DOI:
10.1039/C8RA01295D
(Paper)
RSC Adv., 2018,
8, 17079-17090
Geographical patterns of denitrifying bacterial communities associated with different urban lakes†
Received
12th February 2018
, Accepted 30th April 2018
First published on 9th May 2018
Abstract
The geographical variation of denitrifying bacterial communities and water quality parameters in urban lakes distributed across nine provinces in China were determined. The Illumina sequencing data of the denitrifying encoding gene nirS was examined in the samples collected from nine localities (pairwise geographical distance: 200–2600 km). The results showed that fundamental differences in water quality were observed among different urban lakes. The highest nitrate (2.02 mg L−1) and total nitrogen (3.82 mg L−1) concentrations were observed in Pingzhuang (P < 0.01). The algal cell concentration ranged from 1.29 × 108 to 3.0 × 109 cell per L. The sequencing data generated a total of 421058 high quality nirS gene reads that resulted in 6369 OTUs (97% cutoff), with Proteobacteria and Firmicutes being the dominant taxa. A co-occurrence network analysis indicated that the top five genera identified as keystone taxa were Dechlorospirillum sp., Alicycliphilus sp., Dechloromonas sp., Pseudogulbenkiania sp., and Paracoccus sp. A redundancy analysis (RDA) further revealed that distinct denitrifying bacterial communities inhabited the different urban lakes, and influenced by urban lake water ammonia nitrogen, manganese and algal cell concentrations. A variance partitioning analysis (VPA) also showed that geographic location was more important than water quality factors in structuring the denitrifying bacterial communities. Together, these results provide new insight into understanding of denitrifying bacterial communities associated with geographically distributed urban lakes on a larger scale, and these results also expand our exploration of aquatic microbial ecology in freshwater bodies.
1. Introduction
Global warming can lead to more extreme climate change events, especially in the vast territory of China.1 With the rapid development of urbanization, urban environment conditions have changed dramatically, such as heavy rains and windstorms occurring more often in the south, mild winters in the northwest, and more extreme weather in the Northeast of China.2,3 Combined with the development of the manufacturing industry and human activity, urban water environmental conditions are seriously threatened.4 Over the past several decades, urban lakes have been widely created to store rainwater and for recreational purposes.4 Urban lakes are distinct from natural lakes, they are highly artificial and have recreational function. Compared with natural lakes, most urban lakes are exposed to heavy pollution and eutrophication due to the large amount of pollutants (e.g., nitrogen and heavy metals) discharged and flushed into urban lakes, especially when short-duration extreme events occur, resulting in water turbidity, toxic algal blooms and higher loading of municipal and industrial contaminants.5 Thus, understanding the fundamental features of urban lake ecosystems is of great interest and importance because it may shed light on the management and restoration processes of urban lakes.
In freshwater ecosystems, water microorganisms play crucial roles in regulating the health status of water bodies by decomposing the organic matter (OM) and driving the nitrogen (N), phosphorus (P) and sulfur (S) biogeochemical cycles.6 To date, most studies have focused on exploring microbial community diversity in drinking water reservoirs,7,8 polluted rivers,9,10 drinking water pipes,11 and entropic lakes.12,13 In lake ecosystems, it has long been of significant interest to study the relationships between water quality and sediment bacterial,13 archaeal,14 and fungal15 communities. Numerous water physical–chemical factors, such as the pH, total nitrogen (TN) and organic carbon levels, have been evaluated to understand their effect on the distribution of the microbial community.7,9,13 Hengy et al.16 recently revealed the relationships between water quality and bacterial communities within four freshwater lakes around the same island at a local scale and suggested that water temperature and pH were significantly related to bacterial communities associated with surface and bottom water columns, but the dissolved organic carbon (DOC) was not related to the bacterial community structure. However, the study focused on exploring denitrifying bacterial community in lakes is limited.
In the past few decades, the rapid development of genomic and bio-informatics techniques has informed freshwater microbial ecology.17 Compared to the denaturing gradient gel electrophoresis (DGGE), terminal restriction fragment length polymorphism (T-RFLP), catalyzed reporter deposition-fluorescence in situ hybridization (CARD-FISH) and clone sequence methods,18 high-throughput sequencing is a powerful approach for broad microbial investigation. The Illumina Miseq sequencing technique has received increasing attention and is widely used to explore environmental microbial communities.7–9,18 In a recent study, with the aid of the Illumina Miseq sequencing platform, Boenigk et al.19 determined the effect of mountain ranges as biogeographic barriers on spatial patterns and the protist community structure in 218 European freshwater lakes and suggested that the protist community composition in lowland lakes was affected by geographic distance.
Denitrifying bacteria, a fundamental and diverse group of microorganisms, essentially drive nitrogen cycling processes in water bodies.20 For denitrifying bacteria, geographic gradients are generally known but presumably weaker in lakes than in wastewater treatment systems. Up to now, although some recent studies have attempted to investigate the denitrifying bacterial community in urban lakes using a high-throughput sequencing technique,21,22 these studies were mainly carried out in the same region with similar source water supplies. In a previous study, Kang et al.22 determined the water denitrifying bacterial community based on nirS gene in six urban lakes in Xi'an, Shaanxi province, Northwest China, and found that individual urban lakes were chemically and physically distinct, even within the same geographic area, meanwhile the TN and CODMn were observed as the dominant factors affecting denitrifying bacteria at local small-spatial scales. Unfortunately, the forces that govern their structure and diversity associated with urban lakes across large geographic spaces remain unclear. Studies of urban lake water-based denitrifying bacterial communities and distance dynamics are both needed to address this gap in knowledge.
The primary aim of the present study was to explore the biogeography of the urban lake water-based denitrifying bacterial community structure at a large spatial scale. The specific objectives of this work were to: (1) investigate the water quality parameters, (2) profile the nirS-type denitrifying bacterial community structure in urban lakes from 9 different geographic locations using Illumina Miseq sequencing, based on the denitrifying encoding gene nirS, (3) assess the water quality parameters that influence the biogeography of denitrifying bacterial diversity and structure in urban lakes distributed across a wide range of geographic locations in China. The results from this work will produce useful insights into the aquatic microbial ecology characteristics of urban lake ecosystems.
2. Materials and methods
2.1. Site description and field sampling
China has a wide range of climatic conditions that vary with the seasons and geography.3 To compare the denitrifying bacterial community composition of urban lakes from various regions of China in the present study, urban lakes were selected from nine provinces located from the south to the north areas. As shown in Fig. S1,† the urban lakes were located in nine different geographic locations (Beijing, Jilin, Inner Mongolia, Shaanxi, Qinghai, Gansu, Henan, Guizhou, and Guangdong province). The nine urban lakes are Aolinpike (Abbr. ALPK), Daminggong (DMG), Guanshanhu (GSH), Huangshui (HS), Jingyuetan (JYT), Pingzhuang (PZ), Renmin (RM), Yantan (YT), and Zhongxinhu (ZXH) (Table S1†). The abbreviations and main characteristics of these urban lakes are summarized in Table S1.† The field sampling process was conducted in June 2017. In each urban lake, three representative sampling sites (n = 3) were selected as described by Kang et al.,22 and surface water samples (0.5–1.0 m) were collected using sterilized polypropylene containers (Kangning, China). All sample bottles were put into a cooler (8 °C) and transferred to the laboratory at the Institute of Environmental Microbial Technology, Xi'an University of Architecture and Technology (IEMT-XAUAT) within 24 h. One part of the water sample was used to determine the water physicochemical characteristics and algal cell concentration. Another part of the water sample was used to investigate the water denitrifying bacterial community. Each water sample (500 mL) was filtered through 47 mm polycarbonate membrane (0.22 μm pore size, Millipore). The filters were maintained at −20 °C until further treatment.
2.2. Water physicochemical analysis
To measure the water quality of the urban lakes, the pH, nitrite nitrogen (NO2−-N), nitrate nitrogen (NO3−-N), ammonia nitrogen (NH4+-N), total nitrogen (TN), total phosphorus (TP), permanganate index (CODMn), Fe and Mn concentrations were measured. The pH was measured using a pH meter (Hach, USA) in the field. Nitrite nitrogen (NO2−-N), nitrate nitrogen (NO3−-N), ammonium nitrogen (NH4+-N), and total nitrogen (TN) concentrations were determined using a flow injection analyzer (FIA) (Seal Analytical AA3, Norderstedt, Germany) based on a previously described method.22 TP was measured using a spectrophotometer (DR6000, Hach, USA). The Fe and Mn concentrations were measured using Inductively Coupled Plasma Mass Spectrometry (ICP-MS) (ELAN® DRC-E, PerkinElmer, USA).22 To measure the algal biomass, the algal cell concentration was determined using an optical microscope (BX51, Olympus, Japan) and the cell concentration (cells per L) was calculated using the method described previously.23 Each experiment was performed in triplicate (n = 3).
2.3. DNA extraction and purification
To extract the water microbial genomic DNA, the total microbial DNA on the filter was extracted using a water DNA extraction kit (Omega, USA) according to the manufacturer's instructions. The raw extracted DNA was purified using a DNA purification kit (Thermo Fisher Scientific, USA) following the manufacturer's protocols. The quality and quantity of the DNA product (A260/A280) was checked with a Nano-drop UV-Vis Spectrometer (ND 2000, Thermo Scientific, Waltham, MA, USA). The DNA samples were then stored at −80 °C in the laboratory for polymerase chain reaction (PCR) amplification analyses.24 All procedures were performed on ice.
2.4. Illumina Miseq sequencing
To determine the water denitrifying bacterial community, nirS and nirK gene were used as marker genes to explore the diversity of denitrifiers.25 In the present work, the denitrifying functional gene, nirS, was sequenced on an Illumina MiSeq platform.26 Briefly, for each sample, PCR amplification was performed using primers Cd3a-F (5′-GTSAACGTSAAGGARACSGG-3′) and R3cd-R (5′-GASTTCGGRTGSGTCTTGA-3′) for the nirS gene.25 The R3cd-R primer was bar coded with 6 bp error-correcting for each sample. The polymerase chain reaction (PCR) was carried out in a 20 μL mixture containing 10 ng of microbial genomic DNA template, 10 μL 2 × PCR Master Mix (Thermo Fisher Scientific, USA), 1 μL of each primer (10 μM), and 7 μL of PCR-grade water. The PCR was carried out on a Thermal Cycler (ABI-9700, USA) using the following steps: 95 °C for 5 min, 35 cycles of denaturation at 95 °C for 30 s, 30 s at 60 °C, and 30 s at 72 °C, followed by a final extension for 10 min at 72 °C. The PCR products were checked using 1.5% (w/v) agarose gel electrophoresis (Bio-Rad, USA). They were then purified using PCR clean-up kits (Thermo Fisher Scientific, USA) according to the manufacturer's instructions, and quantified using Quanti Fluor TM-ST (Promega, USA). For each sample, independent PCR products (n = 3) were mixed to construct a PCR amplicon library. The following steps for library preparation and sequencing were performed using the Illumina Miseq sequencing platform (Illumina PE250) by Shanghai BIOZERON Biotechnology Co., Ltd. (Shanghai, China) in accordance with the previously described protocols.25–27
2.5. Processing of sequencing data
After Illumina Miseq sequencing, the raw nirS gene sequencing data were further filtered with clean tags and quality-controlled using the Quantitative Insights into Microbial Ecology (QIIME) software package (version 1.17).28 Unspecific PCR amplicons (chloroplast, archaea, unknown domain) were removed. Reads with an average quality score < 20 and chimeric sequences were identified and also removed using the USEARCH algorithm, as well as those shorter than 50 bp. At the same time, singletons were removed. The average read length of nirS was 373 bp. For good quality amplicon sequence data, the sequences were clustered into an operational taxonomic units (OTUs) grouping at 97% similarity using UPARSE pipelines (version 7.1 http://drive5.com/uparse/) and the Mothur software (version 1.35.1) (http://www.mothur.org).29 Under the same sequencing number (28846 reads), the diversity indices between samples were calculated and compared. The nirS gene sequence data was classified using the RDP Classifier (http://rdp.cme.msu.edu/).28
2.6. Nucleotide sequence accession number
The original sequencing data generated in the present study were submitted to the NCBI Sequence Read Archive (SRA) database (http://www.ncbi.nlm.nih.gov/sra) under accession number SRP129549.
2.7. Statistical analysis
To compare the water quality parameters in different urban lakes, we used a one-way ANOVA followed by a post hoc Tukey's honestly significant difference (HSD) test. To assess the diversity of the denitrifying bacterial community, diversity indices, including Chao 1, Shannon's diversity (H′) and the Simpson diversity (D) index, were calculated based on the OTUs described previously.27,28 The number of shared and unique functional clusters are evaluated via a Venn diagram. A heat map profile was used to compare the observed denitrifying bacterial community structure at the genus level.7,8 The distribution of the dominant family was visualized by using Circos software (version 0.69, http://circos.ca/). A co-occurrence network was generated using a visualized Gephi platform (version 0.9.2) to show the biotic relationships among denitrifying bacterial taxa in nine different sequencing libraries, and the number of nodes, graph density, and clustering coefficient were calculated.29 A detrended correspondence analysis revealed that the length of the first axis was less than 3.30 A unimodal denitrifying bacterial species and water quality relationship method was used to generate a redundancy analysis (RDA). The interrelation between the water quality and water denitrifying bacterial communities of the different urban lake samples were measured using multivariate correlation analysis (redundancy analysis, RDA), which was performed using the Canoco software package for Windows (version 4.5) (Ithaca, New York, USA) with Monte Carlo permutation tests (999 permutations). The graphics were generated in Cano Draw (version 3.10) for Windows.30 Furthermore, the contributions of the water quality parameters to the main genera community were quantified by variation partitioning analyses (VPA) using the R package vegan (version 3.4.4).
3. Results and discussion
3.1. Water quality parameters
The physicochemical parameters of the water quality from different urban lakes, such as the pH, NO3−-N, NO2−-N, NH4+-N, TN, TP, CODMn, TOC, Fe and Mn concentrations, were summarized in Table 1. Fundamental differences in water quality were observed among the different urban lakes. The pH values ranged from 7.72 in ZXH to 8.92 in PZ (F = 3.41, P < 0.05). The highest NO3−-N (2.02 mg L−1) and TN (3.82 mg L−1) concentrations were observed in PZ (F = 5.51, P < 0.01; F = 3.84, P < 0.01). The NH4+-N concentrations varied from 0.23 to 0.88 mg L−1 (F = 42.11, P < 0.01). The TOC concentrations (from 2.26 to 13.47 mg L−1) also showed statistically significant differences (F = 120.25, P < 0.01) among the samples. The CODMn was significantly higher in the ZXH samples than that of other sampling sites (F = 30.59, P < 0.01). A significant difference in TP values appears to exist among urban lakes located from the Southern to Northern parts of China (F = 7.38, P < 0.01). The algal cell concentration was high in all lakes, varying between 1.29 × 108 to 3.0 × 109 cell per L (F = 2284, P < 0.001). However, the Mn concentrations were not significantly different between samples (P > 0.05). Similarly, Yang et al.31 sampled water from 16 lakes, and the pairwise distances between the sampled lakes ranged from 9 to 2027 km, and found that pH values varied from 6.9 to 9.8 in 16 lakes in Western China. In the present study, the pairwise distances between the urban lakes ranged from 200 to 2600 km. ZXH was located in Guangdong in Southern China, and JYT was located in Jilin in Northeast China. A number of studies have shown that spatial distance can cause spatial differences in water quality characteristics. JYT, built in 1934, is one of the oldest urban lakes in Jilin. With the rapid development of urbanization of Jilin province, serious water pollution event was occurred during the past decades, such as heavy metals pollution and industrial pollutant emissions. After that, the contaminants loaded in the bottom sediment of urban lakes and released from the sediment into the overlying water. The present work agrees with Andersson et al.32 and suggested that the spatial difference of urban lakes is influenced by environmental conditions as well as historical events. DMG and RM are close to one another, but the algal cell concentrations in RM was 17 times higher than that of DMG. The result shown that urban lakes in locations with similar geographical and weather characteristics may differ in water quality.32 Li et al.33 investigated the water quality in Honghu Lake and found that TN, TP, and CODMn concentrations gradually increased from the Southern to the Northern part of the entire lake basin because the correlations found that submerged aquatic plants can regulate the water quality in Honghu Lake. A previous study revealed that urbanization dramatically influences water chemistry, hydrology and productive parameters.34 In the United States, 30 urban lakes from northern and Southern Wisconsin were explored by Yannarell et al.,35 which revealed that pH value ranged from 5.4 to 8.5, which was lower than that of the present work. It is therefore that water quality of urban lakes can be shaped by environmental geochemical factors and land use in extensively-explored areas of cities.
Table 1 Water quality parameters associated with nine geographically distributed urban lakes, Chinaa
Urban lakes |
Aolinpike (ALPK) |
Daminggong (DMG) |
Guanshanhu (GSH) |
Huangshui (HS) |
Jingyuetan (JYT) |
Pingzhuang (PZ) |
Renmin (RM) |
Yantan (YT) |
Zhongxinhu (ZXH) |
One way ANOVA |
Values showed as means and standard deviations (three replicates). Different capital letter represents statistical significance. *P < 0.05, **P < 0.01, and ***P < 0.001 represent statistical significance using One way ANOVA followed by a post hoc Tukey's honestly significant difference (HSD) test. NS represents no statistical significance. |
pH |
7.93 ± 0.01A |
8.49 ± 0.11A |
7.98 ± 0.08A |
8.01 ± 0.09A |
7.74 ± 0.68A |
8.92 ± 0.80A |
7.97 ± 0.01A |
8.36 ± 0.15A |
7.72 ± 0.12A |
* |
NO2−-N (mg L−1) |
0.02 ± 0.00B |
0.01 ± 0.00B |
0.04 ± 0.00AB |
0.07 ± 0.00A |
0.02 ± 0.02B |
0.02 ± 0.02B |
0.03 ± 0.00B |
0.03 ± 0.00B |
0.03 ± 0.00B |
*** |
NO3−-N (mg L−1) |
0.46 ± 0.01B |
0.40 ± 0.04B |
0.70 ± 0.02AB |
1.32 ± 0.02AB |
0.64 ± 0.08AB |
2.02 ± 1.12A |
0.69 ± 0.02AB |
0.58 ± 0.00AB |
0.30 ± 0.02B |
** |
NH4+-N (mg L−1) |
0.26 ± 0.07C |
0.23 ± 0.01C |
0.38 ± 0.12C |
0.88 ± 0.03A |
0.28 ± 0.07C |
0.43 ± 0.05C |
0.66 ± 0.03B |
0.33 ± 0.01C |
0.31 ± 0.00C |
*** |
TN (mg L−1) |
0.91 ± 0.11A |
1.06 ± 0.25A |
1.30 ± 0.16A |
3.28 ± 0.34A |
0.93 ± 0.07A |
3.82 ± 2.52A |
1.00 ± 0.07A |
1.73 ± 0.32A |
1.34 ± 0.06A |
** |
TP (mg L−1) |
0.09 ± 0.00B |
0.08 ± 0.00B |
0.10 ± 0.04B |
0.10 ± 0.01B |
0.11 ± 0.05AB |
0.05 ± 0.02B |
0.19 ± 0.01A |
0.12 ± 0.03AB |
0.08 ± 0.01B |
*** |
CODMn (mg L−1) |
5.72 ± 0.09C |
4.80 ± 0.11C |
3.81 ± 0.34C |
11.18 ± 0.08A |
5.50 ± 0.53C |
7.18 ± 1.47BC |
5.38 ± 0.14C |
10.68 ± 0.00AB |
12.56 ± 0.12A |
*** |
TOC (mg L−1) |
4.04 ± 1.25DE |
3.84 ± 0.46DE |
2.26 ± 0.25E |
14.15 ± 0.94A |
5.50 ± 0.60CD |
7.18 ± 0.70BC |
4.62 ± 0.38DE |
13.47 ± 0.52A |
9.12 ± 0.35B |
*** |
Fe (mg L−1) |
0.07 ± 0.00A |
0.07 ± 0.00A |
0.05 ± 0.00AB |
0.01 ± 0.00B |
0.04 ± 0.02AB |
0.05 ± 0.02AB |
0.04 ± 0.01AB |
0.06 ± 0.01A |
0.02 ± 0.00B |
*** |
Mn (mg L−1) |
0.00 ± 0.00A |
0.00 ± 0.00A |
0.00 ± 0.00A |
0.00 ± 0.00A |
0.01 ± 0.00A |
0.00 ± 0.00A |
0.01 ± 0.00A |
0.01 ± 0.00A |
0.01 ± 0.00A |
NS |
Algal cell (million per L) |
4469 ± 63C |
344 ± 44F |
2912 ± 56DE |
752 ± 53F |
30 000 ± 500A |
6851 ± 97B |
2316 ± 44E |
3896 ± 83CD |
335 ± 31F |
*** |
3.2. Water denitrifying bacterial community diversity
The Illumina Miseq sequencing data of the nirS gene were used to profile the overall pattern of the denitrifying bacterial community. After quality filtering, denoising and removal of chimera, 438444 sequences were obtained before filtering and quality control. A total of 421058 nirS gene sequence tags were generated through Illumina Miseq sequencing, with an average length of approximately 373 bp. High quality sequencing data were used to calculate the diversity index and to generate the taxonomic communities. In the OTU-based analyses of these sequences, 6369 OTUs were identified (Table 2). The GSH harbored the most OTUs of denitrifying bacteria (942 OTUs) followed by DMG (785 OTUs). The lowest number was observed in PZ with 469 OTUs.
Table 2 Water denitrifying bacterial community diversity index based on the Illumina Miseq sequencing data from nine geographically distributed urban lakes, China. The reads number is 28846 for each sample
Urban lakes |
0.97 level |
OTU |
Chao 1 |
Shannon diversity (H′) |
Simpson diversity (D) |
Coverage |
Aolinpike (ALPK) |
762 |
909(861, 979) |
4.8(4.78, 4.82) |
0.02(0.02, 0.02) |
0.994 |
Daminggong (DMG) |
785 |
913(869, 978) |
4.67(4.65, 4.7) |
0.03(0.03, 0.03) |
0.994 |
Guanshanhu (GSH) |
942 |
1048(1013, 1101) |
5.18(5.16, 5.2) |
0.015(0.015, 0.015) |
0.994 |
Huangshui (HS) |
669 |
775(737, 832) |
4.8(4.79, 4.82) |
0.017(0.016, 0.017) |
0.995 |
Jingyuetan (JYT) |
500 |
526(512, 556) |
4.15(4.13, 4.17) |
0.041(0.040, 0.042) |
0.998 |
Pingzhuang (PZ) |
469 |
511(492, 546) |
4.0(3.98, 4.03) |
0.063(0.061, 0.065) |
0.997 |
Renmin (RM) |
781 |
994(932, 1082) |
4.59(4.57, 4.61) |
0.035(0.034, 0.036) |
0.992 |
Yantan (YT) |
752 |
822(795, 865) |
4.99(4.97, 5.01) |
0.018(0.018, 0.019) |
0.996 |
Zhongxinhu (ZXH) |
709 |
723(715, 740) |
4.97(4.95, 4.99) |
0.019(0.019, 0.02) |
0.998 |
As shown in Table 2, denitrifying bacterial community diversity is undoubtedly immense. Chao 1 ranged from 511 to 1048 across the nine urban lakes. The highest Chao 1 was in GSH, while the lowest was in the PZ water. The GSH urban lake showed the highest Shannon diversity (H′, 5.18). In a total of nine samples, the coverage was higher than 0.99. No significant difference in coverage was observed among the nine sampling sites. Notably, our study revealed a high level of diversity of denitrifying bacterial community associated with urban lake ecosystems. Compared with the previous study conducted by Yi et al.,36 the Shannon diversity (H′) index value of the denitrifying bacteria communities was higher than that of Dianchi Lake in Yunnan, China (1.73 to 2.80). The most important reason was that the Illumina Miseq method was more powerful than denaturing gradient gel electrophoresis (DGGE), because the Illumina Miseq technique can yield detailed information and perform deep surveys of community diversity.27 In this work, JYT is located in a cold area, and a lower Chao 1 index was observed in the JYT urban lake. It is consistent with previous report by Wang et al.,37 who demonstrated that air temperature was significantly correlated with nirS-type denitrifier assemblages in Baiyangdian Lake.
3.3. Geographical patterns of denitrifying bacterial community composition
Overall, the OTUs from nine urban lakes were classified and grouped under 4 bacterial phyla. All the urban lakes were dominated by Proteobacteria. The majority of the OTUs were Proteobacteria (accounting for 94% of the total effective sequences in DMG, 90% in GSH, and 87% in RM), followed by Firmicutes (accounting for 7.7% of the total effective sequences in HS, 2% in YT, and 1.8% in JYT) (Fig. 1). Firmicutes can be frequently found in other environmental ecosystems, which can survive in extreme conditions.7,12,13,31 The largest proportion of the OTUs in PZ with 28% was unclassified. At family level, the majority of sequence reads were affiliated with known members of Rhodocyclaceae. This phylum accounted for 33.44% of total reads (Fig. 2). Pseudomonadaceae was the second most abundant family (29.98% of total reads). Other major family included Sinobacteraceae, Rhodobacteraceae. Likewise, a significant difference in the denitrifying bacterial community was observed among the nine sampling sites at order levels (Fig. 3). At the order level, Pseudomonadales (accounting for 39% of the total effective sequences) was observed in ALPK, as was Rhodocyclales (36%) in DMG, Nevskiales (22%) in HS, and Burkholderiales (15%) in RM. In addition, unclassified OTUs were predominant, accounting for nearly 28% in PZ at the phylum level (Fig. 3). These data indicate that urban lakes harbor an abundance of rare denitrifying bacteria. Notably, the phyla Proteobacteria and Firmicutes generally play a key role in the biogeochemical cycles of urban lake water ecosystems. Consistent with the published literatures, Proteobacteria was the largest and most diverse phylum in the other aquatic environments, such as wetlands (approximately 45% of total OTUs),38 ponds (accounting for 35%),39 and rivers (approximately 39% of total OTUs).40 We also found that the predominant phyla of Proteobacteria and Firmicutes were not correlated with the geographical distance between the sampled lakes (P > 0.05).
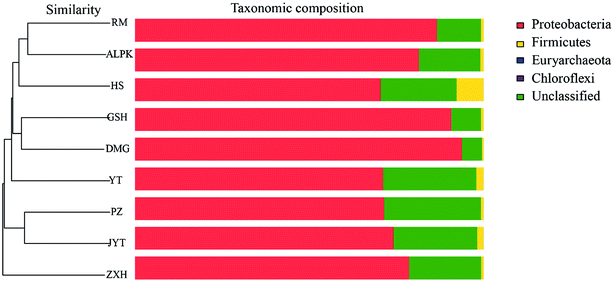 |
| Fig. 1 Taxonomic classification of water denitrifying bacterial community reads obtained from Illumina Miseq data of nine geographically distributed urban lakes at phylum level using the Ribosomal Database Project (RDP) classifier. | |
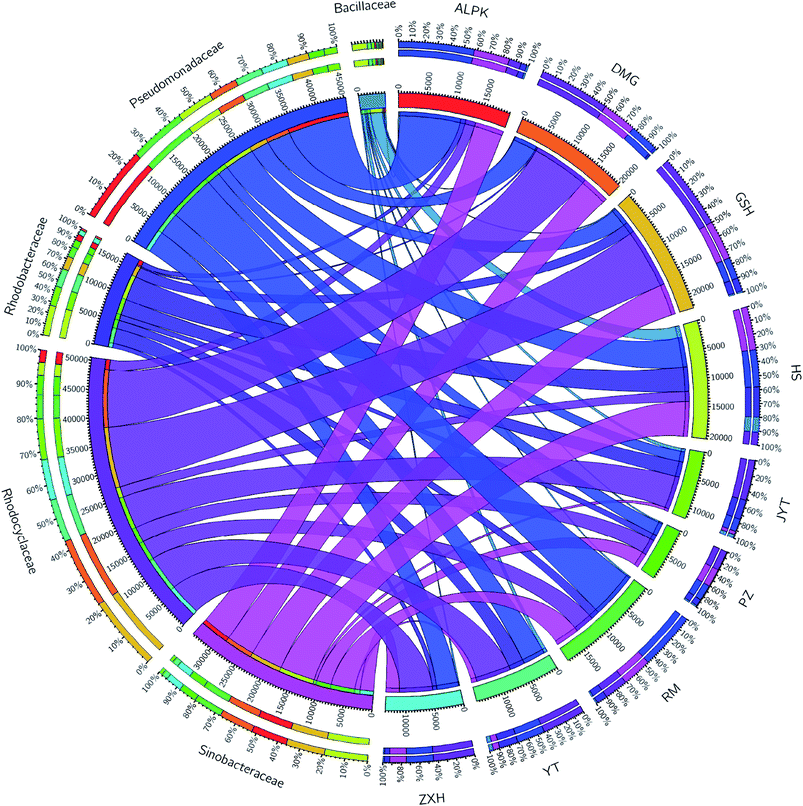 |
| Fig. 2 Circos representation of top most abundant denitrifying bacterial community at family level. The bands with different colors demonstrate the source of different family. The data were visualized via Circos software (http://circos.ca/). | |
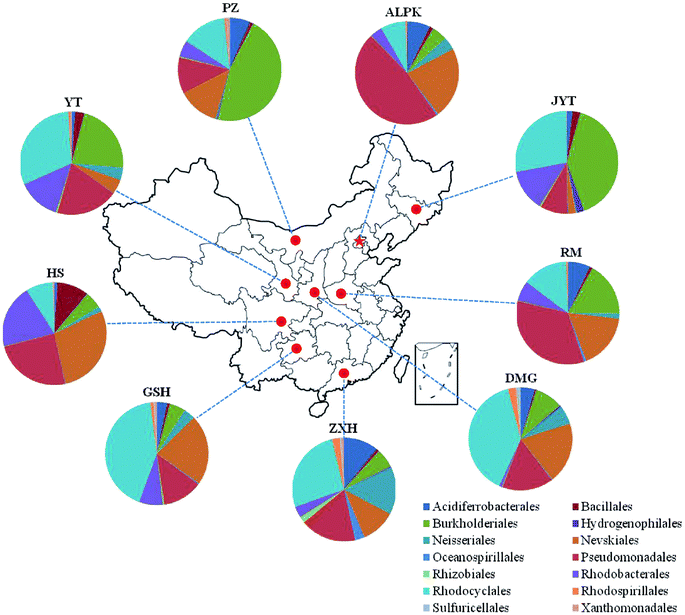 |
| Fig. 3 Taxonomic classification of water denitrifying bacterial community reads obtained from Illumina Miseq sequencing data of nine geographically distributed urban lakes into order level using the Ribosomal Database Project (RDP) classifier. | |
Analyses of a co-occurring network have been successfully conducted to infer microbial co-occurrence patterns, which can allow scientists to elucidate microbial coexistence at different levels of whole communities in diverse aquatic environments.41–44 Accordingly, in this study, to investigate the direct or indirect interactions between denitrifying bacterial taxa (i.e., abundant genera) coexisting in urban lake samples, here we used network analyses to explore significant taxon co-occurrence patterns in urban lakes. Through this investigation, as shown in Fig. 4, we visualized networks within urban lakes for both the negative and positive co-occurrence of ecologically relevant relationships between microbial taxa. Co-occurring denitrifying bacterial genera networks further found that community composition strongly differs among the nine urban lakes. The network incorporated 47 nodes. The results clearly demonstrated that the nodes were classified as five modules, as revealed by five different colors. Modules I and II had 13 and 12 nodes (genera). The connected nodes with a larger node size (e.g., Dechlorospirillum sp.) have higher significant negative or positive correlations with other taxa (genera). The top five genera identified as keystone taxa were Dechlorospirillum sp., Alicycliphilus sp., Dechloromonas sp., Pseudogulbenkiania sp., and Paracoccus sp. Interestingly, the degree (number of connections) for Ralstonia sp. is 2 indicated that only two genera were significantly correlated with Ralstonia sp. Meanwhile, the degree of Dechlorospirillum sp. is 16, revealing more significant correlations with other denitrifying bacteria genera. The network confirms that urban lakes have complex inter-relationships of denitrifying bacteria taxa. Therefore, the assessment of co-occurrence networks is a powerful technique to investigate the interactions occurring within denitrifying bacterial community compositions, such as competition and commensalism.
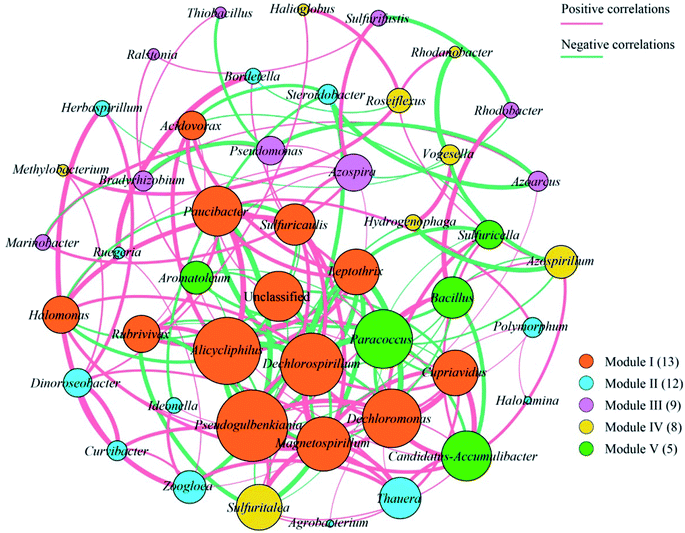 |
| Fig. 4 Network of co-occurring denitrifying bacterial genera based on Spearman's correlation significant analysis (P < 0.01) across nine geographically distributed urban lake samples. The size of node represents proportional to the relative abundance. The nodes were colored by genus. The thickness of each edge (connection between two nodes) is degree to the value of correlation coefficients. | |
To further explore the community profile, as shown in Fig. 5, heat map profiles were generated with the top 48 abundant denitrifying bacterial community composition at the genus level. The profiles showed that several denitrifying bacterial communities exhibit distinct patterns at the genus level, and revealed that the denitrifying bacterial communities in nine urban lakes were more diverse and different. Pseudomonas sp. (39%) and Steroidobacter sp. (19%) were the dominant genera in ALPK, Dechloromonas sp. (24%) and Pseudomonas sp. (15%) were dominant in DMG. Steroidobacter sp. (20%) was dominant in GSH, and Azoarcus sp. (19%) was dominant in JYT (Fig. 5). In PZ, 28% of the OTUs were unclassified. This result is consistent with a recent study conducted by Kang et al.,22 the results found that Pseudomonas sp. and Dechloromonas sp. were abundant in six different urban lakes located in Xi'an, Shaanxi, China. In wastewater ecosystems, Zhang et al.45 also used the terminal restriction fragment length polymorphism (T-RFLP) and cloning sequencing techniques to explore the diversity of nirS-type denitrifiers in Qinling lake, and showed that the dominate genera included Pseudomonas sp. and Steroidobacter sp.
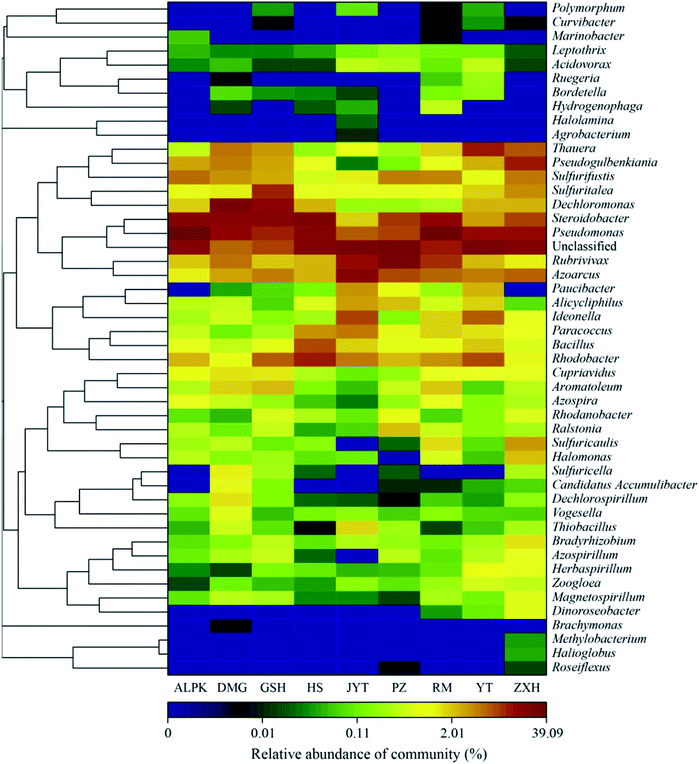 |
| Fig. 5 Heat map profile showing 48 predominant denitrifying bacterial nirS gene based sequence classified at the genus level using the Ribosomal Database Project (RDP) classifier database. | |
3.4. Relationship between denitrifying bacterial communities and water quality
To further explore the relationship between denitrifying bacterial communities and water quality associated with different geographically distributed urban lakes, redundancy analysis (RDA), a linear canonical ordination method, was conducted. As shown in Fig. 6, the first two axes of the RDA analysis explained 63.5% of the total variance for the denitrifying bacterial communities. The Monte Carlo permutation test showed that ammonia nitrogen, Mn concentration, and the algal cell number were significantly correlated with the changes in the denitrifying bacterial composition. This is in line with the findings of Yannarell et al.,46 who suggest that algal biomass (as expressed by chlorophyll a concentrations) was the dominant factor that may regulate the freshwater bacterial community structure in 30 Wisconsin lakes, which were located throughout the Northern Highlands Lake district and several counties in Southern Wisconsin, USA. In general, the RDA biplot clearly showed that ZXH, GSH and DMG were located in the third quadrant, whereas PZ, YT and JYT were located in the fourth quadrant (Fig. 6). The result revealed the differences among the denitrifying bacterial communities in the nine geographically separated urban lakes, which had diverse physicochemical water properties. Similarly, Kang et al.22 revealed that TN and TP had the dramatic influence on the nirS-type denitrifying bacterial communities in Laodong, Yongyang and Fengqing urban lakes. In alkaline lake sediments, pH was the best predictor of bacterial community structure, and both geographical distance and chemical factors governed bacterial biogeography in lake sediments across the Tibetan Plateau, China.47 It is well known that dynamics in water quality can physically disturb denitrifying bacterial activity and affect their community succession.28 This results also expands our current understanding of the relationship between water quality and denitrifying bacterial community compositions in urban lakes.
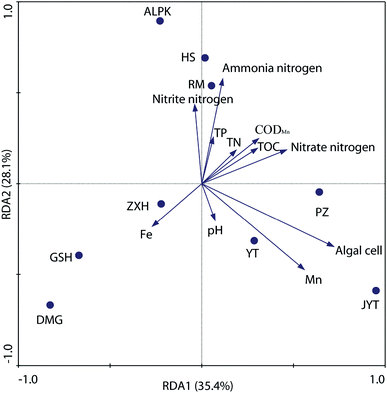 |
| Fig. 6 Redundancy analysis (RDA) of water denitrifying bacterial communities in nine geographically distributed urban lakes. RDA1 explained 35.4%, and RDA2 explained 28.1% of the total variance. Water quality parameters that significantly correlated with denitrifying bacterial community diversity were shown. | |
In addition, a variance partitioning analysis (VPA) was conducted to investigate the relative impacts of water quality and geographic location to the denitrifying bacterial communities. As shown in Fig. 7, water quality parameters and geographic locations explained 48.84% of the total variation, and 45.33% of the variation was unexplained. Thus, the water quality explained 21.28% (P = 0.026), whereas the geographic location explained 27.56% (P = 0.008) of the variation. Thus, both geographic distance and water quality are important factors in structuring denitrifying bacterial community compositions. This agreed with the finding of Yannarell and Triplett,48 who suggest that environmental, regional, temporal, and landscape level characteristics interact to shape the bacterial communities in northern temperate lakes. As mentioned before, another important source of change in this study was the algal cell concentration, which has been implicated by other researchers in study of nirS-type denitrifying bacterial community composition during a cyanobacterial bloom in a eutrophic shallow lake, and suggested that the community of nirS-type denitrifying bacteria shifted with the increase in cyanobacterial density.21 However, in this work, because algal cell concentration provides no algal community structure information and cyanobacterial density, it is not possible for the present study to address the effect of alga on diversity of nirS-type denitrifying bacteria directly.
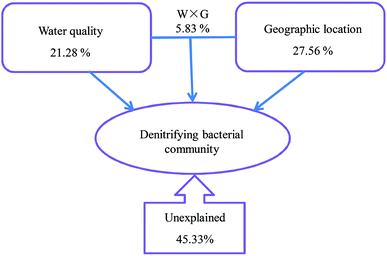 |
| Fig. 7 Variance partitioning analysis (VPA) of the effects of geographic distance and water quality parameters on the denitrifying bacterial communities. W × G represents interaction between water quality parameters and geographic location. | |
Previously, based on the literature that has focused on contemporary microbial biogeography, factors driving the distribution of aquatic denitrifying bacterial community are widely debated and poorly explored. In urban lakes, denitrifying bacteria play fundamental roles in urban lake ecosystems because they are the major drivers of nitrogen cycling, dissolved organic matter decomposition and energy flow. However, relatively little is known about the nirS-type denitrifying bacterial communities among urban lakes with different environmental conditions over a wide range of spatial scales. For that reason, it is crucial to understanding the biogeographically characteristics of urban lakes. Thus, works on the water denitrifying bacterial community of urban lakes and their graphical profiles are of great importance to assessing water microbial functions related to N cycling in these fresh water ecosystems. It is clear from the present work that denitrifying bacterial communities show non-random patterns in diversity and thus have variations in biogeography.
To the best of our knowledge, this study is the first to systematically depict the nirS-type denitrifying bacterial communities in urban lakes across large spatial scales. This highlights the importance of the spatial structure of the denitrifying bacterial community ecology in urban lakes. The denitrifying bacterial community composition we present here can also be useful in understanding the community assembly and coexistence among denitrifying bacterial communities associated with different geographically distributed urban lakes. Despite some of the shortcomings presented in this work, although some general conclusions can be drawn, the study of isolated denitrifying bacterial strains in pure culture conditions may lead to diverging conclusions. In the future, metagenome sequencing combined with a DNA stable isotope probing (DNA-SIP) experiment with an 15N-labeled substrate29 can be used to investigate the metabolic functional performance of the denitrifying bacterial community structure in urban lakes.
4. Conclusions
In summary, the study revealed the water quality in nine urban lakes were different among samples. The Illumina sequencing data indicated that 421
058 nirS gene sequence tags were generated and 6369 OTUs were identified. The majority of the OTUs were Proteobacteria, followed by Firmicutes. A co-occurrence network analysis indicated that the top five genera identified as keystone taxa were Dechlorospirillum sp., Alicycliphilus sp., Dechloromonas sp., Pseudogulbenkiania sp., and Paracoccus sp. RDA combined with VPA suggested that distinct denitrifying bacterial communities in nine urban lakes and that they were mainly shaped by geographic location, ammonia nitrogen, Mn and algal cell concentrations. Together, these results demonstrate that geographic location, coupled with water quality effects, can generate patterns in denitrifying bacterial communities across a variety of urban lakes and that these specific denitrifying bacterial species may drive the nitrogen cycling in urban lakes.
Conflicts of interest
The authors declare no conflict of interest.
Acknowledgements
This research was funded by the National Natural Science Foundation of China (Grant No. 51408466), the National Key Research and Development Program of China (Grant No. 2016YFC0400706), the grants from “Young Outstanding Talents” in General Universities of Shaanxi Province and “Yanta Outstanding Youth Scholar” of XAUAT, and the support from the Foundation of China Scholarship Council (CSC No. 201708610028).
References
- C. Bellard, C. Bertelsmeier, P. Leadley, W. Thuiller and F. Courchamp, Ecology Letters, 2012, 15, 365–377 CrossRef PubMed.
- Y. Sun, X. B. Zhang, G. Y. Ren, F. W. Zwiers and T. Hu, Nat. Clim. Change, 2016, 6, 706–709 CrossRef.
- C. L. Gu, L. Y. Wu and I. Cook, Frontiers of Architectural Research, 2012, 1, 101–149 CrossRef.
- L. Yang, K. M. Ma, J. Z. Zhao, X. Bai and Q. H. Guo, International Journal of Sustainable Development & World Ecology, 2007, 14, 317–327 Search PubMed.
- S. Yu, G. B. Yu, Y. Liu, G. L. Li, S. Feng, S. C. Wu and M. H. Wong, River Res. Appl., 2012, 28, 1135–1148 CrossRef.
- A. D. Kent, A. C. Yannarell, J. A. Rusak, E. W. Triplett and K. D. McMahon, ISME J., 2007, 1, 38–47 CrossRef CAS PubMed.
- H. H. Zhang, S. N. Chen, T. L. Huang, W. X. Ma, J. L. Xu and X. Sun, Int. J. Environ. Res. Public Health, 2015, 12, 6933–6945 CrossRef PubMed.
- X. Yang, T. L. Huang and H. H. Zhang, Water, 2015, 7, 5525–5546 CrossRef CAS.
- A. M. Ibekwe, J. Ma and S. E. Murinda, Sci. Total Environ., 2016, 566–567, 1176–1185 CrossRef CAS PubMed.
- H. Sekiguchi, M. Watanabe, T. Nakahara, B. H. Xu and H. Uchiyama, Appl. Environ. Microbiol., 2002, 68, 5142–5150 CrossRef CAS PubMed.
- H. H. Zhang, S. N. Chen, T. L. Huang, P. L. Shang, X. Yang and W. X. Ma, Int. J. Environ. Res. Public Health, 2015, 12, 13649–13661 CrossRef PubMed.
- J. M. Morrison, K. D. Baker, R. M. Zamor, S. Nikolai, M. S. Elshahed and N. H. Youssef, PLoS One, 2017, 12, e0177488 Search PubMed.
- J. Saarenheimo, S. L. Aalto, A. J. Rissanen and M. Tiirola, Front. Microbiol., 2017, 8, 750 CrossRef PubMed.
- E. Ahmed, L. Parducci, P. Unneberg, R. Ågren, F. Schenk, J. E. Rattray, L. Han, F. Muschitiello, M. W. Pedersen, R. H. Smittenberg, K. AfrifaYamoah, T. Slotte and B. Wohlfarth, Quat. Sci. Rev., 2018, 181, 19–29 CrossRef.
- K. Rojas-Jimenez, C. Wurzbacher, E. C. Bourne, A. Chiuchiolo, J. C. Priscu and H. P. Grossart, Sci. Rep., 2017, 7, 15348 CrossRef PubMed.
- M. H. Hengy, D. J. Horton, D. G. Uzarski and D. R. Learman, PeerJ, 2017, 5, e3937 Search PubMed.
- E. A. Franzosa, T. Hsu, A. Sirota-Madi, A. Shafquat, G. Abu-Ali, X. C. Morgan and C. Huttenhower, Nat. Rev. Microbiol., 2015, 13, 360–372 CrossRef CAS PubMed.
- I. Douterelo, J. B. Boxall, P. Deines, R. Sekar, K. E. Fish and C. A. Biggs, Water Res., 2014, 65, 134–156 CrossRef CAS PubMed.
- J. Boenigk, S. Wodniok, C. Bock, D. Beisser, C. Hempel, L. Grossmann, A. Lange and M. Jensen, Metabarcoding and Metagenomics, 2018, 2, e21519 Search PubMed.
- Y. A. Lipsewers, E. C. Hopmans, F. J. R. Meysman, J. S. Sinninghe-Damsté and L. Villanueva, Front. Microbiol., 2016, 7, 1661 Search PubMed.
- W. G. Zhang, Y. Gao, N. Yi, C. C. Wang, P. P. Di and S. H. Yan, J. Freshwater Ecol., 2017, 32, 467–476 CrossRef CAS.
- P. L. Kang, T. L. Huang, H. H. Zhang, S. N. Chen, P. L. Shang, J. Feng and J. Y. Jia, Environ. Sci., 2017, 38, 5174–5183 Search PubMed.
- X. P. Qiu, T. L. Huang and M. Z. Zeng, J. Freshwater Ecol., 2016, 31, 377–391 CrossRef CAS.
- J. Vierheilig, D. Savio, R. E. Ley, R. L. Mach, A. H. Farnleitner and G. H. Reischer, Water Sci. Technol., 2015, 72, 1962–1972 CrossRef CAS PubMed.
- W. Wei, K. Isobe, T. Nishizawa, L. Zhu, Y. Shiratori, N. Ohte, K. Koba, S. Otsuka and K. Senoo, ISME J., 2015, 9, 1954–1965 CrossRef CAS PubMed.
- B. F. Tan, C. Ng, J. P. Nshimyimana, L. L. Loh, K. Y. H. Gin and J. R. Thompson, Front. Microbiol., 2015, 6, 1027 Search PubMed.
- L. Shi, N. S. Du, S. Shu, J. Sun, S. Z. Li and S. R. Guo, Sci. Rep., 2017, 7, 41234 CrossRef CAS PubMed.
- J. G. Caporaso, J. Kuczynski, J. Stombaugh, K. Bittinger, F. D. Bushman, E. K. Costello, N. Fierer, A. G. Pena, J. K. Goodrich, J. I. Gordon, G. A. Huttley, S. T. Kelley, D. Knights, J. E. Koenig, R. E. Ley, C. A. Lozupone, D. McDonald, B. D. Muegge, M. Pirrung, J. Reeder, J. R. Sevinsky, P. J. Turnbaugh, W. A. Walters, J. Widmann, T. Yatsunenko, J. Zaneveld and R. Knight, Nat. Methods, 2010, 7, 335–336 CrossRef CAS PubMed.
- W. M. Sun, V. Krumins, Y. R. Dong, P. Gao, C. Y. Ma, M. Hu, B. Q. Li, B. Q. Xia, Z. J. He and S. L. Xiong, Microb. Ecol., 2018, 75, 113–122 CrossRef CAS PubMed.
- P. Legendre, J. Oksanen and C. J. F. T. Braak, Methods in Ecology and Evolution, 2011, 2, 269–277 CrossRef.
- J. Yang, H. C. Jiang, G. Wu, W. Liu and G. J. Zhang, Front. Microbiol., 2016, 7, 1728 Search PubMed.
- M. G. I. Andersson, M. Berga, E. S. Lindström and S. Langenheder, J. Ecol., 2014, 95, 1134–1140 CrossRef.
- K. Li, L. Wang, Z. H. Li, Y. J. Xie, X. R. Wang and Q. Fang, Water, 2017, 9, 707 CrossRef.
- J. D. Hosen, C. M. Febria, B. C. Crump and M. A. Palmer, Front. Microbiol., 2017, 8, 1452 CrossRef PubMed.
- A. C. Yannarell and E. W. Triplett, Appl. Environ. Microbiol., 2005, 71, 227–239 CrossRef CAS PubMed.
- N. Yi, Y. Gao, Z. H. Zhang, Y. Wang, X. H. Liu, L. Zhang and S. H. Yan, Int. J. Genomics, 2015, 2015, 572121 Search PubMed.
- F. Wang, Y. Zhao, S. L. Xie and J. Y. Li, Clean, Soil Air Water, 2017, 45, 1500319 CrossRef.
- X. F. Lv, J. B. Yu, Y. Q. Fu, B. Ma, F. Z. Qu, K. Ning and H. F. Wu, Sci. World J., 2014, 2014, 437684 Search PubMed.
- Y. Qin, J. Hou, M. Deng, Q. S. Liu, C. W. Wu, Y. J. Ji and X. G. He, Sci. Rep., 2016, 6, 35232 CrossRef CAS PubMed.
- L. M. Fan, C. Song, S. L. Meng, L. P. Qiu, Y. Zheng, W. Wu, J. H. Qu, D. D. Li, C. Zhang, G. D. Hu and J. Z. Chen, Sci. Rep., 2016, 6, 39147 CrossRef CAS PubMed.
- J. M. Beman, J. A. Steele and J. A. Fuhrman, ISME J., 2011, 5, 1077–1085 CrossRef CAS PubMed.
- F. Ju, Y. Xia, F. Guo, Z. P. Wang and T. Zhang, Environ. Microbiol., 2014, 16, 2421–2432 CrossRef CAS PubMed.
- S. Widder, K. Besemer, G. A. Singer, S. Ceola, E. Bertuzzo, C. Quince, W. T. Sloan, A. Rinaldo and T. J. Battin, Proc. Natl. Acad. Sci. U. S. A., 2014, 111, 12799–12804 CrossRef CAS PubMed.
- A. Eiler, F. Heinrich and S. Bertilsson, ISME J., 2012, 6, 330–342 CrossRef CAS PubMed.
- Y. Zhang, Y. Q. Liu, Z. J. Zhou, S. Y. Wang, X. Y. Cao, C. L. Song and Y. Y. Zhou, Fresenius Environ. Bull., 2016, 25, 5120–5129 CAS.
- A. C. Yannarell and E. W. Triplett, Appl. Environ. Microbiol., 2004, 70, 214–223 CrossRef CAS PubMed.
- J. B. Xiong, Y. Q. Liu, X. G. Lin, H. Y. Zhang, J. Zeng, J. Z. Hou, Y. P. Yang, T. D. Yao, R. Knight and H. Y. Chu, Environ. Microbiol., 2012, 14, 2457–2466 CrossRef CAS PubMed.
- A. C. Yannarell and E. W. Triplett, Appl. Environ. Microbiol., 2005, 71, 227–239 CrossRef CAS PubMed.
Footnote |
† Electronic supplementary information (ESI) available. See DOI: 10.1039/c8ra01295d |
|
This journal is © The Royal Society of Chemistry 2018 |