DOI:
10.1039/C7RA09059E
(Paper)
RSC Adv., 2017,
7, 49097-49101
Qualitative and quantitative determination of coumarin using surface-enhanced Raman spectroscopy coupled with intelligent multivariate analysis
Received
16th August 2017
, Accepted 3rd October 2017
First published on 20th October 2017
Abstract
Coumarin is harmful to health but still used in cosmetics, tobacco, or illegally added into food as a spice in trace amounts so that it is exceedingly difficult to be determined accurately. Thus, it is important to develop a reliable method to qualitatively and quantitatively determine coumarin. Herein, we report a coumarin detection method using surface-enhanced Raman spectroscopy (SERS) coupled with an intelligent multivariate analysis. First, a flower-like silver-based substrate was fabricated and characterized by XRD, TEM, and EDS. Subsequently, coumarin with different concentrations was detected using this flower-like silver as the SERS substrate. The Raman vibration assignments reflect the information about the structure of the coumarin molecule efficiently. The limits of detection (LOD) for coumarin using the flower-like silver substrate can reach 10−8 M. It means the detection limit of coumarin by this method is less than 1.46 μg kg−1, which is much more sensitive than the previously reported one. Based on the Raman characteristic peaks of coumarin, various methods like linear regression, binary linear regression, and PCA were used to quantitatively analyze coumarin. These analysis results show that with the binary linear regression model, a strong linear relationship between lg
I (I is the Raman peak intensity) and −lg
C (C is the concentration of coumarin) can be observed and the correlation coefficient R2 was close to 1. This method provides a high sensitivity and rapid method to detect the additives in food and cosmetics, etc.
Introduction
Coumarin is a natural ingredient in tonka bean, woodruff and sweet clover.1,2 It was first isolated from tonka beans in 1822 and the first chemical synthesis succeeded in 1868.3,4 It smells like fresh hay and vanilla and was originally used as a flavouring agent in food and tobacco. In 1954, coumarin was banned from being added as the flavoring agent in food in the USA because, in some animal experiments, it found to have hepatotoxic effects on rats and might be linked to cancer.5–9 In 1965, coumarin used as the flavouring substance in food was prohibited by British Food Standards Agency.5–9 Then, Germany, China and other countries issued a law to forbid the usage of coumarin as a food additive.10,11 However, in order to pursue the interests, some food processors and distributors illegally added coumarin into food products such as milk powder and soybean milk. Moreover, although it is not allowed to be added to foods, coumarin may present in some parts of the plants added to foods to provide flavor, causing food to contain a trace amount of coumarin. As an exception, coumarin is allowed to be added in cosmetics like body lotion, face-cream and so on.12 However, according to the Federal Institute for Risk Assessment, skin resorption can significantly attribute to the overall exposure of coumarin. Particularly for children, coumarin could be more harmful.12 In cosmetics, the amount of coumarin is limited to 10 μg g−1.10
Thus, analytical methods are needed for the qualitative and quantitative determination of coumarin. Nowadays, several analytical approaches have been used to determine coumarin, such as high performance liquid chromatography (HPLC),13–15 mass spectrometry (MS),16 ultra performance liquid chromatography coupled with mass spectrometry (UPLC-MS),17,18 liquid chromatography mass spectrometry (LC-MS),19,20 gas chromatography (GC),21,22 and gas chromatography with mass spectrometry (GC-MS).23,24 These methods have high selectivity and sensitivity, but they are complex and time-consuming and require pre-treatment, valuable equipment and good operation skills.
The aim of this study was to develop an easy and fast streamlined method for the detection and quantification of coumarin. Compared to other methods, surface-enhanced Raman spectroscopy (SERS) does not need the complex sample pre-treatments, has the advantages of high sensitivity, good flexibility, reliability, and fast detection and can also provide a high degree of structural information about a single molecule.25–29 SERS has been explored for wide application in bio-medicine, chemical and material analysis, and environmental monitoring.30–34 The mechanism of SERS enhancement includes electromagnetic enhancement (EM) and chemical enhancement (CM).35–38 Several researchers have shown that the electromagnetic enhancement factor is about 104 and the chemical enhancement contributes to an average enhancement factor of 100.36–38 The EM is the main contributor to the drastic signal enhancement in SERS. The EM mechanism is based on the size, shape, and type of the noble-metal substrates and their abilities to support plasmon resonances.36–40 The traditional SERS substrates include roughened electrodes, island films, and surface-confined nanostructures.37 These substrates are easy to fabricate but lack reliability and stability, and the SERS signals are not uniform. They have severely limited the wide practical applications of SERS.37,40 To overcome these shortcomings, recently, a number of approaches that use non-traditional substrates such as shell-isolated nanoparticle, silver nanocubes, and nanotriangles have been proposed; however, the reproducibility of these substrates is poor.40–42
In this study, the flower-like silver substrate was fabricated and used to detect coumarin. The substrate showed a high sensitivity for a rapid detection of coumarin. The Raman shifts, their relative intensities, and the assignments of the characteristic peaks of coumarin were summarized. These results provided the structural information of coumarin, which can be used for the qualitative analysis of coumarin. In addition, a quantitative analysis model for determination of coumarin was established using binary linear regression. This model provides a reference to quantitatively detect coumarin.
Experimental
Coumarin (≥98%), polyvinylpyrrolidone (PVP, K17, >95%), silver nitrate (AgNO3, ≥99.8%), ascorbic acid (ASA, >99.0%), and rhodamine 6G (R6G, ≥95%) were obtained from Aladdin Industrial Corporation and used without further purification. Ultrapure water (18.3 MΩ) was used for all solution preparations. A schematic of the fabrication of the flower-like silver substrate is described in Fig. 1. First, 0.2 ml of AgNO3 solution (1 M) and 2 ml of PVP solution (1%) with 10 ml of ultrapure water were added into 30 ml beaker successively. After they were mixed thoroughly in a thermostat with a magnetic stirrer at room temperature, 1 ml of ASA solution (0.1 M) was quickly added into the above-mixed solution, and then the solution was stirred persistently for 10 min (Fig. 1a). Second, the product of reaction was separated from the solution by centrifugation at 8000 rpm for 10 min and then washed with 10 ml of ultrapure water in an ultrasonic reactor. This second step was repeated four times for removing the residual impurities on the surface of silver nanoparticles (Fig. 1b). Then, nano-silver colloidal solution was obtained. Third, the silicon wafer was soaked in this nano-silver colloidal solution and dried in a vacuum oven at 60 °C for 6 h to avoid oxidation (Fig. 1c). Finally, the flower-like silver substrates were obtained (Fig. 1d).
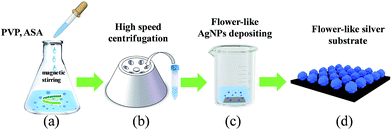 |
| Fig. 1 A schematic illustration of the fabrication of the flower-like silver substrates. | |
The R6G solutions were prepared at concentrations ranging from 10−9 to 10−4 M, and coumarin solutions were prepared at concentrations ranging from 10−8 to 10−4 M. The R6G and coumarin solutions aliquoted with different concentrations were titrated onto the flower-like silver substrates and then transferred into the vacuum oven at 40 °C for 8 h. Their SERS spectra were recorded by a confocal microscope/Raman spectrometer system (Renishaw, inVia Reflex Raman) with a 785 nm excitation wavelength with 5 s exposure time and twice accumulation.
The flower-like silver substrates were characterized field-emission scanning microscopy (HITACHI, SU8010 FE-SEM) and EDS spectroscopy (Team Apollo XL EDS). Their structure was analyzed via X-ray diffraction (XRD) (BRUKER-AXS). The UV-visible spectra were recorded via UV-vis spectroscopy (TU-1901, Purkinje, China). The Raman spectra of the probe molecules and coumarin were measured with the confocal microscope/Raman spectrometer system (Renishaw, inVia Reflex Raman).
Results and discussion
The morphology of the flower-like silver substrate was investigated via a scanning electron microscopy (SEM) system. Fig. 2a shows that the flower-like silver nanoparticles were deposited onto the silicon wafer and well dispersed and had a narrow diameter distribution (600–800 nm). Fig. 2b shows the detailed morphology of the flower-like silver nanoparticles. Numerous ridges with a thickness of 50–75 nm existed over the surface. The XRD pattern of the substrate clearly indicates that the flower-like silver nanoparticles displayed high crystallinity (Fig. 2c). Four characteristic peaks at 38.1, 44.1, 64.7 and 77.5° were assigned to the (111), (200), (220) and (311) planes of Ag crystals, respectively (JCPDS no. 01-1164). The complete composition of the substrate was further confirmed by the energy dispersive spectrum (EDS) (Fig. 2d). The UV-vis absorption spectrum of the flower-like silver colloid was measured in the range of 190–900 nm (Fig. 2e), which exhibited a broad peak from 400 to 800 nm, indicating that the substrate has a broad local surface plasmon resonance region. To evaluate the enhanced effectiveness of the substrate, R6G was chosen as a nonresonant probe molecule with a known molecular footprint. Fig. 2f illustrates the SERS spectra obtained from six different concentrations of R6G. The spectra reveal that an R6G concentration as low as 10−9 M still exhibited an observable SERS signal, indicating that the flower-like silver substrate has high sensitivity.
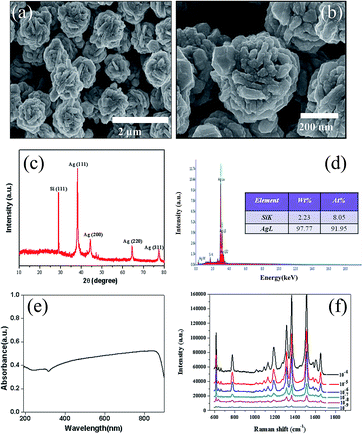 |
| Fig. 2 Basic characterization of the flower-like silver substrate. (a) SEM image (scale bar: 2 μm), (b) SEM image (scale bar: 200 nm), (c) XRD pattern, (d) EDS spectrum, (e) UV-vis spectrum of the flower-like silver substrate, and (f) SERS spectra of R6G. | |
In this study, the SERS enhancement distributed on the surface was measured by randomly collecting the SERS signal intensity from 30 different test areas of the flower-like silver substrate. Fig. 3a shows the stability of the R6G peak at 1510 cm−1 at the concentration of 10−5 M from the flower-like silver substrate. Fig. 3b shows the stability of the R6G peak at 1362 cm−1 at the concentration of 10−5 M from the flower-like silver substrate. The R6G SERS intensity varies by only 8%, indicating reproducibility and that the substrates are highly uniform.
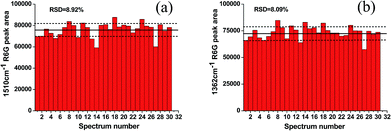 |
| Fig. 3 (a) The stability of the R6G peak at 1510 cm−1 at the concentration of 10−5 M from the flower-like silver substrate. The RSD of the peak intensity is 8.92%. (b) The stability of the R6G peak at 1362 cm−1 at the concentration of 10−5 M from the flower-like silver substrate. The RSD of the peak intensity is 8.09%. | |
As shown in Fig. 4b (top left), coumarin consists of a lactone ring and an aromatic ring. The schematic of a SERS experiment with coumarin on the flower-like silver substrate is shown in Fig. 4a. A 5 mm diameter laser was vertically projected on the sample to be tested, with a laser power of 25 mW and 5 s exposure time and twice accumulation. Fig. 4b shows the SERS spectra of coumarin at different concentrations from 10−8 M to 10−4 M. Some Raman peaks at 813, 1175, 1267, 1431, 1588, and 1665 cm−1 were clearly observed. The detection limit of coumarin was less than 1.46 μg kg−1. Table 1 shows the assignments of the main peaks of coumarin in Raman and vibration modes. These Raman shifts could be used to qualitatively analyze coumarin.
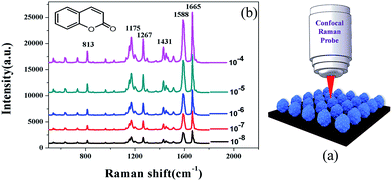 |
| Fig. 4 (a) Schematic diagram of a SERS experiment with coumarin on the flower-like silver substrate and (b) the structure of coumarin molecule (the upper left) and the SERS spectra of different concentrations of coumarin absorbed on the flower-like silver substrate. | |
Table 1 The assignments of the main peaks of coumarin in Raman and vibration modes
SERS spectrum (cm−1) |
Assignment |
813 |
Ring breath |
1175 |
C–H2 twisting and rocking in-plane |
1267 |
Ring breath |
1431 |
Ring stretching |
1588 |
C C stretching |
1665 |
Conjugated C O stretching, C C stretching |
In the SERS spectra of coumarin at different concentrations from 10−8 M to 10−4 M, the SERS signal intensities decreased with decreasing concentration of carmine. However, the characteristic peaks at 1175, 1267, 1558, and 1665 cm−1 remained, indicating that these characteristic peaks can be used for quantitative analysis. The intensity of the peaks followed the order 1665 > 1558 > 1175 > 1267 cm−1.
In order to obtain the quantitative analysis model for coumarin, different methods including linear regression, binary linear regression, and principal component analysis (PCA) were used to analyze the SERS spectra. First, the maximum characteristic peak at 1665 cm−1 was selected, and the correlation between lg
I (I is the intensity) and −lg
C (C is the concentration of coumarin) was established using linear regression. The relevant equation is
|
−Lg C = −4.34 Lg I + 23.32 with R2 = 0.988.
| (1) |
Second, two largest characteristic peaks at 1665 and 1558 cm−1 were selected and the correlation between lg
I and −lg
C was established by the binary linear regression. The relevant equation is
|
−Lg C = −38.86 Lg I1 + 33.64 Lg I2 + 28.11
| (2) |
In eqn (2), C is the concentration of coumarin (mol L−1), I1 is the intensity of the peak at 1665 cm−1, I2 is the intensity of the peak at 1558 cm−1 and R2 = 1.
Finally, three sets of characteristic peaks at (1) 1665, 1558, and 1175 cm−1, (2) 1665, 1558, and 1267 cm−1, and (3) 1665, 1558, 1267, and 1175 cm−1 were selected. The correlation between lg
I and −lg
C was established using the PCA. The relevant equation and R-Square are
|
−Lg C = −4.12 Lg I + 23.18, R2 = 0.985
| (3) |
|
−Lg C = −4.14 Lg I + 23.25, R2 = 0.985
| (4) |
|
−Lg C = −4.05 Lg I + 23.03, R2 = 0.983
| (5) |
Fig. 5 shows the fitting results of lg
I and −lg
C with different characteristic peaks and the relative error of −lg
C (C = 10−6 M) with different relevant equations. The results of the fitting and relative error verification show that the model using the binary linear regression based on the characteristic peaks at 1665 and 1558 cm−1 is the most suitable for quantitative detection of coumarin.
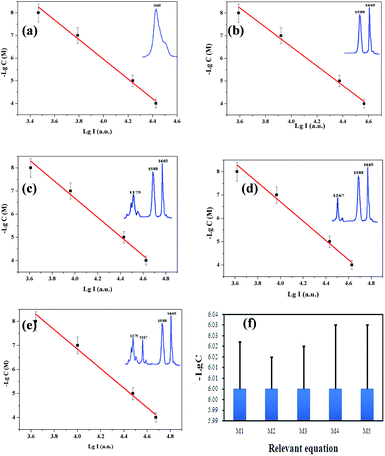 |
| Fig. 5 Fitting results of lg I and −lg C based on different characteristic peaks. (a) Linear regression with the characteristic peak at 1665 cm−1, (b) binary linear regression with the characteristic peaks at 1665 and 1558 cm−1, (c) PCA with the characteristic peaks at 1665, 1558, and 1175 cm−1, (d) PCA with the characteristic peaks at 1665, 1558, and 1267 cm−1, (e) PCA with the characteristic peaks at 1665, 1558, 1175, and 1267 cm−1, and (f) error verification of −lg C with different relevant equations, M1–M5 is the relevant eqn (1)–(5). | |
The flower-like silver substrate was employed for the practical detection of coumarin in a real sample. A type of milk powder was dissolved directly in purified water and 10−5 M coumarin was added to the milk solution. The milk solution containing coumarin was titrated onto the flower-like silver substrate and it was then transferred into the vacuum oven at 40 °C for 8 h. The SERS spectra were recorded using a confocal microscope/Raman spectrometer system (Renishaw, inVia Reflex Raman) with a 785 nm excitation wavelength and 5 s exposure time and twice accumulation. As shown in Fig. 6, some Raman peaks at 813, 892, 1136, 1267, 1360, 1588, and 1665 cm−1 were clearly observed, which include the Raman peaks of coumarin at 813, 1267, 1588, and 1665 cm−1.
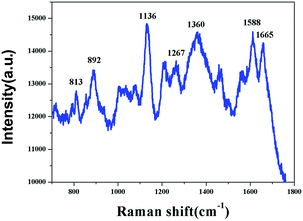 |
| Fig. 6 The SERS spectrum of the milk containing 10−5 M coumarin absorbed on the flower-like silver substrate. | |
Based on the SERS spectrum of the milk containing 10−5 M coumarin, the intensity of the characteristic peaks at 1588 and 1665 cm−1 was selected to calculate the concentrations of coumarin in the milk solution, which was 10−5 M based on the calculations using the quantitative analysis model. The RSD of the concentration was 9%. This result shows that the quantitative analysis model has high accuracy.
Conclusions
In this study, the high-sensitivity SERS substrate was fabricated by depositing flower-like silver on the surface of the silicon wafer. The results of XRD and SEM show that the flower-like silver nanoparticles have high crystallinity and a narrow diameter distribution (600–800 nm). The sensitivity measurement using a nonresonant probe molecule R6G shows that the limit of detection was 10−9 M. With these flower-like silver substrates, the Raman peaks of coumarin at 813, 1175, 1267, 1431, 1588, and 1665 cm−1 are clearly observed. These Raman peaks correspond to different vibration modes, representing the structure information of the coumarin molecule, and can be used to qualitatively analyze coumarin. The data from the SERS spectrum indicate that the detection limit of coumarin is less than 10−8 M and the corresponding mass concentration is 1.46 μg kg−1. The comparison of different data treatments applied on the Raman spectra such as linear regression, binary linear regression, and principal component analysis (PCA) show that the quantitative analysis model using binary linear regression based on the characteristic peaks at 1665 and 1558 cm−1 has a better correlation. The result from testing the real sample shows that the quantitative analysis model has high accuracy. Thus, this study describes a SERS substrate that is highly uniform, shows good reproducibility and high sensitivity and can be used in fast qualitative and quantitative detection of coumarin. In addition, this flower-like silver substrate and the related method can be applied extensively to examine other food additives.
Conflicts of interest
There are no conflicts to declare.
Acknowledgements
The project was supported by the National Science Foundation for Young Scholars of China (Grant No. 31000316), the Application Research Program of Commonweal Technology of Zhejiang Province (No. 2014C37042, 2016C37083), the Zhejiang province university students in scientific and technological innovation activities (No. 2016R409011), and the Science and Technology project of Zhejiang Province (No. 2016C33026).
Notes and references
- T. O. Soine, J. Pharm. Sci., 1964, 53, 231–264 CrossRef CAS PubMed.
- D. L. J. Opdyke, Food Chem. Toxicol., 1974, 12, 385–388 CrossRef CAS.
- D. Egan, R. O'Kennedy, E. Moran, D. Cox, E. Prosser and R. D. Thornes, Drug Metab. Rev., 1990, 22, 503–529 CrossRef CAS PubMed.
- K. Abraham, F. Wohrlin, O. Lindtner, G. Heinemeyer and A. Lampen, Mol. Nutr. Food Res., 2010, 54, 228–239 CAS.
- L. W. Hazleton, T. W. Tusing, B. R. Zeiltlin, R. Thiessen and H. K. Murer, J. Pharmacol. Exp. Ther., 1956, 118, 348–358 CAS.
- S. D. Gangolli, W. H. Shilling, P. Grasso and I. F. Gaunt, Biochem. Soc. Trans., 1974, 2, 310–312 CrossRef CAS.
- A. J. Cohen, Food Cosmet. Toxicol., 1979, 17, 277–289 CrossRef CAS PubMed.
- B. G. Lake, Food Chem. Toxicol., 1999, 37, 423–453 CrossRef CAS PubMed.
- A. J. Edwards, R. J. Price, A. B. Renwick and B. G. Lake, Food Chem. Toxicol., 2002, 38, 403–409 CrossRef.
- European Committee for Standardization, DIN EN ISO 11348–1: 2009-05.
- National Health and Family Planning Commission of the People's Republic of China, GB2760–2014.
- Frequently Asked Questions about coumarin in cinnamon and other foods, Federal Institute for Risk Assessment, 2006.
- R. V. Tamma, G. C. Miller and R. Everett, J. Chromatogr. A, 1985, 322, 236–239 CrossRef CAS.
- A. W. Arche, J. Chromatogr. A, 1988, 447, 272–276 CrossRef.
- M. J. Ahn, M. K. Lee, Y. C. Kim and S. H. Sung, J. Pharm. Biomed. Anal., 2008, 46, 258–266 CrossRef CAS PubMed.
- Y. Shen, C. Han, B. Liu, Z. Lin, X. Zhou, C. Wang and Z. Zhu, Determination of vanillin, ethyl vanillin, and coumarin in infant formula by liquid chromatography-quadrupole linear ion trap mass spectrometry, J. Dairy Sci., 2014, 97, 679–686 CrossRef CAS PubMed.
- M. Vierikova, R. Germuska and J. Lehotay, J. Liq. Chromatogr. Relat. Technol., 2008, 32, 95–105 CrossRef.
- A. Dugrand, A. Olry, T. Duval, A. Hehn, Y. Froelicher and F. Bourgaud, J. Agric. Food Chem., 2013, 61, 10677–10684 CrossRef CAS PubMed.
- L. S. de Jager, G. A. Perfetti and G. W. Diachenko, J. Chromatogr. A, 2007, 1145, 83–88 CrossRef CAS PubMed.
- Z. Ren, B. Nie, T. Liu, F. Yuan, F. Feng, Y. Zhang, W. Zhou, X. Xu, M. Yao and F. Zhang, Molecules, 2016, 21, 1511 CrossRef PubMed.
- D. Chouchi and D. Barth, J. Chromatogr. A, 1994, 672, 177–183 CrossRef CAS PubMed.
- A. A. Rahim, B. Saad, H. Osman, N. H. Hashim, S. Yahya and K. M. Talib, Food Chem., 2011, 126, 1412–1416 CrossRef CAS.
- H. H. Wisneski, J. AOAC Int., 2001, 84, 689–692 CAS.
- M. R. Kim, A. M. A. El-Aty, I. S. Kim and J. H. Shim, J. Chromatogr. A, 2006, 1116, 259–264 CrossRef CAS PubMed.
- S. Nie and S. R. Emory, Science, 1997, 275, 1102–1106 CrossRef CAS PubMed.
- K. Kneipp, Y. Wang, H. Kneipp, L. T. Perelman, I. Itzkan, R. R. Dasari and M. S. Feld, Phys. Rev. Lett., 1997, 78, 1667–1670 CrossRef CAS.
- H. Zheng, D. Ni, Z. Yu and P. Liang, Food Chem., 2017, 217, 511–516 CrossRef CAS PubMed.
- Y. Wu, P. Liang, Q. Dong, Y. Bai, Z. Yu, J. Huang, Y. Zhong, Y. Dai, D. Ni, H. Shu and U. Charles, Food Chem., 2017, 237, 974–980 CrossRef CAS PubMed.
- H. Zheng, D. Ni, Z. Yu, P. Liang and H. Chen, Sens. Actuators, B, 2016, 231, 423–430 CrossRef CAS.
- C. Zhang, R. Hao, B. Zhao, Y. Fu, H. Zhang, S. Moeendarbari, C. S. Pickering, Y. Hao and Y. Liu, Appl. Surf. Sci., 2017, 400, 49–56 CrossRef CAS.
- L. Chen, N. Qi, X. Wang, L. Chen, H. You and J. Li, RSC Adv., 2014, 4, 15055–15060 RSC.
- Y. Wang, B. Yan and L. Chen, Chem. Rev., 2013, 113, 1391–1428 CrossRef CAS PubMed.
- L. Chen, X. Fu and J. Li, Nanoscale, 2013, 5, 5905–5911 RSC.
- J. Li, L. Chen, T. Lou and Y. Wang, ACS Appl. Mater. Interfaces, 2011, 3, 3936–3941 CAS.
- F. J. García-Vidal and J. B. Pendry, Phys. Rev. Lett., 1996, 77, 1163–1166 CrossRef PubMed.
- P. L. Stiles, J. A. Dieringer, N. C. Shah and R. P. V. Duyne, Annu. Rev. Anal. Chem., 2008, 1, 601–626 CrossRef CAS PubMed.
- C. L. Haynes, A. D. McFarland and R. P. V. Duyne, Anal. Chem., 2005, 338A–346A CAS.
- M. Moskovits, J. Raman Spectrosc., 2005, 36, 485–496 CrossRef CAS.
- A. M. Michaels, M. Nirmal and L. E. Brus, J. Am. Chem. Soc., 1999, 121, 9932–9939 CrossRef CAS.
- J. Li, Y. Huang, Y. Ding, Z. Yang, S. Li, X. Zhou, F. Fan, W. Zhang, Z. Zhou, D. Wu, B. Ren, Z. Wang and Z. Tian, Nature, 2010, 464, 392–394 CrossRef CAS PubMed.
- S. Zhou, J. Li, K. D. Gilroy, J. Tao, C. Zhu, X. Yang, X. Sun and Y. Xia, ACS Nano, 2016, 10, 9861–9870 CrossRef CAS PubMed.
- F. Liebig, A. F. Thünemann and J. Koetz, Langmuir, 2016, 32, 10928–10935 CrossRef CAS PubMed.
|
This journal is © The Royal Society of Chemistry 2017 |