DOI:
10.1039/C8LC00746B
(Critical Review)
Lab Chip, 2018,
18, 3333-3352
Caring for cells in microsystems: principles and practices of cell-safe device design and operation
Received
18th July 2018
, Accepted 8th October 2018
First published on 16th October 2018
Abstract
Microfluidic device designers and users continually question whether cells are ‘happy’ in a given microsystem or whether they are perturbed by micro-scale technologies. This issue is normally brought up by engineers building platforms, or by external reviewers (academic or commercial) comparing multiple technological approaches to a problem. Microsystems can apply combinations of biophysical and biochemical stimuli that, although essential to device operation, may damage cells in complex ways. However, assays to assess the impact of microsystems upon cells have been challenging to conduct and have led to subjective interpretation and evaluation of cell stressors, hampering development and adoption of microsystems. To this end, we introduce a framework that defines cell health, describes how device stimuli may stress cells, and contrasts approaches to measure cell stress. Importantly, we provide practical guidelines regarding device design and operation to minimize cell stress, and recommend a minimal set of quantitative assays that will enable standardization in the assessment of cell health in diverse devices. We anticipate that as microsystem designers, reviewers, and end-users enforce such guidelines, we as a community can create a set of essential principles that will further the adoption of such technologies in clinical, translational and commercial applications.
Introduction
A cell's health and biological function are closely regulated by its microenvironment. Adverse perturbations caused by external stimuli (e.g., injury, molecular signals) can steer cellular homeostasis towards a dysfunctional state. To this end, countless platforms and technologies (including microfluidics) have been developed to gain insight into disease biology, as well as for engineering diagnostics and therapeutics. In their application, these devices typically leverage a variety of physical forces and biochemical factors to study and manipulate a broad range of cell types.1,2 Paradoxically, the device microenvironment itself may inevitably impose undesirable changes upon cell health, thereby biasing or invalidating the device's utility. However, how does one avoid unintended device-imposed biological artifacts that can bias results from the intended biological study and application? In other words, how does one design and maintain the device microenvironment in a way that does not stress or harm cells? In this review, our objective is to address these questions and provide practical guidelines for designing and using devices in ways that lower cell damage; thus, negating device-imposed biological artefacts and extending the utility of the device to the broader community.
First, we will define cell health and how various perturbations can steer cells to a stressed state or towards cell death. Next, we will outline common considerations for device design and operating conditions that should help in maintaining viable cells. These considerations should assist the device designer in keeping ‘device-treated’ cells alive and appearing similar to the appropriate reference cells (e.g. cells cultured by standard protocols). With such precautions such cells may appear healthy, however they may still be stressed in ways not always obvious to the end-user. For those interested in maintaining healthy (and not just viable) cells, in the latter part of this review, we recommend quantitative assays that can be used to measure and minimize cell stress within the context of microsystems.
Cell health and stress responses
For a microsystem engineer or user, the first concern is to ensure that their particular platform does not kill cells. Cell death is commonly observed as a decrease in cell numbers due to the exposure to a device environment, while cell damage is commonly inferred through changes in cell morphology or function. However, cell death can be masked by proliferation of surviving cells and ‘washing off’ or disintegration of dead cells. Similarly, activation of cell death mechanisms (e.g. apoptosis) or stress mechanisms may not be reflected in cell morphology or proliferation. In this way, common inferences made by the device designers may be both inaccurate and misrepresentative of cell health. Hence, before describing what can help maintain live and healthy cells, we will first describe to the device designers what it means for a cell to be healthy.
A dysfunctional or damaged cell is an undesirable consequence that needs to be prevented to maintain a healthy physiological state. However, what this healthy state refers to is dependent on a few important aspects. Cells in vivo provide unique functionality through specialized processes, which are tied to their phenotype and microenvironment. While the in vivo purpose and functions may differ among cells, they are all susceptible to stress damage in similar ways. Specifically, stressors can inflict injury to cell membranes, ATP generation processes, protein synthesis and genome integrity and replication processes- all of which can influence the other. We define these as essential processes that are relevant to all cells. The baseline ‘activity’ of each of these essential and specialized processes collectively defines what we will term the “healthy state” of a particular cell (Fig. 1A). Importantly, these states will differ between the natural in vivo microenvironment and typical in vitro culture conditions and depending on the cellular phenotype and origin, the relevant reference must be considered as the healthy state. Additionally, within in vitro culture, primary cells and transformed cell lines (including cancer cell lines) will maintain distinct homeostatic equilibria for each of the essential cellular processes. For cancer or diseased cells this equilibrium is defined as the healthy state, even though it may in fact be associated with disease in vivo. With this context, a stressor is defined as a stimulus that steers the cell away from its preexisting equilibrium healthy state. For example, any perturbation which causes ATP depletion, loss of ionic homeostasis (Ca++, Na+, K+, etc.), generation of reactive free radicals, mitochondrial damage, pH imbalance, defects in membrane permeability, genetic mutations, is a stressor.
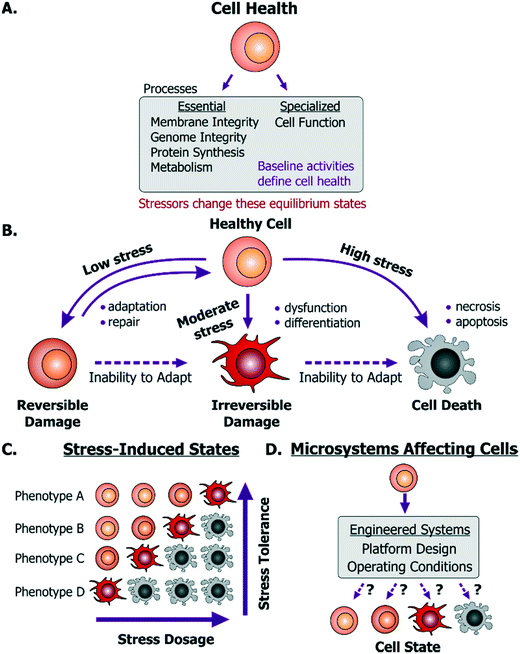 |
| Fig. 1 Cell health and its responses to stressors. A. Cell health is defined as the collective equilibrium activities of essential and specialized cellular processes; while a cell stressor is defined as a stimulus that causes excursion from its equilibrium state. B. Emergent cell states following exposure to low to high stress dosages. C. A cell's base phenotype can influence its response to stress. D. Cell health may be perturbed within microsystems based on platform design or operating conditions. | |
Any external stimulus can potentially harm otherwise healthy cells based on the stimulus' intrinsic characteristics, quantifiable ‘amount’ of stimulus and its duration of exposure. For example, brief exposure to high-energy radiation (e.g. gamma radiation) could damage cells, as could prolonged exposure to comparatively lower-energy radiation (e.g. UV radiation) at the same intensity. Here, we refer to this overall “amount of stress” as a ‘stress dosage’. Typically, a cell would be able to adapt or repair itself following low stress dosage (Fig. 1B). Moderate dosages can stress a cell beyond its tolerance, where it may be forced to change irreversibly to a dysfunctional phenotype. Likewise, high stress dosages or inability to adapt to stress dosage can lead to cell death. Importantly, irreversible damage can activate cell death mechanisms before the effects manifest themselves visually.
Given the broad diversity of cell types, there is wide variation in cells' sensitivity to environmental stress. A particular dose can either stress, irreversibly damage, or even kill a particular cell depending on its genotype and phenotype (Fig. 1C). Examples of observable sub-lethal and reversible changes include cell swelling and fatty changes, while irreversible changes include lysosomal rupture, membrane breakdown and nuclear fragmentation. The central goal is to engineer platforms and their operating conditions to minimize perturbations from the healthy phenotype (Fig. 1D) by leveraging known biological stress responses. Within mammalian cells there are several stress pathways: pro-survival mechanisms as well as apoptosis pathways that are conserved among species and various tissue types. Probing such conserved pathways provides a means to discover conditions that are safe for a variety of cell types.
Several cell-stressing stimuli are found broadly in vitro. These stressors exist both in macro-scale and in microscale systems. For instance, all aspects of cell culture, handling, manipulation or analysis affect phenotype. Studies have revealed how different aspects of the cellular microenvironment regulate cell state,3 such as the culture substrate chemical composition4,5 its mechanical properties,6–8 the culture medium composition9 and culture architecture (2D vs. 3D).10,11 In this context, cell state regulation by these factors is of equal significance to both macroscale and microscale cell culture system design. This similarity of how cells can get stressed in macroscale systems and microscale systems is important. In particular, the microsystems designer can apply knowledge learned at the macroscale to the microscale. Given the lack of relevant reviews directed to the microsystems community, we will discuss how these stressors emerge within microsystems through design and instrumentation choices. With this context, we will first discuss mechanisms of cell injury and then explain how they are activated in microsystems.
Exposure to stressors within microsystems can impact cells through direct and indirect ways. For instance, fluid shear stress (FSS) can directly damage or rupture the cell membrane; high-energy light exposure can directly cause DNA strand breaks; and electric fields can induce joule heating that denatures intracellular proteins (Fig. 2).
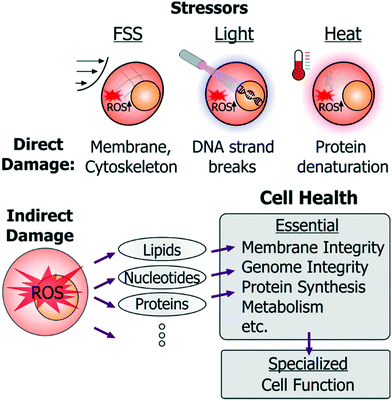 |
| Fig. 2 Examples of direct and indirect cell damage by microenvironment stressors. FSS, light and heat are the prominent initiators of cell stress. Each of these can cause direct and indirect harm to cells. FSS can directly damage cell membranes and cytoskeleton; high-dosage light exposures can damage DNA; and cell heating can directly denature proteins. Each of these stressors also induces intracellular ROS. ROS imparts indirect harm to cells by attacking cellular lipids, nucleotides and proteins, thereby impairing a number of the essential cell health processes. | |
In addition to such direct damage, microsystem stressors can harm cells indirectly. While indirect damage can occur by stress-induced ionic imbalance, pH changes, etc., the predominant cell damage occurs from excessive generation of reactive oxygen species (ROS). These species attack nucleotides (causing DNA strand breaks or crosslinking), cellular proteins (by inducing protein aggregation), or membrane lipids (through peroxidation), as well as lead to mitochondrial damage. In this way essential cellular processes (Fig. 1A) such as membrane integrity, genome integrity, protein synthesis, metabolism, are all susceptible to damage in microsystems through direct damage as well as to indirect damage orchestrated via ROS.
These stressors can further impinge upon conserved stress pathways that regulate cell state and function. ROS generated by phototoxicity can damage DNA and thus activate p53-regulated DNA repair and cell-cycle arrest mechanisms. More generally, excessive intracellular ROS as well as cellular heating can damage proteins that are then recognized and degraded by protein folding and trafficking chaperones via the heat-shock pathway. Mechanical injury, including that by FSS, can activate multiple mechanisms such as ROS, calcium signaling, mitogen-activated kinase pathway (MAPK), inflammatory nuclear factor kappa-B (NF-κB) pathway and others. Calcium imbalance and oxidative stress can both stress the endoplasmic reticulum,12 which initiates the unfolded protein response pathway.13 Glucose-oxygen deprivation (or metabolic stress) also upregulate this pathway through its regulator BiP,14 and can also drive KRAS and BRAF gene mutations, leading to tumorigenesis.15 On the other hand, the MAPK/ERK pathway regulates cell cycle and proliferation and its dysregulation can steer cells towards a cancerous state.16
Additionally, the NF-κB pathway closely regulates the homeostasis between survival and apoptotic pathways.17 In septic infections, toll-like receptors and other receptors recognize unique non-self-molecules (termed pathogen-associated molecular patterns) to sense microbes and for consequent activation of innate inflammatory pathways (regulated by several transcription factors such as NF-κB, AP-1, ATF-6, etc.).18 On the other hand, sterile stimuli like dead cell debris, toxins or cytotoxic irritants (e.g. silica dioxide, iron oxide, and other crystalline particulates) can also activate innate inflammation, mediated by the IL-1 pathway and by the secretion of a cohort of cytokines and chemokines.18,19
Altogether then, when introducing cells to device microenvironments it becomes important to identify cell-stressing stimuli and use that information to design appropriate devices and operating conditions that minimize adverse effects.20,21
Device design and operation
With this framework of cell health and cell stress responses, we will now cover recommendations for the microsystem designer to improve cell health in devices.
One important consideration is to define which cells are intended to be used within a particular device. There are two broad categories of devices that study or manipulate cells. First, there are platforms that are designed for specific models, for instance devices to study stem cell fate choice,22 hepatocyte toxicity,23,24 cell mechanobiology,25etc. Additionally, in this category are ‘organ-on-a-chip’ devices, which may utilize distinct cell types to mimic complex physiological behavior.2,26,27 A key aspect in the validation of such in vitro models is to recapitulate specific phenotypes. Hence, device operating conditions are established to demonstrate such functions26,27 in order to convince others (e.g., biologists) of the relevance of such systems.
The second category consists of devices that are not specific to a particular cell type. Such devices include cell sorters that utilize optical,28–30 electrical,31–33 magnetic,34–36 acoustic,37,38 or hydrodynamic inertial forces,39,40etc. Other examples include sample preparation devices,41–44 droplet-based platforms,45–48 or platforms meant for automated cell culture49–51 or perfusion culture.52–55 In such devices, assessment of the device microenvironment can arguably be harder because there may not necessarily be a ‘standard’ or well-defined cellular function that could be measured for validation, as for the cell-type specific devices.
Although this review, will focus on the second class of devices, there are several device design and usage conditions that are relevant to both these categories. To cover these, we classify microfluidic experimentation into three generic stages: 1) introducing cells into devices 2) keeping cells in devices 3) retrieving cells or information from the device. For each of these three stages, we will cover predominant stressors and provide recommendations to designers for minimizing their impact on cells. We have incorporated essential messages from this section into Table 1, which should better aid the readers in distilling our specific recommendations.
Table 1 Essential considerations for device design and recommendations for operating conditions that should assist in lowering cell damage during distinct stages of device operations
Issue |
Relevance to cell stress |
Recommendations |
Ref. |
Design |
Operating conditions |
STAGE 1: cell preparation and introduction |
Cell harvesting and suspension |
• Mechanical stresses (e.g. by cell isolation, purification, trypsinization) |
• Minimize cell suspension and cell loading time |
• Maintain cells in iso-osmotic solutions |
56, 58, 80, 75 |
• Ionic and nutrient imbalances in suspension solutions |
• Suspend cells in solutions compositionally similar to culture medium |
• Maintain liquid sterility |
Device treatment |
• Cytotoxicity (complement activation, protein adsorption or fouling) |
• Use biocompatible materials for fabrication |
• Thorough washing if alcohol, detergents or disinfectants are used for device priming |
18, 19, 57 |
• Anti-fouling coatings, UV treatment, autoclaving |
• Adsorbing or crosslinking ECM proteins prior to culture |
STAGE 2: cell maintenance in devices |
Shear stress |
• Membrane and cytoskeletal damage |
• Design higher and wider channels to lower FSS |
• Lower FSS by flow rate. |
58, 59, 70 |
• Activation of mechano-stress pathways |
• Designs that “shield” cells from FSS (e.g. microwells) |
• Lower transient FSS gradients for cells flowing through devices |
• ROS-induced stress |
• Avoid recirculating cells in peristaltic pumps |
Bubbles |
• Membrane damage and necrosis |
• Avoid abrupt geometries that cause dead volumes |
• Prime with low surface-tension liquids (e.g. ethanol) |
73
|
• Use integrated on-chip, or off-chip debubblers |
• Dead-end flow into gas-permeable materials |
• Avoid electrolysis generated bubbles |
• Operate at pressures above atmospheric pressure and avoid liquid suction operations |
Pathogen contamination |
• Activation of immune pathways and cell death mechanisms |
• Avoid reusing devices and tubing and interfaces |
• Assemble devices and interfaces with standard aseptic techniques |
17, 59 |
• Use fabrication materials compatible with detergents and disinfectants |
• Prefilter liquids and use in-line 0.2 μM filters in fluidic system |
• Sterilize devices with bleach and ethanol and wash thoroughly |
Nutrient stress and imbalance |
• Metabolic stress by waste accumulation and inadequate nutrient perfusion |
• Avoid cell encapsulation in environments with limited nutrient transport |
• Avoid long term nutrient deprivation by media perfusion |
56, 59, 74, 75, 80, 87 |
• Alteration of juxtacrine and paracrine signaling |
• Avoid elastomeric materials with propensity of solvent or cross-linker leaching to cells |
• Use solvent extraction and surface passivation |
• Ensure O2 and CO2 availability and equilibration to cells |
• Provide pre-equilibrated medium by convective or diffusive transport |
• Minimize medium evaporation |
• Can consider using conditioned medium, increased serum or growth factor content for cells with limited nutrient transport |
STAGE 3: cell or information retrieval |
Light |
• DNA damage, phototoxicity |
• Minimize light exposures |
• Use genetically-encoded probes instead of fluorescent organic dyes |
90, 99, 100 |
• ROS-induced stress |
• Use radical-scavenging components |
• Lower light source intensity, use shorter exposures and longer wavelengths |
Heat |
• Heat shock pathway activation |
• Integrate on-chip or off-chip probes to monitor and regulate device temperatures |
• Avoid heating cells (>2 °C) from their physiological setpoint |
109, 119, 120 |
• ROS-induced stress |
• Avoid thermosensitive hydrogels needing large thermal gradients for gelation |
• Avoid fabrication materials and interfaces that are poor thermal conductors |
• Interface devices with heat sinks |
STAGE 1: cell preparation, device treatments, and cell introduction
Cells constantly respond to environmental stimuli and hence can become stressed even before they are introduced into a device. Specifically, cell states can be influenced by how they are harvested and prepared before device loading. Relevant stressors in this context include:
Cell harvesting and suspension
Relevance.
The first step of interfacing cells with microfluidic devices involves harvesting or sourcing cells, as well as immobilization that can allow for the user to introduce cells into the device. Although the complexity and nature of these processes may vary between devices, it is important to realize common aspects of cell harvesting and suspension that may damage cells prior to device exposure. Importantly, as this step interfaces macroscale cell handling to microscale device environments, users need to consider nutrient and ionic imbalances at both scales and how they may impact cells. For instance, cells maintained in culture typically have access to nutrients in the medium, which are maintained in excess. Furthermore, any macroscale mixing or convective transport can assist in transport of nutrients to cells, as well as the removal of waste products from the cellular boundary layer. However, as cells enter the microscale, the designers need to consider specific channel length scales, as well as transport (diffusive, reactive and convective) timescales in their device that would be different from the macroscale culture or from cell suspensions.
Recommendations.
Barring mechanical stresses that may emerge from dissociation (e.g. for adherent cells), centrifugation (e.g. during purification), or cell isolation methods, an important factor in regulating cell viability and health is the suspension liquid that is used for introducing cells in devices. Particularly, maintaining cells in an iso-osmotic liquid is critical. Cells can also get stressed by ionic imbalances (e.g. calcium) as well as pH imbalances, and hence liquids with chemical composition and properties similar to culture media should be used. In regards to maintaining cellular homeostasis with the soluble microenvironment, we recommend designers to calculate relevant transport numbers such as the Peclet number, Damkohler number and the Sherwood number to estimate which transport mechanism dominates in their microenvironment, and subsequently design for device functionalities that allow for tuning that transport modality to avoid undesirable molecular imbalances. We point the reader to relevant analytical considerations from specific reviews elsewhere.56
If the liquid contains dissociation enzymes (e.g. trypsin) then these should be inactivated to prevent membrane damage that can cause necrosis. Furthermore, it is important to ensure that liquids are sterile, which can be achieved with autoclaving or alternatively, filtering with a 0.2 μm filter, and by maintaining aseptic techniques. These considerations are also applicable to liquids in which cells may be recovered into after they are exposed to devices.
Device treatment
Relevance.
Material bulk and surface properties impact cell viability and function. While the material and surface properties are often determined by the device application, it is common practice to ‘treat’ devices prior to introducing cells.
Recommendations.
This can be achieved by using anti-fouling (e.g. PEG-based, polyzwitterion-based or paralene-based) coatings.57 These coatings lower non-specific protein adsorption and can shield biological liquids and cells from charged surfaces. Omitting these precautions increases the risk of activating the complement-system proteins (present in serum-containing liquids), which can lead to activation of innate inflammatory pathways in mammalian cells. For device sterilization, it is common to autoclave devices, use UV-treatments, or perfuse devices with 70–80% ethanol. While UV-treatments can kill bacteria, they also generate short-term radical species, and leave endotoxins on device surfaces that can be detrimental to cells. On the other hand, autoclaving or ethanol perfusion (particularly in PDMS devices) poses the risk of water or ethanol absorption into liquid-permeable material. We recommend rigorous and prolonged flushing of the device with appropriate buffers or media to mitigate these risks. When cells are kept in devices for long durations, it is important to also consider potential transport of molecular species to and from the bulk device material, which we will address in the subsequent sections. For adherent cell culture devices, users should consider covalently attaching or adsorbing appropriate extracellular matrix proteins to device surfaces to improve cell health.
STAGE 2: cell maintenance in devices
Considerations for cell maintenance in devices depends on the device application as well as the time cells spend in devices. However, there are certain considerations that are generally important for maintaining viable and healthy cells in devices. These include minimizing mechanical stresses (particularly by fluid shear stress) and nutritional imbalances. While it is preferable to maintain cells at physiologic temperatures at all times, the considerations for thermal stress minimization are broadly related to lowering thermal gradients induced by physical forces and will be discussed in the following sections.
Shear stress
Relevance.
Since cells are cultured, sorted and manipulated in liquid environments, cell-based operations involve fluid flows with, or around, cells. Such flows consequently impart FSS upon cells. FSS is thus the ubiquitous mechanical stressor in microfluidic systems. As a microsystem designer, one needs to decide how to sustain flows within a device environment (i.e., through pumps or pressure sources). These choices will impact how cells are introduced into the device, maintained in the device, and how they may eventually be retrieved from such environments. Hence flow systems and operating conditions play a critical role in regulating cell physiology in microsystems.
Depending on the application, the intensity and duration of applied FSS can vary significantly across platforms. For instance, flow-based microfluidic sorters can impart short-but-intense FSS (100–1000s dynes per cm2 for ms-sec durations) while cell culture devices can subject cells to ‘chronic-but-gentle’ FSS (0.001–1 dyne per cm2 for hrs-days duration).58–60 Other microfluidic devices provide moderate FSS for ∼min durations.
FSS can have beneficial properties towards cells, such as maintenance of endothelial cell function.61 Nevertheless, in most microsystem applications it is viewed as a stressor.62–65 Consequently, to lower FSS-induced damage, microsystem designers may lower FSS by decreasing fluid flow rates, designing high-aspect-ratio chambers, and by other geometric designs (e.g. microwells) that shield cells from applied FSS.59 However, the ‘dosage’ at which FSS becomes a stressor is not always obvious. Despite lowered magnitudes, reported ‘safe FSS’ setpoints vary drastically among devices, even among those working with the same cell types. For instance, Villa-Diaz et al. cultured human embryonic stem cells (hESCs) in their microfluidic platform and reported that long-term exposure to the device FSS of 0.6 dynes per cm2 did not affect cell adhesion, and did not impact hESC differentiation.66 In contrast, Titmarsh et al. reported an optimal hESC culture FSS of 0.005 dynes per cm2, beyond which cells would detach and show signs of differentiation in their platform.67 On the other hand, Yoshimitsu et al. reported that device FSS of 0.01 dyne per cm2 did not affect the self-renewal marker Oct3/4 in human induced pluripotent stem cells after 3 days of culture.68 These examples provide evidence for a ∼100× variance in what was concluded as non-harmful FSS, making it challenging to identify absolute deleterious effects within a low FSS regime. This is important because many devices are designed to deliver FSS values to be below a certain setpoint (hence ‘safe’ for cells), however such notions are not generally applicable across cells or platforms.
Dose-dependent FSS activates complex biological cascades and mechanisms, such as activation of mechanosensitive pathways and calcium signaling in mammalian cells (reviewed elsewhere69), and as noted previously, can induce ROS and lead to compromised viability.64 In our lab, we specifically investigated sub-lethal stresses that can be generated by flow regimes relevant to various microfluidic devices by developing a cell-based FSS sensor.58 In this work, we observed sensor activation both when cells were cultured in perfusion as well as when they were introduced into a ‘flow-through’ microfluidic cell sorter at high FSS, learning that FSS can activate stress pathways which would not be apparent by assessing metrics such as viability or cell morphology alone. In this way our results and those of others collectively support FSS as a cell stressor.
Recommendations to lower FSS-induced stress.
The most straightforward method of lowering FSS is to lower flow rates (regardless of how FSS is generated). This is beneficial for devices whose dimensions are fixed for other reasons. In other cases, designing higher or wider channels prior can lower FSS. It is also important to note that inferring and lowering FSS-induced stress on non-adherent cells or cells that flow through a device environment requires a few additional considerations to those suggested above.70 Specifically, a free-floating cell that can move with the flow may be able to reach a force equilibrium to balance the velocity gradient of the applied flow (e.g. a FSS gradient force generated in Poiseuille may be balanced by a wall-induced lift force). While at such an equilibrium the cell may not experience WSS, it will become important to consider the timescale required to achieve this equilibrium and whether cells are perturbed in that transient state. We and others have found that such transient stresses could indeed be sensed by circulating cells in microfluidic devices,58,70 and such an impact could be lowered by reducing the shear stress gradients (e.g. by the flow rate). Furthermore, in cases where the channel characteristic dimension is similar to the free-floating cell's diameter (e.g. in constriction channel devices), one would also need to consider additional mechanical stresses could arise and cause cell deformation-induced cell damage. In regards to flow sources, from our experience and others,71,72 we have found that peristaltic pumps can activate stresses and alter cellular function when recirculating media72 or cells71 and hence should be used with caution in such applications. These considerations are also applicable to Stage 3, where fluid flows are utilized for retrieval of biological information or cells.
Bubbles
Relevance.
Most microfluidic engineers and users would acknowledge bubbles as a source for unpredictable device failure. Although bubbles can be avoided by careful introduction of bubble-free liquids into devices, typically the source for bubble generation relates to how devices are used or maintained over time. For instance, electric field-induced electrolysis can induce bubbles in the short term as well as change the dissolved gas composition in the perfused liquids.
Recommendations to prevent bubbles.
Although undesirable bubble generation impedes every fluidic device application, precautions can lower occurrence of such failure modes. Device designs that minimize the abrupt geometries that cause dead volumes make it easier to remove bubbles during flow filling. Devices can also be ‘primed’ to remove pre-existing gas and bubbles in the device, by washing with a low surface-tension liquid like ethanol, or by dead-end flow into gas-permeable materials. For PDMS- or gas-permeable culture devices, we advise users to pre-equilibrate devices in the relevant CO2 environment prior to cell culture. Care should also be exercised for using gas-permeable tubing through which dissolved gasses can be transported and hence impact bubble generation. Additionally, to avoid externally generated bubbles from being introduced into devices, one can use integrated micro-debubblers73 or discrete macroscale debubblers at the device inlet. Finally, operating at pressures above atmospheric pressure (pushing liquids) avoids bubble introduction through permeable devices that can occur when operating below atmospheric pressure (pulling liquids).
Pathogen contamination
Relevance.
Pathogen exposure, endotoxin- or device material-induced toxicity are important regulators of cell health. With our recommendations mentioned earlier, one can sterilize and prime devices before introducing cells with aseptic techniques. While a large majority of microfluidic devices are single-use, some users may wish to reuse devices and fluidic components. In some other cases, one may need to disconnect and reconnect tubing during experimentation. In such cases, it is important to ensure that these operations do not expose cells to pathogen contamination, which can lead to cell inflammatory response and eventual death.
Recommendations to prevent contamination.
We recommend users to sterilize all fluidic components prior to use. If users wish to reuse tubing and connectors, it is critical to wash them immediately after experimentation. One protocol that works in our hands is as follows: wash with water or saline buffer (to flush systemic fluids), bleach or ethanol (for system decontamination), water (to remove residual solvent), a strong enzymatic detergent (to remove protein residues), and a final wash with water again. For device recycling, users should ensure that device materials are compatible with chemicals used for cleaning. Finally, to minimize pathogen contamination during experimentation, it is beneficial to form fluidic connections in a sterile environment (e.g. in a biosafety cabinet) and use inline 0.2 μm filters in the fluidic network as appropriate.
Nutrient stress and imbalance
Relevance.
Long-term cell culture devices need to provide cells with sufficient nutrients and oxygen, while removing waste products to maintain cellular homeostasis. Nutrient deprivation may happen when lowering device culture FSS by restricting media flow rate, and consequently lowering the nutrient delivery and waste perfusion rates. Nutrient deprivation is also be relevant to devices where cells are encapsulated in isolated volumes such as droplets, gels, or microchambers. Encapsulated cells may also be prevented from receiving soluble signaling (e.g. paracrine growth factors) that they would otherwise receive from neighboring cells in culture. Such isolation will also disable juxtacrine signaling. In the presence of convective perfusion, autocrine soluble can also be removed, further altering the cellular microenvironment.
Another route towards nutrient depletion in such culture devices is by the adsorption and losses of nutrients to the device materials, such as PDMS. Specifically, PDMS is known to adsorb proteins and absorb hydrophobic molecules, imparting nutritional imbalance upon cells.74 Furthermore, media evaporation in PDMS microfluidic devices can lead to osmotic changes that can hinder cell growth and development.65,75
While depriving cells of carbon sources or failing to remove waste products such as lactate is universally harmful, oxygen has a more varied effect on cell physiology. Metabolically active cells such as hepatocytes require high amounts of oxygen,76,77 while other cells types (e.g., stem cells78,79) have enhanced phenotypes at the low oxygen tensions found in most in vivo microenvironments. While prolonged changes in oxygen tension can manifest into functional adaptations, short-term exposures are less commonly found in microsystems but remain to be explored in the context of cell health.
Recommendations to lower nutrient stress.
While some cells can adapt and remain viable after short-term nutrient deprivation (e.g. suspending in PBS during FACS sorting), longer-term deprivation should be avoided by perfusion or provision of ‘fresh’ medium with dissolved gasses.
It is important to provide ‘fresh’ equilibrated medium either by convective flow or diffusive transport. Since different cells have different metabolic requirements, the exact amount of medium required must be determined empirically using cell health assays described later on. Nutrient stress is affected by the cell density, and a helpful guideline is to use densities similar to those used in traditional culture dishes. An important caveat is that both the areal density and volumetric cell density matter, an aspect that has been reviewed in detail elsewhere,80 so a direct translation from macro to micro is not possible. At one extreme, it is important to avoid creating a confluent-like soluble environment that can cause reduction in cell growth.81 In the other extreme, excess medium availability that will desirably dilute waste products will also undesirably dilute autocrine factors important for cell growth, as often observed with macroscale single-cell cloning. One workaround is to create conditioned medium (CM) that contains secreted factors (but may also contain waste products) and use it for perfusion or medium replacement.82 Other strategies include increasing serum content (hence growth factor availability) in the medium, or using chemically defined medium that has been optimized for single-cell culture and maintenance.83 In all cases, use of cell health assays as described later in this review are critical to determine that the operating conditions are suitable.
For devices with restricted gas-permeability (e.g. 3D-printed plastic devices or droplet-cell culture platforms) it is important to maintain cells in liquids containing relevant amounts of dissolved O2 and CO2 to prevent stress induced by hypoxia and pH imbalance in addition to that by nutrition imbalance.84 For mitigating leaching of uncured crosslinkers from PDMS devices that can impart cell stress, we recommend solvent extraction as well as surface passivation techniques,85 or use of newer elastomers that have been specifically designed for long-term cell culture.86 Other detailed strategies for lowering PDMS adsorption, evaporation in devices, and related nutrient deprivation in microsystems have been reviewed elsewhere.59,87
Stage 3: cell or information retrieval
Depending on the particular application, there can be several modalities (optical, electrical, mechanical, thermal forces, etc.) in which a device may be used to monitor cells or retrieve cells. We will recommend strategies to minimize cell stress induced by some of such approaches.
Light
Relevance.
Light is broadly used within microsystems, particularly for monitoring and manipulating cells. Visible light has been used to image live cells, with and without fluorescent molecules present as proteins or staining dyes, at tissue-scale resolution to near-molecular resolution.88,89 However most cells in vivo are not exposed to light, and microscopy-induced photoxicity90,91 can become a stressor in microsystems. Ultraviolet light, which is known to cause cell stress via DNA damage and ROS,92 has been utilized in microsystems for cell encapsulation via photopolymerization.93–95 UVA and violet light (340–380 nm) is typically used for imaging blue fluorophores (such as Hoechst 33342), blue light (460–500 nm) is used for imaging green fluorophores (such as GFP, or Calcein-AM); and green light (528–553 nm) is used to image red fluorophores (such as ethidium homodimer-1). In other applications, optical forces have been applied in microsystems to sort cells with the use of lasers.28–30,96–98 Optical tweezers typically employ near infrared (NIR)-wavelength lasers, which stress by indirect photothermal heating.
Recommendations to lower light-induced damage.
Phototoxicity has been studied extensively and several precautionary measures have been identified to minimize detrimental effects, comprehensively reviewed elsewhere.90,99,100 Briefly, we recommend using genetically-encoded probes instead of fluorescent organic dyes, which will lower ROS generation by imaging.101 Otherwise, the use of radical-scavenging components can be added the imaging medium.91 To lower photoxicity in long-term imaging, users should lower the light source intensity, use optimized optical filters, and use shorter exposure times.102 Longer excitation wavelengths have also been shown to reduce stress.92,103 Users should also consider increasing binning for increasing signal and thus allowing reduced exposures that lower phototoxicity. It should be also realized that many imaging modalities (widefield, confocal) expose cells not being imaged to light, increasing photodamage. Thus, we recommend assessing cell phenotype both in regions of interests and in regions of the device which have not been imaged when optimizing imaging parameters.
Heat
Relevance.
As mentioned earlier, heat stress in microsystems generally occurs as an indirect consequence of physical forces used for cell manipulation, since all dissipation eventually couples into the thermal domain. For instance, laser light exposures can raise culture medium temperatures, typically on the order of 1 K/100 mW.104,105 Heat can also be generated by electric fields and forces used to manipulate, sort or retrieve cells. For instance, electric fields that have been used to manipulate cells, such as via dielectrophoresis, can lead to Joule heating in conductive liquids (∼16 K for 4 V for 50 μm spaced electrodes in culture media, scaling quadratically with voltage106). Depending on the ambient temperature, such elevated temperatures can heat cells significantly greater than the in vivo mammalian temperature of 37 °C. Integrated electrodes in microsystems have been used to maintain physiologically heated cell culture,107 however applying higher temperatures with such electrodes can induce substantial heat stress. This aspect was explored in microdevices to intentionally apply elevated temperatures to study cellular heat shock,108,109 to heat cells for mimicking cell injury110 and even to lyse cells.111
In addition, cells can be exposed to thermal stresses in devices where they are encapsulated within, or retrieved from thermosensitive hydrogels. Hydrogels with sol–gel transition temperature close to physiological temperature have been also been used in cell printing applications. Certain biomaterials (e.g. collagen and elastin) form gels when heated above their transition temperature, while others (e.g. agarose and gelatin) form gels when cooled (reviewed elsewhere112). However in such processes, cells may be exposed to large thermal gradients (e.g. 40 °C to 4 °C, and 70 °C to room temperature) for seconds-minutes duration.113,114
Cells may also experience thermal stimuli by other physical forces such as from radiation forces in acoustophoresis. Specifically, losses in the piezoelectric transducers used in these devices generate voltage-dependent heat which can couple into the fluid.115,116 Such heating can cause drifts in the resonance frequencies and hinder device performance,115 hence many devices have incorporated heat sinks or active temperature controllers to maintain operational temperatures (typically pinned around room temperature117,118). While subjecting cells to ambient temperature may not activate heat shock pathways, prolonged exposures can activate unique cold shock stress pathways in mammalian cells.119,120 Overall, cell exposure to significant temperature changes above or below their physiologic range can occur in a variety of platforms, and, in return, pose risks of cell damage in microsystems.
Recommendations for lowering heat-stress.
Most microfluidic materials (polymers, glass) are poor thermal conductors, and thus cannot quickly transfer heat out of or into the device; silicon is a notable exception, though it is not transparent and expensive and thus not commonly used. Even with thermal insulators, keeping thermal path lengths short (thin substrates, narrow sidewalls) can increase heat transfer and help regulate temperatures.
When using thermosensitive hydrogels, designers can model the heat transfer within the biomaterial, and also measure gelation properties of microscale thermosensitive hydrogels for cell encapsulation.121 In order to minimize thermal gradients applied to cells during gelation (e.g. encapsulation) or during release, designers may modify concentrations of cross-linking agents and may add chemical modifications to the biomaterial.122
In terms of operating conditions, we recommend users to ensure that the cells generally do not experience elevated temperatures (>2 °C) from their physiological setpoint to avoid heat shock pathway activation. Temperature regulators, or electrical or molecular thermal probes should be used to characterize the temperature in the device. In many cases, users may need to keep devices at room temperature and use heat sinks to ensure that the temperatures in their device do not exceed the heat shock activation thresholds. The impact of keeping cells on ice or introducing them to ice-cooled devices is poorly characterized in comparison to what is known about cell cryopreservation or heat shock activation and hence should be characterized in the particular device and cellular context as appropriate.
Methods for assessing cell health in microsystems
With the stresses delineated, it is clear that empirical investigation is important to develop operating conditions that ensure cell health in devices. Cell health assays can be divided into those provide a gross/global view of cell state (generic assays) and those that provide specific information.
Generic assays.
Cell health has been reported both by assaying cells directly, and by indirect assessment of the cellular microenvironment. While there exist examples of the latter, (e.g. monitoring medium pH123,124 or dissolved oxygen levels124,125), it is generally more common to assay cells directly.
To investigate if a device and its operating conditions are not detrimental to cells, the most obvious (hence most popular) measurement reported is that of cell viability30,39,126–130 (Fig. 3A), followed by assessment of cell morphology and proliferation49,50,54,129–132 (Fig. 3B).
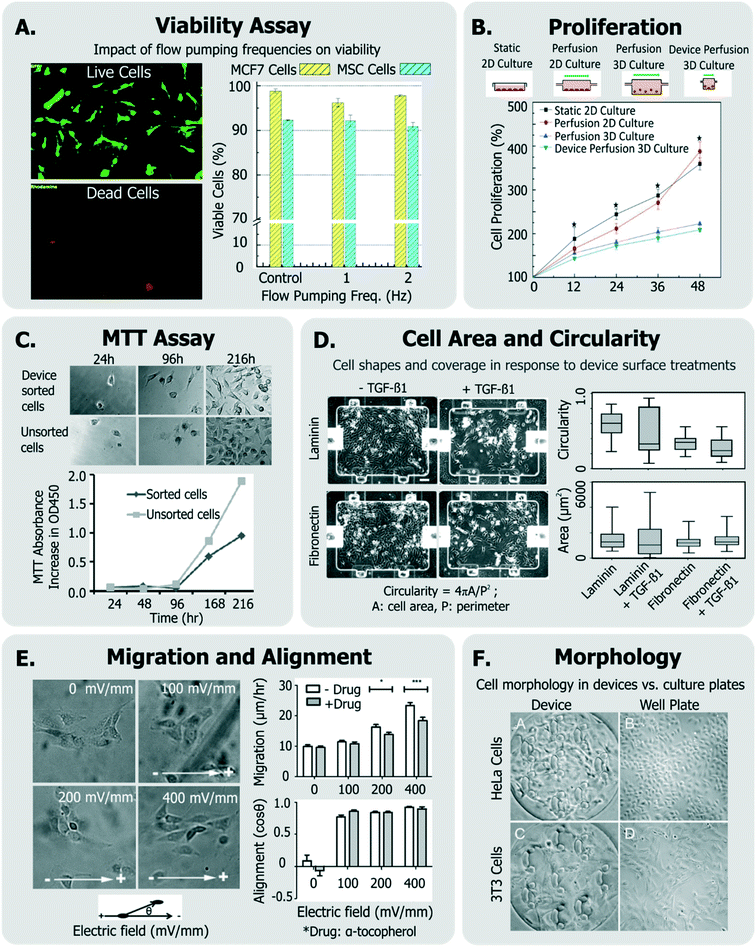 |
| Fig. 3 Exemplary generic assays for measuring cell health. A. Viability of MCF7 and MSC cells assessed in response to device flow pumping rates using a live-dead assay (adapted from ref. 129). B. Comparison of cell proliferation rates within device perfusion platforms and macroscale analogues (adapted from ref. 139, with permission from the Royal Society of Chemistry). C. Dynamics of cell metabolism assessed of device-sorted cells compared to unsorted cells using the MTT assay (adapted from ref. 131). D. Changes in cell circularity and area quantified in response to device surface with laminin or fibronectin in order to assess ability of cells to undergo EMT within device environment (adapted from ref. 63, with permission from the Royal Society of Chemistry). E. Impact of applied electric fields upon migration rate and cell alignment to electric fields (adapted from ref. 150). F. Morphology and adhesion of HeLa and BALB/3T3 cells in a microfluidic cytotoxicity analysis device qualitatively compared to culture plates (adapted from ref. 51, with permission from the Royal Society of Chemistry). | |
Viability can be quantified by a variety of assays,133 though it is most conveniently done so by using colorimetric or fluorescent probes which can imaged within microsystems. In many cases,132,134 viability has been assessed by exclusively labeling live cells using cell-permeant Calcein-AM stain, which becomes fluorescent and cell membrane-impermeant by intracellular esterases in viable cells. On the other hand, others135–138 have measured fractions of cells with compromised membranes (labeled dead), with Trypan blue stain.
While simple, the drawback of using these assays is that they do not label the “other” cells. A better approach is to have two stains, so that both live and dead cells are positively labeled. These ‘live/dead’ stains30,63,129,139,140 typically use Calcein-AM (which makes live cells fluoresce green) and ethidium homodimer-1 (which makes dead cells fluoresce red). This method is particularly useful as it quantifies relative impacts of proliferation and cytotoxicity.
Viability-only assays fail to identify early apoptotic (and thus likely stressed) cells, as these cells do not have a compromised membrane and are undetectable by Trypan blue, propidium iodide (PI), ethidium homodimer-1, and 7-aminoactinomycin D (7-AAD) stains. Phosphatidylserines, which flip from the cytoplasmic to extracellular leaflet during early apoptosis, can be stained with annexin V. Though not previously applied for device design or operating condition optimization, annexin V staining combined with PI141 or with 7-AAD142 has been conducted within microfluidics to identify viable (double negative), early apoptotic (annexin V positive, PI negative), or late apoptotic and dead (double positive) cells. Viability and apoptosis assays within microfluidic devices have been reviewed elsewhere.143
Viability can also be discerned by measuring cellular metabolism, such as by monitoring culture glucose consumption rates,144 or by monitoring the activity of cytochrome p450 intracellular enzymes (in the case of hepatocytes124,145). The metabolic dye 3-(4,5-dimethylthiazol-2-yl)-2,5-diphenyltetrazolium bromide (MTT) is applicable to a broad variety of cells, where the cell-soluble tetrazolium is metabolized to cell-insoluble blue formazan crystals that can be measured by colorimetric methods.133 The MTT assay has been also been utilized to report on cell proliferation as a means of representing cell heath (Fig. 3C).131,146 Although the readout of the MTT assay is sensitive to cell metabolism, it is also sensitive to the number of cells in the assay, the incubation time, requires colorimetric measurement, does not report on how many dead cells are in the system, and the short path length of microsystems makes colorimetric assay difficult. Given these challenges, one would choose MTT over live/dead assay when the system is not amenable to detailed microscopic imaging, or where metabolism itself is of primary interest.
Cell proliferation has been also measured qualitatively, and used to infer that cells did not incur damage within different microsystems.51,54,147,148 Other generic assays include measurements of cell shape and elongation63 spreading,149 migration,150 or other aspects of cell morphology.54,66,107,146 For instance, among a panel of assays, Park et al. quantified cellular circularity, elongation and area in response to various device surface coatings and treatments to discern the epithelial-mesenchymal transition (EMT) potential of device cultured cells63 (Fig. 3D). Wu et al. investigated the impact of applied electric fields (EFs) upon fibroblast cell alignment migration rate, where they noted both increased migration rate and ROS production with increased field strengths150 (Fig. 3E). In another example, Wang et al. validated their microfluidic cytotoxicity analysis platform by qualitatively comparing cell morphology and adhesion of two cell lines (BALB/3T3 and HeLa cells) within their device to that within standard culture well plates (Fig. 3F), noting similar appearance.51
Specific assays.
A viable cell does not ensure that the cell is stress-free or unperturbed. With this rationale, many groups have investigated cell health in their platforms beyond the generic assays. One broad way to group these assays is based on whether they affect upstream, short-term, and relatively broad aspects of cell phenotype, or downstream, long-term, and more specific aspects.
Some groups have investigated short-term changes (seconds-min range) in response to the device conditions (Fig. 4). Such assays typically focus on intracellular signaling. For instance, Perroud et al. measured NF-κB (a transcription factor that regulates inflammatory programs) translocation via engineered reporters, and extracellular signal-regulated kinase (ERK) phosphorylation (a mitogen activated protein kinase) via staining, within cells that were sorted through their microfluidic device30 (Fig. 4A). El-Ali et al. focused more broadly on the stress-inducible mitogen-activated protein kinase (MAPK) pathway,151 through the phosphorylation of ERK, JNK and p38 kinases in cells passed through their device.128 Importantly, NF-κB, ERK, and MAPK are involved in transducing many of the stressors described earlier.
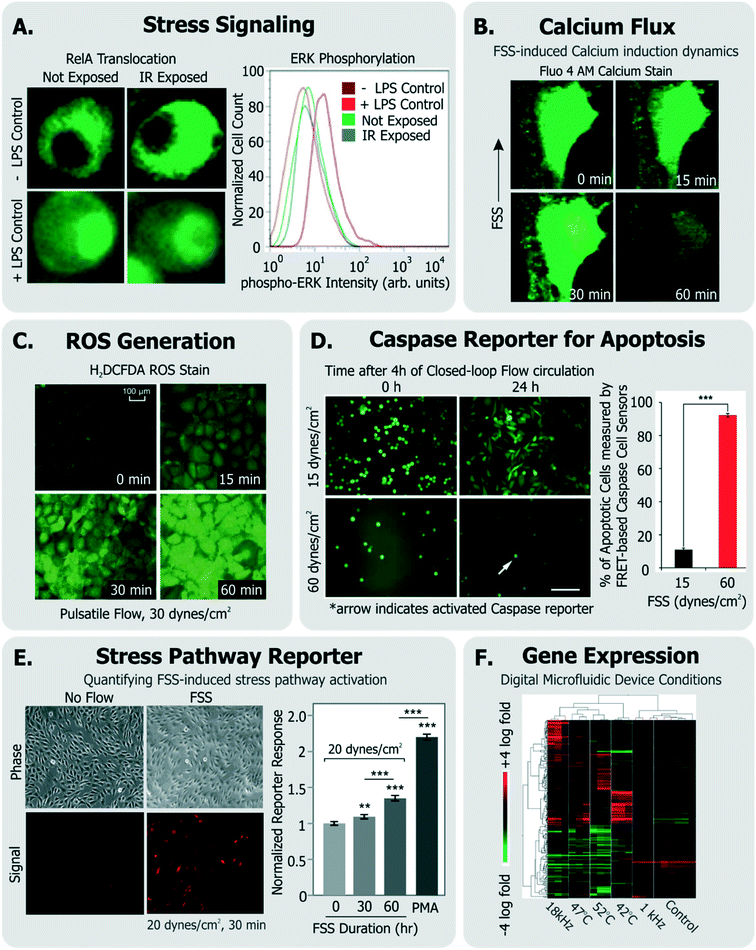 |
| Fig. 4 Exemplary specific assays for measuring cell health. A. Translocation of transcription factor RelA and flow cytometry measurement of ERK-phosphorylation in cells exposed to IR laser within a microfluidic sorter (adapted from ref. 30). B. Flow-induced changes in calcium flux and cell area measured using fluorescent Fluo-4 AM dye (adapted from ref. 149, with permission from the Royal Society of Chemistry). C. Accumulation of ROS in cells exposed to pulsatile flow measured using the fluorescent probe H2DCFDA (adapted from ref. 155, with permission from the Royal Society of Chemistry). D. Quantification of apoptotic cells among cells continually circulated within a closed-loop flow system using a FRET-based caspase reporter (adapted from ref. 71, under CC BY 4.0 license). E. Quantification of FSS-induced stress pathway activation and RFP induction using a cell-based FSS sensor (adapted from ref. 58, with permission from the Royal Society of Chemistry). F. Microarray analysis of cells exposed to various stimuli experienced in a digital microfluidic device in contrast to cells given heat shock (adapted from ref. 21, with permission from the Royal Society of Chemistry). | |
Calcium is an intracellular messenger important in regulating several essential cellular functions, and disturbances in its homeostasis can lead to many diseases.152 FSS-induced calcium signaling has been measured by many groups137,149 (Fig. 4B). For instance, Yin et al. measured calcium flux in CHO cells in response to a large range of FSS (∼0.01–10 dynes per cm2) in their devices and noted FSS-dose dependent induction of Ca++ signals, which matched signals from chemical agonists, even at moderate FSS.137
Cellular ROS and its direct effects can also be assessed. A common example is the probe 2′-7′-dichlorodihydrofluoresce diacetate (DCFDA), which is taken up by a variety of cells, where it fluoresces in response to intracellular ROS.153 Wu et al. used DCFDA to measure cell stress induced by electric fields in their device,150 Lo et al. used the same measurement against oxygen gradients in their device,154 and Chin et al. used it to measure ROS induction in response to shear stress155 (Fig. 4C). An immediate effect of ROS is DNA damage, which can be characterized by measuring histone γ-H2AX phosphorylation,156 which is known to occur following DNA double strand breaks.157
Other examples of short-term cellular responses to stress include changes in membrane receptor display, as well as expression of immediate-response stress genes. Adams et al. investigated platelet activation in their acoustophoresis device in response to the applied acoustic fields.117 Specifically, they reported that expression of CD62 did not immediately vary in platelets with or without device acoustic fields, compared to off-chip prothrombin-treated positive controls. Wang et al. developed a microfluidic platform which utilized optical forces in sorting cells.28 They measured the viability of sorted HeLa cells, and furthermore measured the expression of heat shock sensitive HSPA6 gene and multifactorial cell stress sensitive Fos gene immediately after sorting to assess if the device activated stress pathways.
Short-term molecular assays have the advantage that they can be run quickly after exposure, rather than requiring extended culture. This feature is appealing for the device user or designer. They also have a higher level of specificity than generic assays, as they focus on individual molecules and pathways. The drawback is their assay complexity; since they may require genetically modified cell lines (in the case of NF-κB translocation) or immunofluorescence with phospho-specific antibodies (in the case of kinase assays).
For longer term changes (∼hours), an assay will focus on gene expression or cell function. Assays focusing on expression or function have the advantage of specificity; upregulation of a gene involved in the heat shock pathway is strong evidence that the cell underwent stress. Additionally, expression assays do not require many cells (a few thousand is routine, and single cell is possible), and the results can be quantitative (e.g., fold upregulation). Following this approach to quantify changes in gene expression, Hur et al. performed microarray analysis of 100 s of genes in MCF7 cells sorted through their inertial microfluidic device against unsorted controls to assess whether there were any global transcriptional changes as a result of sorting.140 Similarly, Sharei et al. developed a microfluidic platform for intracellular delivery, and measured expression profiles of the POUF5 and ALP genes within mouse embryonic stem cells that passed through the device, to assess if certain conditions could initiate cell differentiation programs.127 The drawbacks of these approaches are that 1) it takes ∼hours for expression to occur, which can increase optimization time, and 2) the exact time from stress to readout will affect the assay results, since the amount of mRNA or protein present will depend on both synthesis and degradation. Additionally, most of these assays are endpoint, and so require cell recovery and isolation, though live-cell reporters do exist.
As an alternative, some groups have utilized live cell-based sensors that can provide an integrated response to environmental stressors. Specifically, Davidsson et al. utilized a luciferase-based sensor in HeLa cells (activated by MAPK through G-coupled protein receptors158) to monitor long-term cell health their microfluidic platform.159 Regmi et al. used a caspase-activation FRET reporter to monitor apoptosis activation in continually circulating tumor cells within a microfluidic flow system71 (Fig. 4D). In this way, their approach allowed for classifying live, dying and dead cells in response to the flow conditions. Our own group has generated a suite of ‘open-source’ cell-based sensors that report on stress-induced pathways via fluorescence.58,109,160,161 Specifically, we engineered distinct sensors in NIH3T3 cells to express red fluorescence protein (RFP) when they were exposed to FSS,58,161 genotoxic stress,160,161 or to heat shock that activated the respective stress pathways.161 For instance, short term FSS exposures activated our FSS sensors, and their induced RFP could be quantified by both microscopy and flow cytometry58 (Fig. 4E). Our sensors have further been adapted and used by other groups to investigate stress in distinct device microenvironments. For instance, Au et al. utilized our heat shock sensor109 and further performed microarray analysis (Fig. 4F) and comet assay to investigate if their digital microfluidic platform biased cell health.21
Late-term changes in cell health (∼days) have also been reported. Lopacinska et al. measured neuronal-like PC12 cell viability with Calcein staining, cell cycle with PI staining, metabolism with MTT, and gene expression changes with microarrays in response to common culture substrates used in microsystems such as polydimethylsiloxane (PDMS), polymethylmethacrylate (PMMA) and polystyrene.146 As another example, Villa-Diaz et al. measured expression of OCT3/4 and NANOG genes in cultured hESCs to assess if their device FSS altered pluripotency after days of culture.66
A common approach to investigate the cellular inflammatory response is to quantify the release of relevant cytokines, chemokines and interleukins.162,163 These serve as important markers for assessing acute and chronic septic and aseptic inflammation. Despite the prevalence of such assays in biological sciences, they are infrequently performed in the context of microsystem design or assessment. This may in part be a result of technical challenges in assaying secreted proteins in microsystems (e.g. low cell numbers or analyte dilution). These challenges have motivated the development of intricate microsystems for inline detection of inflammatory biomarkers,162,163 or surface patterning of protein detection regions within microfluidic channels.164–166 While these platforms may allow for sensitive and multiplexed detection of secreted analytes, they require specific expertise in device integration and operation and hence it can be challenging to integrate such platforms within typical device workflow and optimization. Notably, bead-based protein sensors that can be introduced and incubated with cells within devices can be relatively convenient in this regard, and can enable both imaging-based167 and off-chip168 non-destructive readouts. Alternative strategies exist (e.g. those applied for immunology studies169) may also provide similar benefits. Broadly, if these aforementioned challenges can be solved with other specific and conveniently-translatable methodologies, then sensitive and specific quantification of secreted cytokines from cells within device environment will serve as a powerful, non-destructive assay for cell health.
Though extensive device optimization or characterization using a variety of cell health assays is not commonly found in literature, the examples from various groups discussed above do demonstrate a few important points. First, the microsystems community does care about cell health in their platforms, and further, it does employ a variety of techniques and assays to investigate the phenomenon. Second, there are several examples of groups using similar assays (e.g. viability, proliferation), but there lacks a consensus when it comes to applying assays specific (and likely more informative) to cell health. We believe that the latter is related to certain inherent challenges in adapting established cell health specific assays within the device design and workflow.
Current challenges in assessing cell health within microsystems
To systematically assess cell state in a device microenvironment, one has to consider the variety of assays (generic and specific) used for assessing cell health. In this regard, it is common to adapt standard assays within engineered microsystems, or apply them to cells that have experienced the device microenvironment. While some assays, such as imaging-based assays, are easily translatable to microscale technologies, there exist challenges in finding assays that are specific to cell health, quantitative, and conveniently adaptable. Some of these challenges are elaborated below.
Challenges and tradeoffs
Challenge of low cell numbers.
In microscale platforms where cells are retained in small volumes (for culture, analysis, manipulation, etc.), there are inherent limitations on the number of cells available for running any assay. ‘Flow-through’ devices such as sorters that do not work with rare cells, or instead work with cell lines are an exception to this limitation, since the cell availability is directly related to the starting amount of cells. In most other cases, with limited cell numbers it becomes challenging to run biochemical assays such as western blots, that typically require >100
000 cells. Even qRT-PCR, which can be run at the single-cell level, is much simpler when one uses >1000 cells.
Complexity of specific assays.
Many specific assays are destructive, costly and complex, and hence technically prohibitive. Assays of intracellular protein levels or gene expression have been performed by our group82 and others49,63 while developing various platforms. However, within the microfluidic community the usage of such methods to interrogate device bias is not routinely performed for certain reasons.
First, it is usually not obvious as to which cell health biomarkers to measure, and hence which assay to run. Second, many relevant assays (such as qRT-PCR, western blotting, flow cytometry) require may steps such as washing or mixing biological materials, isolation, purification, etc. These additional steps need to be incorporated into the device workflow based on practicality and convenience. Obtaining starting materials (cells or cellular constituents) can be done by either taking the cells off-chip for processing, or bringing the assay reagents on to the chip. Retrieving immobilized cells from microfluidic devices is often a non-trivial task. While attached cells can be harvested by flushing enzymatic dissociation buffers,132,170 one has to be careful about cell losses in the fluidic network. Alternatively, cells can be retrieved with optical methods,171 or mechanically (e.g. with micromanipulators147,172 or micropipettes50), each of which require sophisticated technical expertise. On the other hand, while bringing assay reagents to cells is possible through specific fluidic architectures173 or device designs,49,174 these approaches may not be easy to integrate within one's own device workflow. Finally, many specific assays are end-point and thus destroy the cell, making it laborious to investigate longitudinal aspects of cell health.
Lack of standardization.
Perhaps the most noteworthy challenge in assessing cell health in microsystems is the lack of standard assays and associated quantitative metrics. Given the broad diversity of both specific and generic assays to study cell health, microsystems engineers need to pick specific assays which are convenient to adapt given a certain amount of cells in their particular workflow. Such standardization would in turn allow inter-platform and inter-laboratory comparisons.
Guidelines for best practices
Given the combined complexity of cell stress biology, vast number of assay choices, and large parameter space for microsystem design and operation, it's likely that there may be not be an exclusive ‘universal design or operating condition’ for optimizing all devices for healthy cells. However, it is possible to outline as set of best practices for the microsystems engineer and end-user, which they can adapt in their own device context (Fig. 5):
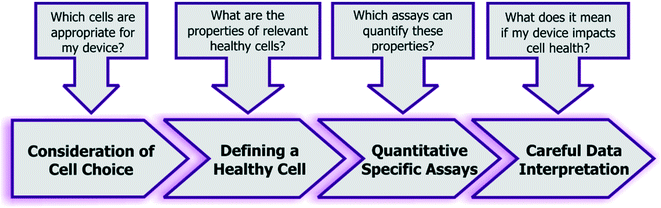 |
| Fig. 5 Recommended guidelines and considerations for assessing the impact of engineered systems upon cell health. | |
Consideration of cell choice.
When designing, it's important to determine if the platform will be used for specific cell types or a variety of cells. For specific phenotypes, one must determine if the microenvironment will mimic physiologic (e.g., bioreactor platforms) or pathologic (disease models) conditions. This is important because it guides the phenotype selection process and defines desirable biological states. For instance, in a disease-mimic model cells can be intentionally stressed, whereas in a physiologic model cells should remain in their in vivo healthy state. For instance, culturing (diseased) cancer cells in a disease-relevant microenvironment makes sense, and by using them one can investigate if the microenvironment is enabling the cancerous cells to remain in their equilibrium biological state. However, using cancer cells to check whether a generic cell culture platform, sorting device, etc., stresses cells is not informative. Such cells were not normal to begin with (i.e., they may have dysfunctional or dysregulated stress-response pathways), and it is impractical to judge and learn from perturbations that make such cells more or less cancerous. For instance, Barnes J. et al. compared primary blood cells, primary epithelial cells, non-transformed cell lines and cancer cell lines in their ability to withstand identical FSS conditions.175 Here they noted that cancer cell lines demonstrated high resistance to FSS-induced damage, in contrast to non-transformed cell lines and primary cells that took a relatively bigger hit on their viability. Results such as these demonstrate the importance of carefully choosing relevant cells for validating the impact of engineered systems on cell health.
Defining the reference cell.
Prior to any optimization, it is important for device designers and users to establish the reference ‘healthy cell’. In some cases it may make sense to use cells cultured in incubators as a reference (such as when working with cell lines). Cells exposed to the device environment can then be compared against cells from a culture incubator to determine device-induced effects. When working with primary cells, rare cells, or cells that require laborious processing prior to usage it may not always be convenient to obtain a reference population. Furthermore, in some of these cases, the cells may already have become stressed or dysfunctional in-part due to the ‘pre-processing’ and hence may be inappropriate for device optimization. Here, one should use a multi-step optimization, first using a conveniently available and relevant reference cell population to ensure the microenvironment does not affect essential cell processes, followed by relevant functional assays based on the original cell phenotype (see following section for recommendations).
Applying specific multiparametric assays.
The strength of the study will depend on the choice of assays employed to assess cell health. The challenge is that if different devices and operating conditions are validated by different combinations of specific assays, each investigating a different set of nodes or pathways, it becomes difficult to collect communal data on cell-safe design rules and operational guidelines. An alternative is to execute tailored multiparametric cell stress assays that provide similar insight. For example, our lab generated cell-based sensors that were genetically engineered to express stress-inducible fluorescence specific to heat shock, FSS or DNA damage pathways.58,109,160,161 These sensors do not require reagents other than for cell culture, and can be assessed by the convenience of microscopy or flow cytometry. Recently, we engineered multiplexed cell-based stress sensors, enabling simultaneous assessment of critical stress nodes.161 Our methodology has begun to be adapted in different laboratories interested in quantifying cell-stress phenomenon in their specific bioinstrumentation context.21
Alternative multiplexed approaches have also been developed by other groups, which may be useful to the broad microfluidics community. Cossarizza et al. have presented a methodology that simultaneously uses 2′,7′-dichlorodihydrofluorescein diacetate (for measuring intracellular H2O2), hydroethidine (for measuring intracellular O2−), monobromobimane (to measure intracellular antioxidants), and TO-PRO-3 stain (to measure cell viability).176 Critically, this approach describes a panel of polychromatic dyes to obtain insight into oxidative stress, antioxidant activity and viability from the same assay, and this can be translated to a variety of cells.
Recommended assays.
Based on the discussions above, we recommend the following as a minimum set of assays (Fig. 6).
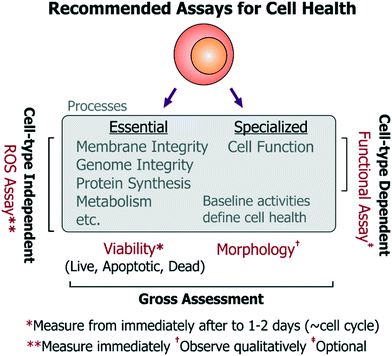 |
| Fig. 6 Recommended minimum viable set of assays to assess cell health in microsystems. For a holistic assessment of cell health, we recommend measuring cell viability by quantifying live, apoptotic and dead cell populations from immediately after exposure to a device environment to ∼1–2 days (which should correspond to a typical cell division timescale). Cell morphology may be assessed qualitatively over this period to check for gross changes in cell state. A ROS assays should be used to get more specific insight into functionality of essential cellular processes (relevant to all cell-types). Additionally, users and designers of cell-type specific technologies should investigate the impact of the device upon specialized cell function using relevant functional assays. | |
• Cell choice: non-transformed cell lines are preferred over cancer cell lines, which may have impaired apoptosis or ROS-responsive pathways and enhanced proliferation mechanisms that can mask cell damage responses. Primary cells may be used if relevant and feasible.
• Viability assay: at the upper and lower limits of the stress dosages, the viability of the chosen cells should be characterized by an assay that reports on live, apoptotic, as well as necrotic cell populations from immediately after treatment to 1–2 days (spanning a typical population doubling time). Performing this assay over time will also provide information regarding differences in proliferation rate and upon possible cell cycle arrest (which would occur ∼cell cycle timescale). Importantly, since cell division does not always imply a healthy state (e.g. endothelial cell proliferation is not a physiological property in vivo), it is more informative to assess viability (important to all cells), while the time-series measurements will reflect upon any changes in proliferation. The assay should be repeated and contrasted with identical cells cultured in the incubator as reference controls, and optionally with a secondary cell type to characterize any bias emerging from background phenotype sensitivity. Altogether, these experiments should provide a nuanced view of cell viability and proliferation, which have typically been measured by the community.
• ROS assay: as mentioned previously, stress-induced ROS can damage a number of essential cell processes in all cell types, which can then impair cell function. To characterize activation of sub-lethal cell-stress mechanisms, intracellular ROS levels should be quantified (e.g. by fluorescent molecular probes) immediately after cells experience the device microenvironment. This may be performed using a variety of commercially available broad-spectrum (low-specificity, high-sensitivity) ROS probes, as the specific ROS identity may not be so informative to the end-user. As an alternative to using commercial ROS probes, one may also use other approaches to report on ROS. For instance, our open-source cell-based stress sensors can be used since they are not only sensitive to ROS, but they also report on stimulus-specific stress pathways that are important for all mammalian cell types. In either case, device-exposed cells should be compared against untreated-culture controls, as well as cells treated with chemical agonists acting as positive/negative controls for the assay. Using these experiments, device conditions that minimize ROS generation and maintain viable cultures can be identified iteratively. These suggested assays are most relevant for devices not dedicated for any particular phenotypes, but are equally pertinent for devices working with rare or primary cells where conditions may first need to be optimized using conveniently-accessible surrogate cells.
• Morphology (observe qualitatively): using microscopy, cell morphology should be assessed against controls to check for drastic effects on cell health, however such observations should be considered qualitative as sub-lethal insults do not always present themselves as morphological changes. Moreover, quantifying morphological changes can lead to subjectivity in assessment of cell health within a platform.
• Functional assays: functional assays should also be identified and measured (e.g. action potentials for neurons, barrier permeability for vascular endothelial cells, albumin production from hepatocytes, etc.) for devices designed for phenotype-specific applications. While functional assays will be useful in assessing the impact of a particular device condition on the cell type of interest, such functional assays will inherently differ in complexity for different cells. Such complexity can make it challenging to iteratively optimize device conditions, but is essential for such devices.
Careful data interpretation.
Subjecting a cell to a device environment is likely going to change the cell state. Whether the change is for the better or worse is often challenging to assess, and it depends on careful execution and interpretation of cellular assays. In many studies, cells experiencing device conditions have been compared against standard tissue culture controls and the similarities and discrepancies in the context of cell health have been reported.50,51,177 Such differences ought to be investigated and explained thoroughly, and if possible, biological phenomenon observed should be contrasted to that in relevant in vivo microenvironments. It is possible that cells appear to function ‘better’ within the device microenvironment and such cases should be supported with evidence negating technical artifacts. Furthermore, even when multiparametric assays are utilized to measure cell health in microsystems, the device designers and users should exercise caution before declaring that a device microenvironment may be universally safe for all cells. We believe careful practices will assist the microsystems community in developing platforms that minimize harm to cells, and additionally, enable effective translation and widespread adoption of such platforms.
Outlook
Understanding cell stress and its quantification is undoubtedly a challenge, given the various complexities highlighted in this review. We anticipate that our review will encourage device designers and users to not seek absolute values of device dimensions, or geometries, or specific operating conditions (such as flow rates, voltages, etc.) as ‘universal solutions’ for keeping cells unstressed in their device. Instead, we believe that by following our guidelines and recommended assays, the microsystem designer should be able to now comprehensively identify stress-inducing phenomena in their device and understand the association of those stressors to cell health. Furthermore, using our framework definitions of cell health and stressors, designers should be able to adapt and apply relevant cell health assays highlighted within this review. Our review also provides recommended quantitative assays which we believe will assist designers and users who are looking for convenient and representative approaches for assessing their devices without having to design detailed cell biological studies. Critically, these assays will allow designers and users discover conditions that minimize cell stresses in their particular device context without limiting their device utility. Collectively, this approach will allow the microsystems community to add ‘cell health’ to their bag of optimization parameters. In this way, we anticipate this review to help readers investigate the impact of their devices on cell health based on its design and usage, prior to drawing biological conclusions or evaluating device utility for its intended applications.
Finally, while new and adaptable solutions to assess cell health will continue to emerge, using current tools the microfluidics community can still benefit from establishing standards and guidelines to measure and report on cell states. We believe that as researchers follow our recommended guidelines, and as reviewers expect and establish standardization, we as a community can assemble a coherent set of device design and usage principles that will guide the field forward.
Conflicts of interest
There are no conflicts to declare.
Acknowledgements
This work was supported by the NIH (GM090194). The authors acknowledge MIT Libraries and its license with Springer, Wiley, AIP and ACS journals to adapt and reproduce figures from their respective manuscripts: http://libraries.mit.edu/scholarly/publishing/using-published-figures/. SV was supported by MIT-GSK Gertrude B. Elion Research Fellowship.
Notes and references
- J. El-Ali, P. K. Sorger and K. F. Jensen, Nature, 2006, 442, 403–411 CrossRef CAS PubMed.
- S. N. Bhatia and D. E. Ingber, Nat. Biotechnol., 2014, 32, 760–772 CrossRef CAS PubMed.
- Y. C. Toh, K. Blagovic and J. Voldman, Integr. Biol., 2010, 2, 305–325 RSC.
- F. Guilak, D. M. Cohen, B. T. Estes, J. M. Gimble, W. Liedtke and C. S. Chen, Cell Stem Cell, 2009, 5, 17–26 CrossRef CAS PubMed.
- E. W. Raines, Int. J. Exp. Pathol., 2000, 81, 173–182 CrossRef CAS PubMed.
- C. A. Mullen, T. J. Vaughan, K. L. Billiar and L. M. McNamara, Biophys. J., 2015, 108, 1604–1612 CrossRef CAS PubMed.
- N. D. Leipzig and M. S. Shoichet, Biomaterials, 2009, 30, 6867–6878 CrossRef CAS PubMed.
- D. E. Discher, P. Janmey and Y. L. Wang, Science, 2005, 310, 1139–1143 CrossRef CAS PubMed.
- Q. Chen, P. Shou, C. Zheng, M. Jiang, G. Cao, Q. Yang, J. Cao, N. Xie, T. Velletri, X. Zhang, C. Xu, L. Zhang, H. Yang, J. Hou, Y. Wang and Y. Shi, Cell Death Differ., 2016, 23, 1128–1139 CrossRef CAS PubMed.
- J. A. Green and K. M. Yamada, Adv. Drug Delivery Rev., 2007, 59, 1293–1298 CrossRef CAS PubMed.
- F. Pampaloni, E. G. Reynaud and E. H. Stelzer, Nat. Rev. Mol. Cell Biol., 2007, 8, 839–845 CrossRef CAS PubMed.
- R. V. Rao, H. M. Ellerby and D. E. Bredesen, Cell Death Differ., 2004, 11, 372–380 CrossRef CAS PubMed.
- C. Xu, B. Bailly-Maitre and J. C. Reed, J. Clin. Invest., 2005, 115, 2656–2664 CrossRef CAS PubMed.
- A. S. Lee, Trends Biochem. Sci., 2001, 26, 504–510 CrossRef CAS PubMed.
- J. Yun, C. Rago, I. Cheong, R. Pagliarini, P. Angenendt, H. Rajagopalan, K. Schmidt, J. K. Willson, S. Markowitz, S. Zhou, L. A. Diaz, Jr., V. E. Velculescu, C. Lengauer, K. W. Kinzler, B. Vogelstein and N. Papadopoulos, Science, 2009, 325, 1555–1559 CrossRef CAS PubMed.
- M. Burotto, V. L. Chiou, J. M. Lee and E. C. Kohn, Cancer, 2014, 120, 3446–3456 CrossRef CAS PubMed.
- M. Barkett and T. D. Gilmore, Oncogene, 1999, 18, 6910–6924 CrossRef CAS PubMed.
- A. Castellheim, O. L. Brekke, T. Espevik, M. Harboe and T. E. Mollnes, Scand. J. Immunol., 2009, 69, 479–491 CrossRef CAS PubMed.
- K. L. Rock, E. Latz, F. Ontiveros and H. Kono, Annu. Rev. Immunol., 2010, 28, 321–342 CrossRef CAS PubMed.
- C. Dusny, A. Grunberger, C. Probst, W. Wiechert, D. Kohlheyer and A. Schmid, Lab Chip, 2015, 15, 1822–1834 RSC.
- S. H. Au, R. Fobel, S. P. Desai, J. Voldman and A. R. Wheeler, Integr. Biol., 2013, 5, 1014–1025 RSC.
- H. W. Wu, C. C. Lin and G. B. Lee, Biomicrofluidics, 2011, 5, 13401 CrossRef PubMed.
- Y. C. Toh, T. C. Lim, D. Tai, G. Xiao, D. van Noort and H. Yu, Lab Chip, 2009, 9, 2026–2035 RSC.
- P. M. van Midwoud, E. Verpoorte and G. M. Groothuis, Integr. Biol., 2011, 3, 509–521 RSC.
- W. J. Polacheck, R. Li, S. G. Uzel and R. D. Kamm, Lab Chip, 2013, 13, 2252–2267 RSC.
- D. Huh, Y. S. Torisawa, G. A. Hamilton, H. J. Kim and D. E. Ingber, Lab Chip, 2012, 12, 2156–2164 RSC.
- D. Huh, B. D. Matthews, A. Mammoto, M. Montoya-Zavala, H. Y. Hsin and D. E. Ingber, Science, 2010, 328, 1662–1668 CrossRef CAS PubMed.
- M. M. Wang, E. Tu, D. E. Raymond, J. M. Yang, H. Zhang, N. Hagen, B. Dees, E. M. Mercer, A. H. Forster, I. Kariv, P. J. Marchand and W. F. Butler, Nat. Biotechnol., 2005, 23, 83–87 CrossRef CAS PubMed.
- J. R. Kovac and J. Voldman, Anal. Chem., 2007, 79, 9321–9330 CrossRef CAS PubMed.
- T. D. Perroud, J. N. Kaiser, J. C. Sy, T. W. Lane, C. S. Branda, A. K. Singh and K. D. Patel, Anal. Chem., 2008, 80, 6365–6372 CrossRef CAS PubMed.
- P. R. Gascoyne, X. B. Wang, Y. Huang and F. F. Becker, IEEE Trans. Ind. Appl., 1997, 33, 670–678 CrossRef PubMed.
- M. D. Vahey and J. Voldman, Anal. Chem., 2008, 80, 3135–3143 CrossRef CAS PubMed.
- B. M. Taff and J. Voldman, Anal. Chem., 2005, 77, 7976–7983 CrossRef CAS PubMed.
- J. D. Adams, U. Kim and H. T. Soh, Proc. Natl. Acad. Sci. U. S. A., 2008, 105, 18165–18170 CrossRef CAS PubMed.
- N. Pamme and C. Wilhelm, Lab Chip, 2006, 6, 974–980 RSC.
- N. Xia, T. P. Hunt, B. T. Mayers, E. Alsberg, G. M. Whitesides, R. M. Westervelt and D. E. Ingber, Biomed. Microdevices, 2006, 8, 299–308 CrossRef CAS PubMed.
- T. Franke, S. Braunmuller, L. Schmid, A. Wixforth and D. A. Weitz, Lab Chip, 2010, 10, 789–794 RSC.
- P. Augustsson, C. Magnusson, M. Nordin, H. Lilja and T. Laurell, Anal. Chem., 2012, 84, 7954–7962 CrossRef CAS PubMed.
- S. S. Kuntaegowdanahalli, A. A. Bhagat, G. Kumar and I. Papautsky, Lab Chip, 2009, 9, 2973–2980 RSC.
- A. J. Mach and D. Di Carlo, Biotechnol. Bioeng., 2010, 107, 302–311 CrossRef CAS PubMed.
- C. J. Easley, J. M. Karlinsey, J. M. Bienvenue, L. A. Legendre, M. G. Roper, S. H. Feldman, M. A. Hughes, E. L. Hewlett, T. J. Merkel, J. P. Ferrance and J. P. Landers, Proc. Natl. Acad. Sci. U. S. A., 2006, 103, 19272–19277 CrossRef CAS PubMed.
- J. S. Marcus, W. F. Anderson and S. R. Quake, Anal. Chem., 2006, 78, 956–958 CrossRef CAS PubMed.
- A. K. White, K. A. Heyries, C. Doolin, M. Vaninsberghe and C. L. Hansen, Anal. Chem., 2013, 85, 7182–7190 CrossRef CAS PubMed.
- M. A. McClain, C. T. Culbertson, S. C. Jacobson, N. L. Allbritton, C. E. Sims and J. M. Ramsey, Anal. Chem., 2003, 75, 5646–5655 CrossRef CAS PubMed.
- J. F. Edd, D. Di Carlo, K. J. Humphry, S. Koster, D. Irimia, D. A. Weitz and M. Toner, Lab Chip, 2008, 8, 1262–1264 RSC.
- M. Chabert and J. L. Viovy, Proc. Natl. Acad. Sci. U. S. A., 2008, 105, 3191–3196 CrossRef CAS PubMed.
- E. Z. Macosko, A. Basu, R. Satija, J. Nemesh, K. Shekhar, M. Goldman, I. Tirosh, A. R. Bialas, N. Kamitaki, E. M. Martersteck, J. J. Trombetta, D. A. Weitz, J. R. Sanes, A. K. Shalek, A. Regev and S. A. McCarroll, Cell, 2015, 161, 1202–1214 CrossRef CAS PubMed.
- L. Mazutis, J. Gilbert, W. L. Ung, D. A. Weitz, A. D. Griffiths and J. A. Heyman, Nat. Protoc., 2013, 8, 870–891 CrossRef CAS PubMed.
- R. Gomez-Sjoberg, A. A. Leyrat, D. M. Pirone, C. S. Chen and S. R. Quake, Anal. Chem., 2007, 79, 8557–8563 CrossRef PubMed.
- V. Lecault, M. Vaninsberghe, S. Sekulovic, D. J. Knapp, S. Wohrer, W. Bowden, F. Viel, T. McLaughlin, A. Jarandehei, M. Miller, D. Falconnet, A. K. White, D. G. Kent, M. R. Copley, F. Taghipour, C. J. Eaves, R. K. Humphries, J. M. Piret and C. L. Hansen, Nat. Methods, 2011, 8, 581–586 CrossRef CAS PubMed.
- Z. Wang, M. C. Kim, M. Marquez and T. Thorsen, Lab Chip, 2007, 7, 740–745 RSC.
- N. Korin, A. Bransky, U. Dinnar and S. Levenberg, Lab Chip, 2007, 7, 611–617 RSC.
- W. Gu, X. Zhu, N. Futai, B. S. Cho and S. Takayama, Proc. Natl. Acad. Sci. U. S. A., 2004, 101, 15861–15866 CrossRef CAS PubMed.
- L. Kim, M. D. Vahey, H. Y. Lee and J. Voldman, Lab Chip, 2006, 6, 394–406 RSC.
- E. Figallo, C. Cannizzaro, S. Gerecht, J. A. Burdick, R. Langer, N. Elvassore and G. Vunjak-Novakovic, Lab Chip, 2007, 7, 710–719 RSC.
- T. M. Squires, R. J. Messinger and S. R. Manalis, Nat. Biotechnol., 2008, 26, 417–426 CrossRef CAS PubMed.
- H. Zhang and M. Chiao, J. Med. Biol. Eng., 2015, 35, 143–155 CrossRef PubMed.
- S. Varma and J. Voldman, Lab Chip, 2015, 15, 1563–1573 RSC.
- L. Kim, Y. C. Toh, J. Voldman and H. Yu, Lab Chip, 2007, 7, 681–694 RSC.
- J. Shemesh, I. Jalilian, A. Shi, G. H. Yeoh, M. L. K. Tate and M. E. Warkiani, Lab Chip, 2015, 15, 4114–4127 RSC.
- J. N. Topper and M. A. Gimbrone, Mol. Med. Today, 1999, 5, 40–46 CrossRef CAS PubMed.
- M. Kolnik, L. S. Tsimring and J. Hasty, Lab Chip, 2012, 12, 4732–4737 RSC.
- E. S. Park, A. C. Brown, M. A. DiFeo, T. H. Barker and H. Lu, Lab Chip, 2010, 10, 571–580 RSC.
- Y. C. Toh, C. Zhang, J. Zhang, Y. M. Khong, S. Chang, V. D. Samper, D. van Noort, D. W. Hutmacher and H. R. Yu, Lab Chip, 2007, 7, 302–309 RSC.
- V. Lecault, M. VanInsberghe, S. Sekulovic, D. J. H. F. Knapp, S. Wohrer, W. Bowden, F. Viel, T. McLaughlin, A. Jarandehei, M. Miller, D. Falconnet, A. K. White, D. G. Kent, M. R. Copley, F. Taghipour, C. J. Eaves, R. K. Humphries, J. M. Piret and C. L. Hansen, Nat. Methods, 2011, 8, 581–U593 CrossRef CAS PubMed.
- L. G. Villa-Diaz, Y. S. Torisawa, T. Uchida, J. Ding, N. C. Nogueira-De-Souza, K. S. O'Shea, S. Takayama and G. D. Smith, Lab Chip, 2009, 9, 1749–1755 RSC.
- D. Titmarsh, A. Hidalgo, J. Turner, E. Wolvetang and J. Cooper-White, Biotechnol. Bioeng., 2011, 108, 2894–2904 CrossRef CAS PubMed.
- R. Yoshimitsu, K. Hattori, S. Sugiura, Y. Kondo, R. Yamada, S. Tachikawa, T. Satoh, A. Kurisaki, K. Ohnuma, M. Asashima and T. Kanamori, Biotechnol. Bioeng., 2014, 111, 937–947 CrossRef CAS PubMed.
- P. F. Davies, Physiol. Rev., 1995, 75, 519–560 CrossRef CAS PubMed.
- M. Mollet, R. Godoy-Silva, C. Berdugo and J. J. Chalmers, Biotechnol. Bioeng., 2007, 98, 772–788 CrossRef CAS PubMed.
- S. Regmi, A. Fu and K. Q. Luo, Sci. Rep., 2017, 7, 39975 CrossRef CAS PubMed.
- D. Massai, E. Bolesani, D. R. Diaz, C. Kropp, H. Kempf, C. Halloin, U. Martin, T. Braniste, G. Isu, V. Harms, U. Morbiducci, G. Drager and R. Zweigerdt, Sci. Rep., 2017, 7, 3950 CrossRef PubMed.
- A. M. Skelley and J. Voldman, Lab Chip, 2008, 8, 1733–1737 RSC.
- S. Halldorsson, E. Lucumi, R. Gomez-Sjoberg and R. M. T. Fleming, Biosens. Bioelectron., 2015, 63, 218–231 CrossRef CAS PubMed.
- Y. S. Heo, L. M. Cabrera, J. W. Song, N. Futai, Y. C. Tung, G. D. Smith and S. Takayama, Anal. Chem., 2007, 79, 1126–1134 CrossRef CAS PubMed.
- A. W. Tilles, H. Baskaran, P. Roy, M. L. Yarmush and M. Toner, Biotechnol. Bioeng., 2001, 73, 379–389 CrossRef CAS PubMed.
- G. A. Ledezma, A. Folch, S. N. Bhatia, U. J. Balis, M. L. Yarmush and M. Toner, J. Biomech. Eng., 1999, 121, 58–64 CrossRef CAS PubMed.
- T. Ezashi, P. Das and R. M. Roberts, Proc. Natl. Acad. Sci. U. S. A., 2005, 102, 4783–4788 CrossRef CAS PubMed.
- L. Studer, M. Csete, S. H. Lee, N. Kabbani, J. Walikonis, B. Wold and R. McKay, J. Neurosci., 2000, 20, 7377–7383 CrossRef CAS PubMed.
- A. L. Paguirigan and D. J. Beebe, Integr. Biol., 2009, 1, 182–195 RSC.
- E. Brouzes, M. Medkova, N. Savenelli, D. Marran, M. Twardowski, J. B. Hutchison, J. M. Rothberg, D. R. Link, N. Perrimon and M. L. Samuels, Proc. Natl. Acad. Sci. U. S. A., 2009, 106, 14195–14200 CrossRef CAS PubMed.
- Y. C. Toh and J. Voldman, FASEB J., 2011, 25, 1208–1217 CrossRef CAS PubMed.
- U. M. Lim, M. G. Yap, Y. P. Lim, L. T. Goh and S. K. Ng, J. Proteome Res., 2013, 12, 3496–3510 CrossRef CAS PubMed.
- S. M. Bjork, S. L. Sjostrom, H. Andersson-Svahn and H. N. Joensson, Biomicrofluidics, 2015, 9, 044128 CrossRef PubMed.
- K. J. Regehr, M. Domenech, J. T. Koepsel, K. C. Carver, S. J. Ellison-Zelski, W. L. Murphy, L. A. Schuler, E. T. Alarid and D. J. Beebe, Lab Chip, 2009, 9, 2132–2139 RSC.
- J. Zhou, A. V. Ellis and N. H. Voelcker, Electrophoresis, 2010, 31, 2–16 CrossRef CAS PubMed.
- E. W. Young and D. J. Beebe, Chem. Soc. Rev., 2010, 39, 1036–1048 RSC.
- E. Tkachenko, E. Gutierrez, S. K. Saikin, P. Fogelstrand, C. Kim, A. Groisman and M. H. Ginsberg, Biol. Open, 2013, 2, 1007–1012 CrossRef CAS PubMed.
- C. B. Rohde, F. Zeng, R. Gonzalez-Rubio, M. Angel and M. F. Yanik, Proc. Natl. Acad. Sci. U. S. A., 2007, 104, 13891–13895 CrossRef CAS PubMed.
- M. M. Frigault, J. Lacoste, J. L. Swift and C. M. Brown, J. Cell Sci., 2009, 122, 753–767 CrossRef CAS PubMed.
- V. Magidson and A. Khodjakov, Methods Cell Biol., 2013, 114, 545–560 Search PubMed.
- C. Kielbassa, L. Roza and B. Epe, Carcinogenesis, 1997, 18, 811–816 CrossRef CAS PubMed.
- D. R. Albrecht, V. L. Tsang, R. L. Sah and S. N. Bhatia, Lab Chip, 2005, 5, 111–118 RSC.
- D. Dendukuri, S. S. Gu, D. C. Pregibon, T. A. Hatton and P. S. Doyle, Lab Chip, 2007, 7, 818–828 RSC.
- M. Castellarnau, G. L. Szeto, H. W. Su, T. Tokatlian, J. C. Love, D. J. Irvine and J. Voldman, Small, 2015, 11, 489–498 CrossRef CAS PubMed.
- X. Wang, S. Chen, M. Kong, Z. Wang, K. D. Costa, R. A. Li and D. Sun, Lab Chip, 2011, 11, 3656–3662 RSC.
- A. Y. Lau, L. P. Lee and J. W. Chan, Lab Chip, 2008, 8, 1116–1120 RSC.
- E. Eriksson, J. Enger, B. Nordlander, N. Erjavec, K. Ramser, M. Goksor, S. Hohmann, T. Nystrom and D. Hanstorp, Lab Chip, 2007, 7, 71–76 RSC.
- S. Skylaki, O. Hilsenbeck and T. Schroeder, Nat. Biotechnol., 2016, 34, 1137–1144 CrossRef CAS PubMed.
- C. M. Brown, J. Cell Sci., 2007, 120, 1703–1705 CrossRef CAS PubMed.
- J. Ge, D. K. Wood, D. M. Weingeist, S. Prasongtanakij, P. Navasumrit, M. Ruchirawat and B. P. Engelward, Cytometry, Part A, 2013, 83, 552–560 CrossRef PubMed.
- M. Purschke, N. Rubio, K. D. Held and R. W. Redmond, Photochem. Photobiol. Sci., 2010, 9, 1634–1639 RSC.
- S. Waldchen, J. Lehmann, T. Klein, S. van de Linde and M. Sauer, Sci. Rep., 2015, 5, 15348 CrossRef PubMed.
- E. J. Peterman, F. Gittes and C. F. Schmidt, Biophys. J., 2003, 84, 1308–1316 CrossRef CAS PubMed.
- Y. Liu, G. J. Sonek, M. W. Berns and B. J. Tromberg, Biophys. J., 1996, 71, 2158–2167 CrossRef CAS PubMed.
- A. Castellanos, A. Ramos, A. González, N. G. Green and H. Morgan, J. Phys. D: Appl. Phys., 2003, 36, 2584 CrossRef CAS.
- C. C. Hsieh, S. B. Huang, P. C. Wu, D. B. Shieh and G. B. Lee, Biomed. Microdevices, 2009, 11, 903–913 CrossRef PubMed.
- P. Ginet, K. Montagne, S. Akiyama, A. Rajabpour, A. Taniguchi, T. Fujii, Y. Sakai, B. Kim, D. Fourmy and S. Volz, Lab Chip, 2011, 11, 1513–1520 RSC.
- S. P. Desai and J. Voldman, Integr. Biol., 2011, 3, 48–56 RSC.
- J. L. Sylman, D. T. Artzer, K. Rana and K. B. Neeves, Integr. Biol., 2015, 7, 801–814 RSC.
- C. Y. Lee, G. B. Lee, J. L. Lin, F. C. Huang and C. S. Liao, J. Micromech. Microeng., 2005, 15, 1215–1223 CrossRef CAS.
- L. Gasperini, J. F. Mano and R. L. Reis, J. R. Soc., Interface, 2014, 11, 20140817 CrossRef PubMed.
- J. H. Park, B. G. Chung, W. G. Lee, J. Kim, M. D. Brigham, J. Shim, S. Lee, C. M. Hwang, N. G. Durmus, U. Demirci and A. Khademhosseini, Biotechnol. Bioeng., 2010, 106, 138–148 CAS.
- Y. Ling, J. Rubin, Y. Deng, C. Huang, U. Demirci, J. M. Karp and A. Khademhosseini, Lab Chip, 2007, 7, 756–762 RSC.
- A. Lenshof, M. Evander, T. Laurell and J. Nilsson, Lab Chip, 2012, 12, 684–695 RSC.
- B. H. Ha, K. S. Lee, G. Destgeer, J. Park, J. S. Choung, J. H. Jung, J. H. Shin and H. J. Sung, Sci. Rep., 2015, 5, 11851 CrossRef PubMed.
- J. D. Adams, C. L. Ebbesen, R. Barnkob, A. H. J. Yang, H. T. Soh and H. Bruus, J. Micromech. Microeng., 2012, 22, 075017 CrossRef.
- P. Augustsson, R. Barnkob, S. T. Wereley, H. Bruus and T. Laurell, Lab Chip, 2011, 11, 4152–4164 RSC.
- T. Neutelings, C. A. Lambert, B. V. Nusgens and A. C. Colige, PLoS One, 2013, 8, e69687 CrossRef CAS PubMed.
- M. B. Al-Fageeh and C. M. Smales, Biochem. J., 2006, 397, 247–259 CrossRef CAS PubMed.
- T. Byambadorj, E. Dashtimoghadam, M. Malakoutian, B. Davaji, L. Tayebi, J. E. Richie and C. H. Lee, PLoS One, 2017, 12, e0183492 CrossRef PubMed.
- S. Selimovic, J. Oh, H. Bae, M. Dokmeci and A. Khademhosseini, Polymers, 2012, 4, 1554 CrossRef CAS PubMed.
- X. Munoz-Berbel, R. Rodriguez-Rodriguez, N. Vigues, S. Demming, J. Mas, S. Buttgenbach, E. Verpoorte, P. Ortiz and A. Llobera, Lab Chip, 2013, 13, 4239–4247 RSC.
- P. M. van Midwoud, G. M. Groothuis, M. T. Merema and E. Verpoorte, Biotechnol. Bioeng., 2010, 105, 184–194 CrossRef CAS PubMed.
- A. Super, N. Jaccard, M. P. Cardoso Marques, R. J. Macown, L. D. Griffin, F. S. Veraitch and N. Szita, Biotechnol. J., 2016, 11, 1179–1189 CrossRef CAS PubMed.
- D. Di Carlo, D. Irimia, R. G. Tompkins and M. Toner, Proc. Natl. Acad. Sci. U. S. A., 2007, 104, 18892–18897 CrossRef CAS PubMed.
- A. Sharei, J. Zoldan, A. Adamo, W. Y. Sim, N. Cho, E. Jackson, S. Mao, S. Schneider, M. J. Han, A. Lytton-Jean, P. A. Basto, S. Jhunjhunwala, J. Lee, D. A. Heller, J. W. Kang, G. C. Hartoularos, K. S. Kim, D. G. Anderson, R. Langer and K. F. Jensen, Proc. Natl. Acad. Sci. U. S. A., 2013, 110, 2082–2087 CrossRef CAS PubMed.
- J. El-Ali, S. Gaudet, A. Gunther, P. K. Sorger and K. F. Jensen, Anal. Chem., 2005, 77, 3629–3636 CrossRef CAS PubMed.
- J. Y. Kim, H. Park, K. H. Kwon, J. Y. Park, J. Y. Baek, T. S. Lee, H. R. Song, Y. D. Park and S. H. Lee, Biomed. Microdevices, 2008, 10, 11–20 CrossRef CAS PubMed.
- A. H. Yang and H. T. Soh, Anal. Chem., 2012, 84, 10756–10762 CrossRef CAS PubMed.
- E. S. Park, C. Jin, Q. Guo, R. R. Ang, S. P. Duffy, K. Matthews, A. Azad, H. Abdi, T. Todenhofer, J. Bazov, K. N. Chi, P. C. Black and H. Ma, Small, 2016, 12, 1909–1919 CrossRef CAS PubMed.
- P. J. Hung, P. J. Lee, P. Sabounchi, R. Lin and L. P. Lee, Biotechnol. Bioeng., 2005, 89, 1–8 CrossRef CAS PubMed.
- M. J. Stoddart, Methods Mol. Biol., 2011, 740, 1–6 CrossRef CAS PubMed.
- H. Somaweera, S. O. Haputhanthri, A. Ibraguimov and D. Pappas, Analyst, 2015, 140, 5029–5038 RSC.
- L. Liu, K. Loutherback, D. Liao, D. Yeater, G. Lambert, A. Estevez-Torres, J. C. Sturm, R. H. Getzenberg and R. H. Austin, Lab Chip, 2010, 10, 1807–1813 RSC.
- Y. B. Bae, H. K. Jang, T. H. Shin, G. Phukan, T. T. Tran, G. Lee, W. R. Hwang and J. M. Kim, Lab Chip, 2016, 16, 96–103 RSC.
- H. Yin, X. Zhang, N. Pattrick, N. Klauke, H. C. Cordingley, S. J. Haswell and J. M. Cooper, Anal. Chem., 2007, 79, 7139–7144 CrossRef CAS PubMed.
- F. Kurth, A. Franco-Obregon, C. A. Bartschi and P. S. Dittrich, Analyst, 2015, 140, 127–133 RSC.
- S. B. Huang, S. S. Wang, C. H. Hsieh, Y. C. Lin, C. S. Lai and M. H. Wu, Lab Chip, 2013, 13, 1133–1143 RSC.
- S. C. Hur, N. K. Henderson-MacLennan, E. R. McCabe and D. Di Carlo, Lab Chip, 2011, 11, 912–920 RSC.
- H. Song, T. Chen, B. Zhang, Y. Ma and Z. Wang, Biomicrofluidics, 2010, 4, 44104 CrossRef PubMed.
- B. Patra, C. C. Peng, W. H. Liao, C. H. Lee and Y. C. Tung, Sci. Rep., 2016, 6, 21061 CrossRef CAS PubMed.
- D. Wlodkowic, K. Khoshmanesh, J. C. Sharpe, Z. Darzynkiewicz and J. M. Cooper, Anal. Chem., 2011, 83, 6439–6446 CrossRef CAS PubMed.
- E. Leclerc, Y. Sakai and T. Fujii, Biomed. Microdevices, 2003, 5, 109–114 CrossRef CAS.
- J. H. Sung, J. R. Choi, D. Kim and M. L. Shuler, Biotechnol. Bioeng., 2009, 104, 516–525 CrossRef CAS PubMed.
- J. M. Lopacinska, J. Emneus and M. Dufva, PLoS One, 2013, 8, e53107 CrossRef CAS PubMed.
- Y. Wang, C. Phillips, W. Xu, J. H. Pai, R. Dhopeshwarkar, C. E. Sims and N. Allbritton, Lab Chip, 2010, 10, 2917–2924 RSC.
- K. Woodruff, L. M. Fidalgo, S. Gobaa, M. P. Lutolf and S. J. Maerkl, Nat. Methods, 2013, 10, 550–552 CrossRef CAS PubMed.
- W. Zheng, Y. Xie, W. Zhang, D. Wang, W. Ma, Z. Wang and X. Jiang, Integr. Biol., 2012, 4, 1102–1111 RSC.
- S. Y. Wu, H. S. Hou, Y. S. Sun, J. Y. Cheng and K. Y. Lo, Biomicrofluidics, 2015, 9, 054120 CrossRef PubMed.
- W. Zhang and H. T. Liu, Cell Res., 2002, 12, 9–18 CrossRef PubMed.
- M. J. Berridge, M. D. Bootman and H. L. Roderick, Nat. Rev. Mol. Cell Biol., 2003, 4, 517–529 CrossRef CAS PubMed.
- X. Wang, H. Fang, Z. Huang, W. Shang, T. Hou, A. Cheng and H. Cheng, J. Mol. Med., 2013, 91, 917–927 CrossRef CAS PubMed.
- J. F. Lo, E. Sinkala and D. T. Eddington, Lab Chip, 2010, 10, 2394–2401 RSC.
- L. K. Chin, J. Q. Yu, Y. Fu, T. Yu, A. Q. Liu and K. Q. Luo, Lab Chip, 2011, 11, 1856–1863 RSC.
- I. K. Zervantonakis and C. D. Arvanitis, Small, 2016, 12, 2616–2626 CrossRef CAS PubMed.
- L. J. Kuo and L. X. Yang, In Vivo, 2008, 22, 305–309 CAS.
- K. Kotarsky, C. Owman and B. Olde, Anal. Biochem., 2001, 288, 209–215 CrossRef CAS PubMed.
- R. Davidsson, A. Boketoft, J. Bristulf, K. Kotarsky, B. Olde, C. Owman, M. Bengtsson, T. Laurell and J. Emneus, Anal. Chem., 2004, 76, 4715–4720 CrossRef CAS PubMed.
- A. Fendyur, S. Varma, C. T. Lo and J. Voldman, Anal. Chem., 2014, 86, 7598–7605 CrossRef CAS PubMed.
- S. Varma, A. Fendyur, A. Box and J. Voldman, Anal. Chem., 2017, 89, 4663–4670 CrossRef CAS PubMed.
- R. Riahi, S. A. Shaegh, M. Ghaderi, Y. S. Zhang, S. R. Shin, J. Aleman, S. Massa, D. Kim, M. R. Dokmeci and A. Khademhosseini, Sci. Rep., 2016, 6, 24598 CrossRef CAS PubMed.
- Q. Luan, S. Cahoon, A. Wu, S. S. Bale, M. Yarmush and A. Bhushan, Biomed. Microdevices, 2017, 19, 101 CrossRef PubMed.
- H. Zhu, G. Stybayeva, M. Macal, E. Ramanculov, M. D. George, S. Dandekar and A. Revzin, Lab Chip, 2008, 8, 2197–2205 RSC.
- R. Fan, O. Vermesh, A. Srivastava, B. K. Yen, L. Qin, H. Ahmad, G. A. Kwong, C. C. Liu, J. Gould, L. Hood and J. R. Heath, Nat. Biotechnol., 2008, 26, 1373–1378 CrossRef CAS PubMed.
- Y. Liu, T. Kwa and A. Revzin, Biomaterials, 2012, 33, 7347–7355 CrossRef CAS PubMed.
- X. An, V. G. Sendra, I. Liadi, B. Ramesh, G. Romain, C. Haymaker, M. Martinez-Paniagua, Y. Lu, L. G. Radvanyi, B. Roysam and N. Varadarajan, PLoS One, 2017, 12, e0181904 CrossRef PubMed.
- V. Chokkalingam, J. Tel, F. Wimmers, X. Liu, S. Semenov, J. Thiele, C. G. Figdor and W. T. Huck, Lab Chip, 2013, 13, 4740–4744 RSC.
- Y. F. S. Seah, H. Hu and C. A. Merten, Mol. Aspects Med., 2018, 59, 47–61 CrossRef CAS PubMed.
- L. M. Przybyla and J. Voldman, Proc. Natl. Acad. Sci. U. S. A., 2012, 109, 835–840 CrossRef CAS PubMed.
- J. Kovac, Y. Gerardin and J. Voldman, Adv. Healthcare Mater., 2013, 2, 552–556 CrossRef CAS PubMed.
- B. Dura, M. M. Servos, R. M. Barry, H. L. Ploegh, S. K. Dougan and J. Voldman, Proc. Natl. Acad. Sci. U. S. A., 2016, 113, E3599–3608 CrossRef CAS PubMed.
- A. K. White, M. VanInsberghe, O. I. Petriv, M. Hamidi, D. Sikorski, M. A. Marra, J. Piret, S. Aparicio and C. L. Hansen, Proc. Natl. Acad. Sci. U. S. A., 2011, 108, 13999–14004 CrossRef CAS PubMed.
- A. J. Hughes, D. P. Spelke, Z. Xu, C. C. Kang, D. V. Schaffer and A. E. Herr, Nat. Methods, 2014, 11, 749–755 CrossRef CAS PubMed.
- J. M. Barnes, J. T. Nauseef and M. D. Henry, PLoS One, 2012, 7, e50973 CrossRef PubMed.
- A. Cossarizza, R. Ferraresi, L. Troiano, E. Roat, L. Gibellini, L. Bertoncelli, M. Nasi and M. Pinti, Nat. Protoc., 2009, 4, 1790–1797 CrossRef CAS PubMed.
- I. Barbulovic-Nad, S. H. Au and A. R. Wheeler, Lab Chip, 2010, 10, 1536–1542 RSC.
|
This journal is © The Royal Society of Chemistry 2018 |
Click here to see how this site uses Cookies. View our privacy policy here.