DOI:
10.1039/C8RA04886J
(Paper)
RSC Adv., 2018,
8, 30061-30070
Network pharmacology combined with functional metabolomics discover bile acid metabolism as a promising target for mirabilite against colorectal cancer†
Received
8th June 2018
, Accepted 1st August 2018
First published on 24th August 2018
Abstract
In this study, a combination of network pharmacology and metabolomics was used to explore the mechanism by which mirabilite regulates bile acid metabolism in the treatment of colorectal cancer. The PharmMapper web server was applied to make preliminary predictions for the treatment targets of mirabilite and to predict the interaction between mirabilite and disease targets using Discovery Studio 2.5. Furthermore, the urine metabolic profile was analyzed by the UPLC-Q-TOF-MS technology. The original data were processed by Progenesis QI software and analyzed by multivariate pattern recognition, which allowed us to reveal the metabolic disturbance in colorectal cancer and explain the therapeutic effect of mirabilite. The network pharmacology results showed that mirabilite can act on the disease targets, and the sites of action include amino acid residues Arg-364 and Asp-533, as well as nucleotides TPC-11, DG-112 and DA-113. Based on metabolomics, potential biomarkers were found to lie in the relevant pathways of bile acid metabolism, such as taurine, chenodeoxycholic acid, cholic acid, and deoxycholic acid. The results showed that mirabilite could regulate the distribution of overall metabolic disturbance, and bile acid metabolism was the main targeted pathway. Additionally, we predicted the upstream targets by ingenuity pathway analysis and found that mirabilite played a significant role in regulating the bile acid-related biomarkers, which allowed comprehensive analysis of the effect of mirabilite on colorectal cancer. This study fully explained the role of mirabilite in inhibiting colorectal cancer, which mainly occurs through bile acid metabolism, via the approach of network pharmacology combined with functional metabolomics.
Introduction
Colorectal cancer (CRC), a high-mortality disease, is the third most common cancer worldwide and has an average annual incidence of more than 1.2 million new cases.1 Approximately 5% of CRC cases originate from gene mutation, such as adenomatous polyposis coli (APC),2 tumor suppressor gene 53 (p53),3 KRAS proto-oncogene (KRAS),4 B-Raf proto-oncogene (BRAF),5 serine/threonine kinase SMAD family member 4 (SMAD4)6 etc. For example, the APC mutation can cause familial adenomatous polyposis (FAP), an inheritable condition. In addition, unsuitable diets, obesity and alcohol are three other tumorigenic factors.7,8 Prevention of CRC also requires effective containment of inflammatory bowel disease (IBD), ulcerative colitis (UC) and Crohn's disease.9–11 The occurrence of CRC is usually insidious, with a high fatality rate. To improve the therapeutic outcomes, we need to fully understand the mechanism of the disease to find new drug targets and develop new therapeutic protocols to improve the survival rate. Traditional medicine is widely used in clinic and plays an important role in disease prevention and treatment. Mirabilite, one of a number of purging drugs in traditional Chinese medicine (TCM), is usually applied as an enema to purge heat, moisten dryness, and for softening and hardening tissue. Mirabilite can reportedly be used to assist in the treatment of gastrointestinal diseases such as acute peritonitis, pancreatitis and abdominal pain etc.12 At present, there is no report on the antitumor effect and mechanism of mirabilite.
Metabolomics plays an important role in the pathogenesis and early diagnosis of diseases such as IBD and Crohn's disease.13 Bile acids (BAs), a class of small molecular metabolites, have a variety of physiological functions, such as raising intestinal absorption, emulsification, and transport of nutrients, lipids and lipophilic vitamins.14 Secondary bile acid is synthesized in the intestine by the gut microbiota and promotes carcinogenesis of CRC,15,16 which suggests that the composition of the bile acid pool is related to CRC.17 The bile acid homeostasis is co-maintained by the synthesis of bile acid in the liver, secretion from bile ducts, microbial metabolism and intestinal reabsorption into the blood.18 Metabolomic technology, such as LC-MS and UPLC-MS, can intuitively visualize the changes of bile acids that are disturbed by CRC, which can be used to discover the key biomarkers affected by CRC and also to assess the therapeutic effect of different medicines on this disease. Network pharmacology is a new method and strategy for drug design based on system biology and multidirectional pharmacology. It predicts the targets of drugs, components, and diseases by considering the whole system.19,20 Network pharmacology, a key technique for discovering the interlocking of drugs and disease, can also analyze the target's corresponding pathways by bioinformatics.21,22 Metabolomics is the study of the overall effects of cellular or bio-fluids, metabolite changes and functional regulation of tissues as a whole system.23 The two research methods are consistent with the concept of “overall efficacy” in the TCM theory.
In this study, we used network pharmacology to predict the possible targets and active pathways of mirabilite. Then, APCmin/+ mice were used as the research object to investigate the therapeutic effect of mirabilite on a model of spontaneous occurrence of intestinal tumors by UPLC-Q-TOF-MS and multivariate statistical analysis. Finally, network pharmacology and metabolomics technology were combined to predict and visualize the mechanism of mirabilite.
Materials and methods
Methods of network pharmacology
Network pharmacology was used to predict the efficacy of mirabilite. The chosen servers for this purpose included Target bank, Drugbank and the TCM pharmaceutical prediction databases, including the Traditional Chinese Medicine Systems Pharmacology Database and Analysis Platform (TCMSP) and Traditional Chinese Medicine Integrated Database (TCMID). The study also used PharmMapper, a free web server for reverse pharmacophore matching developed in 2010. PharmMapper, which is based on the feature target prediction of ligands, has more than 7000 pharmacophore models, and is associated with 1627 drug target datapoints. It can automatically find and score the most matching conformations of the molecular conformation and cover 110 kinds of clinical indications, which has fast operation speed and comprehensive target information. As well as the advantage of fast computing speed it also offers comprehensive target information. ChemDraw was used to draw the spatial structure of the main compound, mirabilite (Na2SO4·10H2O), which was submitted to the above public databases for retrieval of the prediction of active sites. The entry on “colorectal cancer” in the therapeutic targets database (TTD) was imported to screen the active sites of the disease. The results of the two procedures mentioned above were used to find cross targets. Finally, the Discovery Studio 3.0 software was used to complete the molecular docking.
Chemicals and reagents
Both acetonitrile and methanol were obtained from Thermo Fisher Scientific (USA) and were of high performance liquid chromatography grade. Ultrapure water was self-produced by using a Milli-Q system (Millipore, Bedford, MA, USA). Mirabilite was produced by Tong Ren Tang Drug Store (Harbin, China). Farnesoid X receptor (FXR), an immunohistochemistry reagent, was purchased from Enogene Biotech (100 μg, Nanjing, China).
Animals
10 APCmin/+ mice and 5 C57BL/6J mice were purchased by the Nanjing Biomedical Research Institute of Nanjing University and their weight was (20 ± 2) g. The animals were coded with the number N000132. Standardized feed was provided for all the mice. The temperature was 24 ± 2 °C and the range of humidity was 65–75%. All mice were free to feed and drink water. According to their weight, the APCmin/+ mice were randomly divided into the model group and mirabilite group. The mirabilite group was administrated with 0.01 mL g−1 every day for 70 days from the age of 9 weeks old. The mice in the control group and the model group were given the same volume of distilled water daily. The experimental procedures were approved by the Animal Care and Ethics Committee at Heilongjiang University of Chinese Medicine and all experiments were performed in accordance with the Declaration of Helsinki.
Urine collection and preparation
The urine samples were gathered between 5 p.m. and 8 a.m. (the next day) for each mouse and collected every two days. Each urine sample was collected in a 2.0 mL Eppendorf tube. It was then placed in a centrifuge and centrifuged for 10 min (10
000 rpm, 4 °C), which removed impurities. The supernatant was transferred to a 1.5 mL tube and stored at −80 °C. Before analysis, the urine samples were thawed in an icy water bath at room temperature. Next, each urine sample was centrifuged for 10 min at 10
000 rpm (4 °C). Then, 100 μL urine was transferred into another tube and 900 μL double distilled water was added, and the self-made mixture was blended for 30 s and filtered through a 0.45 μm filter. Finally, the filtrate was transferred into a sample cup.
Chromatography
The reverse chromatographic column for the separation of the urine metabolic profile was an ACQUITY UPLCTM C18 column (Waters, USA, 1.7 μm, 100 mm × 2.1 μm i.d.) on an ultra-high-performance liquid chromatography (UPLC) system (Waters Corp., Milford, MA, USA). The temperature of the column was kept at 40 °C. The flow rate was set at 0.4 mL min−1. The sample injection volume was 4 μL. Solvent A was acetonitrile mixed with 0.1% formic acid, and solvent B was water mixed with 0.1% formic acid. The gradient elution program was: 0 min ∼99% B, 0–1 min ∼87% B, 1–2.5 min ∼70% B, 2.5–5 min ∼1% B, 5–7 min ∼99% B. When delta < 20 psi, we began collecting the chromatogram.
Mass spectrometry
An electrospray ionization (ESI) source was used in the mass spectrometry system (high-resolution quadrupole mass spectrometer). The flow and temperature of the desolvation gas were 600 L h−1 and 350 °C, respectively. The flow rate of the cone backflush gas was 50 L h−1. The source temperature was 2.5 °C. The sampling cone was 30 and the source offset was 80. The nebuliser gas flow was 6.5 bar. The ion source temperature was 110 °C and the voltage was set at 30 V. The capillary voltage was 3 kV. Online water quality correction was performed using a Locksray calibration system (Waters Corporation, USA). The calibrated substance was leucine-enkephalin ([M + H]+ = 556.2771, [M − H]− = 554.2771). The mass scan range was 50–1000 Da, scanning in steps of 0.2 s in the centroid mode.
Data processing and analysis
Based on the established methods of preparation and collection of urine samples, the total scanning of positive and negative ion patterns was performed on the 0th to 70th day, at intervals of one week. Then, the metabolic profiling of urine samples from the 0th day to 70th day in the experiment was imported into the Progenesis QI software and a between-subject design was used to allocate the information of each group, in which the peak picking and normalization of chromatography peaks was processed. Then, principal component analysis (PCA) and orthogonal partial least squares-discriminant analysis (OPLS-DA) were performed to classify the different groups in terms of score plot and S-plot. Next, the mass spectrums of the two fragments were collected in MS/MS mode. These were compared with the fragment information in databases such as Human Metabolome Database (HMDB), Metabolite and Tandem MS Database (MetLin), Chemspider, and Kyoto Encyclopedia of Genes and Genomes (KEGG), to identify biomarkers. The data processing was carried out three times.
Pathway analysis and prediction
MetaboAnalyst 4.0 (https://www.metaboanalyst.ca/) is a powerful tool for metabolomics data analysis. This platform currently has nearly 1600 pathways and visualizations for many model organisms, including human, mouse, rat, cow, chicken, zebrafish, malaria, budding yeast, etc. The module of ‘Metabolic pathway analysis’ was applied in the present study to explore the associated metabolic pathways for all the differentiated metabolites.
Ingenuity pathway analysis (IPA) is an integrated bio-path analysis software based on cloud computing. IPA can give key regulatory information for metabolite research, and enables a better understanding of metabolomic data from cell morphology to metabolic mechanism.
Histopathological examination
The collected fresh bowel tissues were received by the Department of Pathology in the Affiliated Hospital of Heilongjiang University of Chinese Medicine, which provided us with the histopathological results of each group.
Results
Potential therapeutic target prediction through reverse docking
The TCMSP and TCMID databases had no results for mirabilite. Therefore, the file of the structure was instead uploaded to the “PharmMapper” database. Then, the predicted target receptors of mirabilite were presented online. We chose the targets with fit scores >2.5, which included SKP1-like protein 1A, probable RNA 2-phosphotransferase, NADH pyrophosphatase, DNA topoisomerase I, dihydro-pyrimidine dehydrogenase [NADP+] and histidinol dehydrogenase as the possible targets of mirabilite. Furthermore, we downloaded the structure of the predicted targets from the Protein Data Bank (PDB) database, which is the main repository of protein structure information.
The therapeutic targets for the disease CRC
We collected the CRC-related therapeutic targets mainly from two sources: one was public network databases, such as TTD, and the other was the published literature. The therapeutic targets included several already-known successful ones, such as DNA topoisomerase I (Top I), vitamin D receptor, serine/threonine protein kinase MEK1 (MEK1), mitogen-activated protein kinase kinase 2 (MEK2), epidermal growth factor receptor (EGFR), vascular endothelial growth factor precursor (VEGF), etc., which had been confirmed and had corresponding therapeutic drugs. In contrast, some targets did not have corresponding therapeutic drugs for CRC, such as insulin-like growth factor II, histone deacetylase 4 and serine/threonine-protein kinase 6, and these also had no diagnosis targets. To further focus on the target of therapy, we also found drug targets in the TCMSP. According to the DrugBank, some of the above therapeutic targets have been applied in the clinic or in clinical trials. For example, Top I was found to be the inhibition site of irinotecan, and was also a common target shared between therapeutic targets and disease targets.
Mechanism prediction by molecular docking
Based on the results of predicted targets and therapeutic targets, we chose the common targets between mirabilite and CRC. Moreover, we carried out molecular docking and target prediction through spatial and energy matching. We used the Discovery Studio 2.5 software to analyze common targets and perform mirabilite-targets network plotting according to the degree of correlation. Here, we chose the human form of Top I as a target (Fig. 1A). Because mirabilite exists in the form of sulphate ions in solution, to elucidate the mode of action of mirabilite and Top I at the molecular level, we placed the sulphate radical in the active pocket of Top I, with an affinity of −3.6 kcal mol−1. From an overall view, the sulphate radical was bound inside the gap between several Top I residues and formed a compact conformation in which it interacted with the amino acids and nucleotides around the active pocket (Fig. 1B). The four oxygen atoms of sulphate formed 9-fold hydrogen bonding, by which the ion interacted with amino acid residues Arg-364 and Asp-533, as well as nucleotides TPC-11, DG-112 and DA-113, which was the main binding force between sulphate and target (Fig. 1B). All these interactions made the sulphate form a stable complex with Top I.
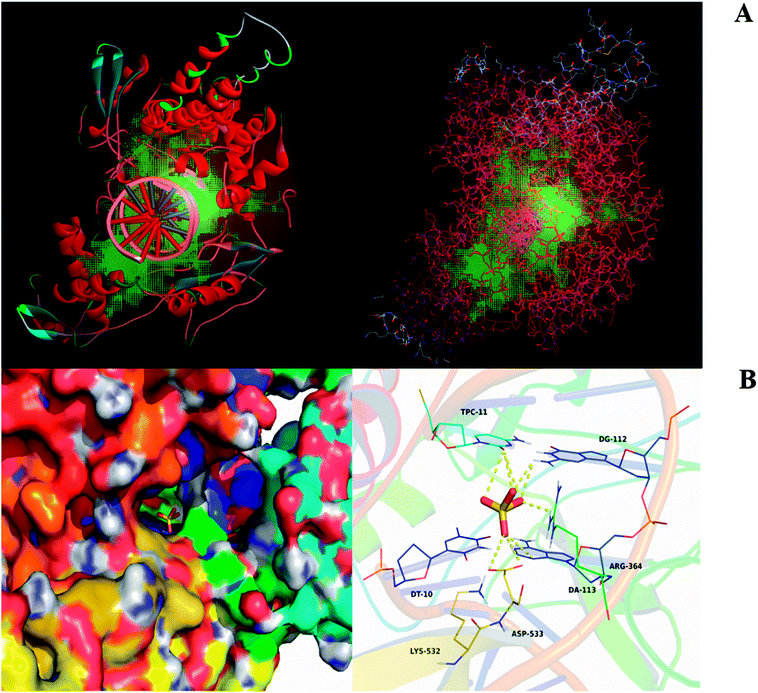 |
| Fig. 1 The network pharmacology analysis of DNA topoisomerase I and mirabilite. (A) The structure of DNA topoisomerase I. The green region represents the strongest site of action. (B) Docking of sulphate radicals in the active pocket of topoisomerase I. | |
Dynamic profile analysis of mirabilite against CRC
During the experiment, the metabolism was disturbed in each group, and the metabolic shift trajectory showed this change intuitively (Fig. 2C). On each individual day, all the sample points had similar intervals to each other and were grouped into one class. However, the samples that were treated with mirabilite at different time points were far away from each other point. The sample distribution circles at each time point were connected to build the sample trace diagram for the mirabilite group. With the extension of treatment time, the samples at each different time point tended to the 0th day, which showed that mirabilite regulated the metabolism of the APCmin/+ mice and had a positive therapeutic effect on CRC.
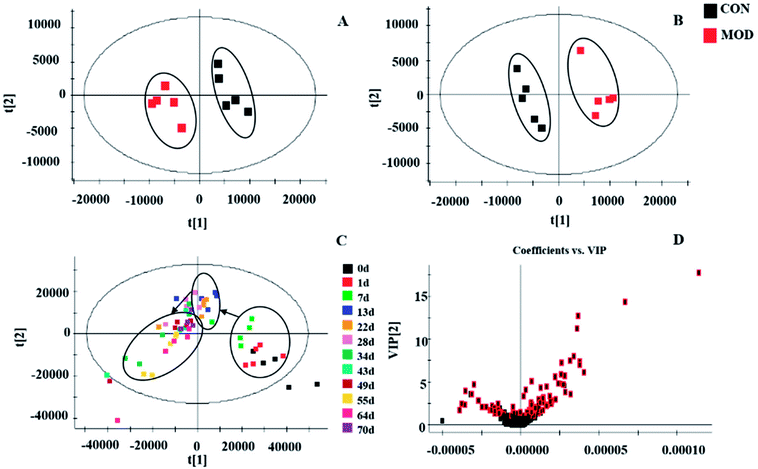 |
| Fig. 2 The multivariate statistical analysis for urine samples of the control group and the model group on the 70th day. (A) The score plot of PCA between the control group and the model group in the positive mode; (B) the score plot of PCA between the control group and the model group in the negative mode; red squares represent model group and black squares represent control group. (C) Trace of urine metabolism in the model group during the experimental period; (D) the VIP diagram of OPLS-DA between the control group and the model group. | |
The perturbed metabolites
The metabolic profiles were distinctly different between the model group and the control group (Fig. S1†) on the 70th day. At this time, we undertook chromatography peak recognition and matching and obtained information on retention time, peak intensity and m/z using Progenesis QI, which gave us 6852 ions in the positive mode and 13
750 ions in the negative mode. Next, we investigated the relationship between the control group and model group using EZinfo 2.0. The score-plot of PCA clearly showed that the urine samples were completely distinct between the different modes, which suggested that the metabolites caused differences in the metabolic profile (the physiological functions) of APCmin/+ mice affected by intestinal tumors (Fig. 2A and B). Moreover, supervised pattern recognition was used to screen compounds that made an important contribution to the classification. The S-plot of OPLS-DA displayed that the control group was far away from the model group (Fig. S2†) and the variable importance in the projection (VIP) diagram visually showed the different contributions of ions in the model (Fig. 2D), which was the basis of our analysis of the metabolic disturbance. We identified potential biomarkers by comparing the parent ion information and fragment ion information with databases such as HMDB, MetLin, Chemspider and KEGG.
Metabolic phenotypic screening of targets for CRC
After 70 days of intragastric administration of mirabilite treatment, it was found that all the samples in the mirabilite group were completely distinct from the model group according to the score-plot created by data preprocessing and statistical analysis, which indicated that the pathological function of the model mice could be reversed by the treatment with mirabilite. The S-plot further showed the difference between the mirabilite group and the model group. Mirabilite regulated some biomarkers to adjust them to the level of the control group, such as taurine, chenodeoxycholic acid, cholic acid, deoxycholic acid, isoursodeoxycholic acid etc. These potential biomarkers are related to primary bile acid biosynthesis, secondary bile acid biosynthesis and bile acid secretion, which suggested that bile acid metabolism could be an important pathway in our study.
Targeted analysis of bile acid metabolites
Among the disturbed biomarkers, taurine, chenodeoxycholic acid, cholic acid, deoxycholic acid, isoursodeoxycholic acid, murocholic acid, ursocholic acid, ursodeoxycholic acid, cholic acid glucuronide, deoxycholic acid 3-glucuronide, and 5-L-glutamyl-taurine made contributions to the intestinal tumors, and all were involved in the enterohepatic circulation and had VIP > 1. Chenodeoxycholic acid is involved in the primary bile acid biosynthesis and secondary bile acid biosynthesis; both taurine and 5-L-glutamyl-taurine belong to the taurine and hypotaurine metabolism; isoursodeoxycholic acid, murocholic acid, ursocholic acid and ursodeoxycholic acid are related to the secondary bile acid biosynthesis (Fig. 3), and were different between the APCmin/+ mice and normal mice. These disturbed metabolites suggested that the bile acid-related pathway had been altered in the CRC mice and that the enterohepatic circulation exhibited abnormal manifestations.
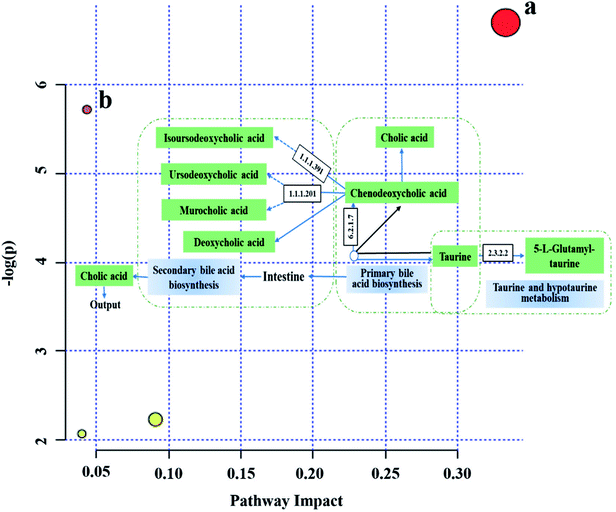 |
| Fig. 3 The bile acid-related pathways existing in the model group plotted by MetaboAnalyst 4.0. (a) Taurine and hypotaurine metabolism; (b) primary bile acid biosynthesis. | |
Bile acid metabolism pathway involved in mechanism of mirabilite against CRC
According to the relative intensity of selected biomarkers, the IPA analysis gave the canonical pathways related to CRC, which mainly included FXR/RXR activation, taurine biosynthesis, hepatic cholestasis and bile acid biosynthesis, neutral pathway (Table S1†). The upstream proteins were serine/threonine kinase (Akt), mitogen-activated kinase-like protein (Mapk), mitogen-activated protein kinase (ERK1/2), nuclear factor kappa B subunit (NF-κB), myosin light chain kinase 3 (MYLK3), RAS-related 2 (RRAS2), paraoxonase 3 (PON3), etc., which have a relationship with taurine and are involved in the inflammatory response (Fig. 4A and 5A). These enzymes play roles in apoptosis, survival, transcription and proliferation in the cell, etc. After the treatment, the increased chenodeoxycholic acid inhibited the ERK1/2, which indirectly restrained the NF-κB, Mapk and Akt. This predicted pathway suggested that primary bile acid had the function of inhibiting the inflammatory reaction. Based on metabolomics, it is known that chenodeoxycholic acid is the starting point of secondary bile acid metabolism and can be converted to secondary bile acids under the action of enzymes, and this process was upregulated by mirabilite. Additionally, the IPA predicted that chenodeoxycholic acid and deoxycholate were related to apoptosis of CRC cell lines (Fig. 5B and S3†). The canonical pathways of the pharmacodynamic biomarkers included the same canonical pathways as the disease biomarkers, and the FXR/RXR activation was upregulated after mirabilite treatment (Fig. 4B). The IPA analysis suggested that mirabilite regulated the inflammation pathway and activated the FXR receptor to prevent CRC.
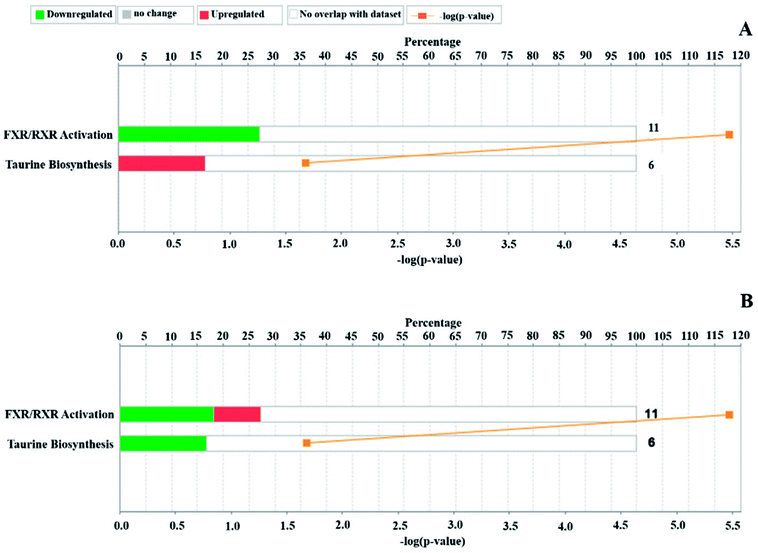 |
| Fig. 4 The canonical pathways affected by biomarkers. (A) The canonical pathways between the control group and the model group; (B) the canonical pathways between the mirabilite group and the model group. | |
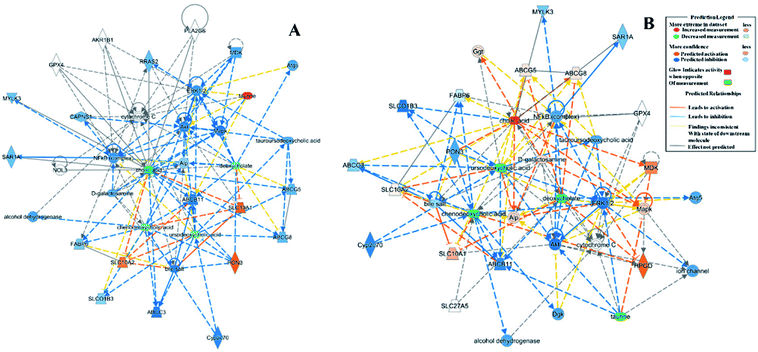 |
| Fig. 5 The relationship of biomarkers and upstream proteins by IPA analysis. (A) The predicted network of the model biomarkers; (B) the predicted network of pharmacophore biomarkers. | |
Histopathological examination
Both network pharmacology and metabolomics gave insights into the mechanism by which mirabilite can prevent CRC. We used histopathology to analyze pathological changes of the host intestinal tissue structure. The histology showed that regional intestinal epithelial cells were arranged in a disordered way in the APCmin/+ mice. In contrast, in the mirabilite group, the intestinal tissue displayed partial repair following the treatment with mirabilite (Fig. 6A). Furthermore, the FXR expression was highest in the control group, and the FXR had a higher intensity in the mirabilite group than the model group (Fig. 6B and C), which was consistent with the metabolomics and IPA results.
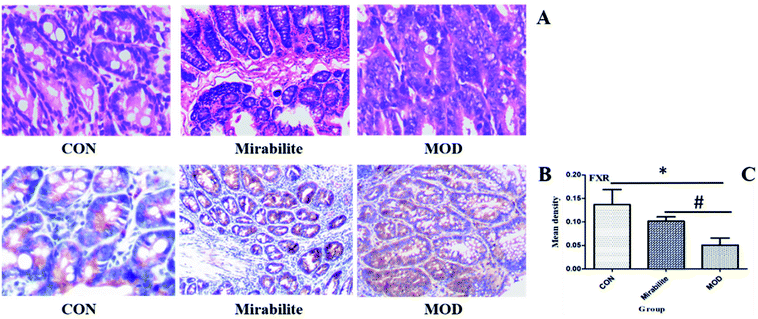 |
| Fig. 6 (A) The histopathological analysis of each group; (B) immunohistochemistry test of FXR; (C) the mean density of FXR in each group. “*” indicates significant difference between model group and control group (p < 0.05); “#” indicates significant difference between model group and mirabilite group (p < 0.05). | |
Discussion
In this study, both network pharmacology and metabolomic technology were used to explore the targets of mirabilite in the treatment of CRC and served as the basis for explaining the mechanism of mirabilite. As known, bile acids play a complex role in the process of gastrointestinal inflammation and carcinogenesis.24,25 BAs are synthesized in the liver using cholesterol as the raw material, and change form in the small intestine. There are two types of BA – primary bile acid and secondary bile acid – and conjugated bile acids are associated with bile acid secretion. Cholic acid (CA) and chenodeoxycholic acid (CDCA) are primary BAs26 and ursodeoxycholic acid is a secondary BA.27 Studies have shown that taurocholic acid is able to stimulate gut bacteria, and taurine can be converted to the gene toxin H2S,28 which can cause DNA damage of colon cells.29
It is known that FXR is the natural ligand of bile acid, and is highly expressed in the hepatocytes and enterocytes.25 The active FXR is beneficial to maintaining the intestinal shielding function.24 CDCA is an activator of FXR while, in contrast, taurocholate is an inhibitor of FXR.30 It is reported that disorders of bile acid metabolism play an important role in the development of intestinal inflammation.31 FXR is an important regulator for maintaining bile acid homeostasis and has anti-inflammatory effects.24,32 In view of this, bile acid metabolism is closely related to the development and inflammatory response of CRC. Herein, the results of metabolomics showed that the relative intensity of each biomarker returned to normal levels after mirabilite treatment and that taurine is downregulated by mirabilite, which suggested that the efficacy of mirabilite is related to changes of the bile acid metabolism. The same conclusions were found by IPA. Meanwhile, it was found by network analysis that mirabilite inhibited inflammatory factors such as NF-γB, which is associated with secondary bile acid.33 The changes of apoptosis-related proteins in IPA reflected the function of nucleic acids.34
DNA topoisomerase I (Top I) is the target of several medicinal compounds against CRC, such as camptothecins and indolocarbazoles,35 which function by suppressing the proliferation of tumor cells.36 It is known that Top I forms 3′-phosphoric acid tyrosine ester bonds and 5′-hydroxyl groups by nucleophilic attack of the phosphoric acid two bond lying in a single-stranded DNA, then the broken single strand revolves around DNA, unscrews the positive superhelix state, and continues to copy the DNA, which is essential for DNA replication and transcription. Significantly, the expression of Top I in tumor cells is higher than that in normal tissues.37,38 The network pharmacology analysis in this study suggested that Top I was a target for mirabilite in the treatment of CRC. The above molecular docking studies provided a plausible explanation for the binding mode of sulphate radicals and DNA topoisomerase I at the molecular level, which provided a theoretical basis for further study of new topoisomerase I inhibitors and showed that mirabilite could act as a potential Top I inhibitor to prevent CRC.
It is important to study the metabolic mechanism of drugs based on metabolomics analysis.39–45 In this study, metabolomics illustrated that the relative intensity of biomarkers associated with bile acid metabolism was regulated to normal levels by mirabilite. These biomarkers directly reflected the status of related upstream proteins such as ABCB11 and NFκB, which are involved in bile acids and FXR/RXR action as well as tumor cell proliferation and apoptosis. The regulated bile acids had a synergistic effect on Top I inhibitors, such as ursodeoxycholic acid.46 This result confirmed the prediction of network pharmacology at the metabolic level. Bile acid metabolism can be regarded as a possible target for mirabilite against CRC.
Conclusion
Based on network pharmacology, we used target screening and docking to predict possible targets of mirabilite; based on metabolomics, we used UPLC-Q-TOF-MS technology and multiple statistical methods to evaluate the effect of mirabilite on intestinal tumors and analyzed the related mechanisms by IPA analysis. Bile acid metabolism was closely related to intestinal tumor development, and the relevant metabolites returned to normal levels after mirabilite treatment. Moreover, the biomarkers of this metabolic pathway had a synergistic effect on the inhibition of Top I. In summary, bile acid metabolism at the metabolic level can be regarded as a potential target for the action of mirabilite. Then, we used histopathology methods to verify the efficacy of mirabilite. The current study explored the active mechanism of mirabilite in the treatment of intestinal tumors and laid the foundation for further research.
Conflicts of interest
There are no conflicts to declare.
Acknowledgements
This work was supported by grants from the Key Program of Natural Science Foundation of State (Grant No. 81430093, 81373930, 81673586, 81302905, 81503386), National Key Subject of Drug Innovation (Grant No. 2015ZX09101043-005, 2015ZX09101043-011), TCM State Administration Subject of Public Welfare of (Grant No. 2015468004), Young Talent Lift Engineering Project of China Association of Traditional Chinese Medicine (QNRC2-B06).
References
- H. Brenner, M. Kloor and C. P. Pox, Colorectal cancer, Lancet, 2014, 383(9927), 1490–1502 CrossRef.
- C. M. Dejea, P. Fathi and J. M. Craig, et al., Patients with familial adenomatous polyposis harbor colonic biofilms containing tumorigenic bacteria, Science, 2018, 359(6375), 592–597 CrossRef PubMed.
- Y. Liu, X. Zhang and C. Han, et al., TP53 loss creates therapeutic vulnerability in colorectal cancer, Nature, 2015, 520(7549), 697–701 CrossRef PubMed.
- A. Bertotti, E. Papp and S. Jones, et al., The Genomic Landscape of Response to EGFR Blockade in Colorectal Cancer, Nature, 2015, 526(7572), 263–267 CrossRef PubMed.
- J. Drost, R. H. van Jaarsveld and B. Ponsioen, et al., Sequential cancer mutations in cultured human intestinal stem cells, Nature, 2015, 521(7550), 43–47 CrossRef PubMed.
- J. Yun, E. Mullarky and C. Lu, et al., Vitamin C selectively kills KRAS and BRAF mutant colorectal cancer cells by targeting GAPDH, Science, 2015, 350(6266), 1391–1396 CrossRef PubMed.
- M. Song, W. S. Garrett and A. T. Chan, Nutrients, foods, and colorectal cancer prevention, Gastroenterology, 2015, 148(6), 1244–1260 CrossRef PubMed.
- A. J. Cross, S. C. Moore and S. Boca, et al., A prospective study of serum metabolites and colorectal cancer risk, Cancer, 2015, 120(19), 3049–3057 CrossRef PubMed.
- H. O. Adami, M. Bretthauer and L. Emilsson, et al., The continuing uncertainty about cancer risk in inflammatory bowel disease, Gut, 2016, 65(6), 889 CrossRef PubMed.
- C. H. Ryan, I. W. Ana and A. Alan, et al., Low-Grade Dysplasia in Ulcerative Colitis: Risk Factors for Developing High-Grade Dysplasia or Colorectal Cancer, Am. J. Gastroenterol., 2015, 110(10), 1461–1471 CrossRef PubMed.
- D. D. Hirsch, D. Wangsa and Y. Hu, et al., Abstract 1438: the dynamics of genetic aberrations in Crohn9s disease associated colorectal carcinogenesis, Cancer Res., 2017, 77(13), 1438 Search PubMed.
- Y. Wang, X. Zhang and C. Li, Applying Hot Compresses with Rhubarb and Mirabilite to Reduce Pancreatic Leakage Occurrence in the Treatment of Severe Acute Pancreatitis, Iran. J. Public Health, 2017, 46(1), 136–138 Search PubMed.
- A. H. Zhang, J. B. Yu, H. Sun, L. Kong, X. Q. Wang, Q. Y. Zhang and X. J. Wang, Identifying quality-markers from Shengmai San protects against transgenic mouse model of Alzheimer's disease using chinmedomics approach, Phytomedicine, 2018, S0944–7113(18), 30104–30111 Search PubMed.
- H. Ajouz, D. Mukherji and A. Shamseddine, Secondary bile acids: an underrecognized cause of colon cancer, World J. Surg. Oncol., 2014, 12(1), 164 CrossRef PubMed.
- H. Shapiro, A. A. Kolodziejczyk and D. Halstuch, et al., Bile acids in glucose metabolism in health and disease, J. Exp. Med., 2018, 215(2), 20171965 CrossRef PubMed.
- P. Louis, G. L. Hold and H. J. Flint, The gut microbiota, bacterial metabolites and colorectal cancer, Nat. Rev. Microbiol., 2014, 12(10), 661–672 CrossRef PubMed.
- S. Ocvirk and S. J. O'Keefe, Influence of Bile Acids on Colorectal Cancer Risk: Potential Mechanisms Mediated by Diet-Gut Microbiota Interactions, Curr. Nutr. Rep., 2017, 6(4), 315–322 CrossRef PubMed.
- T. Yang, T. Shu and G. Liu, et al., Quantitative profiling of 19 bile acids in rat plasma, liver, bile and different intestinal section contents to investigate bile acid homeostasis and the application of temporal variation of endogenous bile acids, J. Steroid Biochem. Mol. Biol., 2017, 69–78 CrossRef PubMed.
- Y. q. Zhang, M. Xia and S. li, et al., Network Pharmacology-based Approaches Capture Essence of Chinese Herbal Medicines, Chin. Herb. Med., 2016, 8(2), 107–116 CrossRef.
- A. Zhang, H. Fang, Y. Wang, G. Yan, H. Sun, X. Zhou, Y. Wang, L. Liu and X. Wang, Discovery and verification of the potential targets from bioactive molecules by network pharmacology-based target prediction combined with high-throughput metabolomics, RSC Adv., 2017, 7, 51069–51078 RSC.
- R. Zhang, S. Yu and H. Bai, et al., TCM-Mesh: the database and analytical system for network pharmacology analysis for TCM preparations, Sci. Rep., 2017, 7(1), 2821 CrossRef PubMed.
- W. J. Xu, L. M. Chen and Z. Y. Wei, et al., Identifying the molecular targets of Salvia miltiorrhiza (SM) in ox-LDL induced macrophage-derived foam cells based on the integration of metabolomics and network pharmacology, RSC Adv., 2018, 8, 3760–3767 RSC.
- C. H. Johnson, J. Ivanisevic and G. Siuzdak, Metabolomics: beyond biomarkers and towards mechanisms, Nat. Rev. Mol. Cell Biol., 2016, 17(7), 451 CrossRef PubMed.
- W. Jia, G. Xie and W. Jia, Bile acid-microbiota crosstalk in gastrointestinal inflammation and carcinogenesis, Nat. Rev. Gastroenterol. Hepatol., 2017, 15(2), 111–128 CrossRef PubMed.
- A. Molinaro, A. Wahlström and H. U. Marschall, Role of Bile Acids in Metabolic Control, Trends Endocrinol. Metab., 2018, 29(1), 31 CrossRef PubMed.
- M. Lyu, Y. F. Wang and G. W. Fan, et al., Balancing Herbal Medicine and Functional Food for Prevention and Treatment of Cardiometabolic Diseases through Modulating Gut Microbiota, Front. Microbiol., 2017, 8, 2146 CrossRef PubMed.
- A. Wahlström, S. Sayin and H. U. Marschall, et al., Intestinal Crosstalk between Bile Acids and Microbiota and Its Impact on Host Metabolism, Cell Metab., 2016, 24(1), 41–50 CrossRef PubMed.
- J. M. Ridlon, P. G. Wolf and H. R. Gaskins, Taurocholic Acid Metabolism by Gut Microbes and Colon Cancer, Gut Microbes, 2016, 7(3), 201–215 CrossRef PubMed.
- F. F. Guo, T. C. Yu and H. Jie, et al., Emerging Roles of Hydrogen Sulfide in Inflammatory and Neoplastic Colonic Diseases, Front. Physiol., 2016, 7, 156 Search PubMed.
- P. Puri, K. Daita and A. Joyce, et al., The presence and severity of nonalcoholic steatohepatitis is associated with specific changes in circulating bile acids, Hepatology, 2017, 67 DOI:10.1002/hep.29359.
- X. Zhou, L. Cao and C. Jiang, et al., PPARα-UGT axis activation represses intestinal FXR-FGF15 feedback signalling and exacerbates experimental colitis, Nat. Commun., 2014, 5, 4573 CrossRef PubMed.
- N. Gomezospina, C. J. Potter and X. Rui, et al., Mutations in the nuclear bile acid receptor FXR cause progressive familial intrahepatic cholestasis, Nat. Commun., 2016, 7, 10713 CrossRef PubMed.
- Y. Calmus and R. Poupon, Shaping macrophages function and innate immunity by bile acids: mechanisms and implication in cholestatic liver diseases, Clin. Res. Hepatol. Gastroenterol., 2014, 38, 550–556 CrossRef PubMed.
- J. R. Plemel, A. V. Caprariello and M. B. Keough, et al., Unique spectral signatures of the nucleic acid dye acridine orange can distinguish cell death by apoptosis and necroptosis, J. Cell Biol., 2017, 216(4), 1163–1181 CrossRef PubMed.
- Y. Arakawa, K. Ozaki and Y. Okawa, et al., Three missense mutations of DNA topoisomerase I in highly camptothecin-resistant colon cancer cell sublines, Oncol. Rep., 2013, 30(3), 1053–1058 CrossRef PubMed.
- N. K. Sharma, A. Kumar and A. Kumari, et al., Nitric Oxide Down-Regulates Topoisomerase I and Induces Camptothecin Resistance in Human Breast MCF-7 Tumor Cells, PLoS One, 2015, 10(11), 0141897 Search PubMed.
- D. C. Gilbert, A. J. Chalmers and S. F. Elkhamisy, Topoisomerase I inhibition in colorectal cancer: biomarkers and therapeutic targets, Br. J. Cancer, 2012, 106(1), 18–24 CrossRef PubMed.
- G. M. Heestand, M. Schwaederle and Z. Gatalica, et al., Topoisomerase expression and amplification in solid tumours: Analysis of 24,262 patients, Eur. J. Cancer, 2017, 83, 80 CrossRef PubMed.
- H. Chu, A. Zhang, Y. Han, S. Lu, L. Kong, J. Han, Z. Liu, H. Sun and X. Wang, Metabolomics approach to explore the effects of Kai-Xin-San on Alzheimer's disease using UPLC/ESI-Q-TOF mass spectrometry, J. Chromatogr. B: Anal. Technol. Biomed. Life Sci., 2016, 1015–1016, 50–61 CrossRef PubMed.
- A. Zhang, H. Sun, G. Yan, P. Wang and X. Wang, Mass spectrometry-based metabolomics: applications to biomarker and metabolic pathway research, Biomed. Chromatogr., 2016, 30(1), 7–12 CrossRef PubMed.
- X. Wang, A. Zhang, G. Yan, W. Sun, Y. Han and H. Sun, Metabolomics and proteomics annotate therapeutic properties of geniposide: targeting and regulating multiple perturbed pathways, PLoS One, 2013, 8(8), e71403 CrossRef PubMed.
- A. H. Zhang, H. Sun, S. Qiu and X. J. Wang, NMR-based metabolomics coupled with pattern recognition methods in biomarker discovery and disease diagnosis, Magn. Reson. Chem., 201, 51(9), 549–556 CrossRef PubMed.
- S. Qiu, A. Zhang, T. Zhang, H. Sun, Y. Guan, G. Yan and X. Wang, Dissect new mechanistic insights for geniposide efficacy on the hepatoprotection using multiomics approach, Oncotarget, 2017, 8(65), 108760–108770 Search PubMed.
- A. Zhang, H. Sun and X. Wang, Emerging role and recent applications of metabolomics biomarkers in obesity disease research, RSC Adv., 2017, 7(25), 14966–14973 RSC.
- A. Zhang, H. Sun and Y. Han, et al., Urinary metabolic biomarker and pathway study of hepatitis B virus infected patients based on UPLC-MS system, PLoS One, 2013, 8(5), e64381 CrossRef PubMed.
- T. Ikegami and Y. Matsuzaki, Ursodeoxycholic acid: Mechanism of action and novel clinical applications, Hepatol. Res., 2010, 38(2), 123–131 Search PubMed.
Footnote |
† Electronic supplementary information (ESI) available. See DOI: 10.1039/c8ra04886j |
|
This journal is © The Royal Society of Chemistry 2018 |
Click here to see how this site uses Cookies. View our privacy policy here.