DOI:
10.1039/C7SC03477F
(Edge Article)
Chem. Sci., 2017,
8, 7228-7235
Design of an enantioselective artificial metallo-hydratase enzyme containing an unnatural metal-binding amino acid†
Received
9th August 2017
, Accepted 1st September 2017
First published on 4th September 2017
Abstract
The design of artificial metalloenzymes is a challenging, yet ultimately highly rewarding objective because of the potential for accessing new-to-nature reactions. One of the main challenges is identifying catalytically active substrate–metal cofactor–host geometries. The advent of expanded genetic code methods for the in vivo incorporation of non-canonical metal-binding amino acids into proteins allow to address an important aspect of this challenge: the creation of a stable, well-defined metal-binding site. Here, we report a designed artificial metallohydratase, based on the transcriptional repressor lactococcal multidrug resistance regulator (LmrR), in which the non-canonical amino acid (2,2′-bipyridin-5yl)alanine is used to bind the catalytic Cu(II) ion. Starting from a set of empirical pre-conditions, a combination of cluster model calculations (QM), protein–ligand docking and molecular dynamics simulations was used to propose metallohydratase variants, that were experimentally verified. The agreement observed between the computationally predicted and experimentally observed catalysis results demonstrates the power of the artificial metalloenzyme design approach presented here.
Introduction
The design of tailored enzymes for new-to-nature reactions is a long-standing challenge that, when achieved, could bring great reward for organic synthesis. Yet, our limited understanding of the relationship between protein structure and catalytic activity makes the design of stable folds for de novo enzymes still far from routine.1 In metalloenzymes, this is further compounded by the challenge of designing a stable and well-defined binding site for a catalytic metal ion using canonical amino acids only. Indeed, examples of de novo design of new catalytically active metalloenzymes are scarce.2–7 For this reason, the redesign of existing proteins into artificial metalloenzymes by introduction of catalytically active transition metal complex into existing protein scaffolds, is an attractive alternative. In this approach, the catalytic chemistry is due to the artificial metal cofactor, whereas the environment and second coordination sphere interactions provided by the protein scaffold will be key to achieving enzyme-like activities and selectivities.8–12
To date, the design of artificial metalloenzymes is often intuitive, based on available X-ray structural information of the protein. While this definitely has proven its value, computational enzyme design methods could add a lot because of the large molecular space available for the design.13 For non-metal containing enzymes this is exemplified by methods such as Rosetta, which has given rise to a few first generation designer enzymes that have proven good starting points for subsequent computational redesign and laboratory evolution.13–19 In case of metalloenzymes, computational studies have mostly focused on the redesign of metalloenzymes,20–22 whereas for artificial metalloenzymes, computational methods have been used to optimize the design and elucidate the mechanism.21,23–26
One of the main challenges in the design of artificial metalloenzymes is identifying catalytically active substrate–cofactor–host geometries. Part of this challenge can be addressed by introduction of unnatural metal chelating amino acids into a protein. From a computational perspective, this is an attractive approach since it reduces some of the complications by providing a stable metal binding site at the onset of the metalloenzyme design process.27,28
Introduction of unnatural amino acids (UAAs), including those containing a metal chelating side chain, can be achieved at the genetic level using the amber stop codon suppression methodology.28–32 Recently, we have applied this expanded genetic code methodology to create a catalytically active artificial metalloenzyme by in vivo incorporation of (2,2′-bipyridin-5-yl)alanine (BpyA) into lactococcal multidrug resistance regulator (LmrR), a transcriptional repressor from L. lactis that has no natural catalytic function.33,34 This was achieved by empirical design, based on available X-ray structural information. The resulting artificial metalloenzymes were employed in the enantioselective Friedel–Crafts alkylation of indoles, resulting in moderate to good enantioselectivities. Encouraged by these results, we decided to focus on catalysis of a particularly challenging reaction: the enantioselective conjugate addition of the water to enones. This is an appealing reaction as it gives rise to chiral β-hydroxy ketones with complete atom economy. In nature, hydration of enones is well known, but because these reactions are part of the primary metabolism, natural hydratases are highly substrate specific.35–37
Enantioselective hydration of enones is still an unsolved problem for conventional asymmetric catalysis, due to the challenges associated with using water as a nucleophile, which includes its small size and low reactivity at neutral pH.38,39 Indeed, the available mechanistic information for natural hydratases35,40–42 as well as the few reported examples of enantioselective hydration of enones by hybrid catalysts43,44 suggest that these reactions critically depend on 2nd coordination sphere interactions to bind, activate and direct the water nucleophile. This makes this transformation an attractive target for computational metalloenzyme design.
Here we show how, based on empirical (bio-)chemical knowledge of the reaction of interest, computational design could be used for the creation of novel artificial metallohydratases, which were then applied in the catalytic enantioselective hydration of enones. A key feature of these artificial metalloenzymes is that they contain a genetically introduced unnatural amino acid for binding of the catalytically active metal ion in their designed active site.
Results and discussion
The approach followed is outlined in Fig. 1. The design starts from (bio-)chemical knowledge of the reaction of interest and the protein scaffold, which are then used as input for the computational design involving a combination of QM, protein ligand docking and MD simulations. On the basis of (bio-)chemical knowledge, a suitable protein comprising an unnatural metal binding amino acid is chosen (cofactor = UAA + metal). The binding of the target substrate to the catalytic site is initially characterized. Reduced cluster models are used to investigate the reaction mechanism and identify molecular features that are important for efficient catalysis. These structural determinants are then used to propose protein variants with expected improved activity. Protein–ligand docking and molecular dynamics simulations allow to investigate the effect of point mutations on the conformational properties of the assembly and thus assess the viability of the candidate variant. The resulting selected designs are then experimentally validated.
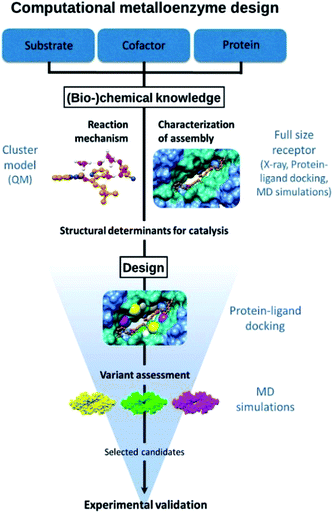 |
| Fig. 1 Outline of the design approach of an artificial metalloenzyme. | |
Design considerations
Natural hydratases have been studied extensively and key to their activity is a dual activation process, which involves (1) an electrophilic activation of the enone, e.g. by hydrogen bonding to the carbonyl and (2) the activation of the water nucleophile by a judiciously placed general base.39–42
Our design was based on the same principle: a Cu(II)–(2,2′-bipyridine) complex, involving the BpyA unnatural amino acid, that acts as Lewis acid to activate the conjugated double bond via coordination to the carbonyl oxygen, and a judiciously positioned carboxylate moiety that acts as a general base (Scheme 1). Moreover, the positioning of this general base with respect to one prochiral face of the enone can be used to induce enantioselectivity in the water addition step. Indeed, quantum chemical cluster calculations and previous mechanistic studies of natural hydratases41,42,45,46 indicate that a properly positioned carboxylic group from aspartate or glutamate residues, acting as general base, favors the hydration of the double bond.
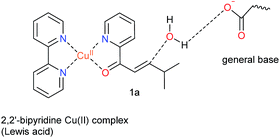 |
| Scheme 1 Dual activation strategy for catalysis of the enantioselective hydration reaction. The Cu(II) ion is bound to 2,2′-bipyridine, the side chain of the unnatural amino acid BpyA at position 89 of LmrR, and to the α,β-unsaturated 2-acyl pyridine, the substrate. Hereafter, this complex is named BpyA–Cu(II)–1a. | |
LmrR,47 an established and versatile scaffold for metalloenzyme design34,48,49 was selected as the protein host. LmrR is a homodimeric protein with a size of 13.5 kDa per monomer, that acts as a transcriptional repressor for the production of a multidrug ABC transporter. LmrR contains a unique flat shaped hydrophobic pore at the dimer interface, where normally hydrophobic antibiotic molecules are bound. This makes LmrR attractive for artificial enzyme design, as the hydrophobic nature of the interior of the pore already provides a generic driving force for binding of organic substrates.34 Since LmrR is a homodimer, genetic introduction of the unnatural amino acid will result in 2 two of these to be present in the hydrophobic pore. Position 89 was selected for introduction of BpyA, because this position is at the far end of the hydrophobic pocket, thus avoiding the risk of formation of poorly active 2
:
1 ligand to metal complexes.22 Indeed, the corresponding Cu(II) enzyme showed moderate activity and selectivity in the catalysed hydration reaction (vide infra), thus confirming this was a viable starting point for the computational studies.
Computational design
DFT calculations, carried out with density functional theory B3LYP:D3
50–52 and the basis set 6-31g** for non-metallic atoms53 and SDD for copper54 (with an added f polarization function), were performed on a reduced model of a Cu(II)–(2,2′bipyridine) complex with substrate 1a bound and surrounded by water molecules and a carboxylate in the second coordination sphere of the metal (Fig. S1†). Those calculations showed that proper arrangement of the electrophilic and nucleophilic activation may result in a low activation barrier for the addition of a water molecule to the conjugated double bond (8.7 kcal mol−1). Based on these calculations, the distance between the oxygen of the side chain carboxylate and the β carbon of the substrate that is attacked by the nucleophilic water molecule needs to be in the range of ca. 3.5 to 5 Å. This is in agreement with the distances found in X-ray structures of hydratases with substrate bound.45,46,55 Protein–ligand docking experiments were performed to generate 3D models of LmrR_M89BpyA (further referred to as LmrR_M89X) with the α,β-unsaturated 2-acyl pyridine substrate 1a bound to the Cu(II) ion as a bidentate ligand in a square planar geometry (BpyA–Cu(II)–1a). The structure of BpyA–Cu(II)–1a was optimized using the same DFT scheme as in our initial cluster models. Protein–ligand docking was carried out following the procedure we optimized for artificial metalloenzymes.56 In short, calculations were performed with the program GOLD and the ChemScore scoring function.57 The covalent docking option was used with a junction between the Cβ position of residue 89 and the 5 position of the 2,2′-bipyridine.24,26 The docking experiments led to good predicted interaction energies of 42 ChemScore units (Table S1†). The two cofactor–1a complexes, one per monomeric unit of LmrR, were found to fit well between the α4 and α4′ helices of the dimer (Fig. 2) and in general a good hydrophobic complementarity was observed between the aromatic rings of the cofactor and the binding site pocket of the protein with a major edging or stacking interaction with F93. Additionally, the pyridine moiety of each substrate was observed to have hydrophobic interactions with the V15 side chain, while their isopropyl groups are sandwiched between the central tryptophans W96 and W96′. Moreover, the docking calculations also reveal that D100 and D100′ are the only potential general base residues near the substrates. A Molecular Dynamics (MD) simulation was performed to explore the conformational properties of the design. 100 ns simulations were collected starting from the best docking solution. From analysis of the simulation, the following features emerged (Fig. 3A): (i) the two cofactor–substrate complexes remain well stabilized at the dimeric interface of LmrR by hydrophobic interactions with neighboring residues including F93 and W96. However, some flexibility was observed: one of the substrate bound cofactors was displaced toward the entrance of the LmrR binding pocket and became more exposed to the solvent. This flexibility and the change in the nature of the interaction of the substrate with the artificial metalloenzyme could affect negatively the catalytic profile of the enzyme but also suggests a possibility for further optimization; (ii) water accessibility is not equivalent on the prochiral faces of the substrate, suggesting that the protein may screen one side of the copper complex and thus induce enantioselectivity; (iii) D100, the only candidate for the role of general base in the hydration process, was found not to approach the double bond of the substrate closely enough; the distance between the oxygen of the carboxylate moiety of the amino acid and the β carbon of the substrate is generally too large. However, pre-reactive configurations, that is the configuration in which the cofactor–substrate complex, the general base and surrounding waters are arranged appropriately for the reaction to proceed, may still be achieved in a fraction of configurations (about 10%). Most of these configurations involve approach from the pro-R face of the substrate. From this, we predicted that LmrR_M89X would show some catalytic activity, forming one enantiomer preferentially, but likely with a modest yield.
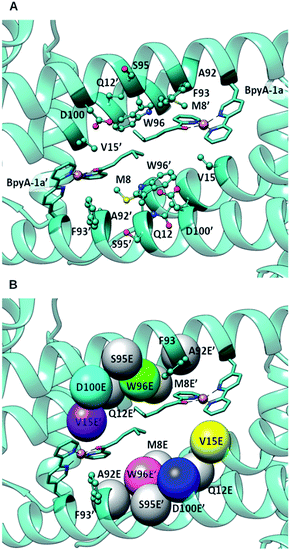 |
| Fig. 2 (A) Docking of BpyA–Cu(II)–1a at position 89 of LmrR; (B) positions selected for the introduction of the glutamate residue that serves as catalytic residue. Models including mutations coloured in grey did not lead to reactive arrangements of the protein/cofactor/substrate assembly. Calculations were carried out using the structure of a drug bound variant of LmrR (pdb code 3F8F).47 | |
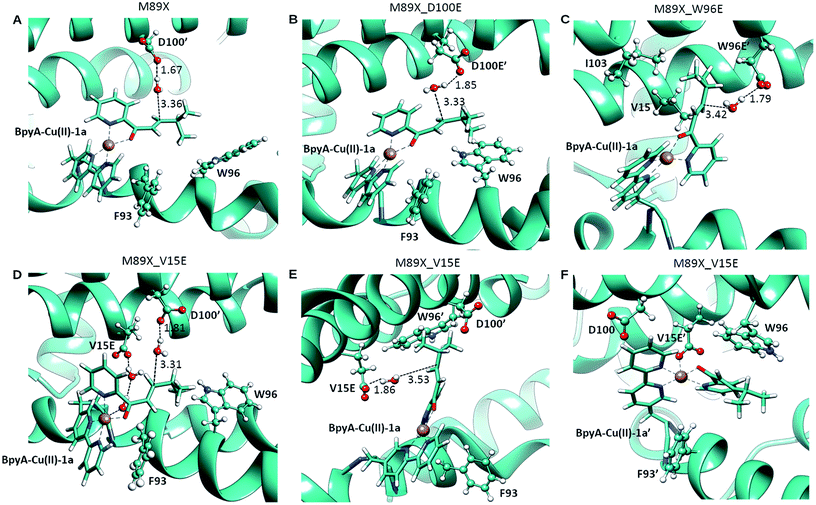 |
| Fig. 3 Pre-reactive conformations from molecular dynamics simulations of (A) LmrR_M89X, (B) LmrR_M89X_D100E, (C) LmrR_M89X_W96E and (D–F) LmrR_M89X_V15E. Pre-reactive conformations are defined on the basis of the closeness of a water molecule to the β carbon of the substrate and the hydrogen-bonding to an aspartate or glutamate residue. Similar pre-reactive conformations are reached in 10% of the simulation by LmrR_M89X, 10% by LmrR_M89X_D100E, 91% LmrR_M89X_W96E and 31% by LmrR_M89X_V15E (Table S3†). The BpyA–Cu(II)–1a force field parameters were calculated with programs MCPB for the bonding terms and RESP for atomic charges, both from the AMBER program package.58 | |
The docking results were analysed further to identify amino acids in the second coordination sphere of the metal that could be mutated to either aspartate or glutamate and fulfil our structural criteria for efficient hydration (Fig. 2B). Residues V15, W96 and F93 were found to have terminal side chain atoms at 5 Å from the electrophilic carbon of 1a. F93 was discarded as possible mutation site since it is involved in π–π stacking with the bipyridine complex. Taking into account the flexibility observed in the MD and the rearrangement of the receptor, we then extended our search to a 7 Å radius, which resulted in 5 more sites as possible candidates: M8, Q12, A92, S95, D100. However, model building the corresponding D or E variants and subsequent docking of the cofactor–substrate moiety showed that positions 8, 12, 92 and 95 were not suitable, as the low energy docking solutions were characterized by a too long distance between the side chain oxygen of the D/E residues and the electrophilic carbon (Tables S1 and S2†). Hence, the M89X_D100E, M89X_W96E and M89X_V15E mutants were selected and further investigated by MD simulations.
In case of LmrR_M89X_D100E, the two cofactor–substrate complexes were not equivalent on the time scale of the simulation. BpyA–Cu(II)–1a explored three successive conformations, approximately equally populated, the last of which featured the cofactor outside the cavity formed by the monomers. Water accessibility at the pro-chiral faces was similar, yet pre-reactive conformations involving E100′ and E100 (first conformation, Fig. 3C) and E107 (second conformation, Table S3†) formed to the pro-R face of the substrate. For BpyA–Cu(II)–1a′ two conformations were observed and pro-reactive conformations, involving E100 at the pro-R face of the substrate, were observed only in a small set of conformations (Table S3†). These data suggest that LmrR_M89X_D100E should display reactivity and enantiomeric preference similar to LmrR_M89X.
In LmrR_M89X_W96E, BpyA–Cu(II)–1a and BpyA–Cu(II)–1a′ display a similar behaviour during the MD simulation (Fig. 3C). Both stay predominantly at the dimer interface; only short fluctuations outside the pocket were observed. For both cofactors, a high number of pre-reactive conformations are attained and these involve more the pro-S than the pro-R face of the substrate. Additionally, E97 and E97′, which are capable of interacting with both substrates, but also D100 and D100′ may be involved in activating the water nucleophile (Fig. S6 and Table S3†). These data suggest that M89X_W96E should display higher activity and have a preference for the opposite enantiomer of the product 2a compared to LmrR_M89X.
MD simulation of LmrR_M89X_V15E showed that the BpyA–Cu(II)–1a complex is more water exposed than BpyA–Cu(II)–1a′. BpyA–Cu(II)–1a mostly maintains a conformation in which the plane of the Cu complex is roughly perpendicular to the axis of the LmrR protein. Interestingly, the substrate displays pre-reactive configurations three times more often than in the simulation of LmrR_M89X. Activation of the H2O nucleophile by E15 preferentially takes place at the pro-R face (Fig. 3E). Surprisingly, D100′ was found also to engage in pre-reactive conformations from the same prochiral face, even somewhat more frequent than E15 (Fig. 3D, Table S3†). BpyA–Cu(II)–1a′ maintained a single conformation within the dimer interface, which is due to the interaction of Cu(II) ion with E15′ (Fig. 3F). This stabilizing interaction is maintained throughout the simulation and hinders the approach of water to the pro-R face of the substrate. The pro-S face is exposed to the solvent, but only rarely accesses configurations compatible with the pre-reactive geometry of reactants. These data suggest that M89X_V15E should display higher activity than the template M89X and preference for the formation same hydrated enantiomer as M89X.
Preparation of mutants
The amber stop codon suppression methodology was used to introduce the unnatural metal-binding amino acid BpyA into LmrR at position M89 (LmrR_M89X).30,34 LmrR_M89X contained two additional mutations, K55D and K59Q, which reduce DNA-binding and thus facilitate the purification, and a C-terminal Strep-tag.34,44 The mutants selected from the in-silico study were prepared using standard Quick-Change mutagenesis methods (Stratagene) and expressed in E. coli BL21(DE3). Successful incorporation of BpyA was confirmed by high-resolution MS (Fig. S7 and S8†). Size-exclusion chromatography showed all LmrR mutants eluting as single peak at an elution volume of 11.6 (±0.4) ml, which is consistent with a homodimeric structure of molecular weight around 30 kDa (Fig. S8†). This suggests that the dimeric structure is retained and neither the BpyA nor any of the mutations caused significant perturbation of the structure.
Catalytic hydration
The catalytic activity of the designed metalloenzymes was tested in the Cu(II)-catalyzed enantioselective 1,4-addition of water to α,β-unsaturated 2-acyl pyridine 1a to yield in corresponding β-hydroxy ketone product 2a (Table 1). After 3 days, a conversion of 11% was obtained in the uncatalyzed reaction, while catalysis by 9 mol% of Cu(NO3)2, in absence of protein, gave rise to 83% conversion (Table 1, entry 1 and 2). The reaction in the presence of protein, but in absence of Cu(II) salts, gave similar results to the uncatalyzed reaction (Table 1, entry 3). Upon binding of Cu(II) to LmrR_M89X, a moderate ee and conversion were obtained (Table 1, entry 4). Gratifyingly, all three designed mutants gave rise to an increased conversion of 1a. Using the M89X_D100E mutant, a modest increase of conversion to 50% was obtained, accompanied by a slight decrease of ee with compared to LmrR_M89X (Table 1, entry 5). In case of the mutant, M89X_W96E the highest increase in conversion was observed, albeit accompanied by a strong decrease of the ee to almost racemic (Table 1, entry 9). The most interesting case was the mutant M89X_V15E which gives rise to both increased conversion and enantioselectivity (Table 1, entry 7). These results suggest that the placement of a general base at an appropriate position with respect to the Cu(II) complex is an effective approach to improve activity and selectivity in the catalysed hydration reaction. This was confirmed by mutagenesis of the introduced glutamate to glutamine, which is sterically similar but lacks the negative charge and, therefore, the ability to act as a general base. As expected, the activity of the glutamine mutants LmrR_M89X_V15Q and LmrR_M89X_D100Q was similar to, or even somewhat lower than LmrR_M89X (Table 1, entries 6, 8 and 10). Moreover, with both glutamine mutants, the enantioselectivity of the reaction was also significantly lowered, confirming that the glutamate residue, in particular in case of LmrR_M89X_V15E, plays a role also in selectively positioning the water nucleophile with respect to one prochiral face of the enone. The W96Q mutant gave similar results to LmrR_M89X_W96E, consistent with the observation from the MD simulations that multiple other E and D residues can act as the general base in this case.
Table 1 Results of the enantioselective hydration reaction catalyzed by LmrR_X_Cu(II)a

|
Entry |
Catalyst |
Substrate |
Product |
Conversion (%) |
ee (%) |
Standard conditions: 9 mol% Cu(H2O)6(NO3)2 (90 μM) loading with 1.25 eq. LmrR_X (in monomer) in 20 mM MOPS buffer (pH 7.0), 250 mM NaCl, for 3 days at 4 °C. All data are the average of 2 independent experiments, each carried out in duplicate. Errors are reported as standard deviation.
Conditions the same as in the experiments with 1a.
|
1 |
— |
1a
|
2a
|
11 ± 3 |
— |
2 |
Cu(NO3)2 |
1a
|
2a
|
83 ± 9 |
— |
3 |
LmrR_M89X (no Cu(II)) |
1a
|
2a
|
9 ± 3 |
— |
4 |
LmrR_M89X_Cu(II) |
1a
|
2a
|
39 ± 7 |
42 ± 6 |
5 |
LmrR_M89X_D100E_Cu(II) |
1a
|
2a
|
50 ± 6 |
30 ± 1 |
6 |
LmrR_M89X_D100Q_Cu(II) |
1a
|
2a
|
35 ± 3 |
<5 |
7 |
LmrR_M89X_V15E_Cu(II) |
1a
|
2a
|
75 ± 9 |
64 ± 2 |
8 |
LmrR_M89X_V15Q_Cu(II) |
1a
|
2a
|
28 ± 5 |
15 ± 3 |
9 |
LmrR_M89X_W96E_Cu(II) |
1a
|
2a
|
79 ± 3 |
6 ± 4 |
10 |
LmrR_M89X_W96Q_Cu(II) |
1a
|
2a
|
64 ± 1 |
6 ± 1 |
![[thin space (1/6-em)]](https://www.rsc.org/images/entities/char_2009.gif) |
Substrate scope
|
11 |
Cu(NO3)2 |
1b
|
2b
|
86 ± 4 |
— |
12 |
LmrR_M89X_Cu(II) |
1b
|
2b
|
37 ± 2 |
41 ± 4 |
13 |
LmrR_M89X_V15E _Cu(II) |
1b
|
2b
|
64 ± 8 |
50 ± 2 |
14 |
Cu(NO3)2 |
1c
|
2c
|
26 ± 4 |
— |
15 |
LmrR_M89X_Cu(II) |
1c
|
2c
|
24 ± 4 |
19 ± 5 |
16 |
LmrR_M89X_V15E_Cu(II) |
1c
|
2c
|
58 ± 5 |
14 ± 1 |
17 |
Cu(NO3)2 |
1d
|
2d
|
37 ± 12 |
— |
18 |
LmrR_M89X_Cu(II) |
1d
|
2d
|
17 ± 4 |
22 ± 2 |
19 |
LmrR_M89X_V15E_Cu(II) |
1d
|
2d
|
45 ± 5 |
57 ± 3 |
Substrate tolerance
The substrate tolerance of the designed artificial metallo-hydratase enzymes was explored by varying the substituent at the β-position of the α,β-unsaturated 2-acyl pyridine (Table 1, substrate 1a–1d). Using LmrR_M89X, good ee's were obtained for products 2a and 2b (Table 1, entries 4 and 12), while with substrates 1c and 1d low conversions and ee's were obtained (Table 1, entries 15 and 18). In the case of LmrR_M89X_V15E, with substrates 1a, 1b good ee's were obtained (Table 1, entries 6 and 13). Interestingly, with all 4 substrates the conversion was higher than in the reactions catalyzed by LmrR_M89X. Notably, in case of substrates 1c and 1d, which contain more hydrophobic substituents at the β position, the conversions obtained with LmrR_M89X_V15E were also higher than those obtained when using Cu(NO3)2 alone. Most likely this is due to the more favorable binding of these substrates in the hydrophobic pocket of LmrR.
Kinetics
The kinetics for the hydration of 1a catalyzed by LmrR_M89X–Cu(II) and LmrR_M89X_V15E–Cu(II) were determined. All the measurements were performed at 20 °C in 20 mM MOPS buffer, 250 mM NaCl, 6.7% MeCN, pH 7.0. Analysis was performed by reversed phase HPLC, monitoring the formation of product 2a over time as a function of the concentration of the substrate 1a (0.1–3 mM). In both cases, saturation kinetics, typically associated with enzyme catalysis, were observed (Fig. 4). The obtained catalytic parameters of the LmrR_M89X are kcat = 0.010 ± 0.003 min−1, KM = 3.69 ± 1.40 mM, kcat/KM = 2.71 min−1 M−1. The catalytic parameters of LmrR_M89X_V15E are kcat = 0.012 ± 0.001 min−1 and KM = 1.79 ± 0.17 mM, kcat/KM = 6.60 min−1 M−1. Fitting the data to Michaelis–Menten kinetics showed a 3-fold increase of the catalytic efficiency of LmrR_M89X_V15E compared to LmrR_M89X. The observed differences are too small to allow for a detailed discussion about the origin, but it does again confirm the viability of the design.
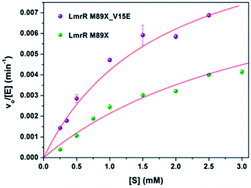 |
| Fig. 4 Kinetics of the hydration of 1a catalyzed by LmrR_M89X_Cu(II) and LmrR_M89X_V15E_Cu(II). The red line represents the fit obtained using the Michaelis–Menten equation (vo/[E] = kcat[S]/(KM[S])). | |
Agreement between computation and experiment
In general, a good agreement between the predictions from computation and the experimental catalysis results was observed. The MD simulation of LmrR_M89X–Cu(II)–1a revealed two phenomena: the possibility for the cofactors to move outside the catalytic pocket and the fact that the distance of the only general base in the vicinity of the electrophilic carbon of the enone, D100, is too large for efficient activation of the water nucleophile. Intuitively, mutagenesis of D100 to E would bring a general base closer to the substrate, resulting in higher activity. But, the MD simulation of M89X_D100E showed that such an improvement is not realized. This, was reflected in the experimental results, which confirmed that this mutant is only slightly better than LmrR_M89X, while it is less enantioselective.
In contrast, variants LmrR_M89X_V15E and LmrR_M89X_W96E were predicted by computation to be more active than LmrR_M89X because they do show pre-reactive conformations in which a general base is at an optimal distance from the electrophilic carbon from the substrate for significantly more times during the MD simulation. This was indeed reflected in the higher conversions obtained with these mutants, as well as in the increase in catalytic efficiency of LmrR_M89X_V15E as observed in the Michaelis–Menten kinetics. Interestingly, in the latter mutant the MD simulations suggested a dual role for the E15/E15′ residues: they can act as a general base, in addition to D100, but also assist in stabilizing the cofactor in the interior of the pore by interaction with the Cu(II) ion.
The MD simulations also allowed qualitative predictions about the enantioselectivity of the reactions by comparison of the prevalence of pro-S and pro-R pre-reactive conformations for the various mutants. In absence of experimental knowledge about the absolute configuration of the products, the predicted stereochemical course of the reactions, giving rise to R or S products, cannot be verified to date. However, a relative comparison of the mutants can be made. LmrR_M89X_V15E was predicted to have the same enantiomeric preference as LmrR_M89X, that is, the same enantiomer of the product would be formed in excess, but with higher enantioselectivity. Indeed, experimentally the same enantiomeric preference was observed for LmrR_M89X_V15E compared to LmrR_M89X, but with an increase of ee from 42 to 64%.
For LmrR_M89X_W96E the enantiomeric preference was predicted to be inverse to the other mutants. Experimentally, this mutant gave rise to near racemic product. This might be related to the fact that for this mutant, additional native glutamate and aspartate residues could contribute to the catalysis, which arguably makes prediction of the enantiomeric outcome more difficult. However, it should be noted that the fact that near racemic product is obtained means that this variant does show a stronger preference for formation of the opposite enantiomer than the other mutants.
Conclusions
Here, we have presented a designed artificial metallo-hydratase enzyme, comprising an unnatural metal binding amino acid, for catalysis of a chemically challenging reaction: the enantioselective conjugate addition of water. The presence of a stable metal binding site in the protein, provided by the unnatural amino acid, BpyA, combined with chemical knowledge of the mechanism of the reaction of interest provided an excellent starting point for the computational design of metalloenzymes. It is gratifying that the computational results provided us with suitable models of our system, allowing us to predict and better understand the positions of a general base in the designed active site. Interestingly, these positions were never considered for mutagenesis based on our previous empirical design,34 showing the utility computational design, especially in absence of structural information of the artificial enzyme. Moreover, the computation in some cases also suggested that previously not anticipated interactions, such as the of binding of the Cu(II) ion by one of the introduced glutamate residues in LmrR_M89X_V15E, could play a role. These insights may be valuable for further optimization of the artificial metalloenzyme. Combined, the results illustrate that, starting from a set of empirical pre-conditions, the combination of QM, docking and MD simulations is a powerful approach to the design of artificial metalloenzymes for novel and challenging reactions.
Conflicts of interest
There are no conflicts to declare.
Acknowledgements
This project was supported by the European Research Council (ERC starting grant no. 280010), the Netherlands Ministry of Education Culture and Science (Gravitation programme no. 024.001.035), Spanish MINECO (project CTQ2014-54071-P) and the Generalitat de Catalunya (project 2014SGR989). Support of COST Action CM1306 is gratefully acknowledged. LAC thanks the Generalitat de Catalunya for a Ph.D. grant. The authors thank Prof. P.G. Schultz (The Scripps Research Institute) for kindly providing the pEVOL plasmid for in vivo incorporation of BpyA, Annika Borg for assistance with the preparation of mutants and Dr Elvira Romero for useful discussion about the kinetic measurement.
Notes and references
- D. Hilvert, Annu. Rev. Biochem., 2013, 82, 447 CrossRef CAS PubMed.
- F. Yu, V. M. Cangelosi, M. L. Zastrow, M. Tegoni, J. S. Plegaria, A. G. Tebo, C. S. Mocny, L. Ruckthong, H. Qayyum and V. L. Pecoraro, Chem. Rev., 2014, 114, 3495 CrossRef CAS PubMed.
- M. L. Zastrow, A. F. A. Peacock, J. A. Stuckey and V. L. Pecoraro, Nat. Chem., 2012, 4, 118 CrossRef CAS PubMed.
- B. S. Der, D. R. Edwards and B. Kuhlman, Biochemistry, 2012, 51, 3933 CrossRef CAS PubMed.
- M. Faiella, C. Andreozzi, R. T. M. de Rosales, V. Pavone, O. Maglio, F. Nastri, W. F. DeGrado and A. Lombardi, Nat. Chem. Biol., 2009, 5, 882 CrossRef CAS PubMed.
- W. J. Song and F. A. Tezcan, Science, 2014, 346, 1525 CrossRef CAS PubMed.
- H.-S. Park, S.-H. Nam, J. K. Lee, C. N. Yoon, B. Mannervik, S. J. Benkovic and H.-S. Kim, Science, 2006, 311, 535 CrossRef CAS PubMed.
- M. E. Wilson and G. M. Whitesides, J. Am. Chem. Soc., 1978, 100, 306 CrossRef CAS.
- J. Bos and G. Roelfes, Curr. Opin. Chem. Biol., 2014, 19, 135 CrossRef CAS PubMed.
- F. Schwizer, Y. Okamoto, T. Heinisch, Y. Gu, M. M. Pellizzoni, V. Lebrun, R. Reuter, V. Köhler, J. C. Lewis and T. R. Ward, Chem. Rev., 2017 DOI:10.1021/acs.chemrev.7b00014.
- J. C. Lewis, ACS Catal., 2013, 3, 2954 CrossRef CAS.
- O. Pàmies, M. Diéguez and J. E. Bäckvall, Adv. Synth. Catal., 2015, 357, 1567 CrossRef.
- G. Kiss, N. Çelebi-Ölçüm, R. Moretti, D. Baker and K. N. Houk, Angew. Chem., Int. Ed., 2013, 52, 5700 CrossRef CAS PubMed.
- D. Röthlisberger, O. Khersonsky, A. M. Wollacott, L. Jiang, J. DeChancie, J. Betker, J. L. Gallaher, E. A. Althoff, A. Zanghellini, O. Dym, S. Albeck, K. N. Houk, D. S. Tawfik and D. Baker, Nature, 2008, 453, 190 CrossRef PubMed.
- L. Jiang, E. A. Althoff, F. R. Clemente, L. Doyle, D. Röthlisberger, A. Zanghellini, J. L. Gallaher, J. L. Betker, F. Tanaka, C. F. Barbas, D. Hilvert, K. N. Houk, B. L. Stoddard and D. Baker, Science, 2008, 319, 1387 CrossRef CAS PubMed.
- J. B. Siegel, A. Zanghellini, H. M. Lovick, G. Kiss, A. R. Lambert, J. L. S. Clair, J. L. Gallaher, D. Hilvert, M. H. Gelb, B. L. Stoddard, K. N. Houk, F. E. Michael and D. Baker, Science, 2010, 329, 309 CrossRef CAS PubMed.
- R. Blomberg, H. Kries, D. M. Pinkas, P. R. E. Mittl, M. G. Grütter, H. K. Privett, S. L. Mayo and D. Hilvert, Nature, 2013, 503, 418 CrossRef CAS PubMed.
- I. V. Korendovych and W. F. DeGrado, Curr. Opin. Struct. Biol., 2014, 27, 113 CrossRef CAS PubMed.
- L. Giger, S. Caner, R. Obexer, P. Kast, D. Baker, N. Ban and D. Hilvert, Nat. Chem. Biol., 2013, 9, 494 CrossRef CAS PubMed.
- N. Yeung, Y.-W. Lin, Y.-G. Gao, X. Zhao, B. S. Russell, L. Lei, K. D. Miner, H. Robinson and Y. Lu, Nature, 2009, 462, 1079 CrossRef CAS PubMed.
- T. Heinisch, M. Pellizzoni, M. Dürrenberger, C. E. Tinberg, V. Köhler, J. Klehr, D. Häussinger, D. Baker and T. R. Ward, J. Am. Chem. Soc., 2015, 137, 10414 CrossRef CAS PubMed.
- S. D. Khare, Y. Kipnis, P. J. Greisen, R. Takeuchi, Y. Ashani, M. Goldsmith, Y. Song, J. L. Gallaher, I. Silman, H. Leader, J. L. Sussman, B. L. Stoddard, D. S. Tawfik and D. Baker, Nat. Chem. Biol., 2012, 8, 294 CrossRef CAS PubMed.
- V. Muñoz Robles, P. Vidossich, A. Lledós, T. R. Ward and J.-D. Maréchal, ACS Catal., 2014, 4, 833 CrossRef.
- V. M. Robles, M. Dürrenberger, T. Heinisch, A. Lledós, T. Schirmer, T. R. Ward and J.-D. Maréchal, J. Am. Chem. Soc., 2014, 136, 15676 CrossRef CAS PubMed.
- E. Sansiaume-Dagousset, A. Urvoas, K. Chelly, W. Ghattas, J.-D. Maréchal, J.-P. Mahy and R. Ricoux, Dalton Trans., 2014, 43, 8344 RSC.
- W. Ghattas, L. Cotchico-Alonso, J.-D. Maréchal, A. Urvoas, M. Rousseau, J.-P. Mahy and R. Ricoux, ChemBioChem, 2016, 17, 433 CrossRef CAS PubMed.
- J. H. Mills, S. D. Khare, J. M. Bolduc, F. Forouhar, V. K. Mulligan, S. Lew, J. Seetharaman, L. Tong, B. L. Stoddard and D. Baker, J. Am. Chem. Soc., 2013, 135, 13393 CrossRef CAS PubMed.
- C. Hu, S. I. Chan, E. B. Sawyer, Y. Yu and J. Wang, Chem. Soc. Rev., 2014, 43, 6498 RSC.
- L. Wang, A. Brock, B. Herberich and P. G. Schultz, Science, 2001, 292, 498 CrossRef CAS PubMed.
- J. Xie, W. Liu and P. G. Schultz, Angew. Chem., Int. Ed., 2007, 46, 9239 CrossRef CAS PubMed.
- A. P. Green, T. Hayashi, P. R. E. Mittl and D. Hilvert, J. Am. Chem. Soc., 2016, 138, 11344 CrossRef CAS PubMed.
- N. Budisa, J.-S. Völler, B. Koksch, C. G. Acevedo-Rocha, V. Kubyshkin and F. Agostini, Angew. Chem., Int. Ed., 2017 DOI:10.1002/anie.201610129.
- P. K. Madoori, H. Agustiandari, A. J. M. Driessen and A.-M. W. H. Thunnissen, EMBO J., 2009, 28, 156 CrossRef CAS PubMed.
- I. Drienovská, A. Rioz-Martínez, A. Draksharapu and G. Roelfes, Chem. Sci., 2015, 6, 770 RSC.
- J. Jin and U. Hanefeld, Chem. Commun., 2011, 47, 2502 RSC.
- For examples of styrene hydratases that do show promiscuous activity see: C. Wuensch, J. Gross, G. Steinkellner, K. Gruber, S. M. Glueck and K. Faber, Angew. Chem., Int. Ed., 2013, 52, 2293 CrossRef CAS PubMed ; and ref. 37.
- X. Sheng and F. Himo, ACS Catal., 2017, 7, 1733 CrossRef CAS.
- C. F. Nising and S. Bräse, Chem. Soc. Rev., 2012, 41, 988 RSC.
- V. Resch and U. Hanefeld, Catal. Sci. Technol., 2015, 5, 1385 CAS.
- B.-S. Chen, L. G. Otten and U. Hanefeld, Biotechnol. Adv., 2015, 33, 526 CrossRef CAS PubMed.
- R.-Z. Liao, J.-G. Yu and F. Himo, Proc. Natl. Acad. Sci. U. S. A., 2010, 107, 22523 CrossRef CAS PubMed.
- R.-Z. Liao and F. Himo, ACS Catal., 2011, 1, 937 CrossRef CAS.
- A. J. Boersma, D. Coquière, D. Geerdink, F. Rosati, B. L. Feringa and G. Roelfes, Nat. Chem., 2010, 2, 991 CrossRef CAS PubMed.
- J. Bos, A. García-Herraiz and G. Roelfes, Chem. Sci., 2013, 4, 3578 RSC.
- B. J. Bahnson, V. A. Anderson and A. P. Petsko, Biochemistry, 2002, 41, 2621 CrossRef CAS PubMed.
- J. P. Bennett, L. Bertin, B. Moulton, I. J. S. Fairlamb, A. M. Brzozowski, N. J. Walton and G. Grogan, Biochem. J., 2008, 414, 281 CrossRef CAS PubMed.
- P. K. Madoori, H. Agustiandari, A. J. M. Driessen and A.-M. W. H. Thunnissen, EMBO J., 2009, 28, 156 CrossRef CAS PubMed.
- J. Bos, F. Fusetti, A. J. M. Driessen and G. Roelfes, Angew. Chem., Int. Ed., 2012, 51, 7472 CrossRef CAS PubMed.
- J. Bos, W. R. Browne, A. J. M. Driessen and G. Roelfes, J. Am. Chem. Soc., 2015, 137, 9796 CrossRef CAS PubMed.
- C. Lee, W. Yang and R. G. Parr, Phys. Rev. B, 1988, 37, 785 CrossRef CAS.
- A. D. Becke, J. Chem. Phys., 1993, 98, 5648 CrossRef CAS.
- S. Grimme, J. Antony, S. Ehrlich and H. Krieg, J. Chem. Phys., 2010, 132, 154104 CrossRef PubMed.
- V. A. Rassolov, M. A. Ratner, J. A. Pople, P. C. Redfern and L. A. Curtiss, J. Comput. Chem., 2001, 22, 976 CrossRef CAS.
- H. Stoll, P. Fuentealba, P. Schwerdtfeger, J. Flad, L. V. Szentpály and H. Preuss, J. Chem. Phys., 1984, 81, 2732 CrossRef CAS.
- P. Kasaragod, W. Schmitz, J. K. Hiltunen and R. K. Wierenga, FEBS J., 2013, 280, 3160 CrossRef CAS PubMed.
- V. Muñoz Robles, E. Ortega-Carrasco, L. Alonso-Cotchico, J. Rodriguez-Guerra, A. Lledós and J.-D. Maréchal, ACS Catal., 2015, 5, 2469 CrossRef.
- M. L. Verdonk, J. C. Cole, M. J. Hartshorn, C. W. Murray and R. D. Taylor, Proteins, 2003, 52, 609 CrossRef CAS PubMed.
- M. B. Peters, Y. Yang, B. Wang, L. Füsti-Molnár, M. N. Weaver and K. M. Merz, J. Chem. Theory Comput., 2010, 6, 2935 CrossRef CAS PubMed.
Footnotes |
† Electronic supplementary information (ESI) available. See DOI: 10.1039/c7sc03477f |
‡ I. D. and L. A.-C. contributed equally to this work. |
|
This journal is © The Royal Society of Chemistry 2017 |
Click here to see how this site uses Cookies. View our privacy policy here.