DOI:
10.1039/C7RA12259D
(Paper)
RSC Adv., 2018,
8, 5286-5297
Discovery of VEGFR2 inhibitors by integrating naïve Bayesian classification, molecular docking and drug screening approaches†
Received
9th November 2017
, Accepted 27th December 2017
First published on 30th January 2018
Abstract
The high morbidity and mortality of cancer make it one of the leading causes of global death, thus it is an urgent need to develop effective drugs for cancer therapy. Vascular endothelial growth factor receptor-2 (VEGFR2) acts as a central modulator of angiogenesis, and is therefore an important pharmaceutical target for developing anti-angiogenic agents. In this study, ligand-based naïve Bayesian (NB) models and structure-based molecular docking were combined to develop a virtual screening (VS) pipeline for identifying potential VEGFR2 inhibitors from FDA-approved drugs. The best validated naïve Bayesian model (NB-c) gave Matthews correlation coefficients of 0.966 and 0.951 for the test set and external validation set, respectively. 1841 FDA-approved drugs were sequentially screened by the optimal model NB-c and molecular docking module LibDock. By analyzing the results of VS, 9 top ranked drugs with EstPGood value ≥ 0.6 and LibDock Score ≥ 120 were chosen for biological validation. VEGFR2 kinase assay results demonstrated that flubendazole, rilpivirine and papaverine showed VEGFR2 inhibitory activities with IC50 values ranging from 0.47 to 6.29 μM. Binding mode analysis with CDOCKER revealed the action mechanism of the 3 hit drugs binding to VEGFR2. In summary, we not only proposed an integrated VS pipeline for potential VEGFR2 inhibitors screening, but also identified 3 FDA-approved drugs as novel VEGFR2 inhibitors, which could be used to design and develop new antiangiogenic agents.
1. Introduction
As a major public health problem, cancer is one of the leading causes of death worldwide. In 2017, it was estimated that 1
688
780 new cancer cases and 600
920 cancer deaths will occur in the United States.1 Recently, great advances have been achieved in the development of therapeutic agents for chemotherapy, hormone therapy and immunotherapy of cancer. However, the cancer mortality remains high due to the bewildering genetic and phenotypic heterogeneity of cancer, as well as the development of resistant phenotypes.2 Thus, it is urgent to develop new anticancer drugs to prolong survival, minimize chemotherapy side effects and improve quality of life for cancer patients.
Abnormal angiogenesis, a process by which new blood vessels sprout from pre-existing vessels, is well recognized as one common characteristic of various cancer types.3,4 By increasing the number of capillaries into the expanding tumor tissues, tumor-associated neo-vasculature is induced to accelerate tumorigenesis through aiding in nutrient supply and metastasis of tumor cells.5 VEGF/VEGFR2 signaling has been widely accepted for its pro-angiogenic role by dominating all steps of angiogenesis including survival, proliferation, migration and capillary-like tube formation of endothelial cells.6 Therefore, inhibition of VEGFR2 activity emerges as a potential therapy strategy against tumor-induced angiogenesis.7 Nowadays, several VEGFR2 inhibitors including sorafenib, sunitinib, pazopanib, vatalanib, axitinib, regorafenib and lenvatinib have been approved by Food and Drug Administration (FDA) for clinical therapy of cancers.8,9 Though the great success in anti-angiogenic therapy of cancers, the clinical usage of VEGFR2 inhibitors is always accompanied with side effects such as diarrhea, hypertension and hand-foot syndrome.10 Besides, emergence of drug resistance leads to a reduction in effectiveness of existing anti-angiogenic agents, thus discovering novel VEGFR2 inhibitors with less side effect and drug resistance is still in great demand.11,12
The currently available experimental screening assays for VEGFR2 inhibitors are labor-extensive, time-consuming and expense-costly. Therefore, it becomes necessary to improve screening efficiency by applying virtual screening (VS) in the lead identification of novel VEGFR2 inhibitors.13 Molecular docking, pharmacophore modeling and machine learning approaches are the most used methodologies in the virtual screening. Machine learning approaches possess robust abilities in separating inhibitors from noninhibitors by building classification models adopting statistical algorithms including linear discriminant analysis (LDA),14,15 support vector machine (SVM),16,17 naïve Bayesian (NB),17–21 recursive partitioning (RP),18 random forest (RF),22 and artificial neural network (ANN).23 SVM and NB models have been successfully applied in virtual screening of inhibitors against butyrylcholinesterase and neuraminidase in our group.17,19 To date, there have been some practical applications of VS in predicting VEGFR2 inhibitors. Zhang et al. proposed a fragment VS concept based on Bayesian categorization, and discovered 20 potential VEGFR-2 scaffolds.24 Besides, a multistep virtual screening protocol comprising ligand-based support vector machines, drug-likeness rules filter and structure-based molecular docking was developed and employed by Chen et al. to identify dual inhibitors of VEGFR2 and Src from a commercial chemical library.16 In another integrated VS with a sequential filter of Lipinski rules, structure-based pharmacophore modeling and molecular docking, 10 VEGFR2 inhibitors with IC50 values ranging from 1 to 10 μM were obtained by screening a commercial library of 82
000 compounds.25 However, by far, the combined application of NB classifiers and LibDock, a site-directed docking program, in VS of potential VEGFR2 inhibitors has not yet been studied.
One short-path approach for developing new drugs is drug repurposing or repositioning, by which existing approved drugs are repurposed for new targets or indications. Considering the known pharmacokinetics and pharmacodynamics of existing drugs, drug repurposing can boost drug discovery by shortening the time of experimental validation and improving the possibility of success.26 One good example of drug repurposing is thalidomide, which was originally launched as a sedative in 1957. Though thalidomide was withdrawn from the market in 1961 because of its severe teratogenic effects, it was later approved by FDA for treatment of erythema nodosum leprosum (ENL) in 1998. And then in 2006, the FDA further approved thalidomide for treatment of newly diagnosed multiple myeloma together with dexamethasone.27
In this study, the detailed workflow for VS of VEGFR2 is shown in Fig. 1. The goal of this study is to construct effective prediction models for VEGFR2 inhibitors, find novel VEGFR2 inhibitors from the approved drugs, therefore provide important information for drug design and new drug development for tumor treatment.
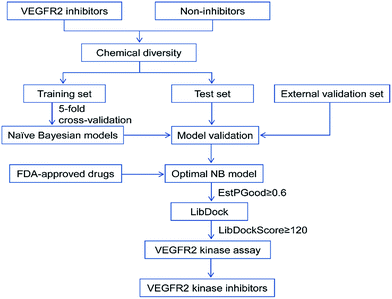 |
| Fig. 1 Workflow of the integrated virtual screening and drug screening approaches for VEGFR2 kinase inhibitors. | |
2. Materials and methods
2.1 Data collection and preparation
The whole date set of VEGFR2 inhibitors was primitively collected from the BindingDB database.28 The date set was then subjected to filtration with following criteria: (1) only compounds targeting human VEGFR2 was selected; (2) in order to make sure the potency of inhibitory activity, only the compounds with IC50 ≤ 10 μM were kept; (3) the duplicated compounds were abandoned. By applying these criteria, a total of 3464 VEGFR2 inhibitors with a large diversity in scaffolds and substituents were obtained. In addition, a date set of decoys (inactive compounds) were automatically generated online in DUD-E database.29 the 3464 inhibitors and 10
392 decoys were then randomly distributed into a training set and a test set at a ratio of 3
:
1. Finally, the training set was composed of 2598 inhibitors and 7794 decoys, and the test set included 866 inhibitors and 2598 decoys. In addition, a total of 82 VEGFR2 inhibitors (Ki ≤ 1000 nM) were collected from literatures and combined with 246 decoys, which are not included in the training set or test set, to form an external validation set. The activities of the inhibitors and decoys in the entire date set were labeled as “1” and “−1” respectively.
Ahead of molecular descriptors calculation, all compounds were prepared by washing and energy minimizing in MOE to remove inorganic counterions, add hydrogen atoms, deprotonate strong acids, protonate strong bases, generate stereoisomers and valid single 3D conformers. The detailed information of the training set, test set and external validation set is described in Fig. S1–S3.†
2.2 Molecular descriptors and fingerprints
A total of 300 molecular descriptors, including 18 AlogP, 163 Estate keys, 21 molecular properties, 55 molecular property counts, 10 surface area and volume, and 43 topological descriptors were calculated in DS 2016 (Discovery Studio version 2016, San Diego, CA, USA).
Besides the molecular descriptors mentioned above, two types of fingerprints named SciTegic extended-connectivity fingerprints (ECFP and FCFP) and Daylight-style path-based fingerprints (EPFP and FPFP) were also calculated in DS 2016. In order to represent the structural fragments in a moderate size, the diameters of each fingerprints were set to be 4 and 6, which were commonly used in ref. 17 and 30. In total, 8 fingerprints named ECFP_4, ECFP_6, EPFP_4, EPFP_6, FCFP_4, FCFP_6, FPFP_4, FPFP-6 were calculated.
2.3 Molecular descriptors selection
A molecular descriptors selection was conducted to find out those molecular descriptors that are highly correlated with activity. Firstly, the molecular descriptors whose values appear in high frequency of more than 50% were eliminated. Secondly, Pearson correlation analysis was performed to exclude the molecular descriptors whose correlation coefficients with activity were lower than 0.1. Once the correlation coefficient between two molecular descriptors was greater than 0.9, the molecular descriptor possessing a higher correlation coefficient with activity was kept. Finally, the remaining molecular descriptors were filtered by a stepwise linear regression, only the molecular descriptors kept in the regression equation were used for building naïve Bayesian models.
2.4 Naïve Bayesian model generation and validation
The NB models were created and validated in DS 2016. NB model is a probabilistic classification model based on Bayes' theorem, detailed information about NB method can be found in ref. 31. By utilizing the compounds in the training set, the NB models are built by learning to distinguish the inhibitors (active) from the decoys (inactive). In detail, the learning process generates a substantial set of Boolean features from the input descriptors. Then, it collects the frequency of occurrence of each feature in the inhibitor subset and in all compounds of the training set. To apply the model to a certain compound, the features of the compound are generated, and a weight is calculated for each feature using a Laplacian-adjusted probability estimate. The weights are summed to provide a probability estimate, which is a relative predictor of the likelihood of that compound being from the inhibitor subset.
The predictive performance of the NB model was evaluated by an internal 5-fold cross validation, as well as its performance on the test set and external validation set. Four evaluation indexes, including sensitivity (SE), specificity (SP), accuracy (Q), and Matthews correlation coefficient (MCC), were calculated using eqn (1)–(4). True positive (TP) represents the number of inhibitors that are correctly classified as active compounds. True negative (TN) represents the number of decoys that are correctly classified as inactive compounds. False positive (FP) represents the number of decoys that are incorrectly classified as active compounds. False negative (FN) represents the number of inhibitors that are incorrectly classified as inactive compounds.
|
 | (1) |
|
 | (2) |
|
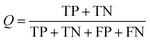 | (3) |
|
 | (4) |
While SE and SP represent the prediction accuracy for inhibitors and decoys respectively, Q indicates the overall prediction accuracy for all compounds in the dataset. MCC, with values ranging from −1 to 1, is the most important indicator for the quality of binary classification. In this study, the MCC value is considered as the main evaluation index.
2.5 Molecular docking
The crystal structure of VEGFR2, complexed with axitinib, was retrieved from the Protein Data Bank (PDB ID: 4AGC).32 The molecular docking studies were performed in DS 2016. For the preparation of the protein, the water and the co-crystallized axitinib were removed and hydrogens were added. The VEGFR2 protein was then further processed with the prepare protein module to model missing loop regions, calculate protein ionization and protonate the protein structure. The prepared protein was defined as the receptor and the binding site was defined from PDB site records with the define and edit binding site module. Finally, the binding site sphere (−23.5892, 1.24355, −12.8675, 10.1) of 4AGC was selected for molecular docking analysis. The reference compound axitinib and the selected compounds were docked into the binding site by utilizing the LibDock and CDOCKER modules. For the LibDock results, the docked pose with the highest LibDock Score was retained for each compound. Besides, the binding modes the docked compounds to the VEGFR2 protein were examined with the view interaction module.
2.6 In vitro VEGFR2 inhibitory assay
2.6.1 Reagents. Axitinib and flubendazole were purchased from Selleck Chemical (Houston, TX, USA). Other compounds including etofenamate, rabeprazole, rilpivirine, lansoprazole, dex-lansoprazole, omeprazole, pranlukast and papaverine were purchased from Target Molecule Corp. (Boston, MA, USA). All compounds were dissolved in dimethyl sulfoxide (DMSO) and stored at −20 °C. DMSO, MgCl2, MnCl2, dithiothreitol (DTT) and adenosine triphosphate (ATP) were obtained from Sigma (St. Louis, MO, USA). Human VEGFR2 (cytoplastic domain expressed as N-terminal GST-fusion protein) was purchased from Carna Biosciences (Kobe, Japan); The HTRF® KinEASETM-TK assay kit (62TK0PEB) was provided by Cisbio Bioassays (Parc Marcel Boiteux, 30200 Codolet, France).
2.6.2 VEGFR2 kinase assay. The VEGFR2 inhibitory assay was conducted with the HTRF KinEASE-TK assay as described previously.33 In detail, 2 μL VEGFR2 kinase (final conc. of 0.1 ng μL−1), 2 μL TK substrate-biotin (final conc. of 0.1 μM L−1), and 4 μL compounds were added to each well of the 96 well low-volume white plates (Cisbio), followed by addition of 2 μL ATP (final conc. of 0.8 μM L−1) to initiate the reaction. The enzymatic reaction was allowed to run for 15 min at 37 °C, then 5 μL streptavidin-XL665 (final conc. of 0.025 μM L−1), and 5 μL TK antibody-cryptate were added to stop the reaction. An Envision 2014 multi-label reader (PerkinElmer, Waltham, MA, USA) was used to measure the fluorescence of the samples at 615 nm (TK antibody-cryptate) and at 665 nm (streptavidin-XL665) after excitation at 320 nm. Then the inhibitory activity of the tested samples was calculated according to the following eqn (5)–(7). Assays were performed in triplicate, and IC50 values were determined by nonlinear regression models (log[inhibitor] vs. normalized response-variable slope) in GraphPad Prism 6 (GraphPad Software, San Diego, CA). |
Ratio = (665 nm/615 nm) × 104
| (5) |
|
Specific signal = ratio of sample − ratio of negative
| (6) |
|
Inhibitory rate (%) = (1 − specific signal of sample/specific signal of positive) × 100%
| (7) |
3. Results and discussion
3.1 Naïve Bayesian model generation and validation
3.1.1 Chemical space diversity analysis. Generally, the performance of machine learning classification models is largely affected by the chemical space diversity of compounds included in the datasets for model training and testing. A wide chemical space diversity of training set usually endows the classification model with high prediction accuracy and strong generalization ability. The chemical space diversity of the entire dataset was explored by principal component analysis (PCA) and Tanimoto similarity analysis.PCA was conducted on molecular descriptors including Molecular_Weight, ALogP, Num_H_Donors, Num_H_Acceptors, Num_RotatableBonds, Num_AromaticRings, Num_Rings, and Molecular_Fractional Polar Surface Area. As shown in Fig. 2, both the principal component 1 and 2 of the entire dataset are distributed in a wide range of space, indicating the chemical diversity of the training set, test set and external validation set.
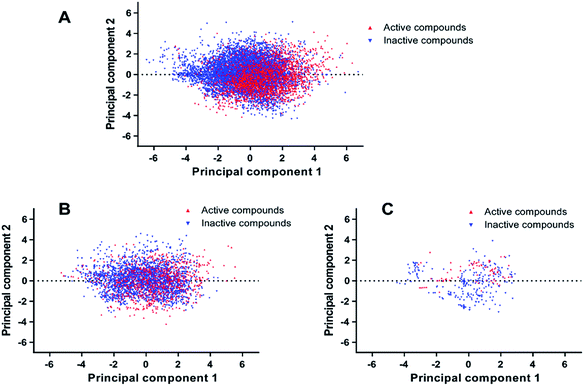 |
| Fig. 2 Chemical space analysis of the training set (A), test set (B), and external validation set (C) by principal component analysis (PCA). | |
The Tanimoto similarity analysis was another approach to investigate the diversity of compounds within the entire dataset.34 The Tanimoto coefficients (Tc) of the entire dataset were calculated with the fingerprint of ECFP_4 and summarized in Table 1. The Tc values of the training set, test set and external validation set range from 0.115 to 0.136. Consequently, we conclude that the entire dataset possesses enough chemical diversity.
Table 1 Detailed statistical description of the entire dataset
Dataset |
Inhibitors (active) |
Decoys (inactive) |
Total |
Tanimoto coefficient (ECFP_4) |
Training set |
2598 |
7764 |
10 392 |
0.115 |
Test set |
886 |
2598 |
3464 |
0.117 |
External validation set |
82 |
246 |
328 |
0.136 |
3.1.2 Performance of naïve Bayesian models. According to the molecular descriptor selection criteria, 16 discovery studio 2D descriptors (DS_2D_MP) and 8 molecular fingerprints (Table 2) were finally chosen for NB model construction. A total of 9 NB models were built by utilizing the training set with different combinations of molecular descriptors. For each NB model, an internal 5-fold cross-validation was performed by randomly partitioning the original training set into 5 equal sized subsets. One of the subsets was kept as a validation set to assess the model, which was created using the rest of the subsets. This process was then repeated 5 times with each subset used as the validation set in turn. Subsequently, the performance of the built NB models was further explored by predicting a test set comprising 886 inhibitors and 2598 inactive compounds.
Table 2 Molecular descriptors used in this study
Descriptor class |
Number of descriptors |
Descriptors |
Discovery studio 2D descriptors (DS_2D) |
16 |
Estate_AtomTypes, AlogP_AtomClassName, logD, Molecular_Solubility, HBA_Count, HBD_Count, Num_AromaticRings, Num_ChainAssemblies, Num_RingAssemblies, Num_StereoAtoms, Molecular_FractionalPolarSASA, Molecular_FractionalPolarSurfaceArea, Molecular_PolarSASA, CHI_V_3_C, Kappa_2, Wiener |
Molecular fingerprints |
8 |
ECFP_4, ECFP_6, EPFP_4, EPFP_6, FCFP_4, FCFP_6, FPFP_4, FPFP-6 |
The statistical results of 5-fold cross-validation and test set validation for the 9 NB models were listed in Table 3. The model NB-a built with 16 DS_2D descriptors showed a moderate performance with the accuracy (Q) of 0.868 and 0.833, the Matthews correlation coefficient (MCC) of 0.686 and 0.619 for the training set and test set, respectively.
Table 3 Performance of the 9 Bayesian models for the training set and test set using different combinations of molecular descriptors
Model |
Descriptors |
Number of descriptors |
Training set |
Test set |
SE |
SP |
Q |
MCC |
SE |
SP |
Q |
MCC |
NB-a |
DS_2D_MP |
16 |
0.872 |
0.866 |
0.868 |
0.686 |
0.853 |
0.826 |
0.833 |
0.619 |
NB-b |
MP + ECFP_4 |
17 |
0.985 |
0.992 |
0.991 |
0.975 |
0.982 |
0.984 |
0.983 |
0.956 |
NB-c |
MP + ECFP_6 |
17 |
0.988 |
0.997 |
0.995 |
0.985 |
0.982 |
0.989 |
0.987 |
0.966 |
NB-d |
MP + EPFP_4 |
17 |
0.937 |
0.932 |
0.933 |
0.833 |
0.937 |
0.904 |
0.912 |
0.789 |
NB-e |
MP + EPFP_6 |
17 |
0.962 |
0.956 |
0.957 |
0.891 |
0.960 |
0.918 |
0.928 |
0.828 |
NB-f |
MP + FCFP_4 |
17 |
0.979 |
0.978 |
0.978 |
0.944 |
0.961 |
0.962 |
0.961 |
0.901 |
NB-g |
MP + FCFP_6 |
17 |
0.987 |
0.990 |
0.990 |
0.973 |
0.971 |
0.987 |
0.983 |
0.955 |
NB-h |
MP + FPFP-4 |
17 |
0.952 |
0.948 |
0.949 |
0.870 |
0.938 |
0.920 |
0.924 |
0.815 |
NB-i |
MP + FPFP-6 |
17 |
0.955 |
0.973 |
0.968 |
0.917 |
0.958 |
0.931 |
0.938 |
0.847 |
The moderate performance of NB-a could be explained by the failure of DS_2D descriptors to characterize the important substructures and molecular fragments which are critical for VEGFR2 inhibition. Hence, DS_2D descriptors, combined with molecular fingerprints, were used simultaneously to establish NB models. As a result, the addition of fingerprints can improve the performance of the NB models, as the Q and MCC values of the NB models (NB-b ∼ NB-i) based on both DS_2D descriptors and molecular fingerprints are higher than those of NB-a, which is based on DS_2D descriptors only. Considering the length of the fingerprints, the NB models based on fingerprints of diameter 6 perform better than those based on fingerprints of diameter 4. For example, while NB-b (MP + ECFP_4) gives MCC values of 0.975 and 0.956 for the training set and test set, NB-c (MP + ECFP_6) outperforms NB-b with MCC values of 0.985 and 0.966 for the training set and test set, respectively. The same situation applies to other fingerprints, as shown by NB-d and NB-e, NB-f and NB-g, NB-h and NB-i. Additionally, among the fingerprints of diameter 6, ECFP_6 surpasses other fingerprints in establishing NB models, since NB-c (MP + ECFP_6) has the highest sensitivity (SE) value of 0.982, specificity (SP) value of 0.989, Q value of 0.987, and MCC value of 0.966 for the test set.
Since the compounds from the training set and test set share great similarity in chemical diversity, NB models developed by the training set may easily acquire a good value of Q and MCC for the test set. Therefore, a good performance on test set cannot necessarily guarantee a good predictive ability for compounds whose chemical structures differ greatly from those of the training set. To this end, 82 VEGFR2 inhibitors with Ki ≤ 1000 nM were extracted from literatures.35–40 Molecular scaffold analysis of the 82 VEGFR2 inhibitors resulted in six molecular scaffolds as summarized in Fig. S1.† Then six ECFP_4 based similarity search queries were used to find similar molecules for VEGFR2 inhibitors sharing the same scaffold from the 2598 inhibitors in the training set. While 45 similar molecules were retrieved for the inhibitors sharing scaffold 3, only 4 and 5 similar molecules were retrieved for the inhibitors sharing scaffold 1 and 6, respectively. It is worth noting that none similar molecules were retrieved for inhibitors sharing scaffold 2, 4 and 5. Thus, we conclude that the chemical structures of the 82 VEGFR2 inhibitors differ from those of the training set. The 82 VEGFR2 inhibitors was then combined with 246 decoys which are not included in the training and test sets to make up the external validation set.
The external validation set was used to further validate the predictive abilities of the 4 NB models (NB-b, NB-c, NB-f and NB-g) with MCC values higher than 0.9 for both training set and test set. The 4 NB models exhibit good performances for the external test set, with Q values ranging from 0.960 to 0.982, and MCC values ranging from 0.884 to 0.951 (Table 4). Finally, NB-c (MP + ECFP_6), with the highest Q value of 0.982 and MCC value of 0.951 for the external validation set, was selected as the optimal model and applied for further virtual screening of potential VEGFR2 inhibitors.
Table 4 Performance of the 4 Bayesian models for the external validation set using different combinations of molecular descriptors
Model |
Descriptors |
External validation set |
SE |
SP |
Q |
MCC |
NB-b |
MP + ECFP-4 |
0.939 |
0.976 |
0.967 |
0.911 |
NB-c |
MP + ECFP-6 |
0.963 |
0.988 |
0.982 |
0.951 |
NB-f |
MP + FCFP-4 |
0.890 |
0.980 |
0.960 |
0.884 |
NB-g |
MP + FCFP-6 |
0.896 |
0.992 |
0.970 |
0.914 |
3.1.3 Y-scrambling. Y-scrambling is a classical method for evaluating the chance correlation possibility of a predictive model. Herein, 40 times of Y-scrambling were used to validate that the good performance of the optimal model NB-c was not a result of chance correlation. The performance of the scrambled models and NB-c (unscrambled) on the test set and external validation set are summarized and visualized in Fig. 3. The 40 scrambled models have MCC values less than 0.3 and accuracy (Q) values less than 0.6 for both test set and external validation set. By comparison, the MCC and accuracy values of NB-c are much higher than those of the scrambled models, indicating that the optimal model NB-c is not built by chance.
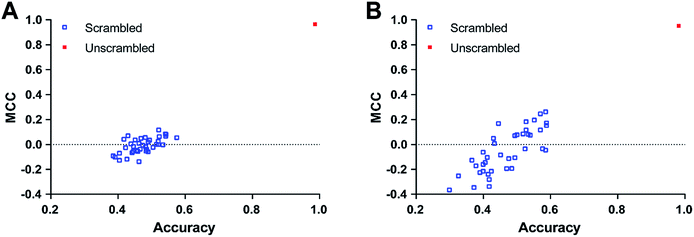 |
| Fig. 3 Y-scrambling results of NB-c. The MCC and accuracy values of the scrambled models (blue square) and NB-c (red square) on test set (A) and external validation set (B) are visualized. | |
3.1.4 Good and bad fragments given by NB model. The addition of fingerprint in NB model can not only improve the performance of the built model, but also aid in analyzing the good and bad fragments for compounds to be active against a certain target. The 15 good and 15 bad fragments with favorable and detrimental effects for inhibitory activities against VEGFR2 were given by NB-c (Fig. 4). All the fragments were ranked by the Bayesian scores assigned by NB-c. The fragment with a higher Bayesian score represent its higher occurrence probability in VEGFR2 inhibitors and more favorable contribution for certain compound to be VEGFR2 inhibitors.
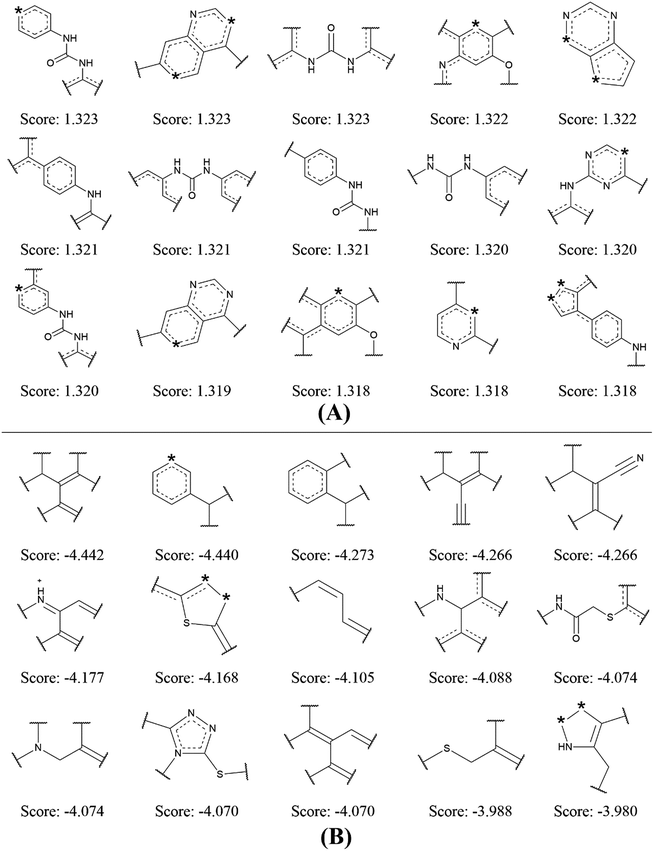 |
| Fig. 4 Examples of the top 15 good (A) and bad (B) fragments for VEGFR2 inhibition as estimated by NB-c model. The Bayesian score (Score) is given for each fragment. | |
By analyzing the 15 good fragments, we noticed that the majority of good fragments contain one or more nitrogen atoms. Some of these nitrogen atoms exist in saturated nitrogen-containing heterocycles, while others are linked with hexatomic rings or carbonyl groups. It is speculated that these nitrogen-containing fragments facilitate the competition of VEGFR2 inhibitors with ATP at the ATP binding-site of VEGFR2 by mimicking the adenine structure in ATP.41 Besides, the nitrogen atoms in these fragments can serve as hydrogen bond acceptors in forming hydrogen bond interactions with the kinase domain of VEGFR2. For example, the crystal structure of VEGFR2 in complex with axitinib shows that one nitrogen of the indazole scaffold forms a hydrogen bond with the N–H group of the cys919.32
It is interesting to notice that 4 out of the 15 bad fragments contain one sulphur atom, which is not found in the good fragments, indicating the existence of sulphur is unfavorable for VEGFR2 inhibition. For the rest 11 bad fragments, though one or more nitrogen atoms are found in 5 fragments, none of these nitrogen atoms is linked with hexatomic ring or carbonyl group, or exist in saturated nitrogen-containing heterocycle. The analysis on the favorable and unfavorable fragments for VEGFR2 inhibition will be beneficial for de novo molecular design and synthesis for novel VEGFR2 inhibitors.
3.2 Validation of molecular docking
Molecular docking has been widely used for structure-based virtual screening, as well as exploring the binding modes of small molecules within protein–ligand complexes. Herein, molecular docking analysis were performed for VEGFR2 by applying two docking algorithms named LibDock and CDOCKER. LibDock, a site-feature docking algorithm developed by Diller and Merz, can easily provide a rapid virtual screening campaign on millions of compounds by matching the physicochemical properties of the compounds to the polar and apolar features in the protein binding sites.42 CDOCKER is another powerful docking method, which is CHARMm-based and has been validated for generating highly accurate docked poses.43
The ligand axitinib was extracted from the VEGFR2 crystal structure (PDB ID: 4AGC), and then re-docked into the active site of VEGFR2. As shown in Fig. 5, the docked ligand poses obtained by the two docking methods can be well aligned with the ligand in the crystallographic complex. The root-mean-square distance (RMSD) values between the docked poses and the corresponding binding pose in the crystallographic complex were 1.05 and 0.43 for LibDock and CDOCKER respectively, indicating the high accuracy and reliability of the docking methods.
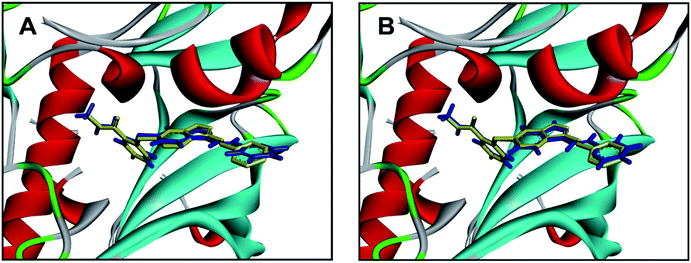 |
| Fig. 5 Alignment of the docked ligand poses (shown in blue) by LibDock (A) and CDOCKER (B), with the corresponding binding pose (shown in yellow) in the crystallographic complex. | |
3.3 Validation of the integrated VS pipeline with FDA-approved anti-angiogenic drugs
Since each individual VS method has its own advantages and disadvantages, therefore, we combined the ligand-based NB-c with structure-based LibDock in a sequential manner to form an integrated VS pipeline for lead identification of VEGFR2 inhibitors. The predictive ability of the integrated VS pipeline was evaluated by its performance on the 8 VEGFR2-targeting antiangiogenic agents approved by FDA (Table 5). In detail, each antiangiogenic agent was subjected to activity prediction by NB-c, followed by molecular docking analysis with LibDock. As a probabilistic model based on Bayes's theorem, the Bayesian categorization model not only predicts “True” or “False”, but also gives the estimated probability (EstPGood) that a compound is in the active class. Normally, the compound assigned with a higher value of EstPGood (0 ≤ EstPGood ≤ 1) has a greater potential of being active against certain target. As a result, all the 8 antiangiogenic agents were predicted to be active against VEGFR2 by NB-c and successfully docked into the active site of VEGFR2 by LibDock, with EstPGood values ≥ 0.95 and LibDock Scores ≥ 120. The above results demonstrated the ability of the integrated VS pipeline in identifying VEGFR2 inhibitors.
Table 5 The performance of the integrated VS pipeline on the FDA-approved antiangiogenic agents
Drugbank ID |
Drug name |
Structure |
Company |
LibDock Score |
Bayesian model (NB-c) |
EstPGood |
Prediction |
DB00398 |
Sorafenib |
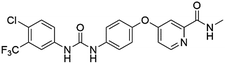 |
Bayer |
148.031 |
1 |
True |
DB01268 |
Sunitinib |
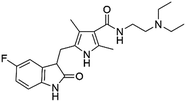 |
Pfizer |
125.116 |
0.978 |
True |
DB06589 |
Pazopanib |
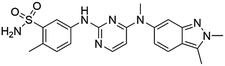 |
GSK |
136.442 |
0.986 |
True |
DB05294 |
Vandetanib |
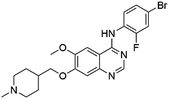 |
AstraZeneca |
126.119 |
0.997 |
True |
DB06626 |
Axitinib |
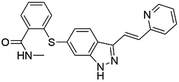 |
Pfizer |
144.994 |
0.953 |
True |
DB08896 |
Regorafenib |
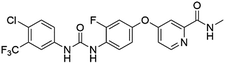 |
Bayer |
139.278 |
1 |
True |
DB08875 |
Cabozantinib |
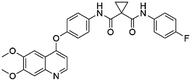 |
Exelixis |
127.297 |
1 |
True |
DB09078 |
Lenvatinib |
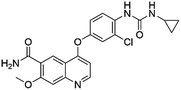 |
Eisai |
140.146 |
0.979 |
True |
3.4 Virtual screening of VEGFR2 inhibitors
With the aim of repurposing FDA-approved drugs as novel antiangiogenic agents, we applied the integrated VS pipeline on a dataset of 1841 FDA-approved drugs, which were collected from the Drugbank database and haven't yet been recorded for their VEGFR2 inhibitory activities.44
Initially, 120 drugs were predicted to be active against VEGFR2 by NB-c. In the following molecular docking analysis with LibDock, 95 out of the 120 hit drugs by NB-c were successfully docked into the active site of the VEGFR2. The detailed prediction results of the 95 drugs passing through the integrated VS pipeline are summarized in Fig. S1.† Besides, we visualized the predicted results of the 95 drugs and 8 antiangiogenic agents by plotting the EstPGood value to the LibDock Score. As shown in Fig. 6, some drugs possess high probabilities of VEGFR2 inhibitory activities, as indicated by relatively high EstPGood value and LibDock Score. Finally, the 9 top ranked drugs with EstPGood value ≥ 0.6 and LibDock Score ≥ 120 were selected for final biological validation (Table 6).
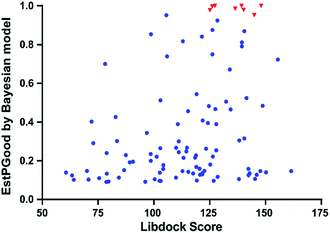 |
| Fig. 6 EstPGood values and LibDock Scores of FDA-approved drugs as predicted by NB-c and LibDock. While the 8 VEGFR2-targeting antiangiogenic agents are represented in red triangles, other drugs are represented in blue squares. | |
Table 6 Detailed prediction results of the 9 drugs selected for biological validation
Drugbank ID |
Drug name |
Structure |
FDA indication |
LibDock Score |
Bayesian model (NB-c) |
EstPGood |
Prediction |
DB08984 |
Etofenamate |
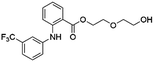 |
Anti-inflammatory |
128.425 |
0.924 |
True |
DB08974 |
Flubendazole |
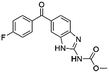 |
Anthelmintic |
126.236 |
0.875 |
True |
DB01129 |
Rabeprazole |
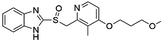 |
Anti-ulcer |
140.515 |
0.869 |
True |
DB08864 |
Rilpivirine |
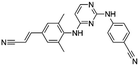 |
Anti-HIV |
121.471 |
0.841 |
True |
DB00448 |
Lansoprazole |
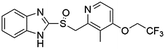 |
Anti-ulcer |
139.411 |
0.812 |
True |
DB05351 |
Dex-lansoprazole |
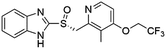 |
Anti-ulcer |
139.411 |
0.791 |
True |
DB00338 |
Omeprazole |
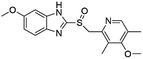 |
Anti-ulcer |
126.228 |
0.749 |
True |
DB01411 |
Pranlukast |
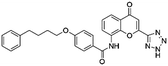 |
Anti-asthmatic |
155.53 |
0.723 |
True |
DB01113 |
Papaverine |
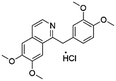 |
Vasodilator |
133.967 |
0.671 |
True |
3.5 In Vitro VEGFR2 inhibitory assay
Homogeneous time-resolved fluorescence (HTRF) based VEGFR2 kinase assays were performed for biological validation of the 9 selected drugs, with axitinib served as the reference compound. The experimental IC50 value of axitinib was 2.82 nM, which was in consistence with the previous study.25 Three out of the 9 tested drugs, namely, flubendazole, rilpivirine and papaverine were found to inhibit enzymatic activity of VEGFR2 at micromolar levels. Inhibitory curves and IC50 values of the 3 hit drugs and reference compound axitinib against VEGFR2 are presented in Fig. 7.
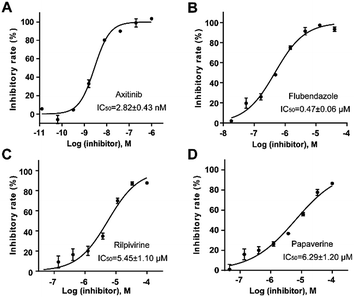 |
| Fig. 7 Inhibitory curves and IC50 values for the reference compound axitinib (A) and three FDA-approved drugs flubendazole (B), rilpivirine (C), papaverine (D) against VEGFR2. | |
Flubendazole was identified as the most potent inhibitor in this study, with IC50 value of 0.47 μM against VEGFR2. As a synthetic anthelmintic compound, flubendazole has been widely used in human and veterinary medicine against parasitic worms. In recent years, flubendazole has attracted great attention of scientists for its antiproliferative activities against various malignant types including leukemia, myeloma, neuroblastoma, breast cancer, colorectal cancer and melanoma.45–47 However, the antiangiogenic potential of flubendazole has been little studied. The validation of flubendazole as VEGFR2 inhibitor support its potential usage in anticancer therapy. Rilpivirine, a non-nucleoside reverse transcriptase inhibitor, has been approved for the treatment of HIV-1 infection in treatment-naïve adults in combination with other antiretroviral agents.48 By scaffold analyzing of the 3 hit drugs, the scaffold of rilpivirine turned out to be novel scaffold against VEGFR2, which meant the established VS pipeline was capable of identify new VEGFR2 inhibitor with novel scaffold.
Considering the inherent advantages and disadvantages of each single VS method, it is proposed to strengthen the robustness of VS by integrating different VS strategies either in a parallel or sequential manner. Zhang et al. developed an integrated two-layer workflow for VS of VEGFR2 inhibitors by using a 2D fingerprints similarity search followed by molecular docking and then a strict multi-complex pharmacophore screening. The effectiveness of the integrated VS method was then validated by a retrospective comparison, in which it outperformed 43 out of 45 methods.49 In the present study, a hit rate of 33% (3 out of 9) was achieved by the integrated VS pipeline combining ligand-based naïve Bayesian model and structure-based molecular docking. We expect to further apply the integrated VS pipeline to other commercial available compound libraries for potential VEGFR2 inhibitors.
3.6 Docking studies and proposed binding mode of the identified VEGFR2 inhibitors
Docking studies with CDOCKER method were performed to explore the binding modes of the identified inhibitors to the VEGFR2 protein. By analyzing the receptor–ligand interactions with the docking results, all the 3 identified VEGFR2 inhibitors retained the important common binding features for the reference compound axitinib (Fig. 8).
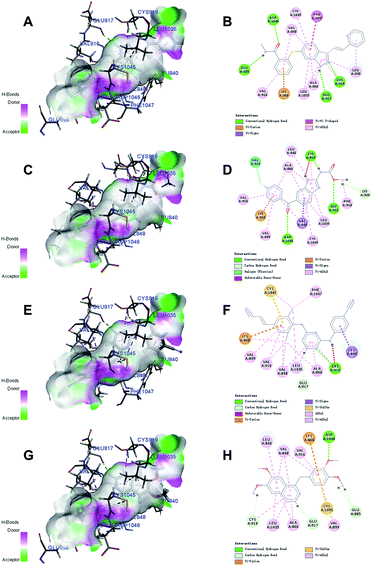 |
| Fig. 8 The receptor–ligand interactions of the axitinib (A, B), flubendazole (C, D), rilpivirine (E, F), papaverine (G, H) with the active site of VEGFR2. | |
While the head group amide substituent of axitinib formed two conventional hydrogen bonds with amino acids Asp1046 and Glu885, its indazole scaffold formed another two hydrogen bonds with Glu917 and Cys919.32 Other interactions of axitinib included Pi–cation interaction with Lys868, Pi–Pi stacked interactions with Phe1047, and Pi–alkyl interactions with Cys1045, Leu1035, Val916, Ala866, Val848, Leu840. As for flubendazole, rilpivirine and papaverine, they all formed Pi–cation interaction with Lys868, and similar Pi–alkyl interactions as axitinib. Moreover, flubendazole formed three conventional hydrogen bonds with Asp1046, Gly922, Cys919 and one additional carbon hydrogen bond with Lys920. Rilpivirine formed one conventional hydrogen bond with Cys919 and one carbon hydrogen bond with Glu917. Papaverine was found to interact with Asp1046 via one conventional hydrogen bond, as well as Cys919, Glu917 and Glu885 via carbon hydrogen bonds.
4. Conclusions
In this study, we constructed an integrated VS pipeline for VEGFR2 inhibitors screening by combining the ligand-based NB models and structure-based molecular docking. The integrated VS pipeline was validated for its excellent predictive accuracy by identifying 8 FDA-approved antiangiogenic agents. For the biological validation of the 9 hits selected on the basis of EstPGood value and LibDock Score, three drugs named flubendazole, rilpivirine and papaverine were identified as VEGFR2 inhibitors with IC50 values ranging from 0.47 to 6.29 μM. The established classification models can be used for further screening of available compound libraries, and the identification of 3 hit drugs as novel VEGFR2 inhibitors provided powerful insight into the repurposing of existing drugs as antiangiogenic agents.
Conflicts of interest
There are no conflicts to declare.
Acknowledgements
This research work was financially supported by grants from National Great Science and Technology Projects (No. 2013ZX09402203, 2014ZX09507003002), CAMS Innovation Fund for Medical Sciences (CIFMS) (No. 2016-I2M-3-007), and National population and health scientific data sharing platform (No. 2016-NCMI-ZX-05, NCMI-AGE05-201609).
References
- R. L. Siegel, K. D. Miller and A. Jemal, Ca-Cancer J. Clin., 2017, 67, 7–30 CrossRef PubMed.
- D. Urbach, M. Lupien, M. R. Karagas and J. H. Moore, Trends Genet., 2012, 28, 538–543 CrossRef CAS PubMed.
- D. Hanahan and R. A. Weinberg, Cell, 2011, 144, 646–674 CrossRef CAS PubMed.
- N. Ferrara and R. S. Kerbel, Nature, 2005, 438, 967–974 CrossRef CAS PubMed.
- G. Tortora, D. Melisi and F. Ciardiello, Curr. Pharm. Des., 2004, 10, 11–26 CrossRef CAS PubMed.
- A. S. Chung, J. Lee and N. Ferrara, Nat. Rev. Cancer, 2010, 10, 505–514 CrossRef CAS PubMed.
- V. Granci, Y. M. Dupertuis and C. Pichard, Curr. Opin. Clin. Nutr. Metab. Care, 2010, 13, 417–422 CrossRef CAS PubMed.
- N. Ferrara and A. P. Adamis, Nat. Rev. Drug Discovery, 2016, 15, 385–403 CrossRef CAS PubMed.
- F. W. Peng, D. K. Liu, Q. W. Zhang, Y. G. Xu and L. Shi, Expert Opin. Ther. Pat., 2017, 27, 987–1004 CrossRef CAS PubMed.
- N. Bhojani, C. Jeldres, J. J. Patard, P. Perrotte, N. Suardi, G. Hutterer, F. Patenaude, S. Oudard and P. I. Karakiewicz, Eur. Urol., 2008, 53, 917–930 CrossRef CAS PubMed.
- Y. Haga, T. Kanda, M. Nakamura, S. Nakamoto, R. Sasaki, K. Takahashi, S. Wu and O. Yokosuka, PLoS One, 2017, 12, e0174153 Search PubMed.
- N. Yamaguchi, M. Osaki, K. Onuma, T. Yumioka, H. Iwamoto, T. Sejima, H. Kugoh, A. Takenaka and F. Okada, Anticancer Res., 2017, 37, 2985–2992 Search PubMed.
- P. M. Hoi, S. Li, C. T. Vong, H. H. Tseng, Y. W. Kwan and S. M. Lee, Methods, 2015, 71, 85–91 CrossRef CAS PubMed.
- A. Speck-Planche and M. Cordeiro, Mol. Diversity, 2017, 21, 511–523 CrossRef CAS PubMed.
- A. Speck-Planche and M. N. Dias Soeiro Cordeiro, ACS Comb. Sci., 2017, 19, 501–512 CrossRef CAS PubMed.
- S. Chen, C. Qin, J. E. Sin, X. Yang, L. Tao, X. Zeng, P. Zhang, C. M. Gao, Y. Y. Jiang, C. Zhang, Y. Z. Chen and W. K. Chui, Future Med. Chem., 2017, 9, 7–24 CrossRef CAS PubMed.
- J. Fang, R. Yang, L. Gao, D. Zhou, S. Yang, A. L. Liu and G. H. Du, J. Chem. Inf. Model., 2013, 53, 3009–3020 CrossRef CAS PubMed.
- J. Fang, Y. Li, R. Liu, X. Pang, C. Li, R. Yang, Y. He, W. Lian, A. L. Liu and G. H. Du, J. Chem. Inf. Model., 2015, 55, 149–164 CrossRef CAS PubMed.
- W. Lian, J. Fang, C. Li, X. Pang, A. L. Liu and G. H. Du, Mol. Diversity, 2016, 20, 439–451 CrossRef CAS PubMed.
- J. Fang, R. Yang, L. Gao, S. Yang, X. Pang, C. Li, Y. He, A. L. Liu and G. H. Du, Mol. Diversity, 2015, 19, 149–162 CrossRef CAS PubMed.
- J. Fang, X. Pang, R. Yan, W. Lian, C. Li, Q. Wang, A.-L. Liu and G.-H. Du, RSC Adv., 2016, 6, 9857–9871 RSC.
- T. M. Ehrman, D. J. Barlow and P. J. Hylands, J. Chem. Inf. Model., 2007, 47, 264–278 CrossRef CAS PubMed.
- G. M. Keseru, L. Molnar and I. Greiner, Comb. Chem. High Throughput Screening, 2000, 3, 535–540 CrossRef CAS PubMed.
- Y. Zhang, Y. Jiao, X. Xiong, H. Liu, T. Ran, J. Xu, S. Lu, A. Xu, J. Pan, X. Qiao, Z. Shi, T. Lu and Y. Chen, Mol. Diversity, 2015, 19, 895–913 CrossRef CAS PubMed.
- K. Lee, K. W. Jeong, Y. Lee, J. Y. Song, M. S. Kim, G. S. Lee and Y. Kim, Eur. J. Med. Chem., 2010, 45, 5420–5427 CrossRef CAS PubMed.
- N. T. Issa, S. W. Byers and S. Dakshanamurthy, Curr. Top. Med. Chem., 2013, 13, 2328–2336 CrossRef CAS PubMed.
- L. Sleire, H. E. Forde-Tislevoll, I. A. Netland, L. Leiss, B. S. Skeie and P. O. Enger, Pharmacol. Res., 2017, 124, 74–91 CrossRef CAS PubMed.
- T. Liu, Y. Lin, X. Wen, R. N. Jorissen and M. K. Gilson, Nucleic Acids Res., 2007, 35, D198–D201 CrossRef CAS PubMed.
- M. M. Mysinger, M. Carchia, J. J. Irwin and B. K. Shoichet, J. Med. Chem., 2012, 55, 6582–6594 CrossRef CAS PubMed.
- L. Wang, L. Chen, Z. Liu, M. Zheng, Q. Gu and J. Xu, PLoS One, 2014, 9, e95221 Search PubMed.
- A. Bender, Methods Mol. Biol., 2011, 672, 175–196 CAS.
- M. McTigue, B. W. Murray, J. H. Chen, Y. L. Deng, J. Solowiej and R. S. Kania, Proc. Natl. Acad. Sci. U. S. A., 2012, 109, 18281–18289 CrossRef CAS PubMed.
- T. T. Zhou, L. He, M. Yan, L. Y. Zhang, J. G. He and X. P. Rao, Chin. J. Nat. Med., 2013, 11, 506–513 CrossRef CAS PubMed.
- D. Bajusz, A. Racz and K. Heberger, J. Cheminf., 2015, 7, 20 Search PubMed.
- M. H. Potashman, J. Bready, A. Coxon, T. M. DeMelfi Jr, L. DiPietro, N. Doerr, D. Elbaum, J. Estrada, P. Gallant, J. Germain, Y. Gu, J. C. Harmange, S. A. Kaufman, R. Kendall, J. L. Kim, G. N. Kumar, A. M. Long, S. Neervannan, V. F. Patel, A. Polverino, P. Rose, S. Plas, D. Whittington, R. Zanon and H. Zhao, J. Med. Chem., 2007, 50, 4351–4373 CrossRef CAS PubMed.
- M. Pannala, S. Kher, N. Wilson, J. Gaudette, I. Sircar, S. H. Zhang, A. Bakhirev, G. Yang, P. Yuen, F. Gorcsan, N. Sakurai, M. Barbosa and J. F. Cheng, Bioorg. Med. Chem. Lett., 2007, 17, 5978–5982 CrossRef CAS PubMed.
- C. Dominguez, L. Smith, Q. Huang, C. Yuan, X. Ouyang, L. Cai, P. Chen, J. Kim, T. Harvey, R. Syed, T. S. Kim, A. Tasker, L. Wang, M. Zhang, A. Coxon, J. Bready, C. Starnes, D. Chen, Y. Gan, S. Neervannan, G. Kumar, A. Polverino and R. Kendall, Bioorg. Med. Chem. Lett., 2007, 17, 6003–6008 CrossRef CAS PubMed.
- M. Krug, G. Erlenkamp, W. Sippl, C. Schachtele, F. Totzke and A. Hilgeroth, Bioorg. Med. Chem. Lett., 2010, 20, 6915–6919 CrossRef CAS PubMed.
- L. Liu, M. H. Norman, M. Lee, N. Xi, A. Siegmund, A. A. Boezio, S. Booker, D. Choquette, N. D. D'Angelo, J. Germain, K. Yang, Y. Yang, Y. Zhang, S. F. Bellon, D. A. Whittington, J. C. Harmange, C. Dominguez, T. S. Kim and I. Dussault, J. Med. Chem., 2012, 55, 1868–1897 CrossRef CAS PubMed.
- J. K. Kawakami, Y. Martinez, B. Sasaki, M. Harris, W. E. Kurata and A. F. Lau, Bioorg. Med. Chem. Lett., 2011, 21, 1371–1375 CrossRef CAS PubMed.
- S. J. Boyer, Curr. Top. Med. Chem., 2002, 2, 973–1000 CrossRef CAS PubMed.
- D. J. Diller and K. M. Merz Jr, Proteins, 2001, 43, 113–124 CrossRef CAS.
- G. Wu, D. H. Robertson, C. L. Brooks III and M. Vieth, J. Comput. Chem., 2003, 24, 1549–1562 CrossRef CAS PubMed.
- D. S. Wishart, C. Knox, A. C. Guo, D. Cheng, S. Shrivastava, D. Tzur, B. Gautam and M. Hassanali, Nucleic Acids Res., 2008, 36, D901–D906 CrossRef CAS PubMed.
- Z. J. Hou, X. Luo, W. Zhang, F. Peng, B. Cui, S. J. Wu, F. M. Zheng, J. Xu, L. Z. Xu, Z. J. Long, X. T. Wang, G. H. Li, X. Y. Wan, Y. L. Yang and Q. Liu, Oncotarget, 2015, 6, 6326–6340 Search PubMed.
- M. Michaelis, B. Agha, F. Rothweiler, N. Loschmann, Y. Voges, M. Mittelbronn, T. Starzetz, P. N. Harter, B. A. Abhari, S. Fulda, F. Westermann, K. Riecken, S. Spek, K. Langer, M. Wiese, W. G. Dirks, R. Zehner, J. Cinatl, M. N. Wass and J. Cinatl Jr, Sci. Rep., 2015, 5, 8202 CrossRef PubMed.
- K. Canova, L. Rozkydalova and E. Rudolf, Acta Med., 2017, 60, 5–11 Search PubMed.
- M. Sharma and L. D. Saravolatz, J. Antimicrob. Chemother., 2013, 68, 250–256 CrossRef CAS PubMed.
- Y. Zhang, S. Yang, Y. Jiao, H. Liu, H. Yuan, S. Lu, T. Ran, S. Yao, Z. Ke, J. Xu, X. Xiong, Y. Chen and T. Lu, J. Chem. Inf. Model., 2013, 53, 3163–3177 CrossRef CAS PubMed.
Footnote |
† Electronic supplementary information (ESI) available. See DOI: 10.1039/c7ra12259d |
|
This journal is © The Royal Society of Chemistry 2018 |
Click here to see how this site uses Cookies. View our privacy policy here.