DOI:
10.1039/C8LC00498F
(Paper)
Lab Chip, 2018,
18, 2410-2424
Quantitative and multiplex microRNA assays from unprocessed cells in isolated nanoliter well arrays†
Received
15th May 2018
, Accepted 4th July 2018
First published on 6th July 2018
Abstract
MicroRNAs (miRNAs) have recently emerged as promising biomarkers for the profiling of diseases. Translation of miRNA biomarkers to clinical practice, however, remains a challenge due to the lack of analysis platforms for sensitive, quantitative, and multiplex miRNA assays that have simple and robust workflows suitable for translation. The platform we present here utilizes functionalized hydrogel posts contained within isolated nanoliter well reactors for quantitative and multiplex assays directly from unprocessed cell samples without the need of prior nucleic acid extraction. Simultaneous reactor isolation and delivery of miRNA extraction reagents is achieved by sealing an array of wells containing the functionalized hydrogel posts and cells against another array of wells containing lysis and extraction reagents. The nanoliter well array platform features >100× better sensitivity compared to previous technology utilizing hydrogel particles without relying on signal amplification and enables >100 parallel assays in a single device. These advances provided by this platform lay the groundwork for translatable and robust analysis technologies for miRNA expression profiling in samples with small populations of cells and in precious, material-limited samples.
Introduction
MicroRNAs (miRNAs) are small (∼22 nucleotides), non-coding RNAs that regulate gene expression and are involved in multiple biological processes.1,2 Many miRNAs expressed in humans are known to be dysregulated in diseases such as diabetes,3 cardiovascular disease,4,5 neurodegenerative diseases,6–8 and lung,9–11 ovarian,12 prostate,13 and other cancers.14,15 miRNAs have emerged as promising disease biomarkers14 because of their higher stability compared to mRNA in cells and bodily fluids16–20 as well as their tissue specificity. Because of the burstiness21,22 of mRNA expression, miRNAs also provide higher information content than mRNA markers in terminal and single time point assays.23 Despite this promise, translation of miRNA to clinical diagnostics has been challenging.17,24,25 Multiple miRNAs are dysregulated in disease tissue compared to normal tissue and thus miRNA panels are typically used for accurate profiling in targeted assays.13,26–29 Therefore, for miRNAs assays to have clinical utility, they need to have multiplexing capabilities and be quantitative across several orders of magnitude in concentration, in addition to having simple, robust workflows suitable for translation.17,25,30
Unfortunately, traditional miRNA analysis techniques are time-consuming, lack multiplexing, throughput, or both, and have clinically impractical assay workflows.31 Simple workflows and compatibility with a wide range of samples are desired, but most existing miRNA analysis technologies require prior nucleic acid extraction and total RNA isolation in order to reduce fouling, remove undesired biological material, and maintain the activity of enzymes used during the assay.32–35 Quantitative real-time reverse-transcription polymerase chain reaction (qRT-PCR) has high sensitivity, but has limited multiplexing and requires extensive sample processing prior to the assay.17 Additionally, primer design requires consideration in qRT-PCR, as target amplification can be affected by sequence bias.25,36 Microarrays enable multiplexing, but also require extensive sample preparation and suffer from long assay times.17,37In situ hybridization is low-throughput, not quantitative,38,39 and for miRNA specifically, only single-plex assays have been developed.31,40 While techniques such as RNA-seq are emerging as powerful tools to elucidate heterogeneity at the gene expression level, they have multiple drawbacks for miRNA analysis specifically, such as limited multiplexing, amplification artifacts, and the need for extensive sample preparation, which limits their applicability to clinical practice and some research questions.17,24,41 Other approaches such as using biosensors to visualize miRNA in living cells suffer from low sensitivity and cumbersome workflows.42 Given this technological gap, there is need for miRNA analysis technologies that have high sensitivity, multiplexing capabilities, and simple workflows that are compatible with complex samples.
miRNA hybridization assays performed with polyethylene glycol diacrylate (PEGDA) hydrogel particles43–46 have demonstrated robust, quantitative analysis from complex samples such as blood serum47 and more recently, unprocessed cells.48 The PEGDA particles were made using stop-flow lithography49,50 and were functionalized with DNA probes complimentary to miRNA targets. PEGDA particles have been developed not only for miRNA assays, but also for detection of other biomolecules such as proteins.51,52 Multiplexing is achieved by including barcoded particles with probes complimentary to different miRNA targets into the same reaction.50 The hydrogel matrix allows for higher probe densities and an aqueous reaction environment that more closely resembles free solution binding kinetics compared to surface-based binding substrates, leading to more efficient and sensitive assays.43,53–55 Additionally, the inert PEGDA matrix is resistant to fouling and the miRNA hybridization assay is compatible with cell lysis and miRNA extraction reagents as well as other components present in the unprocessed samples. For assays from unprocessed cells, the hydrogel particles, cells, hybridization buffer, sodium dodecyl sulfate (SDS), and proteinase K are all added into a single reactor for miRNA extraction and hybridization, with no prior sample preparation required. SDS is included in the buffer to not only lyse cells, but along with proteinase K to also free miRNA from its associated protein complexes.17 miRNA targets captured by the probes linked to the PEGDA hydrogel are then fluorescently labeled for quantitative readout, with the measured signal being proportional to the amount of target miRNA present in the sample. In recent work, the particle-based assay was used to quantify miRNA from unprocessed cell lysates and was shown to have a lower limit of detection (LLOD) of ∼1000 3T3 cells (∼2.5 amol of miRNA) in a 50 μL sample volume.48 While powerful and satisfying many of the crucial needs for clinical applicability17 (namely specificity, reproducibility, multiplexing, quantitation across a wide dynamic range, simple and robust protocol, and compatibility with unprocessed samples), the assay does have some limitations. Because of its LLOD of ∼1000 cells, it is not suitable to look at samples with smaller cell numbers such as 3D spheroids (∼10–10
000 cells),56–58 circulating cell clusters (∼10–100 cells)59,60 organoids (∼1000–10
000 cells),61 early stage embryos (∼10–100 cells),62,63 small whole organisms (∼1000 cells),64 as well as precious, material-limited biopsies. As an example, fine-needle aspiration biopsies may sometimes yield <1000 cells per patient, in which case the ∼2.5 amol LLOD of the particle assay would be inadequate.65 Additionally, because one assay is performed per 50 μL reactor in a single tube, scalability becomes challenging when there is a need to analyze high numbers of samples, such as when assessing heterogeneity in specimen populations. Consequently, due to the lack of sensitive, quantitative, and multiplex miRNA assays with high-throughput and translatable workflows, there are major technical hurdles to quantitatively profile miRNA signatures from samples with small numbers of cells. To address this gap, we introduce here the development of a miRNA quantification platform with the capacity to perform parallel, multiplex, and sensitive miRNA measurements in isolated μm-scale wells that can be applied to unprocessed cell samples with no prior sample preparation (i.e. without nucleic acid extraction).
In our approach presented here, we use nanoliter wells arranged in a dense array to compartmentalize separate nanoliter-volume reactions. In our implementation, the use of well arrays allows for parallel assays in a single device, instead of one assay per tube as done previously miRNA assays done with PEGDA particles,43,45–48 therefore increasing throughput and scalability. PEGDA posts functionalized with DNA probes complimentary to miRNA targets are affixed within the nanoliter wells instead of having free particles introduced into a tube. The μm-scale posts are formed using projection photolithography, as has been demonstrated in similar approaches for attaching PEGDA posts inside microfluidic channels.66 Multiplexing in this configuration is achieved by having multiple posts within a single well, with each post containing probes complimentary to different miRNA targets. The miniaturization of the hybridization reaction in our assay has additionally enabled more sensitive assays, as assay efficiency is enhanced23 by decreasing the total sensor surface used in the particle while leveraging the short diffusive mass transport times within the nanoliter reactor volumes, as detailed later.
Nanoliter wells have been used in other applications to isolate cells,67 to measure cell response to chemical stimuli,68 for antibody screening,69 for analysis of intracellular70–72 and secreted73 proteins, and to measure nucleic acids such as mRNA.74 Our platform enables quantification of miRNA following lysis of unprocessed cells in isolated wells. Aqueous droplets in oil are another approach to isolate nanoliter-volume reactors for biological analysis.44,66,75–77 While droplet platforms generally have advantages in throughput, their need for pumps, precise fluidic controls, and careful engineering design considerations has limited their broad adoption.23 Reactor isolation can also be done via valving in microfluidic devices,78–80 but because of their need for dedicated equipment and limited density, these devices are better suited for applications with less parallelization and more complex workflows.23 Thus, well array-based platforms are better suited for applications were simplicity is essential, such as assays aiming towards translation and clinical applicability.23 Our platform was designed with these considerations in mind, therefore our assay requires no dedicated equipment for fluidic handling. The planar form factor of well arrays also makes them well-suited for applications in which the spatial arrangement of samples23 is important or the imaging of cells prior to lysis is necessary.81
Well-based assays that detect molecules larger than miRNA, such as mRNA and proteins, from cells, have employed different strategies that allow for the delivery of necessary assay reagents while retaining the target molecules in the reactors. These strategies include the use of semipermeable membranes,74 the application of small pore-size hydrogel films over well arrays that deliver reagents (but hinder target diffusion),70,72 and even the direct application of lysis solution over open wells and leveraging rapid assay times to minimize diffusive losses.71,81 For our miRNA platform, we opted to perform our hybridization assay in isolated well reactors due to the need for not only cell lysis, but also miRNA dissociation from associated proteins17 and the small size (and therefore rapid diffusivity) of miRNA molecules. One challenging aspect of performing assays within isolated reactors is the delivery of reagents for the different assay steps. To address this hurdle, we leveraged that the PEGDA-based hybridization step from unprocessed cells is compatible with unprocessed samples and the reagents for miRNA extraction in a single reactor.48 Thus, we designed our platform to perform simultaneous lysis buffer delivery and reactor isolation in a single step by sandwiching a well array containing both the cells and PEGDA posts (bottom layer) with another well array containing the lysis buffer. Magnets applied to the array sandwich keep the wells sealed, but also allow the seal to be reversible. Slide sandwiches have been previously used for the delivery of antibody to tissue sections,82 reversible seals have been used in microfluidic83 and microwell73 devices, and sandwiches of aligned well arrays have been employed for simultaneous delivery and reactor isolation for cell cytotoxicity and viability assays.84 To our knowledge, our approach presented here is the first demonstration of sandwiches of two well arrays used for reagent delivery, nanoliter reactor sealing, cell lysis, miRNA extraction, and miRNA hybridization in a single step. Following the hybridization step done in the sealed configuration, in which miRNA is released and captured onto the PEGDA hydrogel posts, the sandwich is opened which allows the rest of the assay steps to be done by simply exposing the open wells to the desired solutions. In the work presented here, we detail the design and operation of our platform, as well as characterize its performance for quantitative, multiplex miRNA assays from both synthetic miRNA targets and miRNA extracted from unprocessed cell samples.
Experimental
Well array fabrication
Polyethylene glycol diacrylate (PEGDA) hydrogel posts were covalently attached to glass substrates using methacrylate-terminated silane monolayer formation using standard methods.66,71 The plain glass microscope slides (Thermo Fisher) were cut into desired dimensions using a diamond scribe (Ted Pella) and running and nipping pliers (Fletcher–Terry) and stored under vacuum until usage. Polydimethylsiloxane (PDMS, Sylgard® 184, Dow Corning) molds85 were made using standard soft-lithography protocols by mixing elastomer base and curing agent in a 10
:
1 ratio and cured on a SU-8 (MicroChem) master that was prepared using standard photolithography protocols. Molds were designed to create 1 × 1 cm arrays of wells with diameters of 300 μm and 30 μm and depths of 35 μm and 38.6 μm, respectively (Fig. S1†). The arrays contained indexing marks in place of some wells. The individual 1 × 1 cm PDMS molds were cut using a scalpel and had 1.5 mm inlets punched out (Biopsy Punch, Miltex). Norland Optical Adhesive 81 (NOA81, Thorlabs) well arrays were formed on the acrylated glass slides by filling the PDMS molds using degas-driven flow,86 UV curing the NOA81, and removing the PDMS molds (see ESI,† Fig. S2).
Hydrogel post fabrication
Functionalized PEGDA posts were photopolymerized in the nanoliter wells using projection lithography methods adapted from previously described PEDGA particle and post fabrication protocols45,66 (see ESI†). Prepolymer solution containing 18% (v/v) PEGDA 700, 36% (v/v) PEG 200, 4.5% (v/v) Darocur® 1173 photoinitiator (2-hydroxy-2-methylpropiophenone), ∼1× TE buffer, and DNA probes was loaded into the NOA81 300 μm well array (see ESI†). The well array containing prepolymer solution was then covered with a 1–2 mm flat PDMS film. PEGDA posts were then photopolymerized via projection lithography using mylar transparency masks (Fineline) placed in the field-stop slider between a 365 nm UV LED (Thorlabs) and a 20× EC Plan NeoFluor objective (Zeiss) on a Zeiss AX10 inverted fluorescence microscope. Intensities of 720 mW cm−2 and exposure times of 100–200 ms were used to fabricate circular or square 20–200 μm posts, as specified. Following post photopolymerization, the PDMS film was removed and devices were rinsed with 1× TE with 0.05% (v/v) Tween® 20 (1× TET). Following iterative prepolymer solution loading, post photopolymerization, and wash steps, posts containing different DNA probes were formed within the same well. Devices with functional posts were stored at 4 °C in 1× TET until usage. Hydrogel posts were treated with 500 μM potassium permanganate (Sigma) to oxidize hydrophobic, non-reacted acrylate groups to reduce non-specific binding, as specified.44,66
Cell samples and cell handling
Human lung cancer cell line Calu-6 (ATCC® HTB-56™) cells sourced from ATCC were cultured in Dulbecco's modified Eagle medium (high glucose, GIBCO) with 10% fetal bovine serum, 2 mM L-glutamine, and 1% penicillin–streptomycin. Upon reaching 70% confluence, the cells were treated with 0.25% trypsin–EDTA (Gibco) and then frozen with 10% dimethyl sulfoxide (DMSO) (Sigma) in complete culture medium. Frozen cells were kept in liquid nitrogen for long term storage and −80 °C freezer for short term storage before use. Frozen cells were thawed to remove DMSO and were reconstituted into room temperature media before use (see ESI†). The density of cell suspensions were counted using a Bright-Line™ Hemocytometer (Sigma). Immediately preceding experiments with cells, cells were pelleted and resuspended in settling buffer (1× TE, 137 mM NaCl) at the desired densities. A 5 μL drop of the cell suspension was then applied onto the well array devices and cells were allowed to passively settle for 10 min. Devices were then imaged before analysis in order to count the number of cells settled into each well.
miRNA hybridization assay
The miRNA hybridization assay in the well arrays was adapted from prior work45 (see ESI†). For the hybridization step, the 300 μm well array was sealed against a 30 μm well array using 1.2 × 0.16 cm disk-shaped neodymium magnets (Grainger). Stacks of 3 magnets were placed on each side of the sandwich. The hybridization buffer inside the wells contained 1× TE, 0.05% (v/v) Tween® 20, and 350 mM NaCl. For synthetic miRNA assays, the hybridization solution contained target miRNA. For cell assays, the hybridization buffer contained ∼2% sodium dodecyl sulfate (SDS) and ∼15 U mL−1 of proteinase K for cell lysis and miRNA extraction48 (see ESI†). The hybridization step was done for 90 min at 55 °C (VortTemp™ 56, Labnet). Following hybridization, the magnets were removed and the device was rinsed with 1× TE, 0.05% (v/v) Tween® 20, and 50 mM NaCl (R50) by placing the well array slide face down over a glass slide with ∼500 μm spacers and subsequent solution loading and aspiration steps (Fig. S3†). Then, the ligation step was done by loading ligation buffer containing the biotinylated linker and T4 DNA ligase and incubating for 1 hour at room temperature. Following ligation, the well array was rinsed with R50 and labeling was done by loading R50 buffer containing 10 μg mL−1 streptavidin-R-phycoerythrin (SA-PE, Invitrogen) and incubating for 1 hour at room temperature. Devices were then rinsed with R50 to ensure removal of unbound SA-PE before imaging.
Imaging and data analysis
Brightfield and fluorescence imaging was done using a Zeiss Axio Observer A1 inverted microscope equipped with a X-Cite 120LED light source (Lumen), 5×, 10×, and 20× EC Plan NeoFluor objectives (Zeiss), and an Andor Clara CCD camera. Images were captured using 100% intensity with 50 ms exposures and no binning in Andor Solis software. SA-PE and FDG imaging was done using XF101-2 (λex/λem = 525/565 nm) and XF100-3 (λex/λem = 470/545 nm) filter sets (Omega), respectively. Image analysis was done custom ImageJ (National Institutes of Health) and MATLAB (Mathworks) scripts written in-house.
Results and discussion
Well isolation
The miRNA assays were performed in devices consisting of well arrays made of NOA81 formed on glass slide substrates (Fig. 1A). The devices were comprised of two separate layers that were sandwiched together during the miRNA hybridization step to form isolated reactors (Fig. 1B). Wells with 30 μm diameters were chosen for the top layer to ensure no overlap between reactors when the bottom layer well spacing was 30 μm. When the top array with 30 μm wells was applied onto the 300 μm well array without any alignment, on average 27.5 30 μm wells interface with each 300 μm well (see ESI†). Using the geometries of both wells arrays, the volume of each reactor in the sealed microarray sandwich was ∼3.2 nL. There was no overlap between each reactor because the spacing between each 300 μm is greater than or equal to the spacing between each 30 μm well (Fig. 1C). By performing the miRNA hybridization assay in wells instead of a centrifuge tube (50 μL) the volume of each reaction was reduced by over four orders of magnitude. PEGDA posts functionalized with DNA probes complimentary to specific miRNA targets were photopolymerized within the 300 μm wells and covalently attached to the acrylated glass substrate. (Fig. 1D). The hybridization step (where free miRNA binds to complimentary DNA probes in the PEGDA posts) was performed in the sandwiched configuration. Following hybridization, the sandwich was opened and subsequent steps were performed by incubating the 300 μm array in the specified solutions (Fig. S3†). During ligation, biotinylated linkers were ligated to the captured miRNA targets.45 SA-PE was then introduced which binds to the biotinylated linkers and fluorescently labels the captured targets (Fig. 1E).
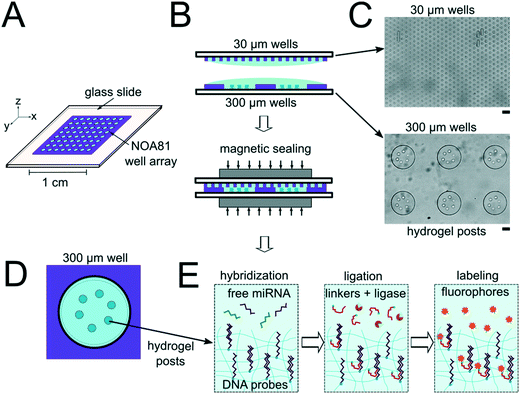 |
| Fig. 1 Device and assay overview. (A) Well array on glass slide schematic. (B) Side view of the isolated reactor formation using nanoliter well arrays. (C) Bright field images of the top (30 μm wells) and bottom (300 μm wells) arrays. (D) Schematic of well in the array containing PEDGA posts functionalized with DNA probes complimentary to miRNA targets. (E) Hybridization and ligation-based miRNA detection assay schematic. Scale bars are 100 μm. | |
In order to determine if the ∼3.2 nL reactors were properly isolated from each other during the hybridization step, we performed a fluorometric assay in the magnetically sealed well array sandwiches (Fig. 2A–C). 200 μm circular posts containing biotinylated DNA probes were photopolymerized in select 300 μm wells (Fig. 2B). Streptavidin-β-galactosidase conjugates (SAB, Invitrogen) were then bound to the DNA probes (see ESI†). The 300 μm well arrays were then sealed against a 30 μm well array containing fluorescein di-β-D-galactopyranoside (FDG, Thermo Fisher) substrate (Fig. 2A). The sandwich was then incubated for 1 hour at room temperature before imaging. High viscosity ethyl cyanoacrylate adhesive (World Precision Instruments) was applied around the edges of the glass slides of array sandwich in order keep the device sealed after the removal of the magnets for imaging. The fluorescence intensity of each well was measured using the average intensity in 100 pixel (246 μm) diameter circular windows from images collected using a 5× objective. The wells with enzyme functionalized posts had mean intensities of 5090 ± 680 AFU (n = 5 wells) while the wells without enzyme functionalized posts had mean intensities of 2490 ± 310 AFU (n = 10 wells). ± values indicate standard deviation (SD) throughout the manuscript. The brighter fluorescence signal was observed only in wells that contained the enzyme-functionalized posts, indicating that the wells were isolated reactors during the timescale of the experiment.
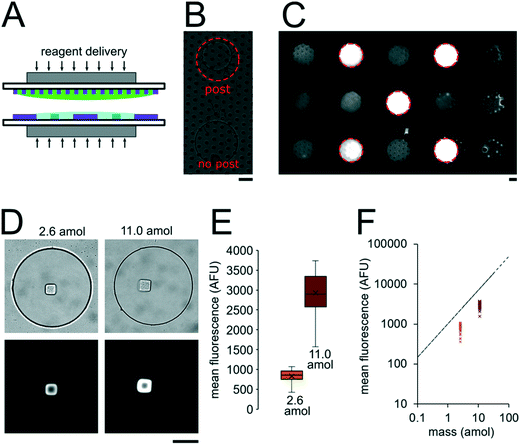 |
| Fig. 2 Nanoliter well array sealing and reagent delivery. (A) Schematic for reagent delivery during sandwich assembly. (B) Brightfield image of well array sandwich. (C) Fluorescence micrograph of enzymatic reaction in well array sandwich 60 min after sealing. Dashed red circles indicate wells with enzyme-functionalized posts. (D–F) SA-PE binding assay in sealed wells. 2.6 amol of SA-PE delivered by including 1 μg mL−1 SA-PE in the 30 μm well array before sealing (left) and 11.0 amol delivered by including 1 μg mL−1 SA-PE in both the top layer and bottom layer before sealing. (D) Brightfield and fluorescence micrographs following binding assay. (E) Box plots showing the distribution of mean fluorescence of posts in each condition. The error bars indicate minimum and maximum of the distribution, the ends of the box are the first and third quartiles, the vertical line in the box is the median, and the x is the mean (n = 40 wells for 2.6 amol, n = 39 wells for 11.0 amol). (F) Plot showing mean post fluorescence vs. loaded mass for each well. The dashed line represents the theoretical maximum mean fluorescence in a 40 μm post estimated for the mass loaded. Scale bars are 100 μm. | |
To assess the reproducibility of reagent delivery during the nanoliter reactor assembly process, we performed a SA-PE binding assay on 40 μm PEGDA posts functionalized with biotinylated probes housed inside 300 μm wells sealed against a 30 μm well array (Fig. 2D). These experiments were also used to determine if the amount of captured SA-PE after the assay was consistent with the amount we theoretically delivered to each well. 2.6 amol of SA-PE was delivered by including 1 μg mL−1 SA-PE in the 30 μm well array before sealing and 11.0 amol of SA-PE was delivered by including 1 μg mL−1 SA-PE in both the 30 μm and 300 μm well arrays before sealing. For simplicity, the volume occupied by the gel posts was neglected from the volume calculations. After completing the binding assay, the mean fluorescence intensity of posts was measured in wells where SA-PE was included in just the top (30 μm well) array (Itop, n = 40 wells from 2 separate devices), and in wells where SA-PE was included in both arrays before assembly (Itop+bottom, n = 39 wells from 2 separate devices). As expected, values were not normally distributed because the maximum possible SA-PE delivered has an upper bound capped by reactor volume and were thus displayed in box plots (Fig. 2E). Based on the estimated differences in loaded mass, we expected a ratio of Itop+bottom/Itop ∼ 4.3 and observed a ratio of ∼3.5. In order to compare the resulting fluorescence values to the fluorescence expected from the loaded SA-PE mass, we developed a calibration curve of SA-PE fluorescence (see ESI,† Fig. S5). The trend in fluorescence values observed was consistent with the expected values and both mean values fall under the theoretical maximum mean fluorescence possible based on the amount of SA-PE loaded, consistent with well isolation (Fig. 2F). A lower coefficient of variability (CV ∼ 16%) was observed when SA-PE is loaded in both layers before isolation, compared to a CV ∼ 21% when SA-PE was included only in the top layer. This decrease in CV is consistent with variability in delivered mass attributable to geometric factors, as when SA-PE in included in both layers, a smaller fraction of the volume containing SA-PE changes due to variations in well overlap numbers (see ESI†). However, not all of the variability observed in either case can be attributed to geometric factors alone. Further research is ongoing to minimize loading variability and explore the effects of fluid flow, air bubble trapping, reagent binding kinetics, and other factors. Given that we can quantitatively meter analytes and isolate wells during the device sandwich assembly, we next performed quantitative miRNA assays in our platforming and developed a framework for understanding assay performance.
miRNA binding assay performance
A theoretical framework for describing the performance of the hydrogel-based particle miRNA assay was previously developed43 and is governed by the following equation: | 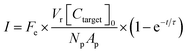 | (1) |
where I is the net mean fluorescence measured in the hydrogels corresponding to captured miRNA signal at the end of the assay, Fe is a fluorescence efficiency constant depending on the fluorophore and imaging parameters, Vr is the reaction volume the assay takes place in, [Ctarget]0 is the initial concentration of the target analyte in the reaction volume, Np is the number of particles in the reaction volume, Ap is the 2D area of each particle measured during imaging, t is the assay hybridization time, and τ is a time constant describing the timescale of capturing all of the targets in the reaction volume (see ESI†). Thus, when t ≫ τ, we can assume that approximately all of the target mass (masstarget = Vr ×[Ctarget]0) is captured and the maximum fluorescence for that device geometry and setup is achieved: | 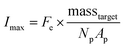 | (2) |
Due to the high porosity of the PEGDA structure and large amount of DNA probes incorporated in the hydrogels, analyte binding is fast compared to analyte diffusion within the hydrogel structure (Damköhler number (Da) ≫1), thus τ is dominated by mass transport.43 This effect manifests itself experimentally as the appearance of a boundary layer of brighter fluorescence at the edges of the hydrogel sensing surface, as targets are captured in that boundary layer before they are able to diffusively penetrated further into the hydrogel structure.43,45,48 In the particle-based assay Vr ∼ 50 μL and hybridization times t ∼ 90 min, thus τ is minimized by using vigorous convective mixing to ensure efficient transport of the targets to the hydrogel particle sensing surfaces and also by loading Np ∼ 50 particles per reaction to increase total sensing surface.45,48 However, eqn (2) indicates that increasing Np lowers Imax, meaning there is a tradeoff in assay sensitivity and assay time. In other words, increasing total sensing area (Atotal = Np × Ap) allows faster capture of the available targets, but lowers the fluorescence signal per area measured upon completion of the assay.
In the nanoliter well-based assay presented here, Vr is reduced from 50 μL to 3.2 nL by miniaturization of each reaction volume. Using just diffusive mass transport of miRNA targets to a single 40 μm hydrogel post in a 300 μm well we can estimate that τ < 6 min, and thus t ≫ τ for a hybridization time of t = 90 min (see ESI†). Thus, the reduction of Vr enables a reduction in Atotal without needing to increase assay hybridization time t. In addition to reducing Np from 50 to 1, the hydrogel area Ap can also be reduced without any challenges in handling because the posts are covalently attached within the wells. Therefore, by reducing Atotal by a factor of ∼100, this theoretical framework predicts that the well-based assay should be ∼100× more sensitive (detection of 100× less masstarget in a given reactor) compared to the particle-based assay without using any signal amplification. For the well-based assay, I ≈ Imax and therefore assay performance is described by:
| 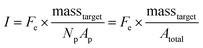 | (3) |
In order to validate our theoretical framework developed above, we performed miR-21 assays in isolated wells with different number of posts and posts of different sizes with DNA probes complementary to miR-21. miR-21 has been shown to be upregulated in non-small cell lung cancers and has potential as a biomarker for patient outcome.10,28,29,31,87 During sandwich assembly, 0.5 amol of miR-21 was delivered to each nanoliter reactor. Wells contained a single square post of varying area (Fig. 3A) or varying numbers of posts (Fig. 3B). This approach allowed us to independently vary post area (Ap) and the number of posts (Np). The net mean signal of each post decreased with increasing post area, as well as with increasing number of posts per well, as expected from eqn (3) (Fig. 3C). In order to determine that the observed differences in mean fluorescence signal were not the result of changes in probe incorporation in posts of different area, we made a well array with posts of different area that contained biotinylated probes in different wells and performed a binding assay by incubating with SA-PE (Fig. S6†). Because in this configuration the wells were not isolated and an excess of SA-PE molecules was present in solution, approximately all DNA probes in the hydrogels were labeled with SA-PE. We did not observe a statistically significant difference in mean fluorescence signal for posts of different area when the binding assay was done without well isolation, indicating that our fabrication did not result in posts of different area having different probe incorporation efficiencies. Therefore, our results indicate that when assays in the well arrays were done with isolated wells, the differences in mean fluorescence signal measured was the result of the same loaded target mass binding to different hydrogel sensing areas.
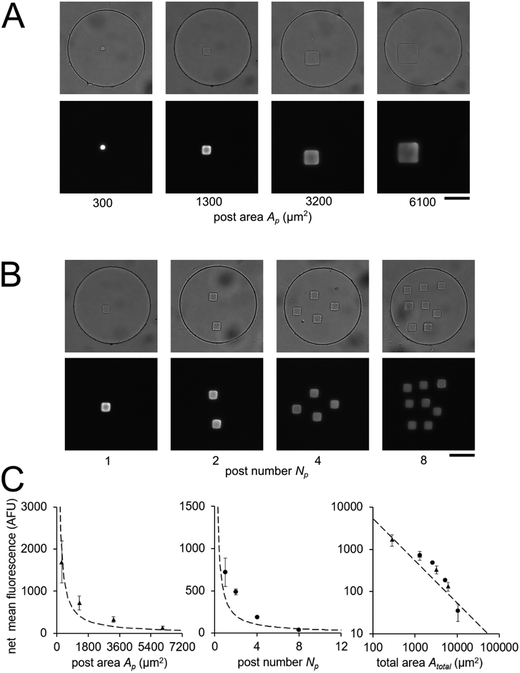 |
| Fig. 3 Quantitative miRNA assays in isolated nanoliter wells. Brightfield and fluorescence micrographs following miR-21 hybridization assay in sealed nanoliter wells (A) with posts of different area Ap and (B) with varying number of posts Np with Ap ∼ 1300 μm2. 0.5 amol of miR-21 was loaded into each well. (C) Plots of net mean intensity of posts in each condition versus post area Ap, post number Np, and (in log–log axes) total area Atotal = Ap × Np. Error bars represent ± SD (n = 3 wells for each condition). Dashed line represents theoretically estimated fluorescence intensity Imax. Scale bars are 100 μm. | |
From eqn (3), our model predicts that net mean fluorescence signal I should scale with Atotal−1. By plotting the I measured from the isolated well array miR-21 assays with Atotal as the independent variable, we observe that our results are qualitatively consistent with the predicted relationship (Fig. 3C). Additionally, using an experimentally estimated Fe for our system (see ESI,† Fig. S5), our results have quantitative agreement with the theoretically expected I values. Our model predicts that as long as I ≈ Imax, minimizing post area (Ap) results in the best assay performance. As expected from theory, our experimental results show that the posts with the smallest area (Ap ∼ 300 μm2) have the highest net mean fluorescence I upon completion of the miR-21 assay (Fig. 3A and C). Because these posts have an aspect ratio greater than 1 (post width <20 μm), we did occasionally observe toppled posts (results not shown). Thus, posts with widths ∼40 μm were chosen as the optimal size due to their balance of reliability and performance in the 35 μm deep wells. Experimental deviations from our simplified theoretical model may be the result of breakdowns of one or more of our assumptions, which include complete binding of loaded miRNA independent of post surface area, complete ligation, labeling, and retention of captured miRNA targets, perfect well isolation, and miRNA target delivery not affected by posts.
Having developed an understanding of our assay performance, we performed experiments to estimate the lower limit of detection (LLOD). By delivering 0.5 amol of miR-21 to each isolated nanoliter reactor (Fig. 3), we demonstrated miRNA assays able to detect lower miRNA masses per reactor compared to previously demonstrated particle-based assays without signal amplification which have an estimated LLOD of ∼2–5 amol.45,48 By varying the amount of miRNA mass delivered to different nanoliter well reactors (each with a single square post), we constructed a calibration curve determine the quantitative dynamic range and LLOD of our assay. We delivered 0, 0.025, 0.05, 0.1, 0.5, 1, 5, and 10 amol of miR-21 to each well in different devices and measured net mean fluorescence of posts for each condition. (Fig. 4A). As expected from eqn (3), as masstarget per well decreases, the measured net mean fluorescence decreases linearly (Fig. 4B), which enables the assay to perform quantification of unknown analyte quantities. The net mean fluorescence showed this linear relationship with loaded mass for over 3 orders of magnitude with R2 = 0.99. Using these results, we extrapolated the concentration at which signal over noise (SNR) is equal to 3,45,48 and estimate an LLOD of 0.004 amol for our assay. As expected from our theoretical model, our LLOD is >100× lower than previously reported for hydrogel particle-based assays done without signal amplification.45,48 While the PEGDA hydrogel-based miRNA hybridization assay's sensitivity can be further increased using signal amplification (not target amplification) after miRNA hybridization,44,47,66 in this work we aimed to keep the assay protocol simple. Our current results demonstrate the ability to perform sensitive and quantitative miRNA assays across a large dynamic range. Because multiplexing capabilities are needed for translational miRNA assays,17 a wide dynamic range is critical given that expression levels vary across orders of magnitude for different miRNA targets. Enhanced sensitivity enables analysis of miRNA targets with low expression levels and from precious, material-limited specimens. Further sensitivity enhancements via geometry optimization and signal amplification will be explored in future work.
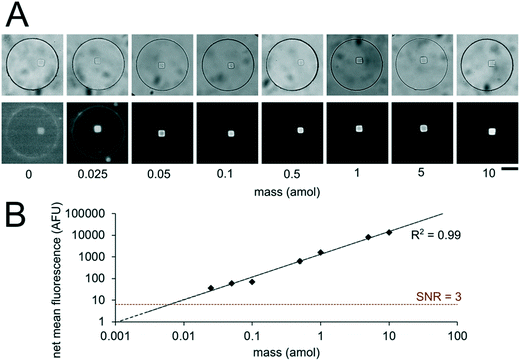 |
| Fig. 4 miRNA assay quantitation and sensitivity. (A) Brightfield and fluorescence micrographs (at different contrasts to aide viewing across multiple orders of magnitude) following miR-21 hybridization assay in sealed nanoliter wells. 0, 0.025, 0.05, 0.1, 0.5, 1, 5, 10 amol per well of miR-21 was loaded into different devices. Wells had a single square post with probes complementary to miR-21. (B) Log–log plot of net mean fluorescence versus mass per well. Error bars represent ± SD (n ≥ 6 wells for each condition). Dashed lines indicate linear fit and LLOD at SNR = 3. Scale bars are 100 μm. | |
Multiplex miRNA assays
In order to realize multiplex miRNA hybridization assays, our approach necessitated the formation of different PEGDA posts each functionalized with DNA probes complimentary to different miRNA targets within a single well. We previously demonstrated the fabrication of multiple posts within a single well (Fig. 3B). However, in these results all posts contained DNA probes complimentary to the same target and thus we could photopolymerize multiple posts in different regions within the well without needing to exchange the PEGDA prepolymer solution. To form posts functionalized with different DNA probes within a single well, we performed alternating prepolymer solution loading and exposure steps (Fig. 5A). In order to evaluate the fabrication reproducibility of this approach and assess proper prepolymer solution loading and exchange, we photopolymerized circular PEGDA posts with or without biotinylated DNA probes in alternating steps. The loading and exposure steps were done 6 times resulting in each well containing 6 posts (3 for each condition). Circular posts were used in order to facilitate alignment of multiple posts relative to each other. After fabrication, we performed a SA-PE binding assay by incubating the device in a solution containing SA-PE (Fig. 5B). The mean fluorescence signal of each post following the binding assay completion was measured using a circular windows of 40 pixels (24 μm) placed over each post (Fig. 5C), resulting in an average CV of the net mean fluorescence between different wells of 0.3 ± 0.1% (3 posts of each type per well, n = 6 wells). Within each well, the fluorescence signal from blank posts had an average CV of 1.9 ± 0.8% (3 posts per well, n = 6 wells), and the fluorescence signal from biotinylated posts had an average CV 0.3 ± 0.1% (3 posts per well, n = 6 wells). These results show that fabrication of posts at different positions within wells and across different wells is reproducible and that our protocol achieves proper prepolymer solution exchange between each post fabrication step.
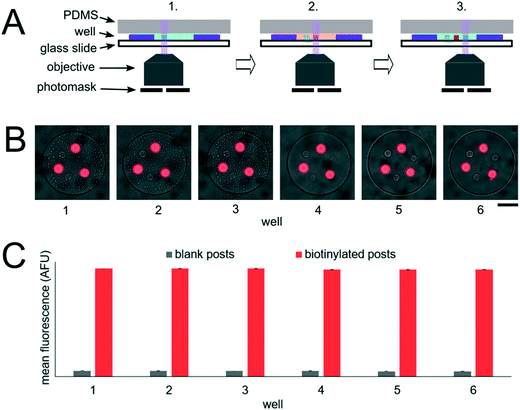 |
| Fig. 5 Post fabrication reproducibility. (A) Post fabrication schematic. Steps 1–3 show photopolymerization of 3 posts within a well with alternating functionalization. Step 1: Load prepolymer solution for blank posts, photopolymerize first post. Step 2: Exchange to prepolymer solution for biotinylated posts, photopolymerize second post. Step 3: Exchange to prepolymer solution for blank posts, photopolymerize third post. (B) Composite brightfield and fluorescence micrographs following SA-PE binding assay. Each post was photopolymerized in different steps alternating between prepolymer solutions with and without (blank) biotinylated DNA probes. (C) Plot showing mean fluorescence signal for each type of post in 6 different wells. Error bars represent ± SD (n = 3 posts for each condition per well). Scale bar is 100 μm. | |
Having demonstrated our ability to reproducibly fabricate differently functionalized posts within separate wells, we next performed multiplex miRNA assays. We used the same fabrication protocol to make devices with circular PEGDA posts with probes complimentary to 6 different miRNA targets (cel-miR-238, cel-miR-54, miR-21, let-7a, miR-210, miR-155) within a given well (Fig. 6A). Panels of 3 to 7 miRNAs have been demonstrated for targeted profiling assays of lung cancer and other diseases.13,26–29 let-7a, miR-210, and miR-155 have been shown to be dysregulated in cancer.9,15,88cel-miR-238 and cel-miR-54 are expressed in C. elegans.89 We performed miRNA assays in isolated well devices, each with different amounts of the different miRNA targets. Cel-miR-238 was used as a negative control and kept at 0 amol per well for all assays. cel-miR-54 was used as a loading control and kept at 0.5 amol per well for all assays. miR-21, let-7a, miR-210, and miR-155 amounts were varied between 0, 0.05, 0.5, and 5 amol in the different assays (Table S4†). Using the internal negative and positive controls as loading controls (see ESI†), we obtained linear calibration curves for all 4 miRNA targets that varied in mass per well, with R2 values of ∼1 for all for targets. (Fig. 6B). These results demonstrate the capacity of our platform for quantitative, multiplex assays. The multiplexing scheme used here requires spatial separation of the different posts and therefore, multiplexing is limited by the number of posts that can be made within a given well. Using 20 μm posts (Fig. 3A) with 20 μm spacing, ∼50 posts can be fabricated within a 300 μm single well. If even higher multiplexing were needed, biotinylated adapters with different chemistries could be used to attach different fluorophores to allow spectral multiplexing within posts functionalized with multiple probes to different targets.
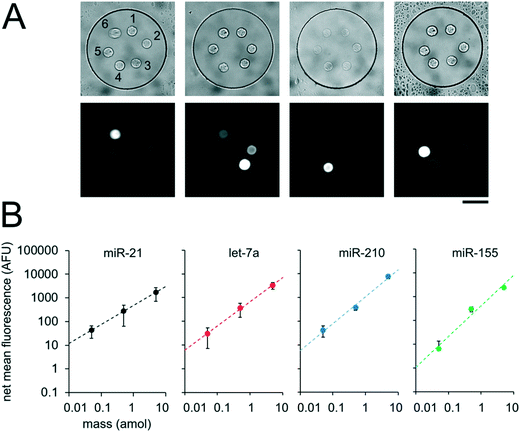 |
| Fig. 6 Multiplexed miRNA assays in sealed nanoliter wells. (A) Brightfield and fluorescence micrographs following multiplex miRNA hybridization assay in sealed wells. The circular posts contained DNA probes complementary to (1) cel-miR-238, (2) cel-miR-54, (3) miR-21, (4) let-7a, (5) miR-210, and (6) miR-155, as labeled. (B) Plots showing net mean fluorescence for different miRNAs as a function of loaded mass per well. cel-miR-238 was used as a negative control and cel-miR-54 was used as a positive loading control. Error bars represent ± SD (n ≥ 4 wells for each condition). Dashed lines indicate linear fit, R2 = 1.00 for all conditions. Scale bar is 100 μm. | |
miRNA assays from unprocessed cells
For assays with synthetic miRNA targets, miRNA was delivered to the nanoliter reactors during the well array sandwich assembly. For assays with cells, however, cells were first settled into the bottom layer that contained the PEGDA posts and lysis reagents were delivered to the reactors for miRNA extraction during the well array sandwich assembly. Calu-6 cells were settled into devices with 300 μm wells containing PEGDA posts with probes complementary to miR-21. Cells were suspended to densities of 0.25. 1, 2, and 8 million per mL which resulted in 14 ± 8 (n = 37), 53 ± 20 (n = 28), 110 ± 19 (n = 71), and 200 ± 26 (n = 116) cells per well, respectively, after 10 min of settling (n = number of wells) (Fig. 7B). Because cells were passively sedimented into the wells from a 5 μL droplet applied onto the well array surface, varying numbers of wells contained cells depending on the cell suspension density. A top layer with 30 μm wells containing lysis buffer was then applied onto the devices with settled cells and magnetically sealed for the cell lysis, miRNA extraction, and miRNA hybridization step (Fig. 7A, see ESI†). After 90 min at 55 °C, the devices were opened and the ligation and labeling steps were conducted as detailed previously for assays with synthetic targets. The mean intensity of the posts in each well was then determined and the net mean intensity was calculated by subtracting the mean intensity of negative controls calculated from devices in which no cells were settled. The negative control wells had mean signals of 0.5 ± 0.3 AFU (n = 18 wells). From a total of 252 wells with cells analyzed, 27 wells had net mean miR-21 signal <0. This corresponds to ∼57% of wells with ≤ 30 cells (24 out of 42 wells), ∼18% of wells with 30 < cells ≤ 60 (2 out of 11 wells), ∼5% of wells with 60 < cells ≤ 90 (1 out of 19 wells), and 0% of wells with >90 cells. For wells showing net positive signal, the net mean intensity of miR-21 correlated with the number of cells per well (R2 = 0.45) (Fig. 7C). Using the linear fit, we estimate a LLOD ∼16 cells per well (see ESI†). As detailed previously, miniaturization of the reaction volumes from 50 μL to 3.2 nL along with reducing the PEGDA sensing surface enhanced our previously demonstrated sensitivity, in this case from ∼1000 cells, down to ∼16 cells. With the demonstrated sensitivity, our platform enables high-throughput analysis of specimens with small cells numbers, such as 3D spheroids,56–58 circulating cell clusters59,60 organoids,61 early stage embryos,62,63 small whole organisms,64 and precious, material-limited biopsies. While a notable improvement, further improvements can be made by optimizing post geometry, as predicted by our theoretical model and experimental results (Fig. 3A and B). Additionally, instead of relying only on passive sedimentation, cells may be captured and even selected by implementing chemistries that bind to cell surface markers90 within wells.73,91 Using signal amplification schemes such as rolling-circle amplification47 instead of labeling capture miRNA with a single fluorophore done here, sensitivity can be further increased leading down to single-cell sensitivity, and ability to detect miRNAs expressed at lower levels.
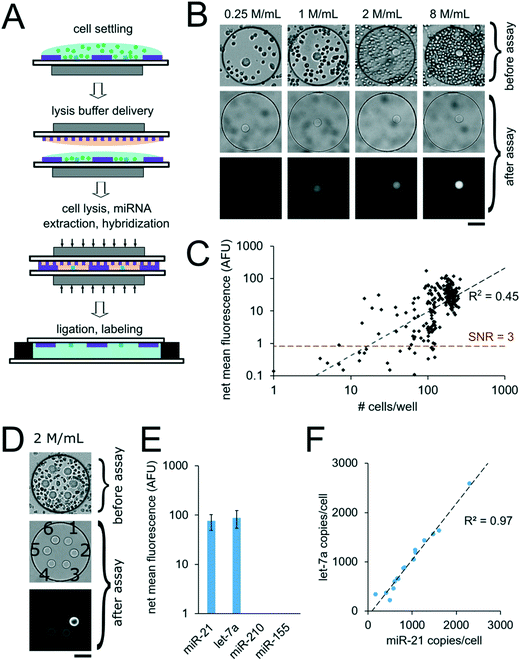 |
| Fig. 7 miRNA assays using unprocessed cells. (A) Cell assay schematic. (B and C) miRNA assays from Calu-6 cells in wells containing circular posts functionalized with probes complementary to miR-21. (B) Brightfield images after cell settling and after assay, and fluorescence micrographs after assay of representative wells from devices loaded with different densities of suspended cells: 0.25, 1, 2, and 8 million per mL (n = 37, 28, 71, and 116 wells, respectively). (C) miR-21 net mean fluorescence versus number of cells per well. Dashed lines indicate linear fit and LLOD at SNR = 3. (D–F) multiplex miRNA assays from Calu-6 cells in well array. (D) Brightfield images after cell settling, and following assay and fluorescence micrograph (at same contrast) of representative well following assay. The circular posts contained DNA probes complementary to (1) cel-miR-238, (2) cel-miR-54, (3) miR-21, (4) let-7a, (5) miR-210, and (6) miR-155, as labeled. 0.12 amol of synthetic cel-miR-54 was included in the lysis solution as a positive control and cel-miR-238 was used as a negative control. Cells were settled at a suspension density of 2 million per mL resulting in 98.2 ± 50.4 cells per well. (E) Net mean fluorescence for the miRNA targets. Error bars indicate ± SD (n = 16 wells). (F) miR-21 copy number per cell versus let-7a copy number per cell estimated for each well. Dashed line indicates linear fit. Scale bars are 100 μm. | |
In order to demonstrate multiplex assays from unprocessed cells, we settled Calu-6 cells into wells containing six posts functionalized with probes complimentary to different miRNA targets (cel-miR-238, cel-miR-54, miR-21, let-7a, miR-210, miR-155). Cells suspended at a density of 2 million cells per mL resulted in an average of 98 ± 50 cells per well (n = 16 wells) after settling for 10 min (Fig. 7E). 0.12 amol of synthetic cel-miR-54 target was included in the lysis solution to serve as a positive control. The post with probes complementary to cel-miR-238 was used as a negative control and the mean signal from this post was subtracted from the mean signal from other posts in a given well to calculate the net mean intensity for each miRNA. The posts with probes complementary to cel-miR-54 had an average net mean intensity of 180 ± 31 AFU (n = 16 wells), which was consistent with the expected signal for the amount of miRNA mass delivered, demonstrating that the presence of the cells and lysis reagents did not interfere with the assay (see ESI†). Net mean positive signal was measured for miR-21 and let-7a, with miR-21 having ∼15% lower signal compared to let-7a. Signals for miR-210 and miR-155 were below the LLOD (Fig. 7F). Normalizing the resulting fluorescence signal by the number of cell per well makes each well a biological replicate. In order to validate these results, we performed analogous experiments measuring the same miRNA targets from Calu-6 cells using the well-established particle-based assay48,92 (Fig. S8A†). Using the particle-based assay with ∼64
000 Calu-6 cells per tube, net mean positive signal was detected for miR-21 and let-7a, with miR-21 having ∼32% lower signal compared to let-7a. While miR-155 was detected using particles, its signal was ∼28× lower compared to let-7a signal, meaning it was below the LLOD of the well array assay when using ∼100 cells per well. miR-210 signal was not detected in the Calu-6 cells in either assay. Using calibration curves for miR-21 for the particle assay (Fig. S8B†) and the well array (Fig. 6B), we estimated the miR-21 copy number per cell in both assay formats. We obtained comparable values of ∼2000 miR-21 copies per cell using the particle assay and ∼1000 miR-21 copies per cell using the well array assay (see ESI†). In the well array assay, the signal for cel-miR-54 (which served as a positive control) did not show correlation with cell number per well (R2 = 0.00), as expected.
Interestingly, the estimated miR-21 and let-7a copy number per cell in different wells showed strong correlation with R2 = 0.97 (Fig. 7F). Because miR-21 and let-7a signal per well showed weak inverse correlation with positive control cel-miR-54 signal per well (R2 = 0.24 and R2 = 0.29, respectively), experimental assay variability does not explain the correlation in miR-21 and let-7a copy number per cell in different wells. These results suggest instead that in the Calu-6 cell line there is some heterogeneity in miR-21 and let-7a expression, but both miRNAs tend to be expressed at a constant ratio. We hypothesize that the correlation of miR-21 and let-7a signal as well as the heterogeneity of miRNA expression in the Calu-6 cells could be related to variabilities in cell health resulting from the freezing and reconstitution process. Further work will need to explore our platform's ability to accurately resolve population heterogeneity and the minimum number of well assays needed to converge to average population values.
Conclusions
We have presented the design and characterized the performance of a miRNA analysis platform that utilizes nanoliter well reactors containing functionalized PEGDA posts for miRNA capture. Nanoliter reactor isolation and reagent delivery (Fig. 2) were achieved by sandwiching well arrays against each other, enabling not only delivery of synthetic miRNA targets, but also miRNA extraction reagents for miRNA measurements directly from unprocessed cells. The well array form factor enabled >100 parallel assays within a single device, and also resulted in more sensitive assays. From our understanding of mass transport, we validated the quantitative performance of our assay and presented future avenues for optimization and sensitivity gains. Using synthetic miRNA targets we show sensitivity down to 0.025 amol, an 100× improvement without using signal amplification compared to previous similar techniques that utilized PEGDA particles.48 We also show compatibility of our platform with unprocessed cells, allowing measurements of miRNA from <20 cells without any prior nucleic acid extraction. Our robust hydrogel post fabrication approach demonstrated fabrication of multiple posts functionalized to capture different miRNA targets, enabling multiplex measurements. Future work will aim to increase the efficiency of device fabrication by utilizing contact lithography93 to simultaneously polymerize PEGDA posts within every well in a device instead of using projection lithography to individually polymerize posts. Cell retention and selection within wells can also be improved by capturing cells based on surface markers rather than utilizing passive sedimentation. The effects of cell size and cell health on miRNA signal readout also need to be further explored. Additionally, we hope to increase the utility of our platform by performing whole-cell imaging prior to lysis.81 With the demonstrated sensitivity, we envision application of the current platform to applications in which small cell numbers are assayed, such as 3D spheroids, cell clusters, organoids, and precious, material-limited biopsies. As an example, our platform would enable multiplex miRNA profiling from fine needle aspiration biopsies that result in limited cell numbers, and allow for multiple assays without depleting the entire sample. By using single amplification techniques such as rolling circle amplification which has been previously demonstrated in miRNA analysis using PEGDA particles,47 we hope to realize single-cell sensitivity and expand the analytical range of the platform.
Conflicts of interest
There are no conflicts to declare.
Acknowledgements
This work was supported by The Bridge Project, a partnership between the Koch Institute for Integrative Cancer Research at MIT and the Dana-Farber/Harvard Cancer Center, and partially by Cancer Center Support (core) Grant P30-CA14051 from the National Cancer Institute. The authors also gratefully acknowledge research funding support from a Ford Foundation Postdoctoral Fellowship (A. M. T.), a Ludwig Center Fund Postdoctoral Fellowship (A. M. T.), an NIGMS/NIH Interdepartmental Biotechnology Training Program Fellowship (M. B. N.), a Samsung Scholarship (J. J. K.), NIH-NRSA 5T32HL007893-20 (W. C. Z.), the NIH-YALE SPORE in Lung Cancer P50CA196530-03S1 (F. J. S.) and NIH-NIBIB Grant 5R21EB024101-02 (P. S. D.).
References
- R. C. Lee, R. L. Feinbaum and V. Ambros, Cell, 1993, 75, 843–854 CrossRef PubMed.
- N. C. Lau, L. P. Lim, E. G. Weinstein and D. P. Bartel, Science, 2001, 294, 858–862 CrossRef PubMed.
- A. K. Pandey, P. Agarwal, K. Kaur and M. Datta, Cell. Physiol. Biochem., 2009, 23, 221–232 CrossRef PubMed.
- E. M. Small and E. N. Olson, Nature, 2011, 469, 336–342 CrossRef PubMed.
- T. Thum and M. Mayr, Cardiovasc. Res., 2012, 93, 543–544 CrossRef PubMed.
- P. Shah, S. K. Cho, P. W. Thulstrup, M. J. Bjerrum, P. H. Lee, J.-H. Kang, Y.-J. Bhang and S. W. Yang, J. Mov. Disord., 2017, 10, 18–28 CrossRef PubMed.
- L. Qiu, E. K. Tan and L. Zeng, Adv. Exp. Med. Biol., 2015, 888, 85–105 CrossRef PubMed.
- P. T. Nelson, W. X. Wang and B. W. Rajeev, Brain Pathol., 2008, 18, 130–138 CrossRef PubMed.
- J. Takamizawa, H. Konishi, K. Yanagisawa, S. Tomida, H. Osada, H. Endoh, T. Harano, Y. Yatabe, M. Nagino, Y. Nimura, T. Mitsudomi and T. Takahashi, Cancer Res., 2004, 64, 3753–3756 CrossRef PubMed.
- H. Stenvold, T. Donnem, S. Andersen, S. Al-Saad, A. Valkov, M. I. Pedersen, L. T. Busund and R. M. Bremnes, BMC Clin. Pathol., 2014, 14, 9 CrossRef PubMed.
- A. L. Kasinski and F. J. Slack, Cancer Res., 2012, 72, 5576–5587 CrossRef PubMed.
- D. C. Corney, C. Il Hwang, A. Matoso, M. Vogt, A. Flesken-Nikitin, A. K. Godwin, A. A. Kamat, A. K. Sood, L. H. Ellenson, H. Hermeking and A. Y. Nikitin, Clin. Cancer Res., 2010, 16, 1119–1128 CrossRef PubMed.
- Z. H. Chen, G. L. Zhang, H. R. Li, J. D. Luo, Z. X. Li, G. M. Chen and J. Yang, Prostate, 2012, 72, 1443–1452 CrossRef PubMed.
- G. A. Calin and C. M. Croce, Nat. Rev. Cancer, 2006, 6, 857–866 CrossRef PubMed.
- G. A. Calin, C. Sevignani, C. D. Dumitru, T. Hyslop, E. Noch, S. Yendamuri, M. Shimizu, S. Rattan, F. Bullrich, M. Negrini and C. M. Croce, Proc. Natl. Acad. Sci. U. S. A., 2004, 101, 2999–3004 CrossRef PubMed.
- S. Bail, M. Swerdel, H. Liu, X. Jiao, L. A. Goff, R. P. Hart and M. Kiledjian, RNA, 2010, 16, 1032–1039 CrossRef PubMed.
- C. C. Pritchard, H. H. Cheng and M. Tewari, Nat. Rev. Genet., 2012, 13, 358–369 CrossRef PubMed.
- S. Grasedieck, N. Schöler, M. Bommer, J. H. Niess, H. Tumani, A. Rouhi, J. Bloehdorn, P. Liebisch, D. Mertens, H. Döhner, C. Buske, C. Langer and F. Kuchenbauer, Leukemia, 2012, 26, 2414–2416 CrossRef PubMed.
- Y. Li, Z. Li, S. Zhou, J. Wen, B. Geng, J. Yang and Q. Cui, BioMed Res. Int., 2013, 2013, 368975 Search PubMed.
- M. P. Gantier, C. E. McCoy, I. Rusinova, D. Saulep, D. Wang, D. Xu, A. T. Irving, M. A. Behlke, P. J. Hertzog, F. MacKay and B. R. G. Williams, Nucleic Acids Res., 2011, 39, 5692–5703 CrossRef PubMed.
- L. Warren, D. Bryder, I. L. Weissman and S. R. Quake, Proc. Natl. Acad. Sci. U. S. A., 2006, 103, 17807–17812 CrossRef PubMed.
- O. I. Petriv, F. Kuchenbauer, A. D. Delaney, V. Lecault, A. White, D. Kent, L. Marmolejo, M. Heuser, T. Berg, M. Copley, J. Ruschmann, S. Sekulovic, C. Benz, E. Kuroda, V. Ho, F. Antignano, T. Halim, V. Giambra, G. Krystal, C. J. F. Takei, A. P. Weng, J. Piret, C. Eaves, M. A. Marra, R. K. Humphries and C. L. Hansen, Proc. Natl. Acad. Sci. U. S. A., 2010, 107, 15443–15448 CrossRef PubMed.
- S. M. Prakadan, A. K. Shalek and D. A. Weitz, Nat. Rev. Genet., 2017, 18, 345–361 CrossRef PubMed.
- M. Baker, Nat. Methods, 2010, 7, 687–692 CrossRef PubMed.
- P. Chugh and D. P. Dittmer, Wiley Interdiscip. Rev.: RNA, 2012, 3, 601–616 CrossRef PubMed.
- E. Nadal, A. Truini, A. Nakata, J. Lin, R. M. Reddy, A. C. Chang, N. Ramnath, N. Gotoh, D. G. Beer and G. Chen, Sci. Rep., 2015, 5, 12464 CrossRef PubMed.
- J. Zhou, L. Yu, X. Gao, J. Hu, J. Wang, Z. Dai, J. F. Wang, Z. Zhang, S. Lu, X. Huang, Z. Wang, S. Qiu, X. Wang, G. Yang, H. Sun, Z. Tang, Y. Wu, H. Zhu and J. Fan, J. Clin. Oncol., 2011, 29, 4781–4788 CrossRef PubMed.
- L. Yu, N. W. Todd, L. Xing, Y. Xie, H. Zhang, Z. Liu, H. Fang, J. Zhang, R. L. Katz and F. Jiang, Int. J. Cancer, 2010, 127, 2870–2878 CrossRef PubMed.
- L. Xing, N. W. Todd, L. Yu, H. Fang and F. Jiang, Mod. Pathol., 2010, 23, 1157–1164 CrossRef PubMed.
- S. Lingam, M. Beta, D. Dendukuri and S. Krishnakumar, MicroRNA, 2014, 3, 18–28 CrossRef PubMed.
- J. A. Hanna, H. Wimberly, S. Kumar, F. Slack, S. Agarwal and D. L. Rimm, BioTechniques, 2012, 52, 235–245 CrossRef PubMed.
- H. Nakayama, Y. Yamauchi, M. Taoka and T. Isobe, Anal. Chem., 2015, 87, 2884–2891 CrossRef PubMed.
- C. Yang, B. Dou, K. Shi, Y. Chai, Y. Xiang and R. Yuan, Anal. Chem., 2014, 86, 11913–11918 CrossRef PubMed.
- P. Zhang, J. Zhang, C. Wang, C. Liu, H. Wang and Z. Li, Anal. Chem., 2014, 86, 1076–1082 CrossRef PubMed.
- O. S. Rissland, S. J. Hong and D. P. Bartel, Mol. Cell, 2011, 43, 993–1004 CrossRef PubMed.
- P. Mestdagh, N. Hartmann, L. Baeriswyl, D. Andreasen, N. Bernard, C. Chen, D. Cheo, P. D'Andrade, M. DeMayo, L. Dennis, S. Derveaux, Y. Feng, S. Fulmer-Smentek, B. Gerstmayer, J. Gouffon, C. Grimley, E. Lader, K. Y. Lee, S. Luo, P. Mouritzen, A. Narayanan, S. Patel, S. Peiffer, S. Rüberg, G. Schroth, D. Schuster, J. M. Shaffer, E. J. Shelton, S. Silveria, U. Ulmanella, V. Veeramachaneni, F. Staedtler, T. Peters, T. Guettouche, L. Wong and J. Vandesompele, Nat. Methods, 2014, 11, 809–815 CrossRef PubMed.
- X. Liu, R. Tian, J. Gao, D. Liu and Z. Wang, Analyst, 2017, 142, 4529–4535 RSC.
- N. Crosetto, M. Bienko and A. Van Oudenaarden, Nat. Rev. Genet., 2015, 16, 57–66 CrossRef PubMed.
- J. T. G. Pena, C. Sohn-Lee, S. H. Rouhanifard, J. Ludwig, M. Hafner, A. Mihailovic, C. Lim, D. Holoch, P. Berninger, M. Zavolan and T. Tuschl, Nat. Methods, 2009, 6, 139–141 CrossRef PubMed.
- A. N. Silahtaroglu, D. Nolting, L. Dyrskjøt, E. Berezikov, M. Møller, N. Tommerup and S. Kauppinen, Nat. Protoc., 2007, 2, 2520–2528 CrossRef PubMed.
- E. Andrés-León, R. Núñez-Torres and A. M. Rojas, Sci. Rep., 2016, 6, 25749 CrossRef PubMed.
- S. R. Ryoo, J. Lee, J. Yeo, H. K. Na, Y. K. Kim, H. Jang, J. H. Lee, S. W. Han, Y. Lee, V. N. Kim and D. H. Min, ACS Nano, 2013, 7, 5882–5891 CrossRef PubMed.
- D. C. Pregibon and P. S. Doyle, Anal. Chem., 2009, 81, 4873–4881 CrossRef PubMed.
- J. J. Kim, L. Chen and P. S. Doyle, Lab Chip, 2017, 17, 3120–3128 RSC.
- S. C. Chapin, D. C. Appleyard, D. C. Pregibon and P. S. Doyle, Angew. Chem., Int. Ed., 2011, 50, 2289–2293 CrossRef PubMed.
- S. C. Chapin, D. C. Pregibon and P. S. Doyle, Lab Chip, 2009, 9, 3100 RSC.
- S. C. Chapin and P. S. Doyle, Anal. Chem., 2011, 83, 7179–7185 CrossRef PubMed.
- H. Lee, S. J. Shapiro, S. C. Chapin and P. S. Doyle, Anal. Chem., 2016, 88, 3075–3081 CrossRef PubMed.
- D. Dendukuri, S. S. Gu, D. C. Pregibon, T. A. Hatton and P. S. Doyle, Lab Chip, 2007, 7, 818 RSC.
- D. C. Pregibon, M. Toner and P. S. Doyle, Science, 2007, 315, 1393–1396 CrossRef PubMed.
- D. C. Appleyard, S. C. Chapin and P. S. Doyle, Anal. Chem., 2011, 83, 193–199 CrossRef PubMed.
- D. C. Appleyard, S. C. Chapin, R. L. Srinivas and P. S. Doyle, Nat. Protoc., 2011, 6, 1761–1774 CrossRef PubMed.
- R. Levicky and A. Horgan, Trends Biotechnol., 2005, 23, 143–149 CrossRef PubMed.
- N. V. Sorokin, V. R. Chechetkin, S. V. Pan'kov, O. G. Somova, M. A. Livshits, M. Y. Donnikov, A. Y. Turygin, V. E. Barsky and A. S. Zasedatelev, J. Biomol. Struct. Dyn., 2006, 24, 57–66 CrossRef PubMed.
- A. J. Hughes, R. K. C. Lin, D. M. Peehl and A. E. Herr, Proc. Natl. Acad. Sci. U. S. A., 2012, 109, 5972–5977 CrossRef PubMed.
- P. A. Kenny, G. Y. Lee, C. A. Myers, R. M. Neve, J. R. Semeiks, P. T. Spellman, K. Lorenz, E. H. Lee, M. H. Barcellos-Hoff, O. W. Petersen, J. W. Gray and M. J. Bissell, Mol. Oncol., 2007, 1, 84–96 CrossRef PubMed.
- K. Moshksayan, N. Kashaninejad, M. E. Warkiani, J. G. Lock, H. Moghadas, B. Firoozabadi, M. S. Saidi and N. T. Nguyen, Sens. Actuators, B, 2018, 263, 151–176 CrossRef.
- J. Ruppen, F. D. Wildhaber, C. Strub, S. R. R. Hall, R. A. Schmid, T. Geiser and O. T. Guenat, Lab Chip, 2015, 15, 3076–3085 RSC.
- S. H. Au, J. Edd, A. E. Stoddard, K. H. K. Wong, F. Fachin, S. Maheswaran, D. A. Haber, S. L. Stott, R. Kapur and M. Toner, Sci. Rep., 2017, 7, 2433 CrossRef PubMed.
- Y. Hong, F. Fang and Q. Zhang, Int. J. Oncol., 2016, 49, 2206–2216 CrossRef PubMed.
- Y. Y. Chen, P. N. Silva, A. M. Syed, S. Sindhwani, J. V. Rocheleau and W. C. W. Chan, Proc. Natl. Acad. Sci. U. S. A., 2016, 113, 14915–14920 CrossRef PubMed.
- H. Y. Huang, H. H. Shen, C. H. Tien, C. J. Li, S. K. Fan, C. H. Liu, W. S. Hsu and D. J. Yao, PLoS One, 2015, 10, e0124196 CrossRef PubMed.
- S. Suri, A. Singh, A. H. Nguyen, A. M. Bratt-Leal, T. C. McDevitt and H. Lu, Lab Chip, 2013, 13, 4617 RSC.
- J. E. Sulston and H. R. Horvitz, Dev. Biol., 1977, 56, 110–156 CrossRef PubMed.
- P. H. Lizotte, R. E. Jones, L. Keogh, E. Ivanova, H. Liu, M. M. Awad, P. S. Hammerman, R. R. Gill, W. G. Richards, D. A. Barbie, A. J. Bass, R. Bueno, J. M. English, M. Bittinger and K.-K. Wong, Sci. Rep., 2016, 6, 31745 CrossRef PubMed.
- H. Lee, R. L. Srinivas, A. Gupta and P. S. Doyle, Angew. Chem., Int. Ed., 2015, 54, 2477–2481 CrossRef PubMed.
- E. Ostuni, C. S. Chen, D. E. Ingber and G. M. Whitesides, Langmuir, 2001, 17, 2828–2834 CrossRef.
- S. Yamamura, H. Kishi, Y. Tokimitsu, S. Kondo, R. Honda, S. Ramachandra Rao, M. Omori, E. Tamiya and A. Muraguchi, Anal. Chem., 2005, 77, 8050–8056 CrossRef PubMed.
- J. C. Love, J. L. Ronan, G. M. Grotenbreg, A. G. Van Der Veen and H. L. Ploegh, Nat. Biotechnol., 2006, 24, 703–707 CrossRef PubMed.
- A. M. Tentori, K. A. Yamauchi and A. E. Herr, Angew. Chem., Int. Ed., 2016, 55, 12431–12435 CrossRef PubMed.
- A. J. Hughes, D. P. Spelke, Z. Xu, C.-C. Kang, D. V. Schaffer and A. E. Herr, Nat. Methods, 2014, 11, 749–755 CrossRef PubMed.
- K. A. Yamauchi and A. E. Herr, Microsyst. Nanoeng., 2017, 3, 16079 CrossRef PubMed.
- A. J. Torres, A. S. Hill and J. C. Love, Anal. Chem., 2014, 86, 11562–11569 CrossRef PubMed.
- T. M. Gierahn, M. H. Wadsworth, T. K. Hughes, B. D. Bryson, A. Butler, R. Satija, S. Fortune, J. Christopher Love and A. K. Shalek, Nat. Methods, 2017, 14, 395–398 CrossRef PubMed.
- E. Brouzes, M. Medkova, N. Savenelli, D. Marran, M. Twardowski, J. B. Hutchison, J. M. Rothberg, D. R. Link, N. Perrimon and M. L. Samuels, Proc. Natl. Acad. Sci. U. S. A., 2009, 106, 14195–14200 CrossRef PubMed.
- G. Amselem, C. Guermonprez, B. Drogue, S. Michelin and C. N. Baroud, Lab Chip, 2016, 16, 4200–4211 RSC.
- S. S. Bithi and S. A. Vanapalli, Sci. Rep., 2017, 7, 41707 CrossRef PubMed.
- K. W. Oh and C. H. Ahn, J. Micromech. Microeng., 2006, 16, R13–R39 CrossRef.
- N. Beyor, L. Yi, T. S. Seo and R. A. Mathies, Anal. Chem., 2009, 81, 3523–3528 CrossRef PubMed.
- J. S. Marcus, W. F. Anderson and S. R. Quake, Anal. Chem., 2006, 78, 956–958 CrossRef PubMed.
- C. C. Kang, J. M. G. Lin, Z. Xu, S. Kumar and A. E. Herr, Anal. Chem., 2014, 86, 10429–10436 CrossRef PubMed.
- H. Li, G. Brewer, G. Ongo, F. Normandeau, A. Omeroglu and D. Juncker, Anal. Chem., 2017, 89, 8620–8625 CrossRef PubMed.
- A. Khademhosseini, J. Yeh, G. Eng, J. Karp, H. Kaji, J. Borenstein, O. C. Farokhzad and R. Langer, Lab Chip, 2005, 5, 1380 RSC.
- S. Eun Chung, J. Kim, D. Yoon Oh, Y. Song, S. Hoon Lee, S. Min and S. Kwon, Nat. Commun., 2014, 5, 3468 CrossRef PubMed.
- J. J. Kim, K. W. Bong, E. Reátegui, D. Irimia and P. S. Doyle, Nat. Mater., 2017, 16, 139–146 CrossRef PubMed.
- D. Y. Liang, A. M. Tentori, I. K. Dimov and L. P. Lee, Biomicrofluidics, 2011, 5, 24108 CrossRef PubMed.
- B. Li, S. Ren, X. Li, Y. Wang, D. Garfield, S. Zhou, X. Chen, C. Su, M. Chen, P. Kuang, G. Gao, Y. He, L. Fan, K. Fei, C. Zhou and G. Schmit-Bindert, Lung Cancer, 2014, 83, 146–153 CrossRef PubMed.
- M. P. Puisségur, N. M. Mazure, T. Bertero, L. Pradelli, S. Grosso, K. Robbe-Sermesant, T. Maurin, K. Lebrigand, B. Cardinaud, V. Hofman, S. Fourre, V. Magnone, J. E. Ricci, J. Pouysségur, P. Gounon, P. Hofman, P. Barbry and B. Mari, Cell Death Differ., 2011, 18, 465–478 CrossRef PubMed.
- L. P. Lim, N. C. Lau, E. G. Weinstein, A. Abdelhakim and S. Yekta, Genes Dev., 2003, 17, 991–1008 CrossRef PubMed.
- L. Chen, H. Z. An, R. Haghgooie, A. T. Shank, J. M. Martel, M. Toner and P. S. Doyle, Small, 2016, 12, 2001–2008 CrossRef PubMed.
- M. C. Jones, J. J. Kobie and L. A. Delouise, Biomed. Microdevices, 2013, 15, 453–463 CrossRef PubMed.
- N. W. Choi, J. Kim, S. C. Chapin, T. Duong, E. Donohue, P. Pandey, W. Broom, W. A. Hill and P. S. Doyle, Anal. Chem., 2012, 84, 9370–9378 CrossRef PubMed.
- G. C. Le Goff, J. Lee, A. Gupta, W. A. Hill and P. S. Doyle, Adv. Sci., 2015, 2, 1500149 CrossRef PubMed.
Footnote |
† Electronic supplementary information (ESI) available. See DOI: 10.1039/c8lc00498f |
|
This journal is © The Royal Society of Chemistry 2018 |
Click here to see how this site uses Cookies. View our privacy policy here.