DOI:
10.1039/C8AN01363B
(Paper)
Analyst, 2018,
143, 6037-6048
New insights of Raman spectroscopy for oral clinical applications
Received
20th July 2018
, Accepted 22nd October 2018
First published on 23rd October 2018
Abstract
Oral injuries are currently diagnosed by histopathological analysis of biopsy, which is an invasive procedure and does not give immediate results. On the other hand, the Raman spectroscopy technique is a real-time and minimally invasive analytical tool with a notable diagnostic capability. At the current stage, researchers are widely aware of the diagnostic potential of the technique and how it is considered promising for providing biochemical information in real time and without damaging the tissue. The problem originates from a lack of relevant studies and clinical trials that could show the actual use of Raman spectroscopy to help patients. Our goal here is to narrow the relationship between physicists, chemists, engineers, computer scientists, and the medical community, and in fact discuss the potential of Raman spectroscopy as a novel clinical analysis method. In the present study, we focused on the use of Raman spectroscopy as a daily clinical practice. In this context, additional studies and in vivo tests should be performed with the same approach as a real application. We want to show the scientific and industrial community what is really necessary for this, starting from a clinical point of view. Using our previous experience publishing different oral pathologies and types of samples, we also aim to discuss the current state and potential of Raman spectroscopy and what is required to implement Raman spectroscopy for oral clinical applications.
Introduction
Many researchers have conducted studies to introduce Raman spectroscopy as a new approach for clinicians. However, there is very little data suggesting that it has strong potential to help patients. At this stage, the point is how many of its capabilities we could use in our daily clinic. First, we need to share the applications for different purposes. As an example, a cell culture study which reports the difference between the nucleus and the cytoplasm is very important to show the big potential of the technique, but it does not have any clinical applications in terms of in vivo diagnostics or surgical guidance. Even though the technique (Raman spectroscopy) is the same, we have shown that if the instrument, probe, samples, etc. are different, the final spectrum is totally different and cannot be related without taking into consideration the optical properties of biological tissues.1–6
In this way, we should primarily target diverse clinical applications. This includes on-site clinical applications and surgical guidance, involving the use of in vivo portable Raman systems or rapid assessment of ex vivo samples in the hospital, very similar to the evaluation of a frozen biopsy. Second, aiding the pathologists in their laboratories, it would be possible to use a micro-Raman system for cell cultures or histological samples to obtain molecular information, which cannot be assessed by using standard histological analysis. This information is extremely valuable and important to be publicized to clinicians so that they could contribute by improving the ideas about what patients really need. Obviously, many initial studies were performed by physicians without the presence and opinions of clinicians because they are really preliminary. However, at the current stage, we consider many of these studies to be not suitable for clinical translation, as, from our clinical experience, their published results will never be applied in the clinic. This occurs due to the simple fact that these studies do not have any applicability.
Also, two important professionals should be supported in the whole process, apart from clinicians, physicians and chemists: biomedical engineers and computer scientists. We should incorporate these professionals into the team in order to give research groups a chance to interact with medical device companies that build Raman systems and to develop customized instruments for clinical applications. Computer scientists and related professionals are required in order to create and adapt scripts (in MATLAB or other software) for control, automation, and generation of user-friendly interfaces, and fast data acquisition and processing to help patients in terms of diagnostic and treatment monitoring.
This paper aims to discuss the current state and potential of Raman spectroscopy and what is required to implement Raman spectroscopy for oral clinical applications.
Oral diseases and optical biopsy
Oral health has a strong impact on systemic health and quality of life. It is defined as a “state of being free from mouth and facial pain, oral and throat cancer, oral infection and sores, periodontal (gum) disease, tooth decay, tooth loss, and other diseases and disorders that limit an individual's capacity in biting, chewing, smiling, speaking, and psychosocial wellbeing”1 by the World Health Organization (WHO). The WHO has promoted health improvements and oral disease prevention by building an oral health database2 through the “WHO Oral Health Country/Area Profile Programme” in the Country/Area Profile Project CAPP and by implementing the directions of the Global Oral Health Programme.3,4
Global initiatives allowed the development of preventive dentistry. In preventive dentistry, important initiatives for the implementation of oral health programmes and integrated interventions were discussed in the last 11th International Association for Dental Research (IADR) World Congress on Preventive Dentistry.5 Among the oral health topics, the prevention of oral disorders and conditions such as dental cavities (60–90% of school children and nearly 100% of adults have dental cavities worldwide), periodontal disease (severe cases are found in 15–20% of middle-aged (35–44 years) adults), tooth loss (globally, about 30% of people aged 65–74 have no natural teeth), oral cancer (incidence ranges from one to 10 cases per 100
000 people in most countries), fungal, bacterial or viral infections in HIV, oro-dental trauma, noma, and cleft lip and palate is highlighted.1
In order to prevent oral disorders, many programmes have focused on optical technologies, which can provide a fast, non-invasive, molecular-sensitive, and in situ analysis of biological tissues. Optical techniques have the potential to be integrated in medical/dentist tools and can be relatively low cost compared to techniques such as magnetic resonance imaging. These techniques are widely exploited in pre-clinical and clinical studies to evaluate the potential for implementation of an “optical biopsy”6–23 or “spectral cytopathology”.24 These latter concepts refer to the use of optical technologies as tools for clinical diagnostics, having a strong association with the current gold standard for disease diagnosis: the biopsy followed by histopathology analysis. In a conventional clinical setting, patients need to wait for a clinical-laboratory diagnosis, which may be time-consuming. This includes a set of examinations including biopsies and biofluid analysis. The waiting time can be extremely dangerous for certain oral injuries, since most of them are diagnosed in a later stage. This makes the treatment of oral diseases more expensive and difficult, compromises patients’ prognosis, and, consequently, increases the demand for doctors’ and dentists’ attention. With this in mind, optical biopsies and biofluid analyses can be accelerated by using optical diagnosis technologies, such as Raman spectroscopy.
Raman spectroscopy
Two of the most powerful optical techniques are Raman spectroscopy and imaging (or mapping). They have the advantage of being extremely molecular specific, since the captured signal has the features of molecular bonds. Both optical techniques rely on the collection of Raman scattered photons, i.e., photons that undergo inelastic scattering through energy exchange with vibrational or rotational modes of molecular bonds.25 Many studies in ex vivo and in vivo biological tissues have reported the success of the proof-of-concept in the use of Raman spectroscopy and imaging for the identification of oral diseases such as cancer or inflammatory diseases.26 In addition, in vitro studies report changes at a subcellular and a cellular level, which can be associated with what is observed in a clinical study.24 However, biological variability is a factor that must be considered and is not consistently monitored. Advances in instrument probe design and automated analysis are still under development.25,27,28
In this context, Raman spectroscopy can be applied in many areas, such as the diagnosis of early and difficult diseases, but there is a need for communication between basic science (physicists, chemists, engineers and others) and applied science (doctors and dentists, among others) so that researches have a higher usability and are not lost with time. For example, an in vivo Raman system will be used clinically when we need a fast real-time response, and an imaged micro-Raman system may assist the pathologist in a laboratory for more elaborate analyses that are difficult to perform. The pathologist often needs complementary information from immunohistochemical techniques and immunofluorescence, since histology alone is not enough.
Many clinicians are not aware of Raman spectroscopy or of its diagnostic ability. This hinders the progression of Raman spectroscopy in the clinical environment. The importance of presenting research at medical conferences, and in scientific journals with medical applications is certainly a viable alternative to increase clinicians’ participation in Raman spectroscopy projects.
Raman spectroscopy and imaging have been implemented for in vitro cell studies with two main approaches: identifying cancer cells (Raman microscopy or microspectroscopy) and investigation of subcellular processes and/or the microenvironment. The first one was successful in differentiating a variety of cell types including neoplastic or normal hematopoietic cells,29 and leukemia cells, among other types of blood cells,30 and oral normal, dysplastic epithelial cells, and oral epithelial cancer.24 These differences result mostly from the analysis of nucleic acids, lipids, carbohydrates, and protein components (such as amide I).
Studies of subcellular processes have focused on the discovery of cellular origins of diseases that can be probed with Raman spectroscopy. Once these origins are clarified, choosing specific diseases to probe using Raman spectroscopy and evaluating its potential for diagnostics become easier. One example is the composition of lipid droplets in the cell cytoplasm, which is related to atherosclerosis and hepatitis C infection.31–33 The cellular microenvironment has been studied using surface enhanced Raman spectroscopy (SERS) to monitor pH and enzymatic activity in cells.34–38 Although SERS studies present a very advantageous concept, we have observed in recent years that its application has become increasingly distant from clinical practice, due to the instability of the signal found, difficult manipulation and reproducibility.
Previously reported studies involving ex vivo tissues are divided into two main approaches: a complementary tool for histopathological analysis and an alternative analysis of freshly excised tissues. The first approach aims to help pathologists by providing a chemical analysis after processing and staining the specimen. One alternative is reported by Lutz et al., who demonstrated a similar performance between SERS immunostaining and conventional fluorescent immunostaining techniques.39 The second approach is focused on the identification and quantification of chemical differences in freshly excised tissues. This is a powerful method in terms of reducing the time required to process the sample, since Raman spectroscopy and/or imaging can provide an immediate biochemical analysis of the specimen. However, differences in methodology such as the time between the tissue removal and data collection, the time when the blood supply is restricted, ambient conditions (such as ambient light, temperature, and humidity), and probe contact pressure hinder the possibility of collecting reproducible and reliable data. Ex vivo studies using this approach include the differentiation between benign and cancerous lesions in breast tissue,40 identification of parathyroid adenomas and hyperplasia in parathyroid tissue,41 and estimation of higher lipid, protein, collagen, nucleic acid, and glycogen content in normal and cancerous bladder tissues.42 By using Coherent anti-Stokes Raman scattering (CARS), a good correlation between H&E staining and CARS imaging was reported in ex vivo mouse brain tissue,43 and tissue morphological changes could be monitored in endothelial and smooth muscle cells of carotid arteries (collagen fibrils and elastin),44 spinal tissues and mouse sciatic nerves (demyelination and myelin repair processes in myelin sheaths).45
Oral clinical applications of Raman spectroscopy
Recent oral clinical applications of Raman spectroscopy can be divided into disease detection,46,47 determination of surgical margins,48 and estimation of treatment response.47,49–51 Disease detection includes studies using point measurements,52–54 Raman microscopy,55–58 and Raman mapping.59–63 Raman microscopy can give access to biomolecular and cellular information in the same image, which may be useful to understand pathological processes involved in the studied diseases. On the other hand, Raman microscopy may be difficult to translate to the clinic. The advantage of Raman mapping is its spatial resolution that allows border identification and image feature extraction. However, most of the reported studies use point measurements in order to obtain better spectral quality in shorter times. Disease detection applications are also dependent on the sample that is being probed and will be further discussed in the next section. Assessment of surgical margins can be performed by both point measurements52 and Raman imaging.61,64,65 Imaging is more suitable for this particular application and can be better adapted to display the surgical margins for precise lesion removal. However, the implementation of this application includes the development of sophisticated apparatus to provide real-time and user-friendly information to guide the surgeon. Therefore, this implementation may be challenging to accomplish at low cost, especially when the existing technology available in the market is taken into consideration. Finally, few studies report treatment response prediction, including oral cancer cell monitoring,66 serum analysis,67 and in vivo analysis.68 In this case, studies in ex vivo and in vivo tissues are still required for the next step for the proof of concept of Raman spectroscopy for treatment response monitoring.
Samples for oral disease detection
Oral clinical measurements of Raman spectroscopy can be performed in biofluids, exfoliated cell samples, and ex vivo and in vivo tissues.
Biofluid analysis has the potential to enable low-cost and distance diagnosis,49 but its implementation in the clinic is still challenging and may take a longer time than other approaches. Its implementation requires standardizing operational (e.g. when the sample is analysed in the clinic workflow), sample collection (e.g. fasting or not fasting), and sample handling (e.g. sample volume, dilutions, and storage) procedures. Taking into account biological variability and patient behaviour aspects (e.g. smoking and hydration) is necessary once the first standardization takes place. Successful studies of biofluids for oral applications were mostly performed in blood69,70 (especially serum,67,71–74 saliva,75–78 and urine).79 However, even though previous studies report the proof of concept studies, further investigation is essential to move forward to large-scale clinical settings.
Analysis of exfoliated oral cells has potential to be a screening tool suitable for routine clinical applications. On the other hand, clinical translation is dependent on the same standardization procedures as those discussed for biofluids. In addition, the use of microscopes in the clinic is not common, which means the microscopy market needs further development in order to meet clinicians’ requirements before exfoliated cell applications take place. These applications are based on the assumption that slight biochemical changes in the diseased condition can be identified earlier than morphological alterations. Therefore, the sensitivity provided by Raman spectroscopy could be used to enhance diseased tissue features and diagnose disease at an early stage. As enhanced features are mostly known by molecular biologists and pathologists, supervision of these professionals is critical for the success of multicentre exfoliated cell studies and clinical translation. Research groups have reported advances in spectral differential analysis for normal, dysplastic, and squamous cell carcinoma cells,56 and the feasibility of spectral acquisition and cell collection.80,81
Ex vivo studies are easier and quicker to perform for validation purposes, as clinical research ethics approval takes a shorter time for non-interventional studies. Then, more multicentre studies can be carried out and clinical translation may happen earlier than other applications. Conversely, the ex vivo tissues do not reproduce the same conditions as those of in vivo tissues and detection of slight biochemical changes may be more difficult. This may hinder early-stage disease diagnosis. Also, biological variability and handling and storage differences among health institutions must be taken into consideration when analysing ex vivo tissues spectra. Previously reported studies show results for both frozen and fixed tissues to differentiate normal, inflammatory, precancerous, and cancerous tissues.26,46,58,82–86 Good sensitivity and specificity were reported by Girish et al. for tissue sections on the Ag–TiO2 nanostructured SERS substrate.49,87 Initiatives to optimize the data analysis were taken by our group, which evaluate the classification accuracy by multivariate analysis methods.6,7
In vivo studies represent the most advantageous approach for clinical translation. By assessing the variation of the biochemical content in early stage diseases in vivo, slight differences can be observed directly in the patient. Therefore, applications in the clinical routine are more realistic and translation is more feasible. Previous studies report efforts to decrease the time of spectral acquisition,47,88 age-related and tobacco-related86 or oral anatomical subsite variations,89–91 and the feasibility of detecting changes associated with cancer, precancer, and tissue malignancy.92–97 The next step requires real-time analysis improvements in the spectra collection, signal and data processing, and data analysis. These improvements can enhance the data quality, automated system responses, and the generation of user-friendly reports.
Interference due to optical processes and implications in signal processing
Currently, there is no technique capable of measuring only Raman scattered photons in biological tissues. In Raman spectroscopy, several optical processes contribute to the captured signal such as fluorescence, Mie, and Rayleigh scattering. Mie scattering and Rayleigh scattering can lead to biased changes in the captured signal, since higher energy photons scatter more than lower energy ones, e.g., blue light scatters more than red light. This affects both incident and collected light, thus making the efficiency of the collected signal depend on the probe geometry (such as the positions of the light source and the detector and the area of the detector) and the measured tissue profile. Tissue structures such as striations in collagen fibres and mitochondria membranes increase Rayleigh scattering processes, while organelles and cell surfaces contribute to Mie scattering. This happens because Rayleigh scattering and Mie scattering are generated by refractive index mismatches in structures smaller or larger than the light wavelength. On the other hand, fluorescence occurs due to light absorption and subsequent emission of lower energy light by specific biomolecules, as molecules lose energy through vibration before emission. The observed effect in Raman spectra is a broad spectral background present only in biological tissue measurements.
Scattering and fluorescence processes happen together in biological tissues, which hinders extraction of pure Raman intensity spectra out of intensity measurements. In order to perform this extraction, the research community has used a number of background subtraction algorithms98,99 based on scattering correction and polynomial subtraction. However, subtraction of arbitrary baselines will not recover the ideal spectrum constituted only by the intensity of Raman photons, since the minimum of the real Raman spectrum may be different from the spectrum after the subtraction of a polynomial (arbitrary baseline), for example. Therefore, prior knowledge of the processes that contribute to the signal background is required in order to recover the true Raman spectrum.
In order to take optical processes contributing to the intensity of the underlying background in tissue Raman spectra, it is possible to use multimodal optical spectroscopy. By using reflectance and fluorescence spectroscopy, it is possible to extract the fluorescence, absorption and scattering coefficients of biological tissues. This information can be coupled to Raman systems and taken into account when subtracting the signal background. In this case, clinical translation would occur only if processing algorithms for measurements with the three optical techniques could be implemented together to give a real-time analysis of the captured Raman signal. This implementation requires computer science experts in programming, integration, and automation. Another requirement for heterogeneous tissues is collecting the three types of spectra from the same spot, which may be challenging from the instrumentation aspect, as it involves integrating the three techniques into the same measurement probe. The same applies to the analysis of ex vivo samples in a substrate, since the substrate spectrum should be removed by digital dewaxing.100
Instrumentation standards and limitations
Conventional instrumentation for clinical Raman spectroscopy comprises a laser, fibre optic probe, spectrograph, and detector (CCD or spectrometer). Lasers are used to produce light in a specific, stable, and narrow wavelength band, which allows the Raman shifts to be calculated using the spectral distance from the excitation (Rayleigh scattered) band. They produce enough power to generate a significantly high Raman signal, but are limited by the intensity that leads to heating effects that may damage biological tissues. This may lead to time-consuming measurements due to the low probability of producing Raman photons. In addition, the light source wavelength may be absorbed by tissue chromophores (such as oxy and deoxyhaemoglobin, melanin, water, and lipid), making 785 nm the choice of most of the systems for medical applications, as this wavelength is positioned in the “optical window”, the spectral region where biological tissue has the highest light penetration.
Fibre optic probes are generally used for clinical Raman spectroscopy to optimize the light delivery and collection. These probes allow clinicians to access the region of interest anywhere in the patient body and can be designed for specific applications. This design involves the optimum choice of optical fibre material, size, geometry, and surface modifications of the probe. Typically, silica (glass) fibre probes are used thanks to their relatively low cost and ability to be sterilized. However, glass fibres have a strong Raman signal below 700 cm−1,28 which cannot be extracted from the collected measurement signal, in many cases. With regard to the size of the probe, a high collection signal and most of the design configurations are possible for probes with a diameter of the order of centimetres. On the other hand, if millimetre-sized probes are required, the options of the design and intensity of captured signals may drop.28 Major limitations for clinical translations include the high cost of the probes currently used in research (expensive assembly and optical filters) and artefacts of ambient light, which cannot be totally suppressed by the currently used probes. Ambient light is a factor that must be taken into account, since creating a completely dark environment for clinical diagnostics or surgical guidance is not feasible.
Advances in instrumentation
One of the main advances in instrumentation is the design of fibre optic probes for depth selectivity and fast acquisition time.27,28,101–105 Depths from hundreds of micrometres to a few centimetres can be reached by different fibre probe designs and techniques (by changing the shape of the probe tip and the distance between the source and detector fibres) and by the adoption of the spatially offset Raman spectroscopy technique.106–115
Advances in the integration of instrumentation and data analysis reduced the acquisition times to less than one second and still had potential to give a precise diagnosis.25,116–120 These systems are very sophisticated, but the equipment can be bulky and expensive, which hinders prospects for clinical translation. Alternatively, the development of hand-held equipment may diversify the use of expensive instrumentation, since it is more ergonomic and portable.25,119,121 In this case, the instrumentation specifications tend to be high enough to give a precise diagnosis only if it contains expensive components that allow sufficient spectral resolution and acquisition time. For Raman imaging instrumentation, specifications may need to include spatial resolution and field-of-view, which can add even more cost. Ideally, if the instrumentation needs to be used in a clinical routine, alternatives must be sought to allow clinics/hospitals to afford the equipment. Another relevant advance is fabricating low-cost disposable probes. Potentially cheap probes have been proposed by two research groups.122,123 One of them used a disposable needle tip with fused silica fibers connected to a reusable probe with expensive components, while the other could be produced without expensive optical filters.28
Spectral pre-processing techniques
In the Raman spectroscopy context, data pre-processing consists in preparing the data to be analysed after spectra collection. It involves background subtraction for obtaining the desired Raman scattered signal, noise suppression, and enhancement of spectral differences based on the shape, absolute intensity, and spectral position of Raman bands.
Appropriate background subtraction must be performed in order to recover the true Raman spectrum, i.e., the Raman spectrum without the interference of optical processes other than Raman scattering. Background subtraction includes techniques to eliminate the influence of fluorescence and other types of scattering processes, as mentioned in previous sections. One of the few techniques developed to remove the influence of scattering on Raman spectra is called extended multiplicative scatter correction,124 which was initially designed to analyse infrared spectra of powder mixtures. On the other hand, a wide range of methods are used for removing the influence of fluorescence. These methods include wavelet transformation, principal component analysis (PCA), penalized least squares, multistage smoothing, first- and second-order differentiation, polynomial subtraction, and frequency filtering27,125–132. The latter two approaches are the most used ones. Polynomial subtraction consists in fitting a polynomial of sufficiently high order to describe the smooth fluorescence contribution without eliminating high frequency Raman signals and subsequent subtraction of this polynomial. Frequency-domain filtering consists in eliminating low frequency contributions in the signal after applying a fast Fourier transform. More advanced methods include principal component analysis, which removes the components of high spectral variance by assuming that they are associated with the fluorescence background, and wavelet transformation, which relies on the shape of the fluorescence spectrum underlying the Raman signal and on the choice of the decomposition method.27,132–134
Noise suppression is mandatory in Raman spectra, as the real Raman contribution is weak and most of the time hidden in a highly noisy signal. This suppression is especially useful for posterior biochemical characterization based on fitting of the Raman peaks, as it removes high-frequency features that might hinder the right determination of the peak position. It can be performed by applying filters such as Savitzky–Golay, Gaussian, median, and moving average window filters. The order of Savitzky–Golay filters is highly dependent on the system specifications (such as spectral resolution and efficiency of light collection) and measurement conditions (such as short integration time or dark current in the detector) that contribute to the signal noise. Gaussian filters rely on the system spectral resolution and usually have the full width at half maximum set at half this resolution.135–139 Advanced methods to supress high signal frequency components can use multivariate statistics or analysis such as PCA and genetic algorithms.139–143 Even though these methods remove noise extremely efficiently, they must be used carefully to not eliminate features of the true Raman spectrum, which has a significantly higher frequency than the background, but lower frequency than the random signal noise. An alternative to estimate the measurement noise is by probing the different sources of noise in the used instrumentation. These sources may involve all the equipment components, thus making calibration essential to check for reliable noise suppression. In this calibration, a standard substance with a known Raman spectrum should be measured to characterize the system response and whether the processed signal matches with the known (calibrated) Raman spectrum should be checked.
Enhancement of the spectral differences is highly used for tissue classification purposes after spectral pre-processing. It is typically performed by using difference spectra (subtraction from average spectra of the dataset) and normalizing the Raman spectrum in several ways. The most common types of normalization are normalizations to the area under the spectrum, maximum intensity of the spectrum, and mean scaling of the spectrum based on the intensity of the average spectrum in a particular Raman shift for a specific patient. For interpretation purposes, the first type assumes that the same number of photons will be detected in each measurement, including the whole spectrum. On the other hand, the second and third types rely on a constant intensity of the strongest emission of the sample and on a constant emission in a certain wavelength for all the patients.
Opportunities and challenges in data analysis
Analysis for clinical Raman spectroscopy can be divided in three main approaches: spectral feature extraction, tissue classification for direct diagnosis and biochemical characterization for the interpretation of the patient state. Feature extraction is based on the idea of finding new aspects that characterize hidden information or divisions in the patient group under study. As an example, one may be looking for grouping patients with benign or cancerous lesions. However, after analysing how patients are grouped upon a comparison of certain features, one can see that patients could be separated by age instead. This is performed by using unsupervised multivariate analysis methods, i.e., methods to analyse experimental spectra without prior knowledge of the studied subjects. These methods include cluster analysis and component analysis. Cluster analysis is based on grouping sets of subjects. The formed groups can have characteristics assigned to them (such as age, skin colour, and pathology) in order to check whether the groups are compatible with one of these characteristics. If each group obeys a trend in a given characteristic, a potential differentiation of subjects can be performed by using the measurement technique targeted in the study (Raman spectroscopy, in this case). Cluster analysis techniques comprise hierarchical cluster analysis, K-means, fuzzy C means, and Density-Based Spatial Clustering of Applications with Noise.144–153 Component analysis is based on describing the subjects with new features. Examples of component analysis include PCA, linear discriminant analysis, and vertex component analysis. PCA is the most used method. It calculates features based on independent components (sum of weighted signals for each Raman shift) and orders them from the highest to the lowest variance141,143,153–157. This makes it suitable for decreasing the complexity of the spectra, i.e., analysing fewer Raman spectral features. Analysing thousands of values of Raman spectra may be complex and can lead to high computational costs, which means more time is required for data analysis. Therefore, PCA is used to extract combinations of spectral features for quick data analysis while keeping the diagnosis performance.158
Tissue classification is widely used to create models to discriminate/differentiate among several types of biological tissues and predict what the category of a new measured tissue is. It is typically performed by using supervised multivariate analysis methods, i.e., methods that use prior knowledge of the studied subjects to analyse the data (Raman spectra). Classification methods are incredibly powerful and can be used for precise diagnosis of many diseases. However, in order to achieve a reliable tissue classification, three requirements must be met. First, the sample size must be large enough to represent the whole group of studied objects, e.g., oral lesions. When the dataset used to generate the classification model is not sufficiently diverse and large, i.e., if it does not contain the typical heterogeneity of a clinical setting, the model may be too specific for the setting the study was conducted. This was demonstrated by a study that obtained acceptable diagnosis performance by models generated with 2 to 25 subjects and good classification in studies involving 75 to 100 patients.159 Moreover, misclassification can lead to severe consequences for the patients, thus making significant statistical difference a critical factor for diagnosis. Second, the model must be robust enough to achieve the diagnosis performance with every additional input data. In order to do this, the model should have as many internal and external validations as possible. For internal validation, part of the dataset is used as a training set to build the model, while the other part (test set) is used to apply the model and test its accuracy. This can be done repeatedly until all parts of the dataset are used to calibrate the model and test it. The optimum model for each dataset is calculated by using the root-mean squared error of calibration and root-mean-squared error of prediction. Several methods can be employed to estimate this accuracy such as k-fold cross-validation, permutation testing, and leave-one-out.153,160–162 After this, external validation is required for testing the generated model with a second dataset, i.e., a larger dataset of newly measured Raman spectra (independent of the first dataset that was used to generate the model). The external validation is necessary because the first generated model may be biased, since it was optimized to classify data (biological tissues) in the first dataset. Third, the classification must be completely independent of the measurement conditions. This means that a standard procedure must exist in order to make measurements more uniform and systematic across studies, in order to allow comparison among them and ultimately build a general model that is valid for all the sets of instruments of a company, for example. This is important to calibrate Raman spectroscopy systems over time, as they can lose their diagnostic performance and may need maintenance. Tissue classification methods include support vector machines, linear discriminant analysis, quadratic discriminant analysis, partial least squares, logistic regression models, neural networks, decision trees, genetic algorithms, and general optimization techniques.27,141,143,163–170
Finally, biochemical characterization is attractive for estimating biomolecule concentrations in tissues, which can provide metabolic information that may be useful in clinical monitoring cases, where many possibilities can exist such as processes of tissue healing and mineralization, treatment prognosis, and progress of bacterial or fungal infection. In these cases, classifying tissues may be hard due to many changes that can occur, which hinders the differentiation among stages. In this case, biochemical information may be useful for the clinicians to understand the patient situation by taking into consideration both this information and the patient history. Biochemical characterization is usually performed by deconvolution of the Raman spectra peaks into combinations of Gaussian curves. Each curve will be centred in the Raman shift corresponding to the described vibrational mode and the abundance of certain biomolecules can be calculated by the area of the Gaussian curve, which indicates the intensity of the deconvolved peak.
Perspectives of clinical translation
Our team frequently makes clinical diagnosis in our units, and deals with histopathological results regarding biopsies previously performed in our offices. We take a recent clinical case as an example to illustrate some possible applications of the technique and in what direction we can proceed.
In Fig. 1, we show a clear example of a patient in whom optical biopsy procedures may assist both diagnosis and treatment. The patient has two distinct lesions, one in the tongue and one in the lip. The hypothetic clinical diagnosis of these lesions is leukoplakia, which is considered a potentially cancerous lesion. This makes early-stage diagnosis highly recommended.
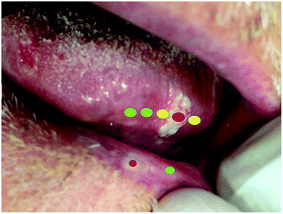 |
| Fig. 1 Early oral squamous cell carcinoma of the tongue. Leukoplaquia in the lip. Using the dots we would like to point out some Raman approaches. The spectrum itself could be translated into colours, as described in the text. | |
Let us consider the lesion of the tongue (Fig. 1), which presented a nodule at the time of diagnosis. This nodule can consequently generate greater severity and is evidence that the lesion can progress towards an epidermoid carcinoma, as was confirmed in the real case afterwards. In this context, traditional incisional biopsy is the chosen procedure for the final diagnosis, but a portable Raman spectroscopy device could help clinicians identify features of each area, as shown in Fig. 1. A “green” spectrum would represent a normal area, a “yellow” spectrum would represent an area doubtful, and a “red” spectrum would represent a potentially malignant/disease area.
The problem addressed above refers to the use of the technique as a diagnostic method. Once the oral lesion is diagnosed as cancer, the procedure that must be performed is the surgical removal with an associated safety margin or not to radiotherapy. Then, we can use again the Raman spectroscopy technique for the surgical procedure to guide us in the healthy or pathological safety margin, thus helping the clinician in his/her limitations (e.g. evaluations using the naked eye) and providing a database for the analysis of oral tissues at the molecular level for fundamental applications.
Researchers have engaged in creating new groups and networks to accelerate the clinical implementation of vibrational spectroscopy techniques. Some of these groups include Raman4clinics and Clinical Infrared and Raman Spectroscopy Network (CLIRSPEC), in which some events are organized to stimulate the international and interdisciplinary community to collaborate and narrow discussions about challenges in the clinical translation of Raman spectroscopy.
What is next?
In terms of instrumentation, Raman spectroscopy systems should include six main features: portability, low cost, user-friendly interface, capability of providing accurate diagnosis, fast data acquisition and analysis, and being automated. Portability and low-cost development rely mostly on instrumentation. User-friendly interface and accurate diagnosis require both instrumentation and software aspects. Building an intuitive interface for clinicians involves the implementation of instrumentation features and software guidance based on a deep understanding of clinicians’ background and work environment. Improvements in instrumentation include buttons at the probe or foot pedals for taking measurements, while software advances may include the possibility of modes for real-time signal update and for recording precise spectra (acquisition mode), real-time display of (easy interpretable) clinical data reports, and intuitive interface for changing equipment configurations. Automation and fast data acquisition and analysis are very dependent on software development. A big step in automation is integrating the data acquisition, data processing, and data analysis software, which should process all the information in real time, including background removal, spectral smoothing, normalization, feature extraction, and classification. For fast data acquisition and analysis (Fig. 2), optimizing both steps with regard to computational costs is essential to provide real-time data updates and generation of intuitive reports.
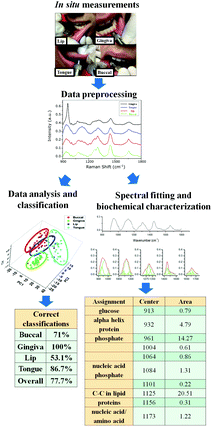 |
| Fig. 2 Workflow of the in vivo Raman system showing our previous healthy oral subsite in vivo measurements. It is important to note that the complete data analysis and report generation should be in “one click”. The report generation should display user-friendly data, i.e., data that is easy to interpret and pertinent for each individual application. | |
Finally, with regard to the use of Raman spectroscopy as a daily clinical practice, obviously more studies and in vivo tests should be performed, but always with a focus that should have a real application; it was our objective in the present work to show the community of spectroscopists what is really necessary, starting from a clinical point of view. Thus, we must expand our studies to: 1. precise identification of potentially cancerous lesions (leukoplakia, erythroplakia, submucous fibrosis and actinic cheilitis), 2. seeking subtle changes in the healthy mucosa of patients at risk (e.g. smokers or those exposed to high levels of solar radiation), changes which we were not able to evidence in routine clinical examination, 3. evaluation of infiltration or margin of lesions of squamous cell carcinoma, or even early stage epidermoid carcinoma, 4. development of a robust in vivo spectroscopy technique to identify other pathological complications that require early diagnosis (including cancer or not), such as autoimmune diseases, and some fungal infections, among others.
Conflicts of interest
There are no conflicts to declare.
Acknowledgements
Luis Felipe CS Carvalho was funded by the Fundação de Amparo à Pesquisa do Estado de São Paulo (FAPESP – 2014/05978-1 and 2018/03636-7), he also was supported by CAPES PNPD – Odontologia UNITAU, Centro Universitário Braz Cubas by the Scientific Initiation Program and CLIRSPEC for SPEC 2018.
Notes and references
- WHO|Oral health, http://www.who.int/oral_health/publications/factsheet/en/ (accessed 19 July 2018).
- WHO|Oral health databases, http://www.who.int/oral_health/databases/en/ (accessed 19 July 2018).
- WHO|Strategies for oral disease prevention and health promotion, http://www.who.int/oral_health/strategies/en/ (accessed 19 July 2018).
- Oral Health Country/Area Profile Project, https://www.mah.se/capp/ (accessed 19 July 2018).
- WHO|11th IADR World Congress on Preventive Dentistry, http://www.who.int/oral_health/events/congress-preventive-dentistry-oct2017-outcomes/en/ (accessed 19 July 2018).
- L. F. C. S. Carvalho, M. S. Nogueira, L. P. M. Neto, T. T. Bhattacharjee and A. A. Martin, Biomed. Opt. Express, 2017, 8, 5218 CrossRef CAS PubMed.
- L. F. C. S. Carvalho, M. S. Nogueira, L. P. M. Neto, T. T. Bhattacharjee and A. A. Martin, Biomed. Opt. Express, 2018, 9, 649 CrossRef PubMed.
- A. Cosci, M. S. Nogueira, S. Pratavieira, A. Takahama, R. de S. Azevedo, C. Kurachi and C. Kurachi, Biomed. Opt. Express, 2016, 7, 4210–4219 CrossRef PubMed.
- A. Cosci, M. S. Nogueira, S. Pratavieira, A. Takahama, R. de S. Azevedo and C. Kurachi, Biomed. Opt. Express, 2016, 7, 4210–4219 CrossRef PubMed.
- L. Pires, M. S. Nogueira, S. Pratavieira, L. T. Moriyama and C. Kurachi, Biomed. Opt. Express, 2014, 5, 3080 CrossRef PubMed.
- A. P. da Silva, M. Saito Nogueira, J. A. Jo, V. Salvador Bagnato and N. Mayumi Inada, Biomed. Opt., 2016, 2016, JTu3A.37 Search PubMed.
- M. Saito Nogueira, A. Cosci, S. Pratavieira, A. Takahama, R. Souza Azevedo and C. Kurachi, Proc. SPIE, 2016, 97031U Search PubMed.
-
M. S. Nogueira, Fluorescence lifetime spectroscopy for diagnosis of clinically similar skin lesions, Master dissertation hosted in Universidade de São Paulo library, Universidade de São Paulo, 2016.
- M. Saito Nogueira and C. Kurachi, Proc. SPIE, 2016, 97031W Search PubMed.
- C. de Paula Campos, C. de Paula D'Almeida, M. S. Nogueira, L. T. Moriyama, S. Pratavieira and C. Kurachi, Photodiagn. Photodyn. Ther., 2017, 20, 21–27 CrossRef PubMed.
- C. Teles de Andrade, M. S. Nogueira, S. C. Kanick, K. Marra, J. Gunn, J. Andreozzi, K. S. Samkoe, C. Kurachi and B. W. Pogue, Proc. SPIE, 2016, 969410, 969410 Search PubMed.
-
M. Saito Nogueira, A. Cosci and C. Kurachi, Biophotonics Photonic Solut. Better Heal. Care VI, 2018, p. 144.
-
B. A. Ono, M. S. Nogueira, L. Pires, S. Pratavieira and C. Kurachi, Opt. Methods Tumor Treat. Detect. Mech. Tech. Photodyn. Ther. XXVII, 2018, vol. 1047616, p. 44.
- M. Saito Nogueira, A. Cosci, R. G. Teixeira Rosa, A. G. Salvio, S. Pratavieira and C. Kurachi, J. Biomed. Opt., 2017, 22, 1 Search PubMed.
-
M. Saito Nogueira, M. Raju, J. Gunther, K. Grygoryev, H. Lu, K. Komolibus and S. Andersson-Engels, Biophotonics Photonic Solut. Better Heal. Care VI, 2018, p. 125.
- M. Saito Nogueira, R. G. Texeira Rosa, S. S. Pratavieira, C. P. D'Almeida and C. Kurachi, Biophotonics, 2015, 9531, 95313D Search PubMed.
-
C. P. D'Almeida, C. Campos, M. S. Nogueira and C. Kurachi, Proc. SPIE 9531, Biophotonics South Am. 953146 (June 19, 2015), 2015, vol. 9531, pp. 1–7.
- C. Kurachi, L. Pires, M. S. Nogueira and S. Pratavieira, Biomed. Opt., 2014, 2014, BS4B.3 Search PubMed.
- L. F. C. S. Carvalho, F. Bonnier, K. O'Callaghan, J. O'Sullivan, S. Flint, H. J. Byrne and F. M. Lyng, Exp. Mol. Pathol., 2015, 98, 502–509 CrossRef CAS PubMed.
- M. Jermyn, J. Desroches, K. Aubertin, K. St-Arnaud, W. J. Madore, E. De Montigny, M. C. Guiot, D. Trudel, B. C. Wilson, K. Petrecca and F. Leblond, Phys. Med. Biol., 2016, 61, R370–R400 CrossRef CAS PubMed.
- R. Malini, K. Venkatakrishna, J. Kurien, K. M. Pai, L. Rao, V. B. Kartha and C. M. Krishna, Biopolymers, 2006, 81, 179–193 CrossRef CAS PubMed.
- I. Pence and A. Mahadevan-Jansen, Chem. Soc. Rev., 2016, 45, 1958–1979 RSC.
- O. Stevens, I. E. Iping Petterson, J. C. C. Day and N. Stone, Chem. Soc. Rev., 2016, 45, 1919–1934 RSC.
- J. W. Chan, D. S. Taylor, T. Zwerdling, S. M. Lane, K. Ihara and T. Huser, Biophys. J., 2006, 90, 648–656 CrossRef CAS PubMed.
- J. W. Chan, D. S. Taylor, S. M. Lane, T. Zwerdling, J. Tuscano and T. Huser, Anal. Chem., 2008, 80, 2180–2187 CrossRef CAS PubMed.
- X. Nan, E. O. Potma and X. S. Xie, Biophys. J., 2006, 91, 728–735 CrossRef CAS PubMed.
- X. Nan, A. M. Tonary, A. Stolow, X. S. Xie and J. P. Pezacki, ChemBioChem, 2006, 7, 1895–1897 CrossRef CAS PubMed.
- H. A. Rinia, K. N. J. Burger, M. Bonn and M. Müller, Biophys. J., 2008, 95, 4908–4914 CrossRef CAS PubMed.
- J. Kneipp, H. Kneipp, B. Wittig and K. Kneipp, Nano Lett., 2007, 7, 2819–2823 CrossRef CAS PubMed.
- A. Ingram, R. J. Stokes, J. Redden, K. Gibson, B. Moore, K. Faulds and D. Graham, Anal. Chem., 2007, 79, 8578–8583 CrossRef CAS PubMed.
- S. W. Bishnoi, C. J. Rozell, C. S. Levin, M. K. Gheith, B. R. Johnson, D. H. Johnson and N. J. Halas, Nano Lett., 2006, 6, 1687–1692 CrossRef CAS PubMed.
- R. J. Dijkstra, W. J. J. M. Scheenen, N. Dam, E. W. Roubos and J. J. ter Meulen, J. Neurosci. Methods, 2007, 159, 43–50 CrossRef CAS PubMed.
- Z. Wang, A. Bonoiu, M. Samoc, Y. Cui and P. N. Prasad, Biosens. Bioelectron., 2008, 23, 886–891 CrossRef CAS PubMed.
- B. Lutz, C. Dentinger, L. Sun, L. Nguyen, J. Zhang, A. J. Chmura, A. Allen, S. Chan and B. Knudsen, J. Histochem. Cytochem., 2008, 56, 371–379 CrossRef CAS PubMed.
- M. V. P. Chowdary, K. K. Kumar, J. Kurien, S. Mathew and C. M. Krishna, Biopolymers, 2006, 83, 556–569 CrossRef CAS PubMed.
- K. Das, N. Stone, C. Kendall, C. Fowler and J. Christie-Brown, Lasers Med. Sci., 2006, 21, 192–197 CrossRef PubMed.
- B. W. D. De Jong, T. C. B. Schut, K. Maquelin, T. Van Der Kwast, C. H. Bangma, D. J. Kok and G. J. Puppels, Anal. Chem., 2006, 78, 7761–7769 CrossRef CAS PubMed.
- C. L. Evans, X. Y. Xu, S. Kesari, X. S. Xie, S. T. C. Wong and G. S. Young, Opt. Express, 2007, 15, 12076–12087 CrossRef CAS PubMed.
- H. W. Wang, T. T. Le and J. X. Cheng, Opt. Commun., 2008, 281, 1813–1822 CrossRef CAS PubMed.
- Y. Fu, H. Wang, T. B. Huff, R. Shi and J.-X. Cheng, J. Neurosci. Res., 2007, 85, 2870–2881 CrossRef CAS PubMed.
- K. Venkatakrishna, J. Kurien, K. M. Pai, M. Valiathan, N. N. Kumar, C. M. Krishna, G. Ullas and V. B. Kartha, Curr. Sci., 2001, 80, 665–669 CAS.
- S. P. Singh, A. Deshmukh, P. Chaturvedi and C. M. Krishna, J. Cancer Res. Ther., 2012, 8(Suppl. 1), S126–S132 CAS.
- T. Upile, W. Jerjes, H. J. C. M. Sterenborg, A. K. El-Naggar, A. Sandison, M. J. H. Witjes, M. A. Biel, I. Bigio, B. J. F. Wong, A. Gillenwater, A. J. MacRobert, D. J. Robinson, C. S. Betz, H. Stepp, L. Bolotine, G. McKenzie, C. A. Mosse, H. Barr, Z. Chen, K. Berg, A. K. D'Cruz, N. Stone, C. Kendall, S. Fisher, A. Leunig, M. Olivo, R. Richards-Kortum, K. C. Soo, V. Bagnato, L. P. Choo-Smith, K. Svanberg, I. B. Tan, B. C. Wilson, H. Wolfsen, A. G. Yodh and C. Hopper, Head Neck Oncol., 2009, 1, 25 CrossRef PubMed.
- A. Sahu and C. M. Krishna, J. Cancer Res. Ther., 2017, 13, 908–915 Search PubMed.
- A. Sahu, A. Deshmukh, A. R. Hole, P. Chaturvedi and C. M. M. Krishna, J. Innovative Opt. Health Sci., 2016, 9, 1650017 CrossRef.
- A. Nijssen, S. Koljenovic, T. C. Bakker Schut, P. J. Caspers and G. J. Puppels, J. Biophotonics, 2009, 2, 29–36 CrossRef CAS PubMed.
- E. M. Barroso, R. W. H. Smits, T. C. B. Schut, I. Ten Hove, J. A. Hardillo, E. B. Wolvius, R. J. Baatenburg De Jong, S. Koljenović and G. J. Puppels, Anal. Chem., 2015, 87, 2419–2426 CrossRef CAS PubMed.
- M. A. Liebert, A. P. Oliveira, M. Sc, R. A. Bitar, M. Sc, L. Silveira, J. R. D. Ph, R. A. Zângaro, D. Ph, A. A. Martin and D. Ph, Photomed. Laser Surg., 2006, 24, 348–353 CrossRef PubMed.
- R. Valdés, S. Stefanov, S. Chiussi, M. Lõpez-Alvarez and P. González, J. Raman Spectrosc., 2014, 45, 550–557 CrossRef.
- S. P. Singh, H. Alam, C. Dmello, H. Mamgain, M. M. Vaidya, R. R. Dasari and C. M. Krishna, J. Biophotonics, 2017, 10, 1377–1384 CrossRef CAS PubMed.
- L.
F. C. S. Carvalho, F. Bonnier, C. Tellez, L. dos Santos, K. O'Callaghan, J. O'Sullivan, L. E. S. Soares, S. Flint, A. A. Martin, F. M. Lyng and H. J. Byrne, Exp. Mol. Pathol., 2017, 103, 255–262 CrossRef CAS PubMed.
- L. Su, Y. F. Sun, Y. Chen, P. Chen, A. G. Shen, X. H. Wang, J. Jia, Y. F. Zhao, X. D. Zhou and J. M. Hu, Laser Phys., 2012, 22, 311–316 CrossRef CAS.
- C. M. Krishna, G. D. Sockalingum, J. Kurien, L. Rao, L. Venteo, M. Pluot, M. Manfait and V. B. Kartha, Appl. Spectrosc., 2004, 58, 1128–1135 CrossRef CAS PubMed.
- F. L. J. Cals, T. C. Bakker Schut, S. Koljenovi
, G. J. Puppels and R. J. B. De Jong, J. Raman Spectrosc., 2013, 44, 963–972 CrossRef CAS.
- A. Daniel, A. Prakasarao, B. David, L. Joseph, C. Murali Krishna, K. D and S. Ganesan, J. Raman Spectrosc., 2014, 45, 541–549 CrossRef.
- E. M. Barroso, R. W. H. Smits, C. G. F. Van Lanschot, P. J. Caspers, I. Ten Hove, H. Mast, A. Sewnaik, J. A. Hardillo, C. A. Meeuwis, R. Verdijk, V. N. Hegt, R. J. Baatenburg De Jong, E. B. Wolvius, T. C. Bakker Schut, S. Koljenović and G. J. Puppels, Cancer Res., 2016, 76, 5945–5953 CrossRef CAS PubMed.
- F. L. J. Cals, S. Koljenović, J. A. Hardillo, R. J. Baatenburg de Jong, T. C. Bakker Schut and G. J. Puppels, Oral Oncol., 2016, 60, 41–47 CrossRef PubMed.
- I. Behl, L. Kukreja, A. Deshmukh, S. P. Singh, H. Mamgain, A. R. Hole and C. M. Krishna, J. Biomed. Opt., 2014, 19, 126005 CrossRef PubMed.
- F. L. J. Cals, T. C. Bakker Schut, J. A. Hardillo, R. J. Baatenburg De Jong, S. Koljenović and G. J. Puppels, Lab. Invest., 2015, 95, 1186–1196 CrossRef CAS PubMed.
- E. M. Barroso, I. ten Hove, T. C. Bakker Schut, H. Mast, C. G. F. van Lanschot, R. W. H. Smits, P. J. Caspers, R. Verdijk, V. Noordhoek Hegt, R. J. Baatenburg de Jong, E. B. Wolvius, G. J. Puppels and S. Koljenović, Eur. J. Cancer, 2018, 92, 77–87 CrossRef CAS PubMed.
- M. Yasser, R. Shaikh, M. K. Chilakapati and T. Teni, PLoS One, 2014, 9, 5 CrossRef PubMed.
- A. Sahu, N. Nandakumar, S. Sawant and C. M. Krishna, Analyst, 2015, 140, 2294–2301 RSC.
- A. Malik, A. Sahu, S. P. Singh, A. Deshmukh, P. Chaturvedi, D. Nair, S. Nair and C. Murali Krishna, Head Neck, 2017, 39, 2216–2223 CrossRef PubMed.
- A. T. Harris, A. Lungari, C. J. Needham, S. L. Smith, M. A. Lones, S. E. Fisher, X. B. Yang, N. Cooper, J. Kirkham, D. A. Smith, D. P. Martin-Hirsch and A. S. High, Head Neck Oncol., 2009, 1, 34 CrossRef PubMed.
-
P. Rekha, P. Aruna, A. Daniel, S. W. Prasanna, K. Udayakumar, S. Ganesan, G. Bharanidharan and B. David, Photonics (ICP), 2013 IEEE 4th Int. Conf., 2013, pp. 135–137.
- A. Sahu, S. Sawant, H. Mamgain and C. M. Krishna, Analyst, 2013, 138, 4161–4174 RSC.
- A. Sahu, S. Sawant, S. Talathi-Desai and C. Murali Krishna, Biomed. Spectrosc. Imaging, 2015, 4, 171–187 Search PubMed.
- A. K. Sahu, S. Dhoot, A. Singh, S. S. Sawant, N. Nandakumar, S. Talathi-Desai, M. Garud, S. Pagare, S. Srivastava, S. Nair, P. Chaturvedi and C. Murali Krishna, J. Biomed. Opt., 2015, 20, 115006 CrossRef PubMed.
- Y. Tan, B. Yan, L. Xue, Y. Li, X. Luo and P. Ji, Lipids Health Dis., 2017, 16, 1–9 CrossRef PubMed.
- J. Chen, Y. Kah, C. Guat and L. Lee, Int. J. Nanomed., 2007, 2, 785–798 Search PubMed.
- K. Davies, J. M. Connolly, P. Dockery, A. M. Wheatley, M. Olivo and I. Keogh, Surgeon, 2015, 13, 321–329 CrossRef CAS PubMed.
- L. R. Bigler, C. F. Streckfus and W. P. Dubinsky, Clin. Lab. Med., 2009, 29, 71–85 CrossRef PubMed.
- J. M. Connolly, K. Davies, A. Kazakeviciute, A. M. Wheatley, P. Dockery, I. Keogh and M. Olivo, Nanomedicine, 2016, 12, 1593–1601 CrossRef CAS PubMed.
- B. Elumalai, A. Prakasarao, B. Ganesan, K. Dornadula and S. Ganesan, J. Raman Spectrosc., 2014, 46, 84–93 CrossRef.
- A. Sahu, N. Shah, M. Mahimkar, M. Garud, S. Pagare, S. Nair and C. M. Krishna, Proc. SPIE, 2014, 8926, 89262N CrossRef.
- A. Sahu, S. Tawde, V. Pai, P. Gera, P. Chaturvedi, S. Nair and C. M. Krishna, Anal. Methods, 2015, 7, 7548–7559 RSC.
- C. Knipfer, J. Motz, W. Adler, K. Brunner, M. T. Gebrekidan, R. Hankel, A. Agaimy, S. Will, A. Braeuer, F. W. Neukam and F. Stelzle, Biomed. Opt. Express, 2014, 5, 3252 CrossRef PubMed.
- P.-H. Chen, R. Shimada, S. Yabumoto, H. Okajima, M. Ando, C.-T. Chang, L.-T. Lee, Y.-K. Wong, A. Chiou and H. Hamaguchi, Sci. Rep., 2016, 6, 20097 CrossRef CAS PubMed.
- N. S. Sunder, N. N. Rao, V. B. Kartha, G. Ullas and J. Kurien, J. Orofacial Sci., 2011, 3, 15–19 Search PubMed.
-
Y. Hu, T. Jiang and Z. Zhao, 2008 First Int. Conf. Intell. Networks Intell. Syst., 2008, pp. 633–636.
- A. Deshmukh, S. P. Singh, C. M. Krishna and P. Chaturvedi, J. Biomed. Opt., 2011, 16, 127004 CrossRef PubMed.
- C. M. Girish, S. Iyer, K. Thankappan, V. V. D. Rani, G. S. Gowd, D. Menon, S. Nair and M. Koyakutty, J. Mater. Chem. B, 2014, 2, 989–998 RSC.
- S. P. Singh, A. Deshmukh, P. Chaturvedi and C. M. Krishna, Proc. SPIE, 2012, 82190K CrossRef.
- K. Guze, M. Short, S. Sonis, N. Karimbux, J. Chan and H. Zeng, J. Biomed. Opt., 2009, 14, 14016 CrossRef PubMed.
- M. S. Bergholt, W. Zheng, K. Lin, K. Y. Ho, M. Teh, K. G. Yeoh, J. B. Y. So and Z. Huang, J. Biomed. Opt., 2011, 16, 37003 CrossRef PubMed.
- H. Krishna, S. K. Majumder, P. Chaturvedi and P. K. Gupta, Biomed. Spectrosc. Imaging, 2013, 2, 199–217 CAS.
- S. P. Singh, A. Deshmukh, P. Chaturvedi and C. Murali Krishna, J. Biomed. Opt., 2012, 17, 1050021 CrossRef PubMed.
- A. Sahu, A. Deshmukh, A. D. Ghanate, S. P. Singh, P. Chaturvedi and C. M. Krishna, Technol. Cancer Res. Treat., 2012, 11, 529–541 CrossRef CAS PubMed.
- H. Krishna, S. K. Majumder, P. Chaturvedi, M. Sidramesh and P. K. Gupta, J. Biophotonics, 2014, 7, 690–702 CrossRef CAS PubMed.
- S. P. Singh, A. Sahu, A. Deshmukh, P. Chaturvedi and C. M. Krishna, Analyst, 2013, 138, 4175–4182 RSC.
- K. Guze, H. C. Pawluk, M. Short, H. Zeng, J. Lorch, C. Norris and S. Sonis, Head Neck, 2015, 37, 511–517 CrossRef PubMed.
- T. C. Bakker Schut, M. J. H. Witjes, H. J. C. M. Sterenborg, O. C. Speelman, J. L. N. Roodenburg, E. T. Marple, H. A. Bruining and G. J. Puppels, Anal. Chem., 2000, 72, 6010–6018 CrossRef CAS PubMed.
- J. T. Bulmer, D. E. Irish, F. W. Grossman, G. Herriot, M. Tseng and A. J. Weerheim, Appl. Spectrosc., 1975, 29, 506–511 CrossRef CAS.
- S. M. Haight and D. T. Schwartz, Appl. Spectrosc., 1997, 51, 930–938 CrossRef.
- A. Tfayli, C. Gobinet, V. Vrabie, R. Huez, M. Manfait and O. Piot, Appl. Spectrosc., 2009, 63, 564–570 CrossRef CAS PubMed.
- S. D. Schwab and R. L. McCreery, Anal. Chem., 1984, 56, 2199–2204 CrossRef CAS.
- J. T. Motz, S. J. Gandhi, O. R. Scepanovic, A. S. Haka, J. R. Kramer, R. R. Dasari and M. S. Feld, J. Biomed. Opt., 2005, 10, 31113 CrossRef PubMed.
- A. Mahadevan-Jansen, M. F. Mitchell, N. Ramanujam, U. Utzinger and R. Richards-Kortum, Photochem. Photobiol., 1998, 68, 427–431 CrossRef CAS PubMed.
- K. Tanaka, M. T. Pacheco, J. F. Brennan Iii, I. Itzkan, A. J. Berger, R. R. Dasari and M. S. Feld, Appl. Opt., 1996, 35, 758–763 CrossRef CAS PubMed.
-
C. Krafft, S. Dochow, I. Latka, M. Becker, R. Spittel, J. Kobelke, K. Schuster, M. Rothardt and J. Popp, Multi-core fiber with integrated fiber Bragg grating for background free Raman sensing, 2013, vol. 20, p. 85770J Search PubMed.
- K. Buckley and P. Matousek, Analyst, 2011, 136, 3039–3050 RSC.
- I. E. Iping Petterson, P. Dvoák, J. B. Buijs, C. Gooijer and F. Ariese, Analyst, 2010, 135, 3255–3259 RSC.
- Y. Hattori, Y. Komachi, T. Asakura, T. Shimosegawa, G. I. Kanai, H. Tashiro and H. Sato, Appl. Spectrosc., 2007, 61, 579–584 CrossRef CAS PubMed.
- P. Matousek and N. Stone, Chem. Soc. Rev., 2016, 45, 1794–1802 RSC.
- J. C. C. Day, R. Bennett, B. Smith, C. Kendall, J. Hutchings, G. M. Meaden, C. Born, S. Yu and N. Stone, Phys. Med. Biol., 2009, 54, 7077–7087 CrossRef CAS PubMed.
- Z. Huang, M. S. Bergholt, W. Zheng, K. Lin, K. Y. Ho, M. Teh and K. G. Yeoh, J. Biomed. Opt., 2010, 15, 37017 CrossRef PubMed.
- P. Matousek, M. D. Morris, N. Everall, I. P. Clark, M. Towrie, E. Draper, A. Goodship and A. W. Parker, Appl. Spectrosc., 2005, 59, 1485–1492 CrossRef CAS PubMed.
- F. Jaillon, W. Zheng and Z. Huang, Phys. Med. Biol., 2008, 53, 937–951 CrossRef PubMed.
- P. Matousek and N. Stone, J. Biophotonics, 2013, 6, 7–19 CrossRef CAS PubMed.
- Y. Komachi, T. Katagiri, H. Sato and H. Tashiro, Appl. Opt., 2009, 48, 1683–1696 CrossRef CAS PubMed.
- M. D. Keller, E. Vargis, N. de Matos Granja, R. H. Wilson, M.-A. Mycek, M. C. Kelley and A. Mahadevan-Jansen, J. Biomed. Opt., 2011, 16, 77006 CrossRef PubMed.
- M. Ji, D. A. Orringer, C. W. Freudiger, S. Ramkissoon, X. Liu, D. Lau, A. J. Golby, I. Norton, M. Hayashi, N. Y. Agar, G. S. Young, C. Spino, S. Santagata, S. Camelo-Piragua, K. L. Ligon, O. Sagher and X. S. Xie, Sci. Transl. Med., 2013, 5, 201ra119 Search PubMed.
- B. G. Saar, C. W. Freudiger, J. Reichman, C. M. Stanley, G. R. Holtom and X. S. Xie, Sci., 2010, 330, 1368–1370 CrossRef CAS PubMed.
- M. Jermyn, K. Mok, J. Mercier, J. Desroches, J. Pichette, K. Saint-arnaud, L. Bernstein, M. Guiot, K. Petrecca and F. Leblond, Sci. Transl. Med., 2015, 7, 274 Search PubMed.
- C.-S. Liao, P. Wang, P. Wang, J. Li, H. J. Lee, G. Eakins and J.-X. Cheng, Sci. Adv., 2015, 1, e1500738 CrossRef PubMed.
- C. W. Freudiger, W. Yang, G. R. Holtom, N. Peyghambarian, X. S. Xie and K. Q. Kieu, Nat. Photonics, 2014, 8, 153–159 CrossRef CAS PubMed.
- J. C. C. Day and N. Stone, Appl. Spectrosc., 2013, 67, 349–354 CrossRef CAS PubMed.
- S. Dochow, I. Latka, M. Becker, R. Spittel, J. Kobelke, K. Schuster, A. Graf, S. Brückner, S. Unger and M. Rothhardt, others, Opt. Express, 2012, 20, 20156–20169 CrossRef PubMed.
- H. Martens, J. P. Nielsen and S. B. Engelsen, Anal. Chem., 2003, 75, 394–404 CrossRef CAS PubMed.
- C. A. Lieber and A. Mahadevan-Jansen, Appl. Spectrosc., 2003, 57, 1363–1367 CrossRef CAS PubMed.
- P. A. Mosier-Boss, S. H. Lieberman and R. Newbery, Appl. Spectrosc., 1995, 49, 630–638 CrossRef CAS.
- T. J. Vickers, R. E. J. Wambles and C. K. Mann, Appl. Spectrosc., 2001, 55, 389–393 CrossRef CAS.
- K. Chen, H. Zhang, H. Wei and Y. Li, Appl. Opt., 2014, 53, 5559 CrossRef PubMed.
- J. Zhao, H. Lui, D. I. McLean and H. Zeng, Appl. Spectrosc., 2007, 61, 1225–1232 CrossRef CAS PubMed.
- R. P. Van Duyne, D. L. Jeanmaire and D. F. Shriver, Anal. Chem., 1974, 46, 213–222 CrossRef CAS.
- A. O'Grady, A. C. Dennis, D. Denvir, J. J. McGarvey and S. E. J. Bell, Anal. Chem., 2001, 73, 2058–2065 CrossRef.
- V. J. Barclay, R. F. Bonner and I. P. Hamilton, Anal. Chem., 1997, 69, 78–90 CrossRef CAS.
- Z. M. Zhang, S. Chen, Y. Z. Liang, Z. X. Liu, Q. M. Zhang, L. X. Ding, F. Ye and H. Zhou, J. Raman Spectrosc., 2010, 41, 659–669 CrossRef CAS.
- P. J. Cadusch, M. M. Hlaing, S. A. Wade, S. L. McArthur and P. R. Stoddart, J. Raman Spectrosc., 2013, 44, 1587–1595 CrossRef CAS.
- A. Savitzky and M. J. E. Golay, Anal. Chem., 1964, 36, 1627–1639 CrossRef CAS.
- U. R. S. Utzinger, D. L. H. An, A. M. Ahadevan-jansen, A. M. Alpica, M. I. Follen and R. Richards-kortum, Appl. Spectrosc., 2001, 55, 955–959 CrossRef CAS.
- B. M. Bussian and W. Haerdle, Appl. Spectrosc., 1984, 38, 309–313 CrossRef CAS.
- W. F. Edgell, E. Schmidlin and M. W. Balk, Appl. Spectrosc., 1980, 34, 420–434 CrossRef CAS.
- D. Feuerstein, K. H. Parker and M. G. Boutelle, Anal. Chem., 2009, 81, 4987–4994 CrossRef CAS PubMed.
- P. M. Ramos and I. Ruisánchez, J. Raman Spectrosc., 2005, 36, 848–856 CrossRef CAS.
- R. Gautam, S. Vanga, F. Ariese and S. Umapathy, EPJ Tech. Instrum., 2015, 2, 8 CrossRef.
- I. Pence and A. Mahadevan-Jansen, Chem. Soc. Rev., 2016, 45, 1958–1979 RSC.
-
A. C. Rencher and W. F. Christensen, Methods of Multivariate Analysis, 3rd edn, 2012, ISBN: 978-0-470-17896-6 Search PubMed.
- Z. Lu and T. Leen, Adv. Neural Inf. Process. Syst. 17, 2005, 849–856 Search PubMed.
- C. Matthäus, T. Chernenko, J. A. Newmark, C. M. Warner and M. Diem, Biophys. J., 2007, 93, 668–673 CrossRef PubMed.
- J. H. Ward Jr., J. Am. Stat. Assoc., 1963, 58(301), 236–244 CrossRef.
- P. Bassan, A. Sachdeva, A. Kohler, C. Hughes, A. Henderson, J. Boyle, J. H. Shanks, M. Brown, N. W. Clarke and P. Gardner, Analyst, 2012, 137, 1370–1377 RSC.
- M. Hedegaard, C. Matthäus, S. Hassing, C. Krafft, M. Diem and J. Popp, Theor. Chem. Acc., 2011, 130, 1249–1260 Search PubMed.
- S. M. Ali, F. Bonnier, A. Tfayli, H. Lambkin, K. Flynn, V. McDonagh, C. Healy, T. Clive Lee, F. M. Lyng and H. J. Byrne, J. Biomed. Opt., 2012, 18, 61202 CrossRef PubMed.
- B. Bergner, B. F. M. Romeike, R. Reichart, R. Kalff, C. Krafft and J. Popp, Analyst, 2013, 138, 3983–3990 RSC.
- S. M. Ali, F. Bonnier, H. Lambkin, K. Flynn, V. McDonagh, C. Healy, T. C. Lee, F. M. Lyng and H. J. Byrne, Anal. Methods, 2013, 5, 2281–2291 RSC.
- M. Miljković, T. Chernenko, M. J. Romeo, B. Bird, C. Matthäus and M. Diem, Analyst, 2010, 135, 2002–2013 RSC.
- H. J. Byrne, P. Knief, M. E. Keating and F. Bonnier, Chem. Soc. Rev., 2016, 45, 1865–1878 RSC.
- J. M. P. Nascimento and J. M. B. Dias, IEEE Trans. Geosci. Remote Sens., 2005, 43, 898–910 Search PubMed.
- S. M. Ali, F. Bonnier, K. Ptasinski, H. Lambkin, K. Flynn, F. M. Lyng and H. J. Byrne, Analyst, 2013, 138, 3946–3956 RSC.
- F. Bonnier and H. J. Byrne, Analyst, 2012, 137, 322–332 RSC.
- T. Chernenko, R. R. Sawant, M. Miljkovic, L. Quintero, M. Diem and V. Torchilin, Mol. Pharm., 2012, 9, 930–936 CrossRef CAS PubMed.
- S. A. Mian, C. Yorucu, M. S. Ullah, I. U. Rehman and H. E. Colley, J. Tissue Eng. Regener. Med., 2017, 11, 3253–3262 CrossRef CAS PubMed.
- C. Beleites, U. Neugebauer, T. Bocklitz, C. Krafft and J. Popp, Anal. Chim. Acta, 2013, 760, 25–33 CrossRef CAS PubMed.
- J. Riedl, S. Esslinger and C. Fauhl-Hassek, Anal. Chim. Acta, 2015, 885, 17–32 CrossRef CAS PubMed.
- B. A. Gutman, X. Hua, P. Rajagopalan, Y. Y. Chou, Y. Wang, I. Yanovsky, A. W. Toga, C. R. Jack, M. W. Weiner and P. M. Thompson, Neuroimage, 2013, 70, 386–401 CrossRef PubMed.
- D. Pérez-Guaita, J. Kuligowski, S. Garrigues, G. Quintás and B. R. Wood, Analyst, 2015, 140, 2422–2427 RSC.
- I. J. Pence, C. A. Patil, C. A. Lieber and A. Mahadevan-Jansen, Biomed. Opt. Express, 2015, 6, 2724 CrossRef CAS PubMed.
-
L.-M. Wong Kee Song, A. Molckovsky, K. K. Wang, L. J. Burgart, B. Dolenko, R. L. Somorjai and B. C. Wilson, Proc. SPIE 5692, Adv. Biomed. Clin. Diagnostic Syst. III, 2005, vol. 5692, pp. 140–146.
- A. J. Berger, T.-W. Koo, I. Itzkan, G. Horowitz and M. S. Feld, Appl. Opt., 1999, 38, 2916 CrossRef CAS PubMed.
-
I. K. Lednev, E. Ryzhikova, O. Kazakov, L. Halamkova, D. Celmins, E. Molho and E. A. Zimmerman, in Annals of Neurology, 2014, vol. 76, pp. S94–S94 Search PubMed.
- I. Barman, N. C. Dingari, A. Saha, S. McGee, L. H. Galindo, W. Liu, D. Plecha, N. Klein, R. R. Dasari and M. Fitzmaurice, Cancer Res., 2013, 73, 3206–3215 CrossRef CAS PubMed.
- Z. Huang, S. K. Teh, W. Zheng, K. Lin, K. Y. Ho, M. Teh and K. G. Yeoh, Biosens. Bioelectron., 2010, 26, 383–389 CrossRef CAS PubMed.
- E. Vargis, E. M. Kanter, S. K. Majumder, M. D. Keller, R. B. Beaven, G. G. Rao and A. Mahadevan-Jansen, Analyst, 2011, 136, 2981–2987 RSC.
- S. K. Teh, W. Zheng, K. Y. Ho, M. Teh, K. G. Yeoh and Z. Huang, J. Biomed. Opt., 2008, 13, 34013 CrossRef PubMed.
Footnote |
† Co-first authors with equal contributions. |
|
This journal is © The Royal Society of Chemistry 2018 |
Click here to see how this site uses Cookies. View our privacy policy here.