Environmental fate and exposure models: advances and challenges in 21st century chemical risk assessment
Received
27th November 2017
, Accepted 20th December 2017
First published on 20th December 2017
Abstract
Environmental fate and exposure models are a powerful means to integrate information on chemicals, their partitioning and degradation behaviour, the environmental scenario and the emissions in order to compile a picture of chemical distribution and fluxes in the multimedia environment. A 1995 pioneering book, resulting from a series of workshops among model developers and users, reported the main advantages and identified needs for research in the field of multimedia fate models. Considerable efforts were devoted to their improvement in the past 25 years and many aspects were refined; notably the inclusion of nanomaterials among the modelled substances, the development of models at different spatial and temporal scales, the estimation of chemical properties and emission data, the incorporation of additional environmental media and processes, the integration of sensitivity and uncertainty analysis in the simulations. However, some challenging issues remain and require research efforts and attention: the need of methods to estimate partition coefficients for polar and ionizable chemical in the environment, a better description of bioavailability in different environments as well as the requirement of injecting more ecological realism in exposure predictions to account for the diversity of ecosystem structures and functions in risk assessment. Finally, to transfer new scientific developments into the realm of regulatory risk assessment, we propose the formation of expert groups that compare, discuss and recommend model modifications and updates and help develop practical tools for risk assessment.
Environmental significance
Multimedia environmental fate models are regularly employed in the risk assessment of chemicals. They historically evolved from simple approaches to more refined and integrated modeling tools, often provided as part of a tiered strategy. Much work has been devoted to improving various aspects at the process and environmental description levels, but some issues need further research and model development, such as the capability to properly simulate the behaviour of polar and ionizable chemicals in the environment, the biovailability of chemicals in aquatic and terrestrial environments, and variability in time and space to account for more ecological relevance. At the same time, it is important to avoid “paralysis by analysis” and develop practical tools for regulatory risk assessment that are fit for purpose. We propose the formation of expert groups to address this task.
|
1. Introduction: evolution and current status of fate and exposure modeling
Assessing the environmental fate and exposure of chemicals used in commerce is a challenging but critical part of assessing the risks associated with their production, use and release. Environmental fate and exposure models play an important role in the chemical assessment process, and when coupled with monitoring data, can provide reliable information about the exposure of humans and the environment to chemicals.1
Over the last three decades, the development and application of environmental fate models has been facilitated by a repeating pattern of co-operation between model developers and users in ad hoc working groups. In 1994, workshops were organized by the Society of Environmental Toxicology and Chemistry (SETAC) at Leuven, Belgium (April, 1994) and later at Denver, Colorado (November, 1994). These two workshops brought together 30 model users and developers to discuss the application of environmental fate and exposure models; to evaluate their performance; to provide guidance on their use; and to make recommendations on how the models could be improved to broaden their acceptability and encourage their use.2 Seven years later, an OECD/UNEP Workshop was organized in Ottawa, Canada (October, 2001), to assess the utility of multimedia fate models for estimating overall environmental persistence and long-range transport of chemicals in the context of assessing potential persistent organic pollutants (POPs) and/or persistent, biaccumulative and toxic substances (PBTs). The Ottawa workshop marked the beginning of a period of activity by a working group of model developers and users (the OECD Expert Group on Multimedia Modeling) that led to the publication of a model comparison study,3 recommendations for assessing persistence and long-range transport with models4 and a consensus model, the OECD Overall Persistence and Long-range Transport Modeling Tool (The OECD Tool5). In 2013 a working group jointly organized by the three Scientific committees of the European Commission, DG SANCO (SCHER, SCHENIR, SCCS),6 published the scientific opinion “Addressing the New Challenges for Risk Assessment” in which a number of opportunities to improve exposure models to increase their ecological realism and predictive ability for the growing number of chemical classes on the market were identified. Some of the fate and exposure assessment challenges from the scientific opinion were reported by Di Guardo and Hermens.7
Most recently, the European Centre for Ecotoxicology and Toxicology of Chemicals (ECETOC) organized a workshop on environmental fate and exposure models in Brussels, Belgium (May, 2017). Following the format of previous workshops, the ECETOC workshop brought together users and developers of environmental fate models. At this workshop, industry scientists and regulators who conduct chemical hazard assessments using PBT criteria, and risk assessments for industrial chemicals, pharmaceuticals, biocides and agricultural chemicals, came together to review current standards of practice in exposure modeling and to identify key challenges for addressing 21st century needs in chemical hazard and risk assessment. The authors of this review article have been active in many of the workshops summarized above, and all participated at the recent ECETOC workshop. This review article summarizes key observations from the workshop, with a particular emphasis on how advances in environmental fate models over the last 20 years have addressed the challenges identified in the 1994 workshops, and to identify the primary needs to further advance the models.
Environmental fate models provide a framework to assemble a quantitative and mechanistic description of the sources of chemicals to the environment, and the pathways they follow en-route to their ultimate degradation or irreversible sequestration. Fate models can be integrated with, or loosely-coupled to, multi-pathway exposure models that describe the transfer of chemicals from the environment into the food and water ingested by humans, and water and soil that directly contacts the human skin.8 Models are currently heavily relied upon to assess chemical hazard, exposure and risk, and to formulate chemical management strategies. But models did not always occupy such a strong position as scientific and policy support tools.
One of the main outputs of the modeling workshops that were held in the 1990s was a report by Cowan et al.2 that identified a number of research areas that required attention in order to strengthen the utility of models as scientific and decision-support tools. Cowan et al.2 recognized that the models were powerful conceptual tools capable of characterizing and quantifying the sources, environmental pathways, and exposure routes for chemicals used in commerce. And, they recognized the importance of identifying and quantifying the uncertainty and variability associated with a model's description of these processes. The following five key areas were identified as requiring attention by Cowan et al.2 in 1995:
(1) Clear classification of chemicals that are within the domain of applicability of models; chemical properties and degradation half-lives and their variability with temperature; emission data, including background environmental concentrations;
(2) Model algorithms and parameterization to increase the fidelity of the model's descriptions of the real environment within the bulk compartments of air, water, soil and sediment that were generally included in models at the time, and to extend the models to describe additional compartments such as vegetation and ice/snow; additionally, the need to develop data sets to describe inter-media transfer coefficients in a variety of environmental scenarios; among the other areas to improve were sub-models for chemical fate in soil and sediments as well as waste-water treatment models, and bioaccumulation and food chain models.
(3) Model comparison exercises to enhance reliability and credibility, especially in model documentation;
(4) Sensitivity and uncertainty analysis to provide confidence intervals to support hazard and risk-based decisions and to identify key areas for further research and model development;
(5) Establishment of a set of guidelines for good modeling practice in scientific and decision-support applications of models, with a particular attention to the model validation issues and the comparison of predicted to measured results.
Model developers have invested considerable resources to address these areas since the publication of Cowan et al.2 in 1995. Moreover, they have expanded the scope and applicability of fate and exposure models in several other dimensions that go beyond these recommendations. Areas where there have been notable developments include:
• Development of models with a range of spatial and temporal resolutions that can be used in concert with field data to rationalize chemical concentrations and estimate the rates of emissions or key fate processes at different spatio-temporal scales;
• Inclusion of transformation products in model scenarios;
• Scenario analysis to investigate the influence of climate variability and climate change on exposure to environmental contaminants;
• Development of fate and exposure models for engineered nanoparticles and for mercury;
• Adaptation of the principles from chemical fate and exposure models to pharmacokinetic models and application to human biomonitoring data.
Here we review the progress made in these areas, discuss the mechanistic foundations of fate and exposure models, and identify open questions and needs for strengthening future model development and application. We first provide a summary of progress in different areas and then highlight selected topics of particular interest.
2. Progress in fate and exposure model development since the mid-1990s
The areas of fate and exposure modeling where progress has been made range from chemical property data needed as model inputs to model parameterization and design, types of scenarios that can be handled by the models, model evaluation by comparison to field data, sensitivity and uncertainty analysis, and more.
Chemical properties, specific model parameters
Chemical property data are essential as model inputs. Since the publication of the property data handbooks by Mackay et al.,9 property data for many organic chemicals have been critically evaluated, missing data have been estimated with improved chemical property estimation methods, and sets of recommended best estimates have been published for several classes of environmentally important chemicals (e.g., PCBs,9,10 HCHs,11 PAHs,12 PFASs,13 siloxanes,14 chlorinated paraffins15 and chlorinated dioxins and furans,16 and complex mixtures of halogenated organics17). Polyparameter linear-free energy relationships (ppLFER)18 have been established to estimate partition coefficients for a range of environmental media for which partitioning cannot be related to the octanol–water partition coefficient (Kow) (e.g., ice/snow,19 mineral surfaces,20–22 aerosols23–25 and polymers and biopolymers26). A great deal of effort has also been invested in expanding the applicability domain of models to better assess the environmental fate and bioaccumulation of polar and ionizable organic chemicals.27–30 Lastly, model parameters describing environmental mass-transfer processes have been critically reviewed and updated.31
Emission data
Two complementary approaches have been employed to estimate the emission source strength of chemicals used in technical applications and consumer products. The first is the bottom-up approach that was pioneered by Breivik et al.32–34 to estimate the emissions of PCBs globally, and applied by Diamond and colleagues35–37 for the city of Toronto. Other bottom-up emission inventories have been compiled by Wang et al.38 for PFCAs, by Glüge et al.39 for chlorinated paraffins, Li et al.40 for α-HCH, and by Zhang and Tao for 16 PAHs.41 The second approach is the back-calculation of emissions from measured concentration data by inverse modeling,14,42–47 which is discussed in more detail below.
Expanding the scope and applicability of the models
A key contribution in this area is the definition of a variety of metrics of exposure-related environmental and human exposure hazard that can be calculated with fate and exposure models. These include metrics of persistence and long-range transport potential that reflect a chemical's tendency to be widely distributed, to form long-lived reservoirs in the environment, and to lead to the formation of persistent transformation products.5,48–58 Models have also been applied to map the remoteness of locations in the global environment from sources of chemical pollution,59,60 see Fig. 1. Metrics of environmental risk and human exposure potential for chemicals have been derived within the framework of fate and exposure models.61–65
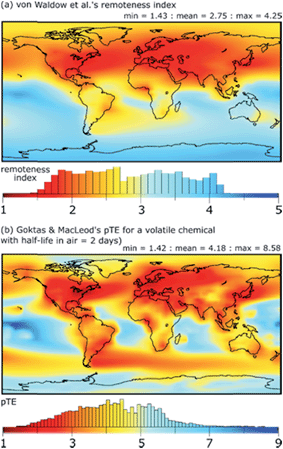 |
| Fig. 1 (a) Remoteness index calculated by von Waldow et al.59 using an atmospheric transport model (chemical independent), and (b) the negative log of transfer efficiency (pTE) of a volatile chemical calculated by Goktas & MacLeod60 using the BETR Global multimedia transport model. Higher values of remoteness index and pTE indicate locations on the Earth that are more remote from emissions of chemicals to air that occur in proportion to the intensity of night-time light observed from space. Histograms illustrate the area distribution of different values of remoteness index or pTE. | |
Multimedia models of the 1990s included bulk compartments for air, water, soil, and sediment. Several additional environmental compartments, sub-compartments and processes have been incorporated into fate and exposure models, including terrestrial and aquatic vegetation,66–79 DOC in terrestrial and aquatic systems,76,80,81 ice and snow,82–84 different types of aerosols,85 mountainous regions,86,87 and the effect of intermittent rain.88–90
The fundamental concept underlying all fate and exposure models is the integration of chemical transport, transfer and degradation processes into mass balance equations for the chemical investigated. This concept of setting up multi-media mass-balances is generally valid and goes beyond the most common type of models that have been developed and applied to non-ionizing, often long-lived, organic chemicals. For example, mass-balance models have been developed for nanoparticles91–98 and recently for microplastic.99
A class of organic chemicals of emerging concern are the ionizable organic substances. Given that the process descriptions of most of the current multi-media environmental fate models were derived from the well-established understanding of partitioning and mass transfer of neutral organic chemicals, expanding the domain of applicability of the models to address ionizable organics represents an important development. Unit world mass balance for ionizing chemicals using activity in lieu of fugacity have been presented.100,101 The multimedia activity model for ionizable (MAMI) organic chemicals has recently been modified in the development of a multimedia chemical fate model for China (SESAMe), designed using the nested structure of SimpleBox.102,103 The SimpleTreat model 4.0
104 has been updated to include improved handling of ionizable organics, thus providing a tool for estimating chemical fate and behaviour in domestic sewage and activated sludge systems for down-the-drain chemicals, such as pharmaceuticals.105
Model evaluation by comparison to field data
Fate and exposure models can be evaluated by comparing the model results to field data. If a model can reproduce field data (collected under conditions that correspond to the assumptions and settings in the model), this strongly increases the credibility of the model and demonstrates that the model is suitable for the chemical and environmental system considered. Results from fate and exposure models have often been found to agree well with field data; this includes at the global level,63,106–111 and at the continental level112–115 for selected persistent organic pollutants, and also in local and regional systems, such as the cases of diuron in a small, highly dynamic tropical catchment,116 plant protection products in agricultural catchments at different scales,117–121 PBDEs in a Swiss lake,122 and PCBs in the Adriatic Sea.123
Sensitivity and uncertainty analysis
Sensitivity and uncertainty analyses have become more and more common and are now an integral part of many fate and exposure modeling studies. MacLeod et al.124 provide a practical guide to sensitivity and uncertainty analysis for fate and exposure modeling studies. In the OECD Pov and LRTP Tool the user can activate the built-in options for a sensitivity and uncertainty analysis of the results for overall persistence and long-range transport potential. Becker et al. (2011)125 provide an extensive uncertainty analysis for the concentrations of endosulfan in air and seawater calculated with a global environmental fate model, and Wöhrnschimmel et al.126 propagated uncertainty in chemical properties and degradation half-lives of persistent pollutants through an analysis of the effects of climate change on pollutant transport to the Arctic.
Good modeling practice
Finally, the need of incorporating good modeling practice in the development and evaluation of environmental models was proposed by Jakeman et al. (2006);127 this was then specified by Buser et al.,128 who set up six principles of good modeling practice in the field of fate and exposure modeling. These principles range from a specification of the goal and scope of the assessment to specifying the model used, specifying all input and all output data, conducting a sensitivity analysis so that the most influential model parameters can be identified, and specifying the limitations of the model results and the modeling exercise.
Improved modeling of bioavailability of contaminants
Bioavailability of chemicals to terrestrial and aquatic environments and more generally the ecological realism of scenario descriptions has been pointed out as a critical issue to evaluate the exposure and therefore the toxicity of hydrophobic chemicals in the environment.7,129,130 Some models were recently published and have incorporated additional compartments and subcompartments (such as dissolved organic carbon, macrophytes, algae) to account for their influence on dynamically changing bioavailable concentrations,76,81,131,132 as well as compartment variability in scenarios.78,132,133
3. Models for small spatial scales and dynamic scenarios
In the 1990s fate and exposure models had mostly been developed to describe long-lived chemicals, such as organochlorine pesticides and PCBs. Analytical chemistry techniques for such substances required large-volume samples and intensive effort in the laboratory for analysis. Thus, the temporal and spatial resolution of available measurements was low, and, due to the persistent nature of the chemicals, long time scales were needed to observe trends in concentrations. Models developed in the 1990s correspondingly described environmental conditions using long-term average conditions for temperature, rain rate, wind speed, etc., and models were often applied using a steady-state assumption that represents a hypothetical conditions where emissions are balanced by removal processes.134 In the years since the 1990s it has been increasingly recognized that environmental fate models can be more flexible if they implement more rapidly changing conditions. This is needed if the chemical considered has short degradation half-lives and/or if there are strong and rapid changes of the environmental conditions. Advances in analytical chemistry capabilities have provided more measurements at higher spatial and temporal scales to provide context for these model scenarios and to support model-measurement comparisons that enable a process-based understanding of chemical dynamics. This also made it possible to appreciate the role of temporal and spatial changes of specific compartments, such as a pond or a forest, in driving concentration changes in the main compartments e.g. water and air.76,132,133,135
For example, Camenzuli et al. (2012)116 investigated the fate of the herbicide, diuron, in a small river catchment in Queensland, Australia. The problem in this case was that appreciable amounts of the diuron used on sugarcane and other crops in Northern Queensland were flushed into the Great Barrier Reef lagoon by water runoff. In the lagoon, the herbicide affects, among other effects, the seaweed and thereby wildlife living on seaweed (dugongs, turtles). There is a strong seasonal change in the rain rates in Northern Queensland and the rain rates in the wet season are very high (with peaks above 200 mm per day). Camenzuli et al. (2012)116 developed a model of the Tully River catchment with six compartments (agricultural soil, non-agricultural soil, seawater, sea sediment, air, water on land that is present under flooding conditions) and ran the model with very high temporal resolution (time steps on the order of seconds). This allowed them to drive the model with the highly dynamic actual rainfall data measured at a station in the Tully River catchment (Fig. 2a).
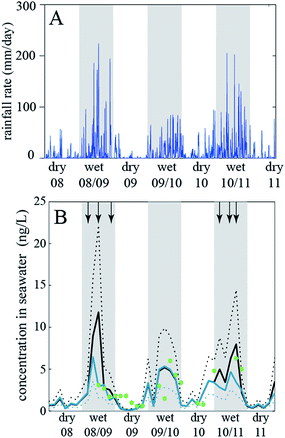 |
| Fig. 2 (A) Rainfall data from the Tully River catchment, Queensland, Australia, and (B) diuron concentrations measured in the Great Barrier Reef lagoon (dots) and calculated with the fate and exposure model (lines) by Camenzuli et al. (2012)116. Reprinted from The Science of the Total Environment, 440, L. Camenzuli et al., Describing the environmental fate of diuron in a tropical river catchment, pp. 178–185, Copyright (2012), with permission from Elsevier. | |
The model results of interest were the fluxes of diuron into the seawater compartment and the diuron concentrations in that compartment. Comparison with diuron concentrations measured in the lagoon shows that the model results agree well with the measured data, see Fig. 2b. The concentrations follow the seasonal rainfall trend.
In this way, the model was established as a tool that was then used to explore the effect of a diuron restriction scenario (no application of diuron in the wettest months of the year, November to April). Such a restriction would lead to a decrease in diuron concentrations in seawater by a factor of two. Overall, this case study shows that fate and exposure models are not limited to cases with “slow” processes and average environmental conditions, which is sometimes seen as a serious limitation of this type of model, but can also be set up in a way that they describe highly dynamic conditions with high accuracy.
Similarly to the diuron case, an integrated compartmental model (DynaPlus) was recently developed and used to predict the surface water contamination by pesticides used in an intensive orchard cultivation in a mountain region in Italy.121 Mountainous regions are characterized by rapid land response to precipitation, resulting in quick runoff chemical peaks and consequent potentially high peak exposure concentrations in aquatic ecosystems receiving such runoff. The model consisted of a dynamic water network model (built upon the Dyna model136) and a spatially explicit terrestrial multimedia model81,120 parametrized using specific scenario and emission data provided by the local authorities. The model incorporated an improved algorithm to calculate water runoff in steep slopes (average 30%, up to 80%) which allowed to more accurately calculate chemical runoff in these conditions. The integrated model was run for two cultivation years and results of chemical runoff from treated orchards were compared to ad hoc measured water concentrations for the organophosphorus insecticide chlorpyrifos in order to benchmark the model against field data. The model simulations showed that concentration peaks of chlorpyrifos in river water (Fig. 3) spanned and oscillated between 3 and 4 orders of magnitude, in the range of 0.1 to 1000 ng L−1, which is well within the range of the acute EC50 for Daphnia (around 100 ng L−1).137
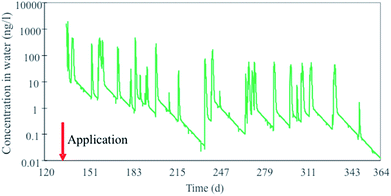 |
| Fig. 3 Peaks of chlorpyrifos concentrations in a mountain river (Novella) in Italy in 2012 calculated with the DynaPlus model of Morselli et al. (2018).121 | |
Given that ecotoxicity data (such as those for Daphnia) are usually determined at constant exposure, the results of these model simulations reveal the difficulty of evaluating the effect of higher concentration peaks but shorter in time (peak exposure) in protecting aquatic ecosystems.
A dynamic model for assessing pesticide residues in fruit in comparison against EU and US maximum residue levels has recently been presented by Mendez et al.138 in relation to the cultivation of bananas in Costa Rica. In addition to water, air, soil, and sediment compartments, Mendez et al.138 also included a banana plant and estimated the residues of diuron, ethoprofos, and epoxiconazole. Their results demonstrate the importance of dynamic models as decision-support tool that complements ongoing efforts to assess the ecological effects of exposure to chemical mixtures and transient pulses. For instance, such models can be helpful in minimizing the occurrence of pesticide pulses, by providing a decision-making tool with respect to the timing of pesticide applications.
4. Day-night cycling of SVOCs in boundary layer air
As sampling and analytical capabilities improved for atmospheric micropollutants, measurements of persistent semi-volatile pollutants with temporal resolution of several hours became more widely available in the 2000s. In 2007, MacLeod et al.139 developed a dynamic multimedia model that included variable atmospheric boundary layer height, temperature, hydroxyl radical concentrations and windspeed. They collected measurement data from six studies that reported concentrations of semi-volatile organic chemicals in air over consecutive 24 hour cycles, and demonstrated that in all cases the model could explain the drivers of day-night variability of concentrations in terms of variable process rates. Their analysis with the dynamic mass balance model identified four controlling factors that determine the day-night variability of concentrations of persistent semi-volatile chemicals in air: (1) source characteristics, (2) temperature dependent air-surface partitioning, (3) atmospheric mixing height and stability, and (4) hydroxyl radical concentration.
The insights from the 2007 modeling study informed the design of day-night measurement campaigns that were designed specifically to determine the rate of emissions of air pollutants from diffuse sources in urban areas. Several of these studies were carried out in Zurich, Switzerland to measure emission rates of PCBs,42,140 PBDEs,43,44 perfluoro alkyl substances,47 cyclic siloxanes,141 and PCDD/Fs.140 The recent quantification of ongoing PCB emissions in Zurich led the Swiss Federal Office for the Environment to re-open the question of PCB emission sources and to commission the set-up of a current and comprehensive PCB emission inventory for Switzerland.142
Mass balance models applied in conjunction with day-night measurement campaign data have demonstrated the potential to infer process rates for hydroxyl radical degradation of PCBs,139 for a more physically-based description of the air compartments in fugacity models, introducing hourly variable PBL (planetary boundary layer) compartment81,143,144 and for reversible air-vegetation exchange of PCBs, PBDEs and chlordane.42,145 Studies of this type provide the opportunity to evaluate the process descriptions provided by mass balance models in a relatively controlled situation, which helps to identify weaknesses in the models that can be improved, and ultimately improve model performance and reliability in applications at all spatio-temporal scales.
5. Environmental fate models as decision-support tools
Applying environmental fate models in decision-making requires that the models are understood by stakeholders, credible and that their reliability has been assessed. For multimedia models, the three key elements are: (i) the models themselves, i.e. the process descriptions and their mechanistic basis, and the model parameterization; (ii) the chemical property data used as model input; and (iii) the emission data, including their temporal and spatial resolution, used as model input.
Importantly, the three elements are independent of one another, which means that each of them may introduce a certain type and degree of error into the model results, but also that errors from these different elements can be traced separately, which makes it easier to constrain the source of error. The first element, process descriptions and model parameterization, strongly relies on the general laws of nature such as the law of the conservation of mass, the law of mass action, and the laws of chemical kinetics and diffusion, as well as empirical data reflecting the environmental conditions assumed in the model. Nevertheless, in setting up a model there is always, to some extent, room for choices to be made by the model developer. The effect of this variability in model setups can be assessed by model–model comparisons. An extensive model comparison was performed for multimedia fate models used to determine the overall persistence (Pov) and long-range transport potential (LRTP) of organic chemicals.3
The other two elements, chemical property data and emission data, often introduce greater error than the choice of model or details of the model setup. Chemical property data available in data bases and compilations often show surprisingly high variability and uncertainty; they need to be selected carefully and are ideally checked for their internal consistency, e.g. the octanol–air partition coefficient (Koa) needs to be equal to the ratio of Kow and the air–water partition coefficient, Kaw.146,147 Emission data are often the most uncertain and incomplete element,148 as discussed above.
Even when the property and emission data are selected carefully, there will be some remaining uncertainty that has to be accepted. For both, property and emission data, it is then advisable to determine a confidence factor or uncertainty range and to propagate this range through the model, for example by means of Monte-Carlo simulation or by applying the rules of error propagation.124
One environmental fate model that was developed explicitly for decision making purposes is the OECD Pov and LRTP Estimation Tool.5 Before the actual tool was created, nine different models for Pov and LRTP calculations were evaluated and compared in much detail.3 Also a set of well-characterized reference chemicals that define the scales of Pov and LRTP was introduced.4 Once the validity of the underlying concepts was established and the scope and purpose of the model within a decision-making context were defined, the specific setup of the tool, i.e. the format of the data input and display of results, the model algorithm, options for uncertainty analysis, and the software environment, was defined.5 Because the tool is based on a robust and common software environment (MS Excel for both Windows and Macintosh computers), is easy to use (reliable and robust handling of incorrect input), offers all results in both a graphical and numerical format, and is available free of charge from a trusted organization (OECD), it was quickly adopted by its users in government, research and industry. Since then, it has been used regularly for the evaluation of possible persistent organic pollutants in the context of the Stockholm Convention.
6. Outlook for improving the application of models within regulatory frameworks
This paper has summarized research conducted in the last three decades that addressed the needs identified by Cowan et al.2 and that evolved in the various workshops that have been held since then. Improvements in characterizing and quantifying physicochemical properties and emission estimates and refinements in model formulation and parameterization have led to better comparability between model results and monitoring data, which in turn imparts greater confidence to the use of models within regulatory chemical risk assessment. Overall, there has been considerable progress in model development, model transparency and model reliability. However, in the context of regulatory risk assessment it is important to strike a balance between increasing model complexity and diversity driven by scientific progress, on the one hand, and the need for models that are practical and relatively easy to use, on the other hand. Ongoing scientific development and the presence of open scientific questions must not reduce the availability of practical tools for regulatory risk assessment out of “paralysis by analysis”. Therefore, there is a continuous need for the translation of new scientific insights into the sphere of – often simplified – modeling tools suitable for regulatory risk assessment.
This translation process is a task in itself and requires time and resources. Participants from the 2017 ECETOC workshop expressed frustration regarding the lack of advances in environmental fate and exposure models that are included within the tools used for regulatory purposes. For instance, as described above, substantial progress has been made in expanding the applicability domain of the models with respect to both chemical and environmental properties. However, the European Union System for the Evaluation of Substances (EUSES), which is used for assessing exposure of general chemicals and biocides regulated under REACH, has not been updated to reflect advances in research, with the most recent update (EUSES 2.1.1) parameterized according to algorithms and assumptions described in the 2003 EU Technical Guidance Document.149 There is clearly a need to improve the pace at which advances in research are implemented within regulatory tools. However the process of evaluating new modeling approaches and adopting them into regulatory tools must be conducted in an open and transparent manner that facilitates building consensus among key stakeholders from industry, NGOs, government, and academia who are actively engaged in the development and use of environmental fate and exposure models.
The development of the OECD Tool demonstrated how bringing an expert group of modelers together can result in successful development and adoption of a regulatory modeling tool. Another example of an expert group building consensus about the application of exposure modeling within a regulatory context is the Forum for Co-ordination of pesticide fate models and their Use (FOCUS). FOCUS was an initiative of the European Commission, with the objective to harmonize the predicted environmental concentrations (PECs) of active substances of plant protection products (PPP) in the framework of the EU Directive 91/414/EEC. It provided a platform for close co-operation of expert groups of scientists drawn from regulatory agencies, academia, and industry under the auspices of the Commission's DG SANTE.
We propose that the creation of expert groups that follow the approaches provided by FOCUS and the OECD Tool is a fundamental element for achieving consensus among stakeholders to update and improve models used in regulatory exposure assessment. Multi-stakeholder expert groups provide a forum for communication and education about developments and application of environmental fate and exposure models. When the groups are tasked with a clear objective, they can work in a focused manner to define the attributes of a fate and exposure model that is “fit for purpose” to confront a regulatory question. Tools used at lower tiers of assessment will likely address questions that differ from tools used at higher tiers of assessment. Consequently, separate expert groups may be needed to confront related questions, or a single expert group might be tasked to develop a tiered set of models that address the problem at several levels of complexity. Ideally, an active and collaborative expert group that represents all key stakeholders should be able to define the tools, assumptions, and acceptable level of uncertainty associated with the various assessment tiers that might be required in a regulatory context. The work of these groups would include model comparison exercises, and critical evaluation of assumptions and limitations of models with the ultimate goal of providing recommendations for the application of existing models, or purpose-built new software for conducting exposure assessments that reflects scientific consensus among stakeholders.
We acknowledge that creating multi-stakeholder expert groups that are tasked to agree on how to best update regulatory tools used for chemical risk assessment is not likely to be a trivial exercise. It will require commitments of time and money from the stakeholders to be successful. In the specific case of EUSES, the process could build on the foundation provided by the list of high-priority corrections, improvements, and extensions that was published by van de Meent et al.,150 the key observations published in the 2013 EU scientific opinion,6 and the recommendations of the 2017 ECETOC workshop participants.151
A high priority for improving exposure models used in regulatory assessments is the need to expand the chemical applicability domain. The rapid development of target and non-target analytical chemistry techniques based on LC/MS over the last decades has made measurement and monitoring of environmental contaminants that are ionisable organic chemicals, including halogenated organic acids and chemicals used in pharmaceuticals and personal care products, an important area of environmental chemistry. Attention placed on these chemicals by their detection in the environment has been a key driver for drawing regulatory attention. Particularly at lower screening and prioritization tiers of assessment, regulatory models are currently not capable of handling these chemicals in a reliable manner, and a lack of reliable property estimation methods for such chemicals further hinders screening level assessments.
Many regulatory models are also not appropriate for describing measurement data collected at high temporal resolution (e.g. hours). The 2013 Scientific Opinion6 expressed a need for criteria and protocols for obtaining and comparing chemical monitoring data, especially for evaluating the fate of chemical mixtures, including metabolites. Such data should be collected and assembled in databases that can be used for model development, validation and benchmarking to support developing and calibrating higher temporal resolution models that could eventually be applied with confidence within a regulatory context.
In the absence of reliable property data and regulatory models that are appropriate for interpreting monitoring data and placing the data in the context of the environmental fate, transport and exposure of chemicals, measurements documenting the occurrence of pollutants place pressure on regulatory authorities to infer potential risks without a quantitative framework.152 Recent experiences with cyclic volatile methyl siloxanes,153,154 and the public policy debate about regulation of microplastics in consumer products155,156 illustrate the confusion this situation can create. It is therefore vital that chemical property measurement and estimation methods, and environmental fate and exposure modeling, continue to advance and evolve to support rational management decisions for the 21st century's environmental contaminants of concern.
Conflicts of interest
There are no conflicts of interest to declare.
Acknowledgements
This review article was motivated by discussions had during an ECETOC workshop, May 2017, the authors wish to acknowledge ECETOC for funding of the workshop and workshop participants. M. S. acknowledges financial support from the Czech Ministry of Education, Youth, and Sports (Grant LM2015051) and Masaryk University (CETOCOEN PLUS Project Grant CZ.02.1.01/0.0/0.0/15_003/0000469).
References
- M. MacLeod, M. Scheringer, T. E. McKone and K. Hungerbuhler, The State of Multimedia Mass-Balance Modeling in Environmental Science and Decision-Making, Environ. Sci. Technol., 2010, 44, 8360–8364 CrossRef CAS PubMed.
-
C. E. Cowan, D. Mackay, T. C. J. Feijtel, D. Van de Meent, A. Di Guardo, J. Davies and N. Mackay, The multi-media fate model: A vital tool for predicting the fate of chemicals, SETAC Press, Pensacola, FL, 1995 Search PubMed.
- K. Fenner, M. Scheringer, M. MacLeod, M. Matthies, T. McKone, M. Stroebe, A. Beyer, M. Bonnell, A. C. Le Gall, J. Klasmeier, D. Mackay, D. van de Meent, D. Pennington, B. Scharenberg, N. Suzuki and F. Wania, Comparing Estimates of Persistence and Long-Range Transport Potential among Multimedia Models, Environ. Sci. Technol., 2005, 39, 1932–1942 CrossRef CAS PubMed.
- J. Klasmeier, M. Matthies, M. MacLeod, K. Fenner, M. Scheringer, M. Stroebe, A. C. Le Gall, T. McKone, D. Van De Meent and F. Wania, Application of Multimedia Models for Screening Assessment of Long-Range Transport Potential and Overall Persistence, Environ. Sci. Technol., 2006, 40, 53–60 CrossRef CAS PubMed.
- F. Wegmann, L. Cavin, M. MacLeod, M. Scheringer and K. Hungerbühler, The OECD software tool for screening chemicals for persistence and long-range transport potential, Environ. Modell. Softw., 2009, 24, 228–237 CrossRef.
-
European Commission, Addressing the new challenges for risk assessment, European Commission and Directorate General for Health & Consumers, http://https://publications.europa.eu/en/publication-detail/-/publication/0132ea87-0420-4258-9ebf-ac49c9e4dcb0/language-en, Luxembourg, 2013 Search PubMed.
- A. Di Guardo and J. L. Hermens, Challenges for exposure prediction in ecological risk assessment, Integr. Environ. Assess. Manage., 2013, 9, e4–14 CrossRef PubMed.
- T. E. McKone and M. MacLeod, Tracking multiple pathways of human exposure to persistent multimedia pollutants: Regional, Continental, and Global-Scale Models, Annu. Rev. Environ. Resour., 2003, 28, 463–492 CrossRef.
-
Handbook of physical-chemical properties and environmental fate for organic chemicals, ed. D. Mackay, W. Y. Shiu and K. C. Ma, CRC/Taylor & Francis, Boca Raton, FL, 1992, vol. 1 Search PubMed.
- N. Li, F. Wania, Y. D. Lei and G. L. Daly, A Comprehensive and Critical Compilation, Evaluation, and Selection of Physical–Chemical Property Data for Selected Polychlorinated Biphenyls, J. Phys. Chem. Ref. Data, 2003, 32, 1545–1590 CrossRef CAS.
- H. Xiao, N. Li and F. Wania, Compilation, Evaluation, and Selection of Physical-Chemical Property Data for α-, β-, and γ-Hexachlorocyclohexane, J. Chem. Eng. Data, 2004, 49, 173–185 CrossRef CAS.
- Y.-G. Ma, Y. D. Lei, H. Xiao, F. Wania and W.-H. Wang, Critical Review and Recommended Values for the Physical-Chemical Property Data of 15 Polycyclic Aromatic Hydrocarbons at 25 °C, J. Chem. Eng. Data, 2010, 55, 819–825 CrossRef CAS.
- Z. Wang, M. MacLeod, I. T. Cousins, M. Scheringer and K. Hungerbühler, Using COSMOtherm to predict physicochemical properties of poly- and perfluorinated alkyl substances (PFASs), Environ. Chem., 2013, 8, 389–398 CrossRef.
- A. M. Buser, S. Schenker, M. Scheringer and K. Hungerbühler, Comparing the Performance of Computational Estimation Methods for Physicochemical Properties of Dimethylsiloxanes and Selected Siloxanols, J. Chem. Eng. Data, 2013, 58, 3170–3178 CrossRef CAS.
- J. Glüge, C. Bogdal, M. Scheringer, A. M. Buser and K. Hungerbühler, Calculation of Physicochemical Properties for Short- and Medium-Chain Chlorinated Paraffins, J. Phys. Chem. Ref. Data, 2013, 42, 023103 CrossRef.
- A. Åberg, M. MacLeod and K. Wiberg, Physical-Chemical Property Data for Dibenzo-p-dioxin (DD), Dibenzofuran (DF), and Chlorinated DD/Fs: A Critical Review and Recommended Values, J. Phys. Chem.
Ref. Data, 2008, 37, 1997–2008 CrossRef.
- A. Gawor and F. Wania, Using quantitative structural property relationships, chemical fate models, and the chemical partitioning space to investigate the potential for long range transport and bioaccumulation of complex halogenated chemical mixtures, Environ. Sci.: Processes Impacts, 2013, 15, 1671–1684 CAS.
- K.-U. Goss and R. P. Schwarzenbach, Linear Free Energy Relationships Used To Evaluate Equilibrium Partitioning of Organic Compounds, Environ. Sci. Technol., 2001, 35, 1–9 CrossRef CAS PubMed.
- C. M. Roth, K.-U. Goss and R. P. Schwarzenbach, Sorption of Diverse Organic Vapors to Snow, Environ. Sci. Technol., 2004, 38, 4078–4084 CrossRef CAS PubMed.
- K. U. Goss, Effects of temperature and relative humidity on the sorption of organic vapors on quartz sand, Environ. Sci. Technol., 1992, 26, 2287–2294 CrossRef CAS.
- K. U. Goss, Adsorption of organic vapors on polar mineral surfaces and on a bulk water surface: development of an empirical predictive model, Environ. Sci. Technol., 1994, 28, 640–645 CrossRef CAS PubMed.
- K.-U. Goss, Conceptual Model for the Adsorption of Organic Compounds from the Gas Phase to Liquid and Solid Surfaces, Environ. Sci. Technol., 1997, 31, 3600–3605 CrossRef CAS.
- C. M. Roth, K.-U. Goss and R. P. Schwarzenbach, Sorption of a Diverse Set of Organic Vapors To Diesel Soot and Road Tunnel Aerosols, Environ. Sci. Technol., 2005, 39, 6632–6637 CrossRef CAS PubMed.
- C. Niederer, K.-U. Goss and R. P. Schwarzenbach, Sorption Equilibrium of a Wide Spectrum of Organic Vapors in Leonardite Humic Acid: Modeling of Experimental Data, Environ. Sci. Technol., 2006, 40, 5374–5379 CrossRef CAS PubMed.
- C. W. Götz, M. Scheringer, M. MacLeod, C. M. Roth and K. Hungerbühler, Alternative Approaches for Modeling Gas-Particle Partitioning of Semivolatile Organic Chemicals: Model Development and Comparison, Environ. Sci. Technol., 2007, 41, 1272–1278 CrossRef.
- E. Reppas-Chrysovitsinos, A. Sobek and M. MacLeod, Screening-level models to estimate partition ratios of organic chemicals between polymeric materials, air and water, Environ. Toxicol. Chem., 2016, 18, 667–676 CAS.
- A. Franco and S. Trapp, Estimation of the soil-water partition coefficient normalized to organic carbon for ionizable organic chemicals, Environ. Toxicol. Chem., 2008, 27, 1995–2004 CrossRef CAS PubMed.
- A. Franco, W. Fu and S. Trapp, Influence of soil pH on the sorption of ionizable chemicals: Modeling advances, Environ. Toxicol. Chem., 2009, 28, 458–464 CrossRef CAS PubMed.
- A. Franco, A. Ferranti, C. Davidsen and S. Trapp, An unexpected challenge: ionizable compounds in the REACH chemical space, Int. J. Life Cycle Assess., 2010, 15, 321–325 CrossRef CAS.
- W. Fu, A. Franco and S. Trapp, Methods for estimating the bioconcentration factor of ionizable organic chemicals, Environ. Toxicol. Chem., 2009, 28, 1372–1379 CAS.
-
Handbook of chemical mass transport in the environment, ed. L. J. Thibodeaux and D. Mackay, CRC Press, Boco Raton, 2011 Search PubMed.
- K. Breivik, A. Sweetman, J. M. Pacyna and K. C. Jones, Towards a global historical emission inventory for selected PCB congeners–a mass balance approach 3. An update, Sci. Total Environ., 2007, 377, 296–307 CrossRef CAS PubMed.
- K. Breivik, A. Sweetman, J. Pacyna and K. Jones, Towards a global historical emission inventory for selected PCB congeners—a mass balance approach1. Global production and consumption, Sci. Total Environ., 2002, 290, 181–198 CrossRef CAS PubMed.
- K. Breivik, A. Sweetman, J. Pacyna and K. Jones, Towards a global historical emission inventory for selected PCB congeners—a mass balance approach 2. Emissions, Sci. Total Environ., 2002, 290, 199–224 CrossRef CAS PubMed.
- M. L. Diamond, L. Melymuk, S. A. Csiszar and M. Robson, Estimation of PCB stocks, emissions, and urban fate: will our policies reduce concentrations and exposure?, Environ. Sci. Technol., 2010, 44, 2777–2783 CrossRef CAS PubMed.
- S. A. Csiszar, S. M. Daggupaty, S. Verkoeyen, A. Giang and M. L. Diamond, SO-MUM: a coupled atmospheric transport and multimedia model used to predict intraurban-scale PCB and PBDE emissions and fate, Environ. Sci. Technol., 2013, 47, 436–445 CrossRef CAS PubMed.
- S. A. Csiszar, M. L. Diamond and S. M. Daggupaty, The magnitude and spatial range of current-use urban PCB and PBDE emissions estimated using a coupled multimedia and air transport model, Environ. Sci. Technol., 2014, 48, 1075–1083 CrossRef CAS PubMed.
- Z. Wang, I. T. Cousins, M. Scheringer, R. C. Buck and K. Hungerbuhler, Global emission inventories for C4-C14 perfluoroalkyl carboxylic acid (PFCA) homologues from 1951 to 2030, Part I: production and emissions from quantifiable sources, Environ. Int., 2014, 70, 62–75 CrossRef CAS PubMed.
- J. Glüge, Z. Wang, C. Bogdal, M. Scheringer and K. Hungerbuhler, Global production, use, and emission volumes of short-chain chlorinated paraffins – A minimum scenario, Sci. Total Environ., 2016, 573, 1132–1146 CrossRef PubMed.
- Y. F. Li, S. Venkatesh and D. Li, Modeling global emissions and residues of pesticides, Environ. Model. Assess., 2005, 9, 237–243 CrossRef.
- Y. Zhang and S. Tao, Global atmospheric emission inventory of polycyclic aromatic hydrocarbons (PAHs) for 2004, Atmos. Environ., 2009, 43, 812–819 CrossRef CAS.
- B. Gasic, C. Moeckel, M. MacLeod, J. r. Brunner, M. Scheringer, K. C. Jones and K. Hungerbühler, Measuring and Modeling Short-Term Variability of PCBs in Air and Characterization of Urban Source Strength in Zurich, Switzerland, Environ. Sci. Technol., 2009, 43, 769–776 CrossRef CAS PubMed.
- C. Moeckel, B. Gasic, M. MacLeod, M. Scheringer, K. C. Jones and K. Hungerbuhler, Estimation of the source strength of polybrominated diphenyl ethers based on their diel variability in air in Zurich, Switzerland, Environ. Sci. Technol., 2010, 44, 4225–4231 CrossRef CAS PubMed.
- C. Bogdal, Z. Wang, A. M. Buser, M. Scheringer, A. C. Gerecke, P. Schmid, C. E. Muller, M. MacLeod and K. Hungerbuhler, Emissions of polybrominated diphenyl ethers (PBDEs) in Zurich, Switzerland, determined by a combination of measurements and modeling, Chemosphere, 2014, 116, 15–23 CrossRef CAS PubMed.
- C. E. Muller, A. C. Gerecke, C. Bogdal, Z. Wang, M. Scheringer and K. Hungerbuhler, Atmospheric fate of poly- and perfluorinated alkyl substances (PFASs): I. Day-night patterns of air concentrations in summer in Zurich, Switzerland, Environ. Pollut., 2012, 169, 196–203 CrossRef PubMed.
- A. Parajulee and F. Wania, Evaluating officially reported polycyclic aromatic hydrocarbon emissions in the Athabasca oil sands region with a multimedia fate model, Proc. Natl. Acad. Sci. U. S. A., 2014, 111, 3344–3349 CrossRef CAS PubMed.
- Z. Wang, M. Scheringer, M. MacLeod, C. Bogdal, C. E. Muller, A. C. Gerecke and K. Hungerbuhler, Atmospheric fate of poly- and perfluorinated alkyl substances (PFASs): II. Emission source strength in summer in Zurich, Switzerland, Environ. Pollut., 2012, 169, 204–209 CrossRef CAS PubMed.
- M. Scheringer, Persistence and Spatial Range as Endpoints of an Exposure-Based Assessment of Organic Chemicals, Environ. Sci. Technol., 1996, 30, 1652–1659 CrossRef CAS.
- D. H. Bennett, T. E. McKone, M. Matthies and W. E. Kastenberg, General Formulation of Characteristic Travel Distance for Semivolatile Organic Chemicals in a Multimedia Environment, Environ. Sci. Technol., 1998, 32, 4023–4030 CrossRef CAS.
- A. Beyer, D. Mackay, M. Matthies, F. Wania and E. Webster, Assessing Long-Range Transport Potential of Persistent Organic Pollutants, Environ. Sci. Technol., 2000, 34, 699–703 CrossRef CAS.
- K. Fenner, M. Scheringer and K. Hungerbühler, Persistence of Parent Compounds and Transformation Products in a Level IV Multimedia Model, Environ. Sci. Technol., 2000, 34, 3809–3817 CrossRef CAS.
- M. Stroebe, M. Scheringer and K. Hungerbühler, Measures of Overall Persistence and the Temporal Remote State, Environ. Sci. Technol., 2004, 38, 5665–5673 CrossRef CAS PubMed.
- C. E. Cowan-Ellsberry, M. S. McLachlan, J. A. Arnot, M. MacLeod, T. E. McKone and F. Wania, Modeling Exposure to Persistent Chemicals in Hazard and Risk Assessment, Integr. Environ. Assess. Manage., 2009, 5, 662–679 CrossRef CAS PubMed.
- M. Scheringer, K. C. Jones, M. Matthies, S. L. Simonich and D. Van de Meent, Multimedia Partitioning, Overall Persistence, and Long-Range Transport Potential in the Context of POPs and PBT Chemical Assessments, Integr. Environ. Assess. Manage., 2009, 5, 557–576 CrossRef CAS PubMed.
- E. Reppas-Chrysovitsinos, A. Sobek and M. MacLeod, Screening-level exposure-based prioritization to identify potential POPs, vPvBs and planetary boundary threats among Arctic contaminants, Emerging Contam., 2017, 3, 85–94 CrossRef.
- F. Wania, Assessing the Potential of Persistent Organic Chemicals for Long-Range Transport and Accumulation in Polar Regions, Environ. Sci. Technol., 2003, 37, 1344–1351 CrossRef CAS.
- F. Wania, Potential of Degradable Organic Chemicals for Absolute and Relative Enrichment in the Arctic, Environ. Sci. Technol., 2006, 40, 569–577 CrossRef CAS PubMed.
- M. MacLeod and D. Mackay, Modeling transport and deposition of contaminants to ecosystems of concern: a case study for the Laurentian Great Lakes, Environ. Pollut., 2004, 128, 241–250 CrossRef CAS PubMed.
- H. von Waldow, M. MacLeod, M. Scheringer and K. Hungerbuhler, Quantifying remoteness from emission sources of persistent organic pollutants on a global scale, Environ. Sci. Technol., 2010, 44, 2791–2796 CrossRef CAS PubMed.
- R. K. Goktas and M. MacLeod, Remoteness from sources of persistent organic pollutants in the multi-media global environment, Environ. Pollut., 2016, 217, 33–41 CrossRef CAS PubMed.
- J. A. Arnot, D. Mackay, E. Webster and J. M. Southwood, Screening Level Risk Assessment Model for Chemical Fate and Effects in the Environment, Environ. Sci. Technol., 2006, 40, 2316–2323 CrossRef CAS PubMed.
- M. Stroebe, M. Scheringer and K. Hungerbuhler, Effects of multi-media partitioning of chemicals on Junge's variability-lifetime relationship, Sci. Total Environ., 2006, 367, 888–898 CrossRef CAS PubMed.
- S. Becker, C. J. Halsall, M. MacLeod, M. Scheringer, K. C. Jones and K. Hungerbühler, Empirical Investigation of the Junge Variability-Lifetime Relationship Using Long-Term Monitoring Data on Polychlorinated Biphenyl Concentrations in Air, Environ. Sci. Technol., 2009, 43, 2746–2752 CrossRef CAS PubMed.
- D. H. Bennett, T. E. McKone, J. S. Evans, W. W. Nazaroff, M. D. Margni, O. Jolliet and K. R. Smith, Defining Intake Fraction, Environ. Sci. Technol., 2002, 36, 206A–211A CrossRef.
- M. MacLeod and T. E. McKone, Multimedia Persistence as an Indicator of Potential for Population-Level Intake of Environmental Contaminants, Environ. Toxicol. Chem., 2004, 23, 2465–2472 CrossRef CAS PubMed.
- S. Trapp, M. Matthies, I. Scheunert and E. M. Topp, Modeling the bioconcentration of organic chemicals in plants, Environ. Sci. Technol., 1990, 24, 1246–1252 CrossRef CAS.
- M. S. McLachlan, K. Welsch-Pausch and J. Tolls, Field Validation of a Model of the Uptake of Gaseous SOC in Lolium multiflorum (Welsh Ray Grass), Environ. Sci. Technol., 1995, 29, 1998–2004 CrossRef CAS PubMed.
- M. S. McLachlan and M. Horstmann, Forests as Filters of Airborne Organic Pollutants: A Model, Environ. Sci. Technol., 1998, 32, 413–420 CrossRef CAS.
- F. Wania and M. S. McLachlan, Estimating the Influence of Forests on the Overall Fate of Semivolatile Organic Compounds Using a Multimedia Fate Model, Environ. Sci. Technol., 2001, 35, 582–590 CrossRef CAS PubMed.
- S. Trapp and K. S. B. Miglioranza, Sorption of Lipophilic Organic Compounds to Wood and Implications for Their Environmental Fate, Environ. Sci. Technol., 2001, 35, 1561–1566 CrossRef CAS PubMed.
- S. Trapp, Dynamic root uptake model for neutral lipophilic organics, Environ. Toxicol. Chem., 2002, 21, 203–206 CrossRef CAS PubMed.
- F. Wegmann, M. Scheringer, M. Möller and K. Hungerbühler, Influence of Vegetation on the Environmental Partitioning of DDT in Two Global Multimedia Models, Environ. Sci. Technol., 2004, 38, 1505–1512 CrossRef CAS PubMed.
- S. Trapp, Fruit Tree model for uptake of organic compounds from soil and air, SAR QSAR Environ. Res., 2007, 18, 367–387 CrossRef CAS PubMed.
- P. Fantke, R. Charles, L. F. de Alencastro, R. Friedrich and O. Jolliet, Plant uptake of pesticides and human health: dynamic modeling of residues in wheat and ingestion intake, Chemosphere, 2011, 85, 1639–1647 CrossRef CAS PubMed.
- F. A. Gobas, L. P. Burkhard, W. J. Doucette, K. G. Sappington, E. M. Verbruggen, B. K. Hope, M. A. Bonnell, J. A. Arnot and J. V. Tarazona, Review of existing terrestrial bioaccumulation models and terrestrial bioaccumulation modeling needs for organic chemicals, Integr. Environ. Assess. Manage., 2016, 12, 123–134 CrossRef CAS PubMed.
- M. Morselli, M. Semplice, F. De Laender, P. J. Van den Brink and A. Di Guardo, Importance of environmental and biomass dynamics in predicting chemical exposure in ecological risk assessment, Sci. Total Environ., 2015, 526, 338–345 CrossRef CAS PubMed.
- I. T. Cousins and D. Mackay, Strategies for including vegetation compartments in multimedia models, Chemosphere, 2001, 44, 643–654 CrossRef CAS PubMed.
- E. Terzaghi, M. Morselli, M. Semplice, B. E. L. Cerabolini, K. C. Jones, M. Freppaz and A. Di Guardo, SoilPlusVeg: An integrated air-plant-litter-soil model to predict organic chemical fate and recycling in forests, Sci. Total Environ., 2017, 595, 169–177 CrossRef CAS PubMed.
- M. S. McLachlan, Bioaccumulation of Hydrophobic Chemicals in Agricultural Food Chains, Environ. Sci. Technol., 1996, 30, 252–259 CrossRef CAS.
- J. M. Armitage, A. Franco, S. Gomez and I. T. Cousins, Modeling the Potential Influence of Particle Deposition on the Accumulation of Organic Contaminants by Submerged Aquatic Vegetation, Environ. Sci. Technol., 2008, 42, 4052–4059 CrossRef CAS PubMed.
- D. Ghirardello, M. Morselli, M. Semplice and A. Di Guardo, A dynamic model of the fate of organic chemicals in a multilayered air/soil system: development and illustrative application, Environ. Sci. Technol., 2010, 44, 9010–9017 CrossRef CAS PubMed.
- F. Wania, D. Mackay and J. T. Hoff, The Importance of Snow Scavenging of Polychlorinated Biphenyl and Polycyclic Aromatic Hydrocarbon Vapors, Environ. Sci. Technol., 1999, 33, 195–197 CrossRef CAS.
- G. L. Daly and F. Wania, Simulating the Influence of Snow on the Fate of Organic Compounds, Environ. Sci. Technol., 2004, 38, 4176–4186 CrossRef CAS PubMed.
- J. Stocker, M. Scheringer, F. Wegmann and K. Hungerbühler, Modeling the Effect of Snow and Ice on the Global Environmental Fate and Long-Range Transport Potential of Semivolatile Organic Compounds, Environ. Sci. Technol., 2007, 41, 6192–6198 CrossRef CAS PubMed.
- C. W. Götz, M. Scheringer, M. MacLeod, F. Wegmann and K. Hungerbühler, Regional differences in gas–particle partitioning and deposition of semivolatile organic compounds on a global scale, Atmos. Environ., 2008, 42, 554–567 CrossRef.
- G. L. Daly and F. Wania, Organic Contaminants in Mountains, Environ. Sci. Technol., 2005, 39, 385–398 CrossRef CAS PubMed.
- M. Morselli, M. Semplice, S. Villa and A. Di Guardo, Evaluating the temporal variability of concentrations of POPs in a glacier-fed stream food chain using a combined modeling approach, Sci. Total Environ., 2014, 493, 571–579 CrossRef CAS PubMed.
- E. G. Hertwich, Intermittent Rainfall in Dynamic Multimedia Fate Modeling, Environ. Sci. Technol., 2001, 35, 936–940 CrossRef CAS PubMed.
- O. Jolliet and M. Hauschild, Modeling the Influence of Intermittent Rain Events on Long-Term Fate and Transport of Organic Air Pollutants, Environ. Sci. Technol., 2005, 39, 4513–4522 CrossRef CAS PubMed.
-
M. MacLeod, M. Scheringer, C. W. Götz and K. Hungerbuehler, in Handbook of Chemical Mass Transport in the Environment, ed. L. J. Thibodeaux and D. Mackay, CRC Press, Boca Raton, FL, 2011, pp. 103–136 Search PubMed.
- M. Scheringer, Environmental risks of nanomaterials, Nat. Nanotechnol., 2008, 3, 322–323 CrossRef CAS PubMed.
- A. Praetorius, M. Scheringer and K. Hungerbuhler, Development of environmental fate models
for engineered nanoparticles–a case study of TiO2 nanoparticles in the Rhine River, Environ. Sci. Technol., 2012, 46, 6705–6713 CrossRef CAS PubMed.
- J. A. Meesters, A. A. Koelmans, J. T. Quik, A. J. Hendriks and D. van de Meent, Multimedia modeling of engineered nanoparticles with SimpleBox4nano: model definition and evaluation, Environ. Sci. Technol., 2014, 48, 5726–5736 CrossRef CAS PubMed.
- R. Jacobs, J. A. Meesters, C. J. Ter Braak, D. van de Meent and H. van der Voet, Combining exposure and effect modeling into an integrated probabilistic environmental risk assessment for nanoparticles, Environ. Toxicol. Chem., 2016, 35, 2958–2967 CrossRef CAS PubMed.
- B. Nowack, Evaluation of environmental exposure models for engineered nanomaterials in a regulatory context, NanoImpact, 2017, 8, 38–47 CrossRef.
- A. L. Dale, E. A. Casman, G. V. Lowry, J. R. Lead, E. Viparelli and M. Baalousha, Modeling nanomaterial environmental fate in aquatic systems, Environ. Sci. Technol., 2015, 49, 2587–2593 CrossRef CAS PubMed.
- K. L. Garner, S. Suh and A. A. Keller, Assessing the Risk of Engineered Nanomaterials in the Environment: Development and Application of the nanoFate Model, Environ. Sci. Technol., 2017, 51, 5541–5551 CrossRef CAS PubMed.
- H. H. Liu and Y. Cohen, Multimedia environmental distribution of engineered nanomaterials, Environ. Sci. Technol., 2014, 48, 3281–3292 CrossRef CAS PubMed.
- E. Besseling, J. T. Quik, M. Sun and A. A. Koelmans, Fate of nano- and microplastic in freshwater systems: A modeling study, Environ. Pollut., 2017, 220, 540–548 CrossRef CAS PubMed.
- S. Trapp, A. Franco and D. Mackay, Activity-based concept for transport and partitioning of ionizing organics, Environ. Sci. Technol., 2010, 44, 6123–6129 CrossRef CAS PubMed.
- A. Franco and S. Trapp, A multimedia activity model for ionizable compounds: validation study with 2,4-dichlorophenoxyacetic acid, aniline, and trimethoprim, Environ. Toxicol. Chem., 2010, 29, 789–799 CrossRef CAS PubMed.
- Y. Zhu, O. R. Price, J. Kilgallon, C. Rendal, S. Tao, K. C. Jones and A. J. Sweetman, A Multimedia Fate Model to Support Chemical Management in China: A Case Study for Selected Trace Organics, Environ. Sci. Technol., 2016, 50, 7001–7009 CrossRef CAS PubMed.
- Y. Zhu, O. R. Price, S. Tao, K. C. Jones and A. J. Sweetman, A new multimedia contaminant fate model for China: how important are environmental parameters in influencing chemical persistence and long-range transport potential?, Environ. Int., 2014, 69, 18–27 CrossRef CAS PubMed.
-
J. Struijs, Application of SimpleTreat 4.0 in European substance regulations, Report 1862–4804, On behalf of the Federal Environment Agency, Germany, Dessau-Roßlau, Germany, 2015 Search PubMed.
- L. S. Lautz, J. Struijs, T. M. Nolte, A. M. Breure, E. van der Grinten, D. van de Meent and R. van Zelm, Evaluation of SimpleTreat 4.0: Simulations of pharmaceutical removal in wastewater treatment plant facilities, Chemosphere, 2017, 168, 870–876 CrossRef CAS PubMed.
- J. M. Armitage, M. MacLeod and I. T. Cousins, Modeling the Global Fate and Transport of Perfluorooctanoic Acid (PFOA) and Perfluorooctanoate (PFO) Emitted from Direct Sources Using a Multispecies Mass Balance Model, Environ. Sci. Technol., 2009, 43, 1134–1140 CrossRef CAS PubMed.
- L. Lamon, H. von Waldow, M. MacLeod, M. Scheringer, A. Marcomini and K. Hungerbühler, Modeling the Global Levels and Distribution of Polychlorinated Biphenyls in Air under a Climate Change Scenario, Environ. Sci. Technol., 2009, 43, 5818–5824 CrossRef CAS PubMed.
- M. MacLeod, W. J. Riley and T. E. McKone, Assessing the Influence of Climate Variability on Atmospheric Concentrations of Polychlorinated Biphenyls Using a Global-Scale Mass Balance Model (BETR-Global), Environ. Sci. Technol., 2005, 39, 6749–6756 CrossRef CAS PubMed.
- F. Wania and D. Mackay, A global distribution model for persistent organic chemicals, Sci. Total Environ., 1995, 160/161, 211–232 CrossRef.
- H. Wöhrnschimmel, P. Tay, H. von Waldow, H. Hung, Y. F. Li, M. MacLeod and K. Hungerbuhler, Comparative assessment of the global fate of alpha- and beta-hexachlorocyclohexane before and after phase-out, Environ. Sci. Technol., 2012, 46, 2047–2054 CrossRef PubMed.
- F. Wania, D. Mackay, Y.-F. Li, T. Bidleman and A. Strand, Global chemical fate of α-hexachlorocyclohexane. 1. Evaluation of a global distribution model, Environ. Toxicol. Chem., 1999, 18, 1390–1399 CrossRef CAS.
- M. MacLeod, D. G. Woodfine, D. Mackay, T. McKone, D. Bennett and R. Maddalena, BETR North America: A regionally segmented multimedia contaminant fate model for North America, Environ. Sci. Pollut. Res., 2008, 8, 156–163 CrossRef.
- K. Prevedouros, K. C. Jones and A. J. Sweetman, European-Scale Modeling of Concentrations and Distribution of Polybrominated Diphenyl Ethers in the Pentabromodiphenyl Ether Product, Environ. Sci. Technol., 2004, 38, 5993–6001 CrossRef CAS PubMed.
- M. R. Earnshaw, K. C. Jones and A. J. Sweetman, A first European scale multimedia fate modelling of BDE-209 from 1970 to 2020, Environ. Int., 2015, 74, 71–81 CrossRef CAS PubMed.
- F. Wania, K. Breivik, N. J. Persson and M. S. McLachlan, CoZMo-POP 2 – A fugacity-based dynamic multi-compartmental mass balance model of the fate of persistent organic pollutants, Environ. Modell. Softw., 2006, 21, 868–884 CrossRef.
- L. Camenzuli, M. Scheringer, C. Gaus, C. A. Ng and K. Hungerbuhler, Describing the environmental fate of diuron in a tropical river catchment, Sci. Total Environ., 2012, 440, 178–185 CrossRef CAS PubMed.
- A. Di Guardo, D. Calamari, G. Zanin, A. Consalter and D. Mackay, A fugacity model of pesticide runoff to surface water: Development and validation, Chemosphere, 1994, 28, 511–531 CrossRef CAS.
- R. Barra, M. Vighi and A. Di Guardo, Prediction of surface water input of chloridazon and chlorpyrifos from an agricultural watershed in Chile, Chemosphere, 1995, 30, 485–500 CrossRef CAS.
- A. Di Guardo, R. J. Williams, P. Matthiessen, D. N. Brooke and D. Calamari, Simulation of pesticide runoff at Rosemaund Farm (UK) using the SoilFug model, Environ. Sci. Pollut. Res., 1994, 1, 151–160 CrossRef PubMed.
- D. Ghirardello, M. Morselli, S. Otto, G. Zanin and A. Di Guardo, Investigating the need for complex vs. simple scenarios to improve predictions of aquatic ecosystem exposure with the SoilPlus model, Environ. Pollut., 2014, 184, 502–510 CrossRef CAS PubMed.
- M. Morselli, C. M. Vitale, A. Ippolito, S. Villa, R. Giacchini, M. Vighi and A. Di Guardo, Predicting pesticide fate in small cultivated mountain watersheds using the DynAPlus model: Toward improved assessment of peak exposure, Sci. Total Environ., 2018, 615, 307–318 CrossRef CAS PubMed.
- C. Bogdal, M. Scheringer, P. Schmid, M. Blauenstein, M. Kohler and K. Hungerbuhler, Levels, fluxes and time trends of persistent organic pollutants in Lake Thun, Switzerland: combining trace analysis and multimedia modeling, Sci. Total Environ., 2010, 408, 3654–3663 CrossRef CAS PubMed.
- L. Lamon, M. MacLeod, A. Marcomini and K. Hungerbuhler, Modeling the influence of climate change on the mass balance of polychlorinated biphenyls in the Adriatic Sea, Chemosphere, 2012, 87, 1045–1051 CrossRef CAS PubMed.
- M. MacLeod, A. J. Fraser and D. Mackay, Evaluating and expressing the propagation of uncertainty in chemical fate and bioaccumulation models, Environ. Toxicol. Chem., 2002, 21, 700–709 CrossRef CAS PubMed.
- L. Becker, M. Scheringer, U. Schenker and K. Hungerbuhler, Assessment of the environmental persistence and long-range transport of endosulfan, Environ. Pollut., 2011, 159, 1737–1743 CrossRef CAS PubMed.
- H. Wöhrnschimmel, M. MacLeod and K. Hungerbuhler, Emissions, fate and transport of persistent organic pollutants to the Arctic in a changing global climate, Environ. Sci. Technol., 2013, 47, 2323–2330 CrossRef PubMed.
- A. J. Jakeman, R. A. Letcher and J. P. Norton, Ten iterative steps in development and evaluation of environmental models, Environ. Modell. Softw., 2006, 21, 602–614 CrossRef.
- A. M. Buser, M. MacLeod, M. Scheringer, D. Mackay, M. Bonnell, M. H. Russell, J. V. DePinto and K. Hungerbuhler, Good modeling practice guidelines for applying multimedia models in chemical assessments, Integr. Environ. Assess. Manage., 2012, 8, 703–708 CrossRef CAS PubMed.
-
R. P. Schwarzenbach, P. M. Gschwend and D. M. Imboden, Environmental Organic Chemistry, John Wiley & Sons, Inc., Hoboken, New Jersey, 3rd edn, 2017.
- X. Cui, P. Mayer and J. Gan, Methods to assess bioavailability of hydrophobic organic contaminants: Principles, operations, and limitations, Environ. Pollut., 2013, 172, 223–234 CrossRef CAS PubMed.
- F. De Laender, P. J. van den Brink, C. R. Janssen and A. Di Guardo, The ChimERA project: coupling mechanistic exposure and effect models into an integrated platform for ecological risk assessment, Environ. Sci. Pollut. Res., 2014, 21, 6263–6267 CAS.
- A. Di Guardo, M. Morselli, G. Morabito, M. Semplice, P. J. Van den Brink and F. De Laender, European environmental scenarios of chemical bioavailability in freshwater systems, Sci. Total Environ., 2017, 580, 1237–1246 CrossRef CAS PubMed.
- E. Terzaghi, G. Zacchello, M. Scacchi, G. Raspa, K. C. Jones, B. Cerabolini and A. Di Guardo, Towards more ecologically realistic scenarios of plant uptake modelling for chemicals: PAHs in a small forest, Sci. Total Environ., 2015, 505, 329–337 CrossRef CAS PubMed.
- F. Wania and D. Mackay, The evolution of mass balance models of persistent organic pollutant fate in the environment, Environ. Pollut., 1999, 100, 223–240 CrossRef CAS PubMed.
- M. Morselli, E. Terzaghi and A. Di Guardo, Do environmental dynamics matter in fate models? Exploring scenario dynamics for a terrestrial and an aquatic system, Environ. Sci.: Processes Impacts, 2018 10.1039/c7em00530j.
- A. Di Guardo, C. Ferrari and A. Infantino, Development of a Dynamic Aquatic Model (DynA Model): Estimating Temporal Emissions of DDT to Lake Maggiore (N. Italy), Environ. Sci. Pollut. Res., 2005, 13, 50–58 CrossRef.
-
U. S. E. P. A. (EPA), ECOTOX database, https://cfpub.epa.gov/ecotox/, accessed October 2017 Search PubMed.
- A. Mendez, L. E. Castillo, C. Ruepert, K. Hungerbuehler and C. A. Ng, Tracking pesticide fate in conventional banana cultivation in Costa Rica: A disconnect between protecting ecosystems and consumer health, Sci. Total Environ., 2017, 613–614, 1250–1262 Search PubMed.
- M. MacLeod, M. Scheringer, H. Podey, K. C. Jones and K. Hungerbühler, The Origin and Significance of Short-Term Variability of Semivolatile Contaminants in Air, Environ. Sci. Technol., 2007, 41, 3249–3253 CrossRef CAS PubMed.
- C. Bogdal, C. E. Muller, A. M. Buser, Z. Wang, M. Scheringer, A. C. Gerecke, P. Schmid, M. Zennegg, M. MacLeod and K. Hungerbuhler, Emissions of polychlorinated biphenyls, polychlorinated dibenzo-p-dioxins, and polychlorinated dibenzofurans during 2010 and 2011 in Zurich, Switzerland, Environ. Sci. Technol., 2014, 48, 482–490 CrossRef CAS PubMed.
- A. M. Buser, A. Kierkegaard, C. Bogdal, M. MacLeod, M. Scheringer and K. Hungerbuhler, Concentrations in ambient air and emissions of cyclic volatile methylsiloxanes in Zurich, Switzerland, Environ. Sci. Technol., 2013, 47, 7045–7051 CrossRef CAS PubMed.
- J. Glüge, C. Steinlin, S. Schalles, L. Wegmann, J. Tremp, K. Breivik, K. Hungerbuhler and C. Bogdal, Import, use, and emissions of PCBs in Switzerland from 1930 to 2100, PLoS One, 2017, 12, e0183768 Search PubMed.
- M. Morselli, D. Ghirardello, M. Semplice and A. Di Guardo, Modeling short-term variability of semivolatile organic chemicals in air at a local scale: an integrated modeling approach, Environ.
Pollut., 2011, 159, 1406–1412 CrossRef CAS PubMed.
- M. Morselli, D. Ghirardello, M. Semplice, G. Raspa and A. Di Guardo, Integration of an atmospheric dispersion model with a dynamic multimedia fate model: development and illustration, Environ. Pollut., 2012, 164, 182–187 CrossRef CAS PubMed.
- C. Moeckel, M. MacLeod, K. Hungerbühler and K. C. Jones, Measurement and Modeling of Diel Variability of Polybrominated Diphenyl Ethers and Chlordanes in Air, Environ. Sci. Technol., 2008, 42, 3219–3225 CrossRef CAS PubMed.
- A. Beyer, F. Wania, T. Gouin, D. Mackay and M. Matthies, Selecting internally consistent physicochemical properties of organic compounds, Environ. Toxicol. Chem., 2002, 21, 941–953 CrossRef CAS PubMed.
- U. Schenker, M. MacLeod, M. Scheringer and K. Hungerbühler, Improving Data Quality for Environmental Fate Models: A Least-Squares Adjustment Procedure for Harmonizing Physicochemical Properties of Organic Compounds, Environ. Sci. Technol., 2005, 39, 8434–8441 CrossRef CAS PubMed.
- A. Hollander, M. Hauck, I. T. Cousins, M. A. J. Huijbregts, A. Pistocchi, A. M. J. Ragas and D. van de Meent, Assessing the Relative Importance of Spatial Variability in Emissions Versus Landscape Properties in Fate Models for Environmental Exposure Assessment of Chemicals, Environ. Model. Assess., 2012, 17, 577–587 CrossRef.
-
European Chemicals Bureau, Technical Guidance Document on Risk Assessment, European Commission, 2003 Search PubMed.
-
D. Van de Meent, J. T. Quik and T. Traas, Identification and preliminary analysis of update needs for EUSES, RIVM, 2014 Search PubMed.
-
ECETOC, Advances in Exposure Modelling: Bridging the gap between research and application, ECETOC, Brussels, 2018.
- A. Cimorelli and C. Stahl, Avoiding “Proofiness”: Addressing uncertainty in environmental characterization, Integr. Environ. Assess. Manage., 2014, 10, 141–142 CrossRef.
- D. Mackay, Risk assessment and regulation of D5 in Canada: Lessons learned, Environ. Toxicol. Chem., 2015, 34, 2687–2688 CrossRef CAS PubMed.
- M. S. McLachlan, Can the Stockholm Convention address the spectrum of chemicals currently under regulatory scrutiny? Advocating a more prominent role for modeling in POP screening assessment, Environ. Sci.: Processes Impacts, 2018 10.1039/c7em00473g.
- A. A. Koelmans, E. Besseling, E. Foekema, M. Kooi, S. Mintenig, B. C. Ossendorp, P. E. Redondo-Hasselerharm, A. Verschoor, A. P. van Wezel and M. Scheffer, Risks of Plastic Debris: Unravelling Fact, Opinion, Perception, and Belief, Environ. Sci. Technol., 2017, 51, 11513–11519 CrossRef CAS PubMed.
- G. A. Burton Jr, Stressor Exposures Determine Risk: So, Why Do Fellow Scientists Continue To Focus on Superficial Microplastics Risk?, Environ. Sci. Technol., 2017, 51, 13515–13516 CrossRef PubMed.
|
This journal is © The Royal Society of Chemistry 2018 |