DOI:
10.1039/C8RA04993A
(Paper)
RSC Adv., 2018,
8, 34408-34417
Genome-wide identification and characterization of long non-coding RNAs responsive to Dickeya zeae in rice†
Received
11th June 2018
, Accepted 9th September 2018
First published on 8th October 2018
Abstract
Plant long non-coding RNA (lncRNA) is a type of newly emerging epigenetic regulator playing a critical role in plant growth, development, and biotic stress responses. However, it is unknown whether lncRNAs are involved in resistance responses between rice and Dickeya zeae, a bacterial agent causing rice foot rot disease. In this study, RNA-seq was performed to uncover the co-expression regulating networks mediated by D. zeae responsive lncRNAs and their candidate target genes. Of the 4709 lncRNAs identified, 2518 and 2191 were up- and down-regulated in response to D. zeae infection, respectively. Expression changes of 17 selected lncRNAs and their predicted targets with a potential role in defense response were investigated by qPCR. The expression levels of five lncRNAs were up-regulated while their cognate candidate target genes were down-regulated upon D. zeae infection. In addition, several lncRNAs were predicted to be target mimics of osa-miR396 and osa-miR156. These results suggest that lncRNAs might play a role in response to D. zeae infection by regulating the transcript levels of their targets and miRNAs in rice.
Introduction
Rice (Oryza sativa L.) is one of the most important grain crops globally. Rice production is limited by pathogens such as fungi, bacteria and viruses. Rice foot rot disease caused by the pathogenic bacteria D. zeae (formerly known as Erwinia chrysanthemi pv. zeae), is a destructive bacterial disease threatening rice yield and quality.1 Several outbreaks and epidemics in China resulting in significant yield losses were observed after the first occurrence of rice foot rot disease in Japan in the 70s of the 20th century.1,2 Multiple resurgences in contemporary rice fields in China were largely due to adoption of new cultivation methods and different climate conditions in China.1 Studies on the pathogenic mechanism of D. zeae suggest that bacterial toxin zeamine and a two-component regulatory system have played an important role in its pathogenicity.3 Cultivating and planting resistant rice varieties against D. zeae is one of the most economical and effective methods for controlling this bacterial disease. However, the underlying genetic mechanism resistant to D. zeae is unknown in rice.
Similar to other plant species, rice has evolved a sophisticated defense mechanism to protect itself from constant invasions of pathogenic microbes.4 Currently, increasingly studies discovered that lncRNAs play a role in resistance of a diverse of fungal and bacterial diseases in rice.5,6 Based on the genome location, lncRNA are divided into three categories: (1) long intergenic non-coding RNAs (lincRNAs), (2) natural antisense transcripts (NATs) and (3) intronic lncRNAs.7
Coupled with the fast development of genome-wide transcriptome sequencing technology, a large amount of lncRNAs responsive to biotic and abiotic stresses have been identified in plants.8,9 Recently, a great number of differentially expressed lncRNAs were detected in rice and Arabidopsis after infection with the fungal pathogens, Magnaporthe oryzae and Fusarium oxysporum, respectively.10,11 Moreover, deep sequencing profiling uncovered a large number of lncRNAs in rice.12,13 The biological functions of most lncRNAs are still unknown although a few lncRNAs were shown to play a role in plant growth and development. For example, the expression level of a male-fertility-associated RNA (lncRNA LDMAR) was inhibited under long day conditions. Reduction of LDMAR transcript resulted in programmed cell death leading to male sterility due to failed anther development.14 Another rice lncRNA XLOC_057324 has been shown to play a role in panicle development and sexual reproduction.15
Competing endogenous RNAs (ceRNAs) of miRNAs or miRNA target mimics have been shown to function in many biological processes through repression of miRNAs.16,17 Plant ceRNAs can act as ‘sponges’ to sequester miRNAs complementary to ceRNAs resulting in unleashing miRNA targets mimics.18,19 For instance, rice lncRNA XLOC_057324 is able to release more transcripts of the targets of miR160 and miR164 by decoying these two miRNAs.15 A ceRNA network was constructed for rice root and shoot tissues under phosphate starvation conditions.20 However, it is unclear whether lncRNA–miRNAs–target interaction networks are involved in immune response to pathogenic bacteria D. zeae in rice.
Studies on the role of lncRNAs in resistance responses can benefit to understand the rice immune mechanism. The objectives of the present study were to (1) identify and characterize lncRNAs in response to inoculation of D. zeae in a resistant rice variety, and (2) explore the crosstalk network among lncRNAs, miRNAs and their targets.
Materials and methods
Plant materials and growth conditions
All rice plants including Nanjing 40, Nanjing 45, Kasalath and Nipponbare were planted in experimental field under natural normal growth conditions, greenhouse and illumination incubator for bacterial pathogen D. zeae inoculation and RNA extraction in Jiangsu Academy of Agricultural Sciences, Nanjing, Jiangsu Province, China.
Pathogen inoculation
The resistance of rice plants to rice foot rot disease pathogen D. zeae was measured by the basal stem and root inoculation method. The resistance rate was classed as five groups by measuring the percentage of diseased area at 10 days after inoculation. No obvious disease symptom in the stem and foot indicates immunity. The disease index less than or equal to 5 indicates highly resistance. A disease index from 5.1 to 12.4 indicates moderately resistance. A disease index from 12.5 to 19.9 indicates moderately susceptibility. A disease index equals to or greater than 20 indicating highly susceptibility.21
Library construction and sequencing
The resistant japonica rice variety Nanjing 40 was selected for lncRNA library construction and RNA sequencing. Trizol kit (TaKaRa, Dalian, China) was used to extract root total RNAs from two samples, K1 (0 hour post inoculation, hpi) and K2, a mixed sample of 6, 12 and 48 hpi based on three time biological replicates.22 The high-throughput RNA sequencing was performed at the LC-Bio Co., Ltd (Hangzhou, China) following the vendor's recommended protocol. The RNA quality was examined with an absorbance at 260/280 nm by using an Agilent 2100 Bioanalyzer. Approximately 5 μg of total RNA was used to deplete ribosomal RNA according to the manuscript of the Ribo-Zero™ rRNA Removal Kit, including four steps, prepare microspheres, treat sample with rRNA removal solution, remove rRNA and purify rRNA-depleted sample (Illumina, San Diego, USA). After removing ribosomal RNAs, the left RNAs were fragmented into small pieces using divalent cations under high temperature. Then the cleaved RNA fragments were reverse-transcribed to create the cDNA. Single- or dual-index adapters are ligated to the fragments, and size (200–600 bp) selection was performed with agarose gel electrophoresis and the ligated products are amplified by PCR. At last, we performed the 100 bp paired-end sequencing on an Illumina Hiseq 2500 following the recommended protocol.
Identification of lncRNAs and analysis of sequencing data
For identification of candidate lncRNAs, a computational pipeline was developed (Fig. 1). The raw data obtained from the two libraries were analyzed to remove adaptor, low-quality and rRNA reads. The remaining clean reads were aligned to the rice genome (https://plants.ensembl.org/info/website/ftp/index.html) using the TopHat2.23 Then, the mapped reads were utilized in transcript assembly by Cufflinks. According to the annotated rice transcripts (Automated Annotation of the Rice Genome), the known protein-encoding transcripts were removed, and unknown transcripts (>200 and <1500 nucleotides) were defined as candidate lncRNA by using Cuffcompare.23 The remaining transcripts were aligned to miRBase to exclude miRNA precursors. Finally, novel lncRNAs were identified based on their non-protein coding potential (score ≤ −1, means that the transcript has no potential probability for coding proteins) calculated by the Coding Potential Calculator (CPC; http://cpc.cbi.pku.edu.cn), and searching against the Pfam database using the software Hmmer to remove known non-coding RNAs.24,25
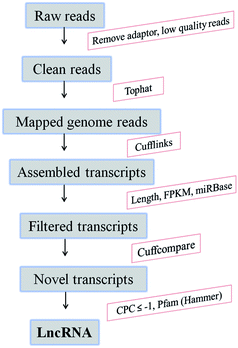 |
| Fig. 1 The computational pipeline for identification of lncRNAs from RNA-seq data in resistant rice. CPC, Coding Potential Calculator. | |
To measure the expression levels of lncRNAs and their targets, Cufflinks 2.0.0 was used to normalize counts of reads based on their lengths. Fragments Per Kilobase of exon model per Million mapped reads (FPKM) represented the normalized expression level of lncRNAs and target genes, and the significance of the expressional difference was tested by using t test. The fold change was measured by the DEGseq package with p values < 0.05 and FDR (False Discovery Rate) < 0.05, fold change >2 or <−2. The heatmap was developed according to the expression abundance of lncRNAs by using the software HemI (Heatmap Illustrator, version 1.0).
To analyze the cis regulation of lncRNAs, the Integrative Genomics Viewer was used to show the genomic loci containing lncRNAs and their adjacent genes. About 100 kb upstream or downstream sequences of lncRNAs were retrieved to search their candidate mRNA targets. Co-expressed lncRNA and target pairs were defined if both were expressed and spaced by less than 100 kb. Furthermore, differentially expressed lncRNAs and mRNAs in response to D. zeae were identified.
Validation of differentially expressed lncRNAs and targets
To test the expression level of lncRNAs and their predicted targets, rice roots after inoculation were collected for extraction of total RNA from two samples, K1 (0 hpi) and K2, a mixed sample of 6, 12 and 48 hpi using the Trizol reagent (TaKaRa, Dalian, China). Quantitative PCR (qPCR) analysis was done using Applied Biosystem 7500 Real Time PCR System and SYBR Premix Ex Taq™ (TaKaRa, Dalian, China) according to the manufacturer's instructions. The first-strand cDNA was synthesized by the First-strand cDNA Synthesis Kit (TaKaRa, Dalian, China) using random hexamer primer (lncRNAs) or oligo dT RTprimer (mRNAs). The EF1-a gene was used as the reference control gene to standardize the RNA sample for evaluating relative expression levels, and all primers used in this study were listed in Table S1.† For qPCR assays, three independent biological samples were carried out, accompanied by three technical replicates.
Results
Response of a resistant japonica rice to D. zeae infection
Hypersensitive response inhibiting the infection and invasion of D. zeae in a resistant rice variety Nanjing 40 was observed whereas three susceptible rice varieties, Nanjing 45, Kasalath and Nipponbare showed typical disease symptoms nearby the site of injection at 10 dpi (Fig. 2A). Moreover, according to the root inoculation experiment, Nanjing 40 was also more resistant to D. zeae than Nanjing 45, which showed withered leaves, black rot symptom and foul smell (Fig. 2B). The results from both two inoculation experiments demonstrated that Nanjing 40 was highly resistance against D. zeae and was thus chosen to analyze resistance mechanism mediated by lncRNA against D. zeae using the high-through sequencing technology.
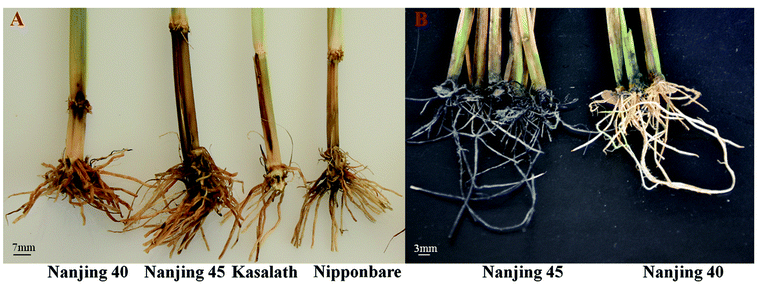 |
| Fig. 2 Resistant and susceptible phenotypes in response to D. zeae infection. (A) and (B) phenotypes observed based on the method of basal stem and root inoculation, respectively. | |
Overview of RNA-seq in an incompatible interaction of rice with D. zeae
In order to understand the molecular mechanisms of rice lncRNAs responsive to D. zeae in resistant rice, the raw reads from RNA-seq data were analyzed respectively, and the raw reads were submitted to NCBI (SRA: SRP149059). 4841 lncRNAs in Nanjing 40 were identified. Among them, 3221 and 2713 transcripts were identified in the infected (K2) and control (K1) sample, respectively (Fig. 3A and Table S2†). The lengths of the detected lncRNAs were in the range from 200 to more than 1500 bp, and 200–300 bp lncRNAs accounted for 60.8% (Fig. 3B). These lncRNAs were evenly distributed on 12 rice chromosomes (Fig. 3C). According to their locations in the genome, 1581, 2138 and 1122 were classified as intronic lncRNAs, lincRNAs (also termed long intergenic noncoding RNAs or intergenic lncRNAs) and antisense lncRNAs. Their distribution on the 12 chromosomes are shown by different concentric rings from outside to inside in Fig. 3D (Fig. S1†).
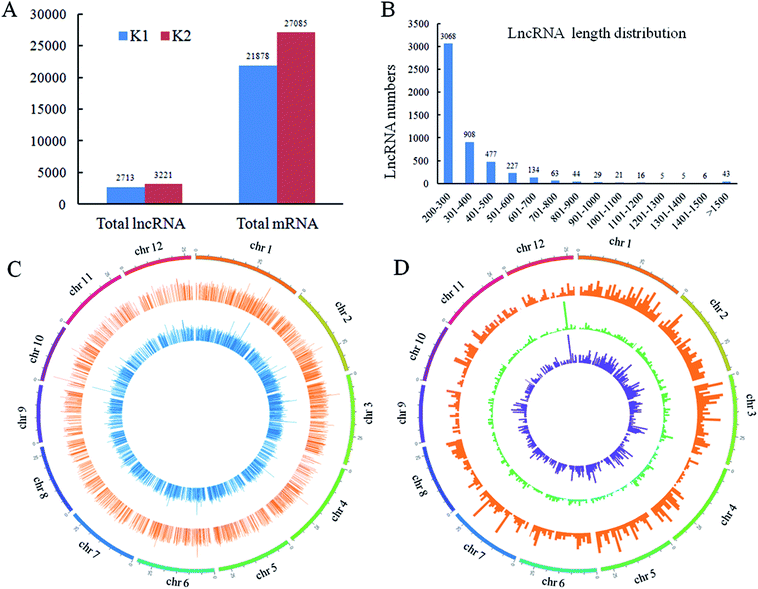 |
| Fig. 3 Results of lncRNA identification. (A) The number of detected lncRNAs and mRNAs. (B) Length distribution of lncRNAs. (C) Genomic distribution of lncRNAs from two libraries. The outside concentric ring represents lncRNAs from K1, the inside concentric ring represents lncRNAs from K2. (D) Distribution of three kinds of lncRNAs. The numbers of intronic lncRNAs (outer orange circle), lincRNAs (middle green circle) and anti-sense lncRNAs (inner blue circle) in physical bins of 500 kb (kilobase) for each chromosome. Intronic lncRNAs are generated from the intron region of protein-coding gene. lincRNAs (long intergenic noncoding RNAs) are transcribed from intergenic regions of the rice genome. Antisense lncRNAs are transcribed from the antisense strand and overlap in part with one or more exons of a protein-coding gene. | |
Identification of D. zeae responsive lncRNAs
A total of 4709 lncRNAs in response to the infection of D. zeae were identified (P-value < 0.05) in roots of K1 sample compared with K2 sample, including 2518 up-regulated lncRNAs and 2191 down-regulated lncRNAs (Fig. 4A, S2 and Table S3†). Additionally, 2123 lncRNAs were uniquely detected in Nanjing 40 after D. zeae inoculation, 1597 lncRNAs were unique to the control, and 989 lncRNAs were detected in both libraries (Fig. 4B and Table S3†). The heatmap in Fig. 4C showed the selected 33 lncRNAs differentially expressed in an incompatible interaction (Fig. 4C and Table S4†). Differential expression of lncRNAs at certain time points after D. zeae infection suggests a role of these lncRNAs in response to D. zeae infection.
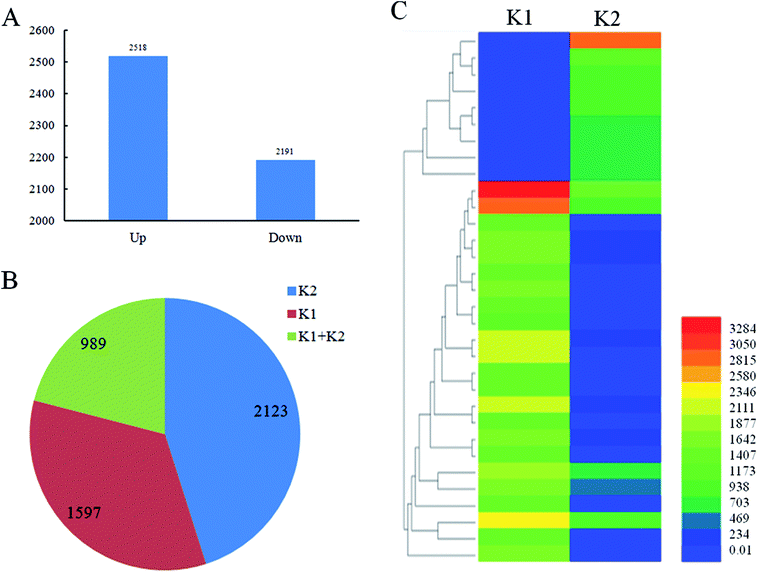 |
| Fig. 4 Identification of lncRNAs responded to the infection of D. zeae in a resistant rice variety. (A) The number of differential expressed lncRNAs in a resistant rice variety after D. zeae inoculation. (B) The lncRNAs responded specifically to the infection by D. zeae in a resistant rice variety. K2 and K1 were the numbers of lncRNAs only detected in a resistant rice variety with and without inoculation. K1 + K2, the number of lncRNAs detected in both libraries. (C) Differentially expressed lncRNAs in two libraries by hierarchical clustering. More than 2 fold change was the cutoff point of the lncRNAs. | |
Co-expression analysis between lncRNAs and their targets in defense pathways
To identify potential mRNA targets of lncRNAs, differential expression was analyzed for the mRNAs within the 100 kb flanking regions of differentially expressed lncRNAs.26 The transcriptome data from RNA-seq identified top ten up-regulated targets involved in multiple signal pathways related to defense responses, such as plant peroxidase, Rab GTPase family 2 (Rab2), alternative oxidase, MATE family protein, MYB family transcription factor, K+ potassium transporter, flavonoid reductase and glutathione S-transferase etc. (Table 1). The top ten down-regulated targets included peroxidase 47, peroxidase A2, two E3 ubiquitin-protein ligases, two DUFs (Table 1). Most of these targets, including peroxidase and E3 ubiquitin-protein ligases, are involved in defense response signal pathways.27 Co-expression analysis of lncRNAs and their flanking mRNAs uncovered that 17 lncRNAs regulated the expression of 17 of 20 targets, except for OS08G0113000, OS05G0110000 and OS02G0101700 (Table 1). Moreover, the interactive networks of 17 lncRNA–target pairs were constructed by the Cytoscape software. Seven of them had networks with more than four nodes, and the remaining ten had networks with three or less nodes (Fig. 5 and S3†). The interactive networks shown in Fig. 5A contained eight target genes, including MYB transcription factor, zinc finger and serine/threonine-protein kinase, which were regulated by three lncRNAs (TCONS_00055600, TCONS_00055598 and TCONS_00055597) in the resistant rice cultivar in response to D. zeae infection. The interactive network shown in Fig. 5B represented three lncRNAs (TCONS_00012775, TCONS_00012782 and TCONS_00012789) regulating six targets, including gene encoding component of the small subunit processome, protein with domains of unknown function (DUFs) and WRKY transcription factor. The targets of TCONS_00080557 and TCONS_00080558 were calcium binding motif-containing protein, phospho-3-sulfolactate synthase, 50S ribosomal protein L24 and peroxidase (Fig. 5C). Moreover, a more complex interactive network was found between six lncRNAs and four targets (Fig. 5D). These four targets were composed of uncharacterized protein (OS02G0317800), alternative oxidase (OS02G0318100), F-box-like protein with seven leucine-rich repeats (LRR; OS02G0317500) and clathrin adaptor (OS02G0317400), which OS02G0318100 and OS02G0317500 may respond to the infection of D. zeae in rice. The remaining 13 interactive networks of lncRNAs–target pairs were shown in Fig. S3.† Targets involved in these networks included plant peroxidase, alternative oxidase, peroxidase, transcription factor, E3 ubiquitin-protein ligase and so on. These lncRNAs–target pairs may have a regulatory role in defense against D. zeae infection.
Table 1 The top ten up- and down-regulated targets in response to D. zeae infection based on RNA-seq
Target |
Gene annotation |
K1 |
K2 |
Regulation |
lncRNA |
OS03G0339400 |
Plant peroxidase |
0 |
60.79 |
Up |
TCONS_00054645 |
OS04G0470100 |
Rab GTPase family 2 (Rab2) |
0.12 |
30.88 |
Up |
TCONS_00064813 |
OS02G0318100 |
Alternative oxidase |
1.04 |
197.93 |
Up |
TCONS_00043825 |
OS08G0480000 |
MATE family |
4.85 |
39.36 |
Up |
TCONS_00096879 |
OS03G0764600 |
MYB transcription factor |
8.01 |
297.03 |
Up |
TCONS_00055598 |
OS08G0536300 |
B-box-type zinc finger protein |
9.76 |
63.87 |
Up |
TCONS_00096627 |
OS03G0576200 |
K+ potassium transporter |
10.08 |
72.83 |
Up |
TCONS_00054751 |
OS02G0811800 |
Flavonoid reductase |
11.94 |
720.39 |
Up |
TCONS_00043541 |
OS12G0207500 |
ATP synthase |
32.99 |
143.67 |
Up |
TCONS_00032691 |
OS01G0369700 |
Glutathione S-transferase |
33.09 |
506.35 |
Up |
TCONS_00011946 |
OS08G0113000 |
Peroxidase 47 |
272.01 |
41.767 |
Down |
|
OS03G0235000 |
Peroxidase A2 |
250.03 |
43.23 |
Down |
TCONS_00054545 |
OS03G0741100 |
Transcription factor BHLH148 |
223.55 |
37.54 |
Down |
TCONS_00053343 |
OS05G0110000 |
E3 ubiquitin-protein ligase |
193.24 |
30.53 |
Down |
|
OS04G0637000 |
Basic leucine zipper protein |
184.53 |
20.63 |
Down |
TCONS_00064963 |
OS02G0101700 |
E3 ubiquitin-protein ligase |
101.94 |
21.5 |
Down |
|
OS02G0669500 |
DUF241 |
174.27 |
26.7 |
Down |
TCONS_00044026 |
OS01G0585600 |
DUF632 |
93.26 |
38.75 |
Down |
TCONS_00012775 |
OS06G0683400 |
Calcium-binding protein |
124.57 |
7.53 |
Down |
TCONS_00080557 |
OS02G0787800 |
Kinase 7 |
113.66 |
26.8 |
Down |
TCONS_00037675 |
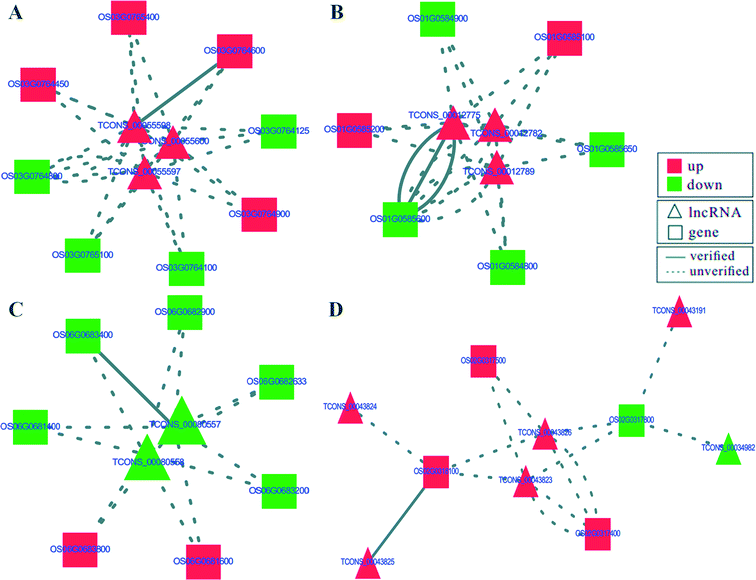 |
| Fig. 5 The predicted interaction networks of four lncRNAs–target pairs. (A)–(D) show the interaction networks of different lncRNA–target pairs. The triangular and rectangular nodes represent lncRNAs and relevant targets, respectively. Red and green represent the up-regulated and down-regulated nodes, respectively. Lines show the interaction of the lncRNAs–target pairs. The solid line and break line represent verified and unverified lncRNA–target pairs, respectively in Table 1. | |
Crosstalk of the lncRNA–miRNA network in an incompatible interaction
Previously, two miRNAs, osa-miR396 and osa-miR156, have been shown to be involved in the rice defense responses respectively.28,29 The lncRNAs acting as potential ceRNAs mimics of both miR396 and miR156 were predicted and their expressional changes were analyzed using the lncRNA sequencing data (Table 2). Three lncRNAs, TCONS_00042166, TCONS_00064123 and TCONS_00081640, were predicted to be ceRNAs mimics of osa-miR396 (Table 2). Six lncRNAs, TCONS_00012844, TCONS_00043299, TCONS_00102384, TCONS_00102574, TCONS_00072934 and TCONS_00013133, were predicted ceRNAs of osa-miR156 (Table 2). It is noticeable, some mRNA genes were presumably the targets of miRNAs osa-miR156 and osa-miR396 (Table 2), predicted to be as ubiquitin-protein ligase, vacuolar-sorting receptor protein, esterase protein and transcription factors involved in defense responses (Table 2) suggesting that lncRNA may regulate miRNAs in resisting D. zeae in an incompatible interaction.
Table 2 Expression profile of lncRNAs acting as ceRNAs in response to D. zeae infection in resistant rice
miR_name |
ceRNA |
Predicted target |
Gene annotation |
ceRNA |
K1 |
K2 |
osa-miR156 |
TCONS_00012844 |
OS01G0633100 |
Glucose pyrophosphorylase |
0 |
60 |
osa-miR156 |
TCONS_00043299 |
OS02G0557300 |
RING-H2 finger protein |
0 |
81 |
osa-miR156 |
TCONS_00102384 |
OS09G0376800 |
Glycosyltransferase |
1980 |
778 |
osa-miR156 |
TCONS_00102574 |
OS09G0252800 |
Ubiquitin-protein ligase |
267 |
163 |
osa-miR156 |
TCONS_00072934 |
OS05G0363100 |
Esterase protein |
460 |
20 |
osa-miR156 |
TCONS_00013133 |
OS01G0944100 |
Vacuolar-sorting receptor |
0 |
28 |
osa-miR396 |
TCONS_00042166 |
OS02G0742100 |
DNA binding protein |
1096 |
481 |
osa-miR396 |
TCONS_00064123 |
OS04G0112200 |
Transcription factor E2FB |
0 |
134 |
osa-miR396 |
TCONS_00081640 |
OS06G0503400 |
Reticulon-like protein B2 |
0 |
193 |
Validation of D. zeae responsive lncRNAs
A total of 17 differentially expressed lncRNAs were randomly chosen for qPCR analysis. Among the 17 lncRNAs, 13 had their number of normalized reads significantly up-regulated, and four significantly down-regulated (Table 3). For example, the number of normalized reads of lncRNA TCONS_00054751 in the infected and control samples were 845.71 and 0, respectively (Table 3), representing the highest induced lncRNA. The qPCR analysis results confirmed the expression changes of 17 lncRNAs, although three lncRNAs (TCONS_00064963, TCONS_00044026 and TCONS_00012775) showed an opposite result (Fig. 6).
Table 3 The 17 differentially expressed lncRNAs based on the normalized data and randomly chosen from RNA-seq data
lncRNA |
K1 |
K2 |
Regulation |
TCONS_00043825 |
120.87 |
980.18 |
Up |
TCONS_00032691 |
0 |
168.89 |
Up |
TCONS_00064597 |
0 |
139.36 |
Up |
TCONS_00054751 |
0 |
845.71 |
Up |
TCONS_00096627 |
0 |
104.63 |
Up |
TCONS_00054645 |
0 |
138.92 |
Up |
TCONS_00054417 |
0 |
97.72 |
Up |
TCONS_00096879 |
0 |
142.38 |
Up |
TCONS_00081726 |
0 |
147.87 |
Up |
TCONS_00064813 |
0 |
99.03 |
Up |
TCONS_00064963 |
0 |
83.99 |
Up |
TCONS_00044026 |
0 |
63.73 |
Up |
TCONS_00012775 |
0 |
77.49 |
Up |
TCONS_00054545 |
1621.13 |
195.11 |
Down |
TCONS_00053343 |
1020.79 |
17.03 |
Down |
TCONS_00037675 |
274.84 |
0 |
Down |
TCONS_00080557 |
620.79 |
0 |
Down |
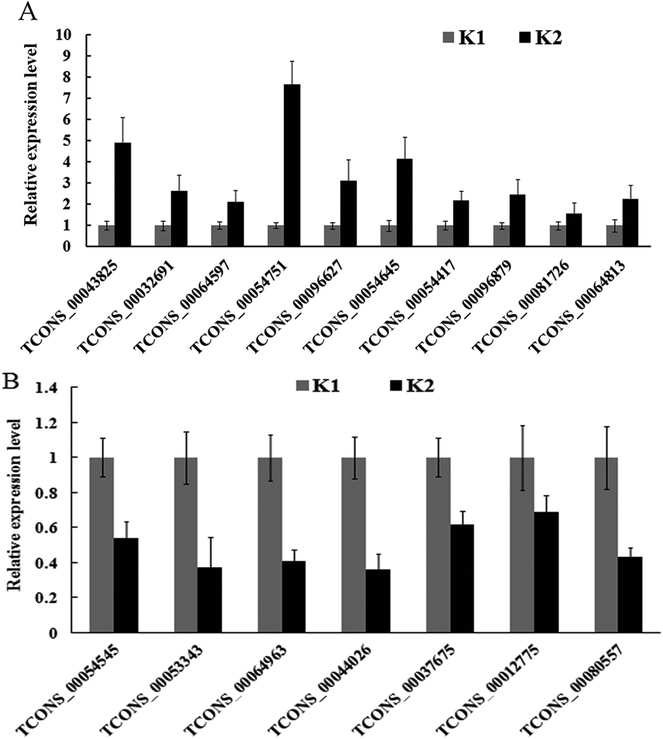 |
| Fig. 6 lncRNAs from RNA-seq verified by qPCR. (A) and (B) the expression level of ten up-regulated and seven down-regulated lncRNAs were validated by qPCR, respectively. Data represent means of three replicates ± standard deviation for each target in the two libraries. | |
Ten targets (five up-regulated and five down-regulated genes in response to D. zeae infection) were randomly selected for confirmation of their differential expression pattern using qPCR (Fig. 7). For all ten genes, their expression patterns were confirmed despite the degree of changes varied between RNA-seq (Fig. 7A) and qPCR (Fig. 7B and C).
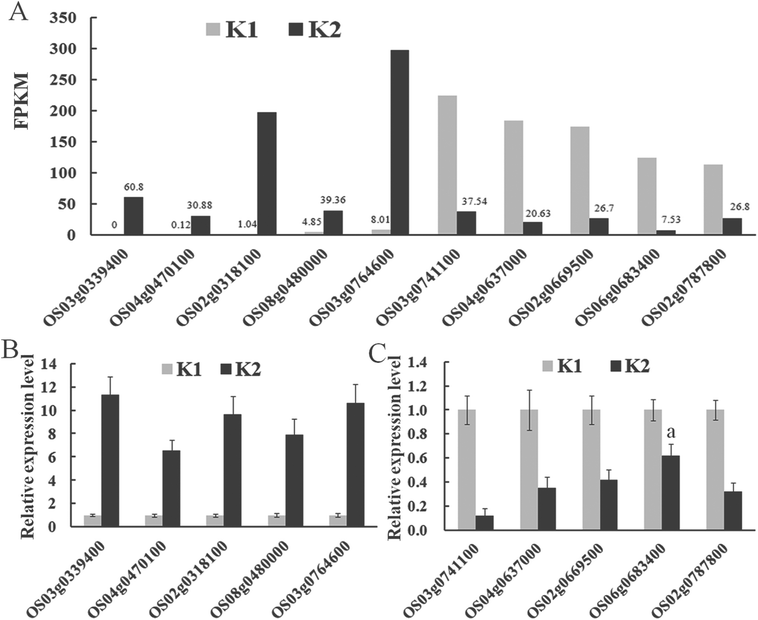 |
| Fig. 7 Expression levels of the ten targets selected for verification by qPCR. (A) Ten significantly differentially expressed target genes based on the number of normalized reads in an incompatible interaction. FPKM (Fragments Per Kilobase of exon model per Million mapped reads) represents the normalized expression level of target genes. (B) and (C) The expression level of five up- and down-regulated targets analyzed by qPCR, respectively. Data represent means of three replicates ± standard deviation for each target in the two libraries in an incompatible interaction. a indicates that a significant difference (P < 0.05) was detected between K1 and K2. | |
Discussion
Rice foot rot disease caused by pathogen D. zeae is one of the most destructive bacterial diseases.1 lncRNAs were involved in the regulation of target gene expression at epigenetic, transcriptional and post-transcriptional levels in rice.16 It was predicted that lncRNA plays a role in a wide range of biological processes including growth and development and stress responses in plants.14,15 In this study RNA-seq was performed to identify the putative rice lncRNAs in response to D. zeae infection in Nanjing 40.
lncRNAs are thought to be involved in complex interaction with target genes and miRNAs.19 lncRNAs may function as ceRNA to bind specific miRNAs to prevent degradation of relevant target mRNAs by miRNAs.18 To a great degree, the biological function of miRNAs partly reflected the biological function of lncRNAs as ceRNAs.19 The Plant ceRNA database (PceRBase, http://bis.zju.edu.cn/pcernadb/index.jsp) contains potential ceRNAs, and ceRNA target-mimic pairs from 26 plant species.17 lncRNAs potentially acting as ceRNAs of two key miRNAs, osa-miR156 and osa-miR396, were deduced and characterized in this study (Table 2). These two miRNAs regulate not only growth and development but also response to pathogen infection in plants.29 Particularly, a recent study showed that rice blast infection significantly induced the accumulation of osa-miR156 and osa-miR396.29 In this study, we identified that ubiquitin-protein ligase and glycosyltransferase are two targets of osa-miR156 (Table 2). Previous study demonstrated that ubiquitin ligase-associated protein SGT1 is required for resistance responses to diverse phytopathogens in Nicotiana benthamiana.30 Recent study showed that E3 ubiquitin ligase EBR1 interacted with target OsBAG4 in triggering programmed cell death and activating innate immunity and resistance to both blast and Xoo in rice.27 Moreover, glycosyltransferase plays a key role in regulating the composition of plant cell wall resulted in increased diseases resistance.31,32 Overexpression of miR396a or miR396b in Arabidopsis significantly contributed resistance to cyst nematode.31 We also identified that reticulon-like protein B2 and transcription factor E2FB-like were the predicted two targets of osa-miR396 (Table 2). The reticulon-like protein B2 gene was clearly induced by the fungal pathogen Fusarium oxysporum in chickpea.33 The E2FB transcription factor in Arabidopsis may be involved in the bacterial disease resistance by regulating the auxin signal pathway.33,34 Another predicted target of osa-miR396 was the growth-regulate factor OsGRF, which is involved in multiple resistance-related signal pathways such as cell-wall modification, cytokinin biosynthesis and the accumulation of secondary metabolites in Arabidopsis.31,32
The reactive oxygen species (ROS) burst was a key defense responses signal molecule conferring the broad-spectrum resistance to fungal and bacterial diseases in rice.35 For example, rapid accumulation of ROS resulting from the inhibition of the expression of peroxidase gene in bsr-d1 knockout plants conferred a broad-spectrum resistance to rice blast fungus.35 We showed that the expression levels of the targets of TCONS_00055598 (OS03G0764600, a MYB transcription factor) and TCONS_00054545 (OS03G0235000, peroxidase A2), were significantly up- and down-regulated, respectively (Table 1 and Fig. 7). In addition, plant peroxidase (OS03G0339400) and alternative oxidase (OS02G0318100), targets of TCONS_00054645 and TCONS_00043825, respectively, were increased in the incompatible interaction (Table 1 and Fig. 7). During an incompatible interaction, increase of peroxidase suggests that lncRNAs may regulate ROS during defense against D. zeae. Consistent with the observation that ROS balance mechanism can be used to prevent the damage resulting from massive ROS burst induced by pathogen infection.36
In this study, we identified and characterized lncRNAs in response to D. zeae infection in a resistant rice variety using RNA-seq. A total of 4709 lncRNAs, 2518 up-regulated and 2191 down-regulated, were detected. The RNA-seq based expression levels of selected lncRNAs and their targets were validated by qPCR. Some lncRNAs were predicted to be target mimics of miRNAs. These results in this study provide evidences for better understanding the role of lncRNAs in regulating resistance to the bacterial rice foot rot disease in rice, as well as show a potential approach for cultivating the resistant rice to foot rot disease by generating lncRNA transgenic plants in the future.
Conflicts of interest
All authors declare that there are no conflicts of interest.
Acknowledgements
We thank Dr Lei Liu of Genepioneer Biotechnologies for his help of bioinformatics analysis. This work was supported by the National Key Research and Development Program (No. 2017YFD0100400-3), the Jiangsu Key Laboratory of Agrobiology Program (No. 49114042015Z006), the Jiangsu Province Key Research and Development Program (Modern Agriculture) Project (No. BE2015355), the Exploratory Project of the Jiangsu Academy of Agricultural Sciences (No. ZX(17)2014), and the Jiangsu Province Natural Science Foundation (No. BK20171326). The United States Department of Agriculture (USDA) is an equal opportunity provider and employer.
References
- Q. G. Liu, Q. Zhang and C. D. Wei, Sci. Agric. Sin., 2013, 46, 2923–2931 CAS.
- M. Goto, Phytopathology, 1979, 69, 213–216 CrossRef.
- J. N. Zhou, H. B. Zhang, J. Wu, Q. G. Liu, P. G. Xi, J. Lee, J. L. Liao, Z. D. Jiang and L. H. Zhang, Mol. Plant-Microbe Interact., 2011, 24, 1156–1164 CrossRef CAS PubMed.
- J. Y. Liu, J. H. Rice, N. N. Chen, T. J. Baum and T. Hewezi, PLoS One, 2014b, 9, e98477 Search PubMed.
- L. Yang and H. Huang, J. Integr. Plant Biol., 2014, 56, 962–970 CrossRef CAS PubMed.
- J. A. Chekanova, Curr. Opin. Plant Biol., 2015, 27, 207–216 CrossRef CAS PubMed.
- X. Liu, L. L. Hao, D. Y. Li, L. H. Zhu and S. N. Hu, Genomics, Proteomics Bioinf., 2015, 13, 137–147 CrossRef PubMed.
- H. Wang, Q. W. Niu, H. W. Wu, J. Liu, J. Ye, N. Yu and N. H. Chua, Plant J., 2015, 84, 404–416 CrossRef CAS PubMed.
- J. Mach, Plant Cell, 2017, 29, 916 CrossRef CAS PubMed.
- Q. H. Zhu, S. Stephen, J. Taylor, C. A. Helliwell and M. B. Wang, New Phytol., 2014, 201, 574–584 CrossRef CAS PubMed.
- H. Zhang, W. G. Hu, J. L. Hao, S. K. Lv, C. Y. Wang, W. Tong, Y. J. Wang, Y. Z. Wang, X. L. Liu and W. Q. Ji, BMC Genomics, 2016, 17, 238 CrossRef PubMed.
- P. J. Chung, H. Jung, D. H. Jeong, S. H. Ha, Y. D. Choi and J. K. Kim, BMC Genomics, 2016, 17, 563 CrossRef PubMed.
- D. D. Niu, X. Zhang, X. O. Song, Z. H. Wang, Y. Q. Li, L. L. Qiao, Z. Y. Wang, J. Z. Liu, Y. W. Deng, Z. H. He, D. L. Yang, R. Y. Liu, Y. L. Wang and H. W. Zhao, Phytopathology, 2018, 108, 60–69 CrossRef PubMed.
- J. H. Ding, Q. Lu, Y. D. Ouyang, H. L. Mao, P. B. Zhang, J. L. Yao, C. G. Xu, X. H. Li, J. H. Xiao and Q. F. Zhang, Proc. Natl. Acad. Sci. U. S. A., 2012, 109, 2654–2659 CrossRef CAS PubMed.
- Y. C. Zhang, J. Y. Liao, Z. Y. Li, Y. Yu, J. P. Zhang, Q. F. Li, L. H. Qu, W. S. Shu and Y. Q. Chen, Genome Biol., 2014, 15, 512 CrossRef PubMed.
- Q. H. Zhu and M. B. Wang, Genes, 2012, 3, 176–190 CrossRef PubMed.
- C. H. Yuan, X. W. Meng, X. Li, N. Illing, A. R. Ingle, J. J. Wang and M. Chen, Nucleic Acids Res., 2017, 45(D1), D1009–D1014 CrossRef CAS PubMed.
- H. J. Wu, Z. M. Wang, M. Wang and X. J. Wang, Plant Physiol., 2013, 161, 1875–1884 CrossRef CAS PubMed.
- Y. Tay, J. Rinn and P. P. Pando, Nature, 2014, 505, 344–352 CrossRef CAS PubMed.
- X. W. Xu, X. H. Zhou, R. R. Wang, W. L. Peng, Y. An and L. L. Chen, Sci. Rep., 2016, 6, 20715 CrossRef CAS PubMed.
- W. Q. Li, J. Yang, J. Wang, F. F. Fan, J. Y. Zhu, F. Q. Wang and W. G. Zhong, Jiangsu Agricultural Sciences, 2014, 42, 139–142 Search PubMed.
- B. A. Besma, W. Sonia, M. Francisco, L. Philippe, d'A. C. Yves, H. Judith, M. Alexis, M. Allison, L. Antoine, M. D. Jean, V. Herve, T. Claude and C. Martin, Genome Res., 2009, 19, 57–69 Search PubMed.
- D. Kim, G. Pertea, C. Trapnell, H. Pimentel, R. Kelley and S. L. Salzberg, Genome Biol., 2013, 14, R36 CrossRef PubMed.
- L. Kong, Y. Zhang, Z. Q. Ye, X. Q. Liu, S. Q. Zhao, L. P. Wei and G. Gao, Nucleic Acids Res., 2007, 35, W345–W349 CrossRef PubMed.
- Y. N. Pang, C. B. Mao and S. R. Liu, Theranostics, 2018, 8, 2496–2507 CrossRef PubMed.
- X. D. Hou, Y. M. Du, X. M. Liu, H. B. Zhang, Y. H. Liu, N. Yan and Z. F. Zhang, Int. J. Mol. Sci., 2008, 19, 101 Search PubMed.
- Q. Y. You, K. R. Zhai, D. L. Yang, W. B. Yang, J. N. Wu, J. Z. Liu, W. B. Pan, J. J. Wang, X. D. Zhu, Y. K. Jian, J. Y. Liu, Y. Y. Zhang, Y. W. Deng, Q. Li, Y. G. Lou, Q. Xie and Z. H. He, Cell Host Microbe, 2016, 20, 758–769 CrossRef CAS PubMed.
- T. Hewezi, T. R. Maier, D. Nettleton and T. J. Baum, Plant Physiol., 2012, 159, 321–335 CrossRef CAS PubMed.
- Z. Y. Li, J. Xia, Z. Chen, Y. Yu, Q. F. Li, Y. C. Zhang, J. P. Zhang, C. Y. Wang, X. Y. Zhu, W. Zhang and Y. Q. Chen, Sci. Rep., 2016, 6, 25493 CrossRef CAS PubMed.
- J. R. Peart, R. Lu, A. Sadanandom, I. Malcuit, P. Moffett, D. C. Brice, L. Schauser, D. A. W. Jaggard, S. Y. Xiao, M. J. Coleman, M. Dow, J. D. G. Jones, K. Shirasu and D. C. Baulcombe, Proc. Natl. Acad. Sci. U. S. A., 2002, 99, 10865–10869 CrossRef CAS PubMed.
- C. Hernández-Blanco, D. X. Fen, J. Hu, A. Sánchez-Vallet, L. Deslandes, F. Llorente, M. Berrocal-Lobo, H. Keller, X. Barlet, C. Sánchez-Rodríguez, L. K. Anderson, S. Somerville, Y. Marco and A. Molina, Plant Cell, 2007, 19, 890–903 CrossRef PubMed.
- H. J. Park, C. S. Kwon, J. Y. Woo, G. J. Lee, Y. J. Kim and K. H. Paek, Plant Pathol. J., 2011, 27, 170–182 CrossRef CAS.
- M. L. Upasani, B. M. Limaye, G. S. Gurjar, S. M. Kasibhatla, R. R. Joshi, N. Y. Kadoo and V. S. Gupta, Sci. Rep., 2017, 7, 7746 CrossRef PubMed.
- Z. Magyar, L. D. Veylder, A. Atanassova, L. Bakó, D. Inzé and L. Bögre, Plant Cell, 2005, 17, 2527–2541 CrossRef CAS PubMed.
- W. T. Li, Z. W. Zhu, M. S. Chern, J. J. Yin, C. Yang, L. Ran, M. P. Cheng, M. He, K. Wang, J. Wang, X. G. Zhou, X. B. Zhu, Z. X. Chen, J. C. Wang, W. Zhao, B. T. Ma, P. Qin, W. L. Chen, Y. P. Wang, J. L. Liu, W. M. Wang, X. J. Wu, P. Li, J. R. Wang, L. H. Zhu, S. G. Li and X. W. Chen, Cell, 2017, 170, 114–126 CrossRef CAS PubMed.
- W. Q. Li, M. Shao, W. G. Zhong, J. Yang, K. Okada, H. Yamane, L. Zhang, G. Wang, D. Wang, S. S. Xiao, S. S. Chang, G. L. Qian and F. Q. Liu, PLoS One, 2012, 7, e43914 CrossRef CAS PubMed.
Footnote |
† Electronic supplementary information (ESI) available. See DOI: 10.1039/c8ra04993a |
|
This journal is © The Royal Society of Chemistry 2018 |
Click here to see how this site uses Cookies. View our privacy policy here.