehensively unravel the potential mechanisms of virus-linked pathogenic molecules and develop new treatments for this tough virus. One efficient approach is to identify and target host factors that regulate viral replication and pathogenesis. However, our understanding of the impact of RSV on hosts remains incomplete, particularly at the metabolic level. In our previous study, we found that metabolites were differentially expressed in plasma and lung from RSV infection in a mouse model, which indicated that many of these metabolites are associated with the regulation of surfactant lipids.4 Thus, in principle, the maintenance of surfactant lipids may provide a protective therapeutic strategy. In recent years, there has been renewed interest in the role of surfactant lipids.5–7 Apart from their well-known function of reducing the surface tension at the air–liquid interface, surfactant lipids have also been recognized as bioactive molecules that play a key role in immunity and inflammation. Complex interactions have been noted between surfactant lipids and viruses. These specific lipids can attenuate inflammation and modify the host response to respiratory viruses, such as influenza A virus and RSV. Unfortunately, to date, due to the limitations in accuracy of detection and identification of complicated lipids, to the best of our knowledge, there are no reports on the comprehensive surfactant lipidome profile in RSV-infected humans or animals, including our previous metabolomics study.
Lipidomics falls into the category of the more general field of metabolomics, which is a newly emerging discipline to study complex lipids on a large scale using the principles and techniques of analytical chemistry, particularly mass spectrometry.8–10 Lipidomics strategies can be divided into untargeted, targeted and semi-targeted approaches, each with their respective characteristics.11 Untargeted lipidomics is the analysis of all the detectable lipids in a sample, including unknowns. Conversely, targeted lipidomics focuses on the measurement of assured lots of lipids. Semi-targeted approaches fall between the untargeted and targeted strategies and aim to quantify hundreds of lipids, whose identity is known before data acquisition. The major differences between untargeted, semi-targeted and targeted studies are (1) the level of sample preparation required, (2) the number of lipids detected, and (3) the level of quantification of the lipids.
Untargeted high-resolution lipidomics can provide a powerful tool for analyzing the lipid structures, mass levels, cell functions and interactions in a spatial and temporal manner.12–14 Accordingly, in the present study, we describe an untargeted high-resolution lipidomics analysis to evaluate the lipidome alteration reflected in lung tissue and plasma from RSV pneumonia mice using high-resolution mass spectrometry (MS) and customized in silico MS/MS libraries using MS-DIAL15 and the LipidBlast template.16–18
MS-DIAL is an open-source software for data-independent acquisition-based identification and quantification of small molecules by mass spectral deconvolution.15 The detailed lipidomic signatures may provide a new and comprehensive appreciation of the importance of the host in viral pathogenesis and offer unprecedented opportunities for better diagnostics and therapeutics for RSV pneumonia.
2. Materials and methods
2.1 Virus, cell and chemicals
The prototype RSV Long strain (RSV/Long; group A) was obtained from the China Type Culture Collection, Wuhan University (Wuhan, China). The viruses were grown in human epithelial type 2 (HEp-2) cells (Cell Resources Center, Shanghai Institutes for Biological Sciences, Chinese Academy of Sciences) cultured in Dulbecco's modified Eagle's medium (DMEM; Wisent, Canada) supplemented with 10% fetal bovine serum, antibiotics, and glutamine.
LC-grade methanol, acetonitrile (ACN), isopropanol, and methyl tertiary-butyl ether (MTBE) were obtained from Merck (Darmstadt, Germany). HPLC-grade formic acid and ammonium formate were purchased from ROE Scientific Inc. (NY, USA). All aqueous solutions were prepared with deionized water purified by a Millipore Ultrapure water system (Massachusetts, USA).
2.2 Animals
Female BALB/c mice, all 6 weeks old and weighing 18–20 g (Beijing Vital River Laboratory Animal Technology Co., Ltd, Beijing, China), were housed in an SPF animal laboratory with a 12 h light/dark cycle. All rodents had free access to water and given food. The mice were provided 1 week to acclimatize before initiating the experiments.
As previously described,4 BALB/c mice were intranasally administered with RSV. After acclimatization for 1 week, forty mice were randomly divided into two groups (control and RSV). For the control group, the mice were intranasally administered with DMEM. The rodents in the RSV group were administered in each nostril with 50 μL intranasal RSV containing 50% of the tissue culture infective dose (TCID50: 105) for 3 consecutive days. The body weight of each mouse in both groups was examined daily. All procedures over mice were performed in accordance with the Guidelines for Care and Use of Laboratory Animals of Nanjing University of Chinese Medicine, and the experiments were approved by the Animal Ethics Committee of our university.
2.3 Sample collection
Five days after the first RSV administration, the mice were euthanized and blood was collected from their lateral caudal tail vein in 300 μL HBSS buffer containing 8 mmol L−1 EDTA. The collected blood was centrifuged (14
000 g) for 30 minutes at 4 °C, and the supernatants were stored at −80 °C until further analysis. Bronchoalveolar lavage was performed following cardiac puncture using a cannula surgically inserted into the trachea. Ice-cold PBS (3 × 500 μL) was instilled into the airways and a consistent volume of BALF (1400–1450 μL) was recovered from each mouse. The lavage was centrifuged to form a cell pellet, and the BALF was stored at −80 °C until further analysis. The excised lung tissues were rinsed in ice-cold PBS, and the lobules of the lungs were collected for histopathological examination; the other parts were stored at −80 °C for lipidomic analysis.
2.4 Histopathology
The lung tissues from each mouse were fixed in 4% neutral buffered paraformaldehyde for 12 h dehydration. Then, they were embedded in paraffin wax to slice into 3 μm-thick sections and stained by hematoxylin and eosin (H&E) staining. Tissue lesions and inflammatory cell infiltrations were examined using a microscope.
2.5 Measurements of inflammatory mediators
The cytokine levels of IL-6 and TNF-α were evaluated using ELISA kits in accordance with the manufacturer's protocol.
2.6 Sample preparation
To perform a comprehensive lipidomic profiling, we developed a sample preparation strategy for plasma and lung tissue, as described previously,13,19,20 for covering different classes of lipids based on the liquid–liquid MTBE extraction.
Briefly, frozen plasma (20 μL) or lung tissue (4 mg) was first sonicated in a water bath for 5 min with 225 μL ice-cold methanol containing a mixture of odd chain and deuterated lipid internal standards lysoPE(17:1) and SM(17:0) for positive ion mode and lysoPE(17:1) and PE(17:0/17:0) for negative ion mode. Next, 750 μL MTBE was added, and the mixture were shaken for 10 min at 4 °C. After the addition of 200 μL of deionized water, the samples were vortexed and then centrifuged at 17
000 rpm at 4 °C. The upper (organic) phase, which mainly contained lipids, was transferred to fresh tubes and dried in a vacuum centrifuge. Finally, the upper phase lipids were reconstituted with 60 μL isopropanol
:
methanol (4
:
1) for LC-MS analysis.
2.7 Untargeted lipidomic analysis
A Dionex UltiMate 3000 ultra-high performance liquid chromatography (UHPLC) system (Santa Clara, CA, USA) coupled online via an electrospray ionization source (ESI) with a Q Exactive™ MS instrument (Thermo Fisher Scientific, USA) was applied for the untargeted lipidomic analysis.
For the detection of lipids, 2 μL aliquots of sample solution, maintained at 4 °C in an auto sampler, was injected onto a reversed phase Waters Acquity UPLC CSH C18 (100 mm × 2.1 mm, 1.7 μm) maintained at 60 °C by gradient elution. Mobile phase A was 40% ACN in water and mobile phase B was isopropanol
:
ACN (9
:
1), both containing 5 mM ammonium formate and 0.1% formic acid. The flow rate was 0.3 mL min−1, with the elution gradient as follows: 0–4.0 min, 15% B; 4.0–5.0 min, 15–48% B; 5.0–22.0 min, 48–82% B; 22.0–23.0 min, 82–99% B; 23.0–24.0 min, 99% B; 24.0–24.2 min, 99–15% B; and 24.2–30.0 min, 15% B.
All MS experiments were performed in positive and negative ion modes using a heated electrospray ionization source. The source and ion transfer parameters applied were as follows: spray voltage 3.5 kV (positive) and 2.8 kV (negative). For both ionization modes, the sheath gas, aux gas, capillary temperature and heater temperature were maintained at 35, 15 (arbitrary units), 325 °C and 300 °C, respectively. The S-Lens RF level was set at 50. The Orbitrap mass analyzer was operated at a resolving power of 60
000 in full-scan mode (scan range: 150–1500 m/z; automatic gain control (AGC) target: 1 × 106) and 15
000 in the Top 10 data-dependent MS2 mode (stepped normalized collision energy: 25 and 30; injection time: 250 ms; isolation window: 1.0 m/z; AGC target: 1 × 105) with a dynamic exclusion setting of 10.0 s.
2.8 Data processing and lipid identification
Raw data files acquired from the Xcalibur 2.2 software (Thermo Scientific) were converted to the ABF format using the ABF converter (accessible at: http://www.reifycs.com/AbfConverter). For data processing, the MS-DIAL (v. 2.78) software program was used. The following parameters were used: retention time begin: 1.5 min; retention time end: 27.5 min; mass range begin: 215 Da; mass range end: 1800 Da; MS1 (centroiding) tolerance: 0.01 Da; smoothing level: 3 scans; minimum peak height: 10
000 amplitude; mass slice width: 0.1 Da; accurate mass tolerance (MS1): 0.01 Da; accurate mass tolerance (MS2): 0.05 Da; retention time tolerance for alignment: 0.1 min; MS1 tolerance for alignment: 0.025 Da.
For lipid identification, accurate mass and MS/MS matching was used with the public LipidBlast library of over 200
000 MS/MS spectra,16–18 covering 11 lipid classes and various molecular species: PC (phosphatidylcholine), PE (phosphatidylethanolamine), PS (phosphatidylserine), PG (phosphatidylglycerol), PI (phosphatidylinositol), lysoPC (lysophosphatidylcholine), lysoPE (lysophosphatidylethanolamine), lysoPS (lysophosphatidylserine), lysoPG (lysophosphatidylglycerol), lysoPI (lysophosphatidylinositol), SM (sphingomyelin), DG (diacylglycerol), TG (triacylglycerol), CL (cardiolipin), Cer (ceramides), ChE (cholesterol ester), CerNS (ceramide non-hydroxy fatty acid-sphingosine), CerNDS (ceramide non-hydroxy fatty acid-dihydrosphingosine), glucosylceramide non-hydroxy fatty acid-dihydrosphingosine (GlcCerNDSd), plasmenyl-PC, plasmenyl-PE and others. Adduct ions were +H, +Na and +NH4 for the positive ion mode and −H, +HCOO and −2H for the negative ion mode. Reverse dot products lower than 700 were not considered.
2.9 Statistical analysis
Multivariate analysis was performed using MetaboAnalyst 4.0 (http://www.metaboanalyst.ca21). Statistical models were created for the TIC normalized data (median) after logarithmic transformation (base 10) and Pareto scaling. The volcano plot shows the significance and the fold change of each identified lipid. The significant lipids were selected by the volcano plot with a fold change threshold > 1.5 (or < 0.5) and p value < 0.05 using the Mann–Whitney test with the Benjamini–Hochberg false discovery rate (FDR) multiple testing correction.22 Principal component analysis (PCA) was performed, and model evaluation with permutation strategy was conducted.
3. Results
3.1 Temporal pathophysiologic profiles in RSV pneumonia mice
We first established the temporal pathophysiologic profiles in RSV pneumonia mice, and the results obtained were consistent with the previous characterization of the same model.4 As shown in Fig. 1A, we observed body weight gain in the normal mice, whereas weight loss was observed in the RSV group with an increase in number of days. This change in body weight was significant (p < 0.01). The lung index, which was calculated as a parameter of pneumonia caused by RSV, was higher in the model group than that in the control group (p < 0.01) (Fig. 1B). Representative histological sections of the lung tissue from the control and RSV-infected mice are shown in Fig. 1C. The pulmonary sections from the control mice exhibited few or no changes in histopathology. In contrast, infiltration of the inflammatory cells (lymphocytes, macrophages, and polymorph nuclear neutrophils) and thickened alveolar and bronchial walls were clearly observed in the lung sections from the RSV-infected mice.
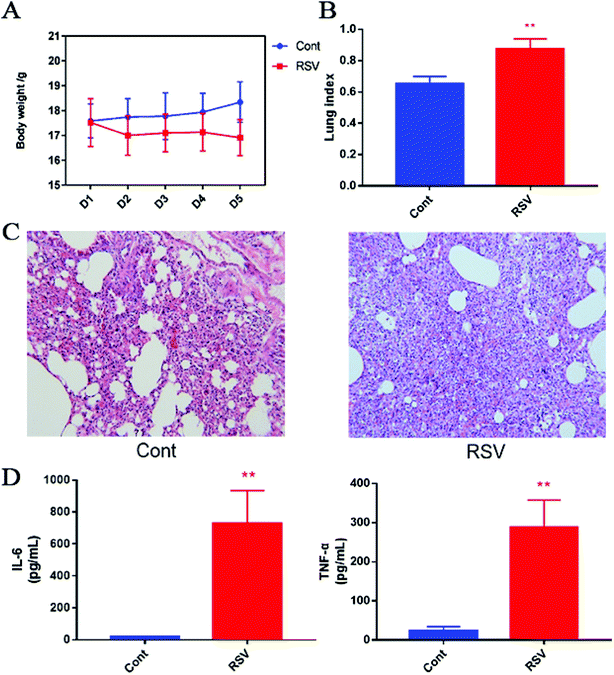 |
| Fig. 1 RSV-induced body weight loss (A), lung index (B), pulmonary histopathological damage (C) and inflammation cytokines changes (D) in the mice. Values are expressed as mean ± SD. (n = 6), **p < 0.01 vs. normal control mice. | |
3.2 RSV-induced pulmonary inflammation and cytokine alteration
BALF cytokines of the control and RSV infected mice were also determined. The results presented in Fig. 1D show that RSV infection led to concomitant increase in IL-6 and TNF-α as compared to those in the normal controls (p < 0.01).
3.3 Data quality assurance
QC samples were provided by pooling equal aliquots of lung tissue or plasma from each sample, and a total of 10 samples were injected into the instrument to monitor the stability of the instrumental analysis and robustness of the sample preparation. The 10 QCs were first tested before the analysis to stabilize the analytical system.23 Tight clustering of the QCs in the PCA scores was observed (Fig. S1†), indicating the good reproducibility of the data.24 The RSD of the intensity and retention time of the lipid internal standards was less than 16% and 0.1 min, respectively. Overall, it is possible to demonstrate that the reproducibility of the current method is competent to ensure data quality in untargeted lipidomic profiling applications by closely monitoring the QC data.25
3.4 Putative lipid identification
We putatively identified the lipids from the LC-MS positive and negative ionizations using the MS-DIAL software coupled with the LipidBlast library, including lysoPC, lysoPE, lysoPG, PC, PE, and PG (Fig. S2†). For lung tissue, we identified a total of 192 lipids in the positive ionization mode and 103 lipids in the negative ionization mode, with the common identification of 30 lipids (Tables S1 and S2,† respectively). For plasma, 177 lipids were identified in the positive ionization mode, whereas 62 lipids were identified in the negative ionization mode, with 8 lipids identified in both negative and positive modes (Tables S3 and S4,† respectively). In summary, we identified a total of 265 lipids in the lung tissue and 231 lipids in the plasma sample.
3.5 RSV infection leads to altered lung lipidome
To provide a first-hand lipidomic understanding of the RSV infected lung tissues, a high-resolution lipidomics approach was used to identify potential novel RSV-induced lipidomic changes in the lungs. The preliminary PCA model of the global lipid changes in the lung tissue reveals consistent separation of the RSV infected lung lipidomic profiles in both positive ionization and negative ionization modes (Fig. 2A and B, respectively) as compared to the normal controls. The heatmap analysis also shows different lipidomic fingerprints between the two groups of mice (Fig. 3A and B, respectively).
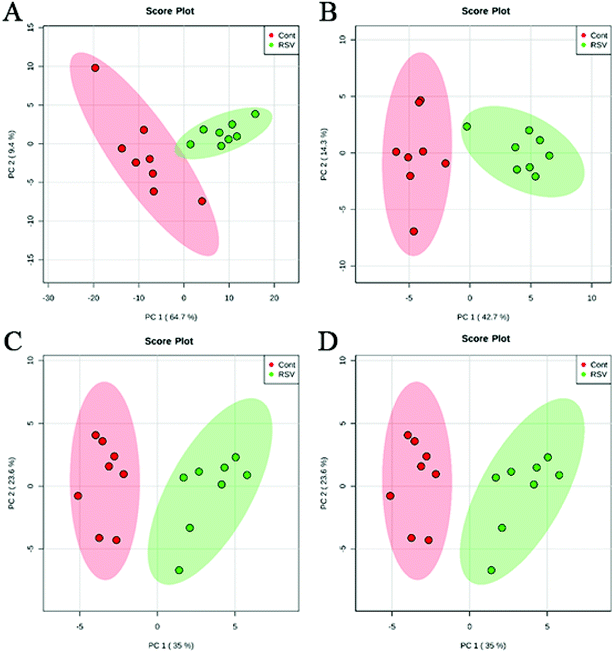 |
| Fig. 2 PCA score plot based on the lipid profiling of RSV pneumonia mice and the normal control mice. (A) lung ESI(+), (B) lung ESI(−), (C) plasma ESI(+), and (D) plasma ESI(−). | |
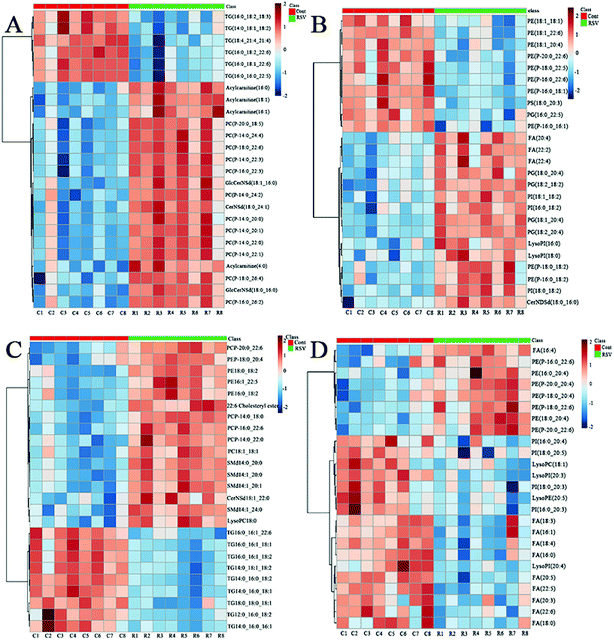 |
| Fig. 3 Heatmaps illustrating the lipid profiles of the RSV pneumonia mice versus the normal control mice: (A) lung ESI(+), (B) lung ESI(−), (C) plasma ESI(+), and (D) plasma ESI(−). The top 25 lipid features were ranked using the t test, distance was measured using the Pearson correlation, and clustering was determined using the Ward algorithm. C1–C8 represent the normal control mice and R1–R8 represent the RSV pneumonia mice. | |
In the volcano plot (Fig. 4A and B), the identified lipids are presented as log
2-fold changes against the −log
10 (p) of the differential expression between the RSV infected mice and normal controls. The volcano plot analysis reveals 64 specific lung lipids above the threshold (FC > 1.5 or FC < 0.67, and p < 0.05) in the positive ionization mode and 29 in the negative ionization mode, with 7 identical lipids, which were significantly changed in the RSV-infected mice as compared to the normal controls (Tables S5 and S6†).
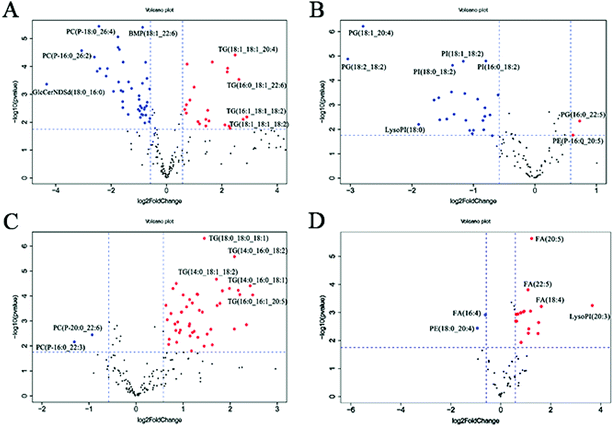 |
| Fig. 4 Volcano plots of lipids of the RSV pneumonia mice versus the normal control mice: (A) lung ESI(+), (B) lung ESI(−), (C) plasma ESI(+), and (D) plasma ESI(−). The x-axis represents the fold change (threshold of 1.5 or 0.67) and the y-axis the adjusted t-test (threshold of 0.05). Both values are median-normalized and log transformed. | |
In total, there were 86 altered lipids in the lung tissue, which induced losses in levels of the DG and TG species and gains in levels of acylcarnitine, CerNDSd, FA, PI, lysoPI and plasmalogen lipids, including plasmenyl-PC and plasmenyl-PE. Interestingly, the effects of infection on the PG-based lipids were variable. Compared to those in the normal control, the levels of palmitoylated PG species such as PG(16:0_22:5), PG(16:0_22:6) and PG(16:0_18:1) (p < 0.05, but FC = 0.69) decreased, but the levels of stearoylated PG lipids such as PG(18:2_20:4), PG(18:2_18:2) and PG(18:1_20:4) increased in the lung tissue infected by RSV. GlcCerNDSd(18:0_16:0), PG(18:2_20:4) and lysoPI(16:0) were the most upregulated lipids in the RSV infection group compared to those in control, while TG(16:1_18:1_18:2) was the most downregulated lipid, as shown in Tables S5 and S6.†
3.6 RSV-induced lung inflammation promotes plasma lipidome changes
To complement the lipidome changes in lung tissues, we sought corresponding distinctions in the plasma lipidomic profiles between the normal control and RSV pneumonia mice using PCA (Fig. 2C and D). Similar to the results lung tissue, the results indicate perfect separation of the plasma lipid profiles between the two groups of mice. The heatmap analysis also shows that RSV induced significant changes in the plasma lipids (Fig. 3C and D).
A total of 68 differentially expressed lipids (49 in the positive ionization mode and 19 in the negative ionization mode, as shown in Tables S7 and S8,† respectively) were identified above the threshold (FC > 1.5 or FC < 0.67, and p < 0.05), which are marked and presented in Fig. 4C and D. There were induced losses in the levels of majority of lysophospholipids, PI, FA and TG, whereas gains in levels of plasmenyl-PC were observed, as identically observed in the lung tissue. However, acylcarnitines were the exception. The levels of this type of lipid decreased in the plasma but increased in the lung tissue. LysoPI(20:3) was the most downregulated lipid in the RSV infection group compared to that in the control, while PC(P-16:0_22:3) was the most upregulated.
3.7 Statistical associations between lung and plasma lipids altered by RSV infection
Next, we compared the specific lipid changes in the lung tissue and plasma following RSV infection in this model of experimental pneumonia. In total, eight of the dysregulated lipids were common to both the lung tissue and plasma, which were further examined to determine whether their response was concordant or discordant between the lung tissue and plasma. For example, DG(18:1_18:2) and five TG were downregulated, whereas PC(P-16:0_22:3) was upregulated in both lung tissue and plasma in the RSV-administered mice compared to the control group. In contrast, lysoPC(18:1), the levels of acylcarnitine(4:0), acylcarnitine(16:0), acylcarnitine(16:1) and FA(22:6) increased in the lung tissue but decreased in the plasma. Furthermore, correlation analysis was employed to explore potential biological links between the lung and plasma lipids (Table S9†). Our correlation analysis identified multiple significant associations between the RSV-altered lung and plasma lipids. The majority of plasma lipids except plasmalogen PC and PE were negatively related to acylcarnitines, CerNS, FA, and most of the phospholipids in the lung tissue, but were positively related to lung DG, TG and two special palmitoylated PG.
4. Discussion
Lipidomics is a rapidly evolving field to fill the crucial gap in systems biology of disease by recognizing variations in lipid abundance and/or flux in biological systems in response or relation to disease or experimental perturbation.9 However, there are limited reports on mass spectrometry-based lipidomics studies on RSV-infected cell lines and animal models.4 Thus, in the present study, to provide a first-hand experimental understanding of the specific lipidomic changes in RSV pneumonia, we applied an LC-MS-based high-resolution lipidomics analysis to identify the potential disease relevant lipid alterations in lung tissues and plasma of RSV pneumonia mice. Our present investigation successfully uncovered the RSV induced lipidomic profile alterations in the lung and plasma of mice 5 days after infection, with severe body weight loss, increased lung injury, and higher secretion of pro-inflammatory cytokines.
To further elucidate the possible functional roles of these changed lipids in response to RSV infection-induced inflammation, a hypothetical metabolic network using the identified lipids was reconstructed by referring to the Kyoto Encyclopedia of Genes and Genomes (KEGG) and LIPID MAPS® Lipidomics Gateway (Fig. 5).
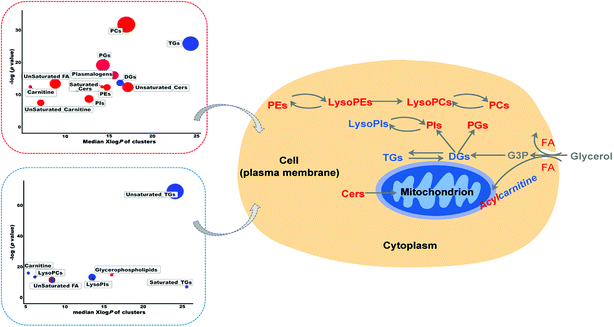 |
| Fig. 5 Metabolic dysregulations and their mapping against the pathway. Each node reflects a significantly altered cluster of metabolites. Enrichment p-values are given by the Kolmogorov–Smirnov-test. Node sizes represent the total number of metabolites in each cluster set. Red-color represents the increased metabolites and the blue-color shows the decreased compounds in the RSV mice compared to the normal control mice. Purple-color nodes have both increased and decreased metabolites. | |
The lipids displaying a trend of increased levels in the pulmonary surfactants after RSV infection were found to be phospholipids. Pulmonary surfactants are a complex mixture comprising 90% lipids and 10% proteins by weight. Approximately 80% of all surfactant lipids are phospholipids, and the phospholipid composition of these surfactants is highly conserved in mammals.26 About half of the surfactant PC, which is the most abundant surfactant phospholipid species overall, is PC(16:0/16:0), also named DPPC. DPPC and related PC species are also the major components of the outer leaflet of the plasma membrane.26 It was slightly strange that there were no significantly changes in PCs, except PC(18:2_18:2) at 5 days after RSV infection.
The other major phospholipid component of the pulmonary surfactants is PG, which is the second-most enriched surfactant and may be central to the surface tension-lowering properties of these surfactants.27 In the lung tissues, we found that RSV infection resulted in a reduction in the palmitoylated PG species, which is consistent with the previous report about lung disease caused by respiratory virus.28 It is very important to note that the level of stearoylated PG lipids increased in the lung tissue infected by RSV. The previous experiment of PG lipid turnover rate in mice revealed that PG(16:0/16:0) converted to PG(16:0/18:1), PG(16:0/16:1) and PG(16:0/18:2) at the earliest time (0–60 min). At longer post inoculation times (60–360 min), the levels of these molecular species continued to increase, and the stearoylated PG molecular species including PG(18:0/20:4) and PG(18:0/22:6) began to appear.29 Our current study detected the lipids in the lung tissue 5 days after RSV infection. Thus, we found the phenomenon that the levels of palmitoylated PG lipids decreased, while the levels of stearoylated PG lipids increased after RSV infection. This indicates that the life cycle including synthesis, secretion and recycling of surfactant lipid-PG may undergo complicated changes after RSV infection, which makes them even more worthy of further investigation. Recent findings revealed that membrane lipid-PG is a potent regulator of innate immunity and respiratory viral infection.30 A few studies reported that both unsaturated PG molecular species, including PG(16:0/18:1), PG(18:0/18:1), PG(18:1/18:1) and PG(18:2/18:2), and saturated PG molecular species PG(16:0/16:0) could antagonize RSV-induced IL-8 production with similar IC 50 values. PG(16:0/16:0) may block RSV attachment to epithelial cells and then inhibit the virus infection in vitro. In the in vivo experiment, PG(16:0/16:0) markedly attenuated infection and associated inflammatory responses in RSV-infected mice, which demonstrated that PG(16:0/16:0) is effective for the short-term protection of mice against subsequent RSV infection and that it has potential for application in humans.29,30
PE is also enriched in mitochondrial membranes.31 The levels of PE(16:0_22:6) and PE(18:0/18:2) in the lung tissue and PE(18:0/20:4) in the plasma all increased significantly. In addition to the above major surfactant phospholipids (PC, PG and PE), pulmonary surfactants contain smaller amounts of PI, PS and SM.27 PI is a minor surfactant phospholipid and is synthesized from cytidine 5′-diphospho-diacylglycerol (CDP-DG) and myo-inositol.31,32 The levels of PI and lysoPI in the lung tissue were both significantly elevated after infection; particularly, the level of lysoPI(16:0) increased up to ten-fold compared to that in the control group. However, complex changes in PI occurred in the plasma. Levels of some surfactant lipid-PI, such as PI(18:0_20:5) and PI(18:0_22:6) exhibited the same increase as that in the lung tissue, whereas levels of some lipids including PI(16:0_20:4) and lysoPI(20:4) decreased in the plasma. PS is generated from PC or PE via base change with serine. This phospholipid is also enriched in the inner leaflet of the plasma membrane and in endosomal membranes.33 We detected several PS lipids in the lung tissue, but the levels of only PS(18:0_20:3) increased significantly (not shown, less than 1.5-fold). Sphingomyelin is a type of sphingolipid found in cell membranes, particularly in the membranous myelin sheath that surrounds some nerve cell axons.34 RSV infection increased the levels of SMd(14:0_20:0) and SMd(14:0_20:1).
In addition, we determined quite a few plasmalogen lipids in the lung tissue and plasma. Plasmalogen lipids are a unique class of membrane glycerophospholipids, which contain a fatty alcohol with a vinyl ether bond at the sn-1 position and are enriched in polyunsaturated fatty acids at the sn-2 position of the glycerol backbone.35,36 In this study, we found that the levels of most of the plasmenyl-PC and plasmenyl-PE increased notably in the lung tissue and plasma with the exception of only a few plasmalogen lipids. This suggests that plasmalogen lipids may play key roles in normal lung physiology. These lipids may protect against pulmonary disease due to their antioxidant role because the lung is a direct target of reactive oxidant species.35 Moreover, lung plasmalogens also play a structural role as surfactants and functional role in immune defenses.37 However, further investigations need to be performed to obtain a complete understanding of the plasmalogen lipid link to RSV pneumonia.
Acylcarnitines, which are acyl esters of carnitine, are essential compounds for fatty acid oxidation (FAO) and organic acid metabolism.38 In our results, the levels of all of the acylcarnitines significantly decreased in the plasma but increased remarkably in the lung tissue. As a class of lipids secreted by mitochondria, a recent study has shown that long-chain acylcarnitines may represent a risk factor for lung injury in humans with dysfunctional FAO.39 This type of lipid would accumulate at the air–fluid interface in long-chain acyl-CoA dehydrogenase−/− lungs when metabolism is inhibited.40 Acylcarnitine accumulation is exacerbated by respiratory viruses such as the influenza virus or by dietary supplementation with L-carnitine. Furthermore, long-chain acylcarnitines can co-localize with pulmonary surfactant phospholipids and proteins that reduce surface tension and prevent alveolar collapse during breathing. In vitro, the long-chain species palmitoylcarnitine directly inhibits the surface adsorption of pulmonary surfactants as well as their ability to reduce surface tension.
Finally, we also detected several FAs and found that their levels almost uniformly increased in the lung tissue but decreased in the plasma. RSV infection led to a decrease in levels of TG lipids in both the plasma and lung tissue, which was also observed in case of the DG lipids.
5. Conclusion
In conclusion, based on our collective results, for the first time, we revealed a comprehensive description of the lipidome alterations in the lung and plasma of a mouse model of RSV pneumonia by high-resolution lipidomics using UHPLC coupled with MS. We observed distinctive compartmental lipid signatures between the lung tissue and plasma, which indicate the lipid differences between systematic and localized host responses to RSV. A total of 87 and 68 differential lipids, including phospholipids, sphingolipids, acylcarnitines, and fatty acids, were discovered in the lung tissue and plasma, respectively. Through our approach, we were able to offer mechanistic insights into RSV-induced lipids, particularly the surfactant disturbances related to inflammation, and the corresponding response of the host towards RSV infection. The differentially regulated lipids are potential candidates as biomarkers for RSV pneumonia. In the future, it is essential to analyze the related lipids in biological samples, particularly in alveolar type 2 cells to illuminate their roles in cellular functioning and pathophysiological events.
Conflicts of interest
There are no conflicts to declare.
Acknowledgements
This study was supported by the National Natural Science Foundation of China (No.81774156); the Natural Science Foundation of Jiangsu Province (BK20161573, BK20151004); the Major Research Plan of Natural Science Foundation of the Higher Education Institutions of Jiangsu Province (16KJA360002, 17KJB360007); Jiangsu Provincial 333 High Levels Talents Cultivation Project (BRA2016427); Jiangsu Provincial Six Talent Peaks Project (YY-022); the Project of the Priority Academic Program Development of Jiangsu Higher Education Institutions (PAPD).
References
- H. Nair, D. J. Nokes, B. D. Gessner, M. Dherani, S. A. Madhi, R. J. Singleton, K. L. O'Brien, A. Roca, P. F. Wright, N. Bruce, A. Chandran, E. Theodoratou, A. Sutanto, E. R. Sedyaningsih, M. Ngama, P. K. Munywoki, C. Kartasasmita, E. A. Simoes, I. Rudan, M. W. Weber and H. Campbell, Lancet, 2010, 375, 1545–1555 CrossRef.
- I. Rudan, C. Boschi-Pinto, Z. Biloglav, K. Mulholland and H. Campbell, Bull. W. H. O., 2008, 86, 408–416 CrossRef PubMed.
- C. Griffiths, S. J. Drews and D. J. Marchant, Clin. Microbiol. Rev., 2017, 30, 277–319 CrossRef PubMed.
- L. N. Du, T. Xie, J. Y. Xu, A. Kang, L. Q. Di, J. J. Shan and S. C. Wang, J. Ethnopharmacol., 2015, 174, 25–36 CrossRef PubMed.
- M. B. Fessler and R. S. Summer, Am. J. Respir. Cell Mol. Biol., 2016, 54, 624–635 CrossRef PubMed.
- S. Han and R. K. Mallampalli, Ann. Am. Thorac. Soc., 2015, 12, 765–774 CrossRef PubMed.
- R. Veldhuizen, K. Nag, S. Orgeig and F. Possmayer, Biochim. Biophys. Acta, Mol. Basis, 1998, 1408, 90–108 CrossRef.
- M. R. Wenk, Nat. Rev. Drug Discovery, 2005, 4, 594–610 CrossRef PubMed.
- X. Han, Nat. Rev. Endocrinol., 2016, 12, 668–679 CrossRef PubMed.
- M. Lagarde, A. Geloen, M. Record, D. Vance and F. Spener, Biochim. Biophys. Acta, 2003, 1634, 61 CrossRef.
- T. Cajka and O. Fiehn, Anal. Chem., 2016, 88, 524–545 CrossRef PubMed.
- X. Han, K. Yang and R. W. Gross, Mass Spectrom. Rev., 2012, 31, 134–178 CrossRef PubMed.
- T. Cajka and O. Fiehn, TrAC, Trends Anal. Chem., 2014, 61, 192–206 CrossRef PubMed.
- M. F. Buas, H. Gu, D. Djukovic, J. Zhu, C. W. Drescher, N. Urban, D. Raftery and C. I. Li, Gynecol. Oncol., 2016, 140, 138–144 CrossRef PubMed.
- H. Tsugawa, T. Cajka, T. Kind, Y. Ma, B. Higgins, K. Ikeda, M. Kanazawa, J. VanderGheynst, O. Fiehn and M. Arita, Nat. Methods, 2015, 12, 523–526 CrossRef PubMed.
- T. Kind, K. H. Liu, D. Y. Lee, B. DeFelice, J. K. Meissen and O. Fiehn, Nat. Methods, 2013, 10, 755–758 CrossRef PubMed.
- T. Kind, Y. Okazaki, K. Saito and O. Fiehn, Anal. Chem., 2014, 86, 11024–11027 CrossRef PubMed.
- T. Cajka and O. Fiehn, Methods Mol. Biol., 2017, 1609, 149–170 CrossRef PubMed.
- V. Matyash, G. Liebisch, T. V. Kurzchalia, A. Shevchenko and D. Schwudke, J. Lipid Res., 2008, 49, 1137–1146 CrossRef PubMed.
- S. Chen, M. Hoene, J. Li, Y. Li, X. Zhao, H. U. Haring, E. D. Schleicher, C. Weigert, G. Xu and R. Lehmann, J. Chromatogr. A, 2013, 1298, 9–16 CrossRef PubMed.
- J. Chong, O. Soufan, C. Li, I. Caraus, S. Li, G. Bourque, D. S. Wishart and J. Xia, Nucleic Acids Res., 2018, 46(W1), W486–W494 CrossRef PubMed.
- D. I. Broadhurst and D. B. Kell, Metabolomics, 2006, 2, 171–196 CrossRef.
- W. B. Dunn, I. D. Wilson, A. W. Nicholls and D. Broadhurst, Bioanalysis, 2012, 4, 2249–2264 CrossRef PubMed.
- H. G. Gika, G. A. Theodoridis, M. Earll and I. D. Wilson, Bioanalysis, 2012, 4, 2239–2247 CrossRef PubMed.
- S. Naz, M. Vallejo, A. Garcia and C. Barbas, J. Chromatogr. A, 2014, 1353, 99–105 CrossRef PubMed.
- M. Agassandian and R. K. Mallampalli, Biochim. Biophys. Acta, 2013, 1831, 612–625 CrossRef PubMed.
- J. Bernardino de la Serna, S. Hansen, Z. Berzina, A. C. Simonsen, H. K. Hannibal-Bach, J. Knudsen, C. S. Ejsing and L. A. Bagatolli, Biochim. Biophys. Acta, 2013, 1828, 2450–2459 CrossRef PubMed.
- P. S. Woods, L. M. Doolittle, L. E. Rosas, L. M. Joseph, E. P. Calomeni and I. C. Davis, Am. J. Physiol., 2016, 311, L1160–L1169 Search PubMed.
- M. Numata, Y. Nagashima, M. L. Moore, K. Z. Berry, M. Chan, P. Kandasamy, R. S. Peebles Jr, R. C. Murphy and D. R. Voelker, J. Lipid Res., 2013, 54, 2133–2143 CrossRef PubMed.
- M. Numata, H. W. Chu, A. Dakhama and D. R. Voelker, Proc. Natl. Acad. Sci. U. S. A., 2010, 107, 320–325 CrossRef PubMed.
- J. E. Vance and G. Tasseva, Biochim. Biophys. Acta, 2013, 1831, 543–554 CrossRef PubMed.
- J. E. Vance, Traffic, 2015, 16, 1–18 CrossRef PubMed.
- J. E. Vance, J. Lipid Res., 2018, 59, 923–944 CrossRef PubMed.
- D. Milhas, C. J. Clarke and Y. A. Hannun, FEBS Lett., 2010, 584, 1887–1894 CrossRef PubMed.
- N. E. Braverman and A. B. Moser, Biochim. Biophys. Acta, 2012, 1822, 1442–1452 CrossRef PubMed.
- R. Maeba, M. Nishimukai, S. Sakasegawa, D. Sugimori and H. Hara, Adv. Clin. Chem., 2015, 70, 31–94 Search PubMed.
- S. Karnati and E. Baumgart-Vogt, Histochem. Cell Biol., 2009, 131, 447–454 CrossRef PubMed.
- P. Rinaldo, T. M. Cowan and D. Matern, Genet. Med., 2008, 10, 151–156 CrossRef PubMed.
- C. Otsubo, S. Bharathi, R. Uppala, O. R. Ilkayeva, D. Wang, K. McHugh, Y. Zou, J. Wang, J. F. Alcorn, Y. Y. Zuo, M. D. Hirschey and E. S. Goetzman, J. Biol. Chem., 2015, 290, 23897–23904 CrossRef PubMed.
- E. S. Goetzman, J. F. Alcorn, S. S. Bharathi, R. Uppala, K. J. McHugh, B. Kosmider, R. Chen, Y. Y. Zuo, M. E. Beck, R. W. McKinney, H. Skilling, K. R. Suhrie, A. Karunanidhi, R. Yeasted, C. Otsubo, B. Ellis, Y. Y. Tyurina, V. E. Kagan, R. K. Mallampalli and J. Vockley, J. Biol. Chem., 2014, 289, 10668–10679 CrossRef PubMed.
Footnotes |
† Electronic supplementary information (ESI) available. See DOI: 10.1039/c8ra05640d |
‡ These authors contributed equally to this work. |
|
This journal is © The Royal Society of Chemistry 2018 |