DOI:
10.1039/C7RA05722A
(Paper)
RSC Adv., 2017,
7, 44186-44198
Exploring potential biomarkers and determining the metabolic mechanism of type 2 diabetes mellitus using liquid chromatography coupled to high-resolution mass spectrometry†
Received
21st May 2017
, Accepted 24th August 2017
First published on 12th September 2017
Abstract
Diabetes mellitus has imposed a huge burden on modern society and is a serious threat to human health globally. Obese people usually have a higher risk of developing T2DM, and this disease is often diagnosed in older persons, accompanied by hypertension, hyperlipidemia, arteriosclerosis and other diseases. In the early stages of T2DM, patients can have difficulties in identifying any symptoms or may only suffer from slight fatigue and thirst with no obvious hyperglycemia. The early detection of this disease may provide a means of preventing the development of this disease in a more timely way. The metabolic profiling of small molecules provides a snapshot of physiological processes. By using a metabolite profiling platform based on mass spectrometry coupled with pattern recognition analysis, outstanding biomarkers that can be used for forecasting disease may be identified. In this study, we attempted to investigate potential key metabolic pathways associated with T2DM and determine its metabolic mechanism. Using multivariate statistical analysis, 33 marker metabolites associated with the metabolism of lipids, amino acids, and carbohydrates were identified. Our experiment yielded substantial new insights into serum metabolism in type 2 diabetes mellitus patients, and these metabolites will be targets for future mechanistic research to help understand the clinical significance of these metabolic abnormalities.
Introduction
Declining trends in morbidity and mortality from communicable diseases have been witnessed in recent years, although there has been a continuing increase in the occurrence of chronic non-communicable diseases (NCDs), such as cardiovascular diseases (CVDs), diabetes, tumors, chronic obstructive pulmonary disease (COPD) and chronic renal failure.1 Among these, diabetes is a prevalent disease that could be regarded as a serious threat to human health.2 According to data from the International Diabetes Federation (IDF),3 370 million people were affected by diabetes in 2011 and an estimated 550 million people will be affected by it by 2030. Diabetes mellitus not only brings about physical and mental injury to patients who suffer from it but also imposes heavy financial burdens on individuals and countries. Research by the Chinese Diabetes Society (CDS) indicates that diabetes mellitus, in particular type 2 diabetes mellitus (T2DM), represents the most significant global cost due to diabetes,4 and diagnosis and preventative work should be carried out. In the current medical situation, diabetes mellitus is still a permanent disease. The Chinese guide for the prevention of type 2 diabetes5 suggests that there are three stages in the treatment of T2DM: preventing the incidence of T2DM, preventing complications in patients diagnosed with T2DM and delaying progressive complications of T2DM, and reducing the rates of disability and fatality due to T2DM. Although the American Diabetes Association guide for the diagnosis6 of T2DM provides important details, additional important diagnostic criteria could have been included. In addition, the interpretation of potential mechanisms may create new insights into the prophylaxis and treatment of T2DM.
Metabolomics is a new study area that focuses on the relationship between genetic variations and environmental triggers of diseases.7 By this method, scientists can determine the precise molecular mechanisms and pathophysiological pathways of diseases, which consequently helps in the early diagnosis of many chronic diseases.8,9 Via metabolomics analysis, disturbances in levels of low-molecular-weight metabolites such as sugars, amino acids, lipids, and nucleotides can be identified, so that potential pathways, upstream enzymes, proteins and targets that are potentially associated with diseases can be reconstructed.10 According to the theory of metabolomics and gratifying progress in biomarker profiling in Chinese populations,11,12 we designed a metabolomics research study that included 64 T2DM patients and 70 healthy participants to determine the potential mechanism of T2DM and offer new insights for formulating diagnostic criteria for T2DM. During this experiment, high-definition mass spectrometry coupled with multivariate analyses was used efficiently, and by means of Ingenuity Pathway Analysis (IPA) new targets were identified systematically.
Experimental work
Ethics statement
Written informed consent was obtained from all subjects. The experimental protocol was reviewed and approved by the Ethical Committee of Heilongjiang University of Chinese Medicine and was conducted according to the principles expressed in the Declaration of Helsinki.
Apparatus and reagents
An ACQUITY UPLC system and a SYNAPT-G2-Si high-definition mass spectrometer from Waters (Milford, MA, USA) were used. Acetonitrile (HPLC grade) was purchased from Merck (Darmstadt, Germany). Methanol for high-performance liquid chromatography was obtained from Fisher Scientific Corporation (Loughborough, UK). Distilled water was purchased from Watson's Food and Beverage Co., Ltd (Guangzhou, China). Formic acid (HPLC grade, FA) was purchased from Aladdin Reagent Co., Ltd (Shanghai, China). Leucine enkephalin was purchased from Sigma-Aldrich (St. Louis, MO, USA). All other reagents were of analytical grade.
Subjects and sample collection
A total of 64 type 2 diabetes patients and 70 healthy participants were enrolled from four different outpatient departments between February 2014 and March 2015, with the informed consent of each participant. The study candidates were all diagnosed via their fasting blood glucose levels, and the patients all had glucose levels that were higher than or equal to 7.0 mmol L−1, which is the threshold in accordance with the 2015 criteria of the American Diabetes Association. All patients underwent differential diagnoses to exclude disturbed or abnormal glucose metabolism in patients due to cirrhosis, chronic renal failure, stress status, acral hypertrophy, Cushing's syndrome, and hyperthyroidism. Consenting patients and healthy participants in this experiment were clinically diagnosed as having type 2 diabetes or not, and the ages of the patients and healthy participants were matched with each other between the ages of 35 and 80 years. Detailed information on the patients and healthy participants was uploaded to the clinical trial management public platform, and partial information on the type 2 diabetes patients is shown in Table S3.† In addition, all candidates strictly conformed to the inclusion and exclusion criteria and were notified that during the week prior to the experiment smoking, alcohol, fast foods containing cheese and preservatives, fruit juice, coffee, tea, chocolate, cola and other caffeinated foods or beverages were forbidden. The exclusion criteria were that patients who had been diagnosed with autoimmune diseases, cancer, infectious diseases, tuberculosis, AIDS, chronic active hepatitis, neuromuscular diseases, and endocrine diseases would be excluded. After a light diet for one week, 5 mL fasting blood samples were collected from the cubital vein in the morning. The blood was allowed to stand for 30 minutes and then immediately centrifuged at 4000 rpm for 30 min at 4 °C. The supernatant was carefully aspirated and then the liquid was transferred into a 1.5 mL centrifuge tube. In order to minimize degradation, the serum supernatants were stored at −80 °C before metabolomics analysis.
Preparation of serum samples and chromatography conditions
Before UPLC/MS analysis, the serum samples were thawed at room temperature, and every sample was prepared by standard procedures and mixed vigorously to remove interference due to macromolecular proteins in our serum samples. A mixture of 200 μL thawed serum and 800 μL methanol was centrifuged at 13
000 rpm for 15 min at 4 °C, and the supernatant (800 μL) was transferred to a new 1.5 mL centrifuge tube and then evaporated to dryness under vacuum in a SpeedVac concentrator at 37 °C. Subsequently, each dried sample was thawed in 100 μL methanol with vigorous vibration for 10 minutes, and 1 μL of the supernatant was injected into the UPLC-MS system. In addition, a pooled sample of all 134 samples was prepared as a QC sample and was dissolved in the same way. The QC sample provided an analyte containing all the samples that would be found during the entire sample sequence and was tested at an average interval of one QC test every 10 injections. Chromatographic separation was undertaken on an ACQUITY UPLC BEH C18 column (50 mm × 2.1 mm, 1.7 μm, Waters Corporation, Milford, USA). The column temperature was maintained at 50 °C and the flow rate was 0.5 mL min−1. The optimal mobile phase contained (A) 0.1% formic acid in acetonitrile and (B) 0.1% formic acid in water, and a linear gradient elution program was performed as follows: 0–0.5 min, 5–20% A; 0.5–2.5 min, 20–60% A; 2.5–4.0 min, 60–66% A; 4.0–5.0 min, 66% A; 5.0–7.5 min, 66–86% A; 7.5–8.0 min, 86–99% A; 8.0–10.0 min, 99% A; 10.0–10.5 min, 99–5% A; 10.5–12.5 min, 5% A. After every sample injection, a needle wash cycle was performed to make sure that the residues were removed and to prepare for the next sample. In addition, the eluent was transferred to the mass spectrometer directly without splitting.
Mass spectrometry
High-definition mass spectrometry was performed with a mass spectrometer system equipped with an electrospray ion (ESI) source, which was controlled by MassLynx software (v4.1, Waters Corporation, Milford, MA, USA). The optimal conditions for analysis were as follows: the parameters were an ion voltage of 3000 V in positive mode and 2500 V in negative mode. The ion source temperature was set at 110 °C, the desolvation gas temperature was 350 °C, the cone gas flow rate was 50 L h−1 and the desolvation gas flow rate was 1000 L h−1. The data acquisition rate was set at 0.2 s per scan, with a delay of 0.1 s between scans. Data were collected in centroid mode from 50 to 1200 Da. For accurate mass acquisition, a lock mass of leucine enkephalin at a concentration of 4 ng μL−1 was used via a lock spray interface at a flow rate of 10 μL min−1 while both ion modes ([M + H]+ = 556.2771, [M − H]− = 554.2615) were monitored to ensure accuracy during MS analysis.
Data processing and multivariate data analyses
The primary data files (formatted as .raw files) for serum samples from T2DM patients and healthy participants were uploaded onto Progenesis QI 1.0 software (Nonlinear Dynamics, 2014, version 1.0) for preprocessing. After peak detection, peak normalization, and auto-alignment, the resulting data matrices were imported into EZinfo 2.0 software for PCA. From these analyses, the variable importance in projection was selected as a standard for screening. For the analysis of the MS/MS fragments, MassFragment™ application manager (Waters Corporation, Milford, MA, USA) was employed. All statistical analyses were performed using Student's t-test. Differences with a p-value of 0.05 or less were considered to be significant. Assays were performed in triplicate, and the results are expressed as the mean ± SD.
Statistical analysis
All data were expressed as the mean ± SD. Student's t-test in SPSS 19.0 for Windows was used for statistical analysis of the biochemical and metabolomics data. PCA was used to reveal unknown trends in the T2DM group. Prediction set modules in the OPLS-DA model were used to show details of unpredictable conditions in patients. For all measurements, a value of p < 0.05 was considered to be statistically significant.
Results
Metabolic profiling analysis
The serum samples were extracted by the UPLC-MS system using a reversed-phase column after careful preparation, and we obtained metabolic profiles of the T2DM patients and healthy participants. The .raw data were imported into the Progenesis QI software, and then a serial workflow comprising vector editing, ion intensity detection, and total ion chromatogram analysis was run to identify the molecular species present in T2DM biomarkers. Peak selection and identification were then carried out after the general conditions were set up. The alignment algorithm generated a 2D ion intensity map, which consisted of approximately 11
068 ion peaks in positive ion mode and 16
581 ion peaks in negative ion mode, according to the normalized data. By importing the data into the Human Metabolome Database (HMDB), we obtained the preliminary results of the analysis, in which 9649 ions matched the detailed information in HMDB in positive ion mode and 9874 ions did so in negative ion mode. A sequential compound statistical analysis was performed, and a .usp profile was obtained for the next step of data processing (Fig. 1).
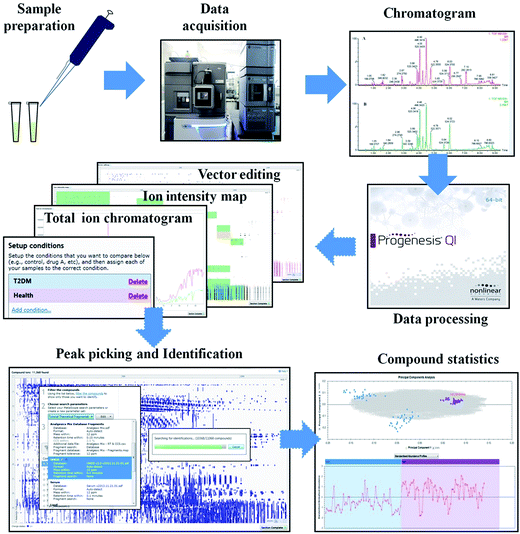 |
| Fig. 1 General workflow used for data processing in this experiment. After the sample preparation and data acquisition, a workflow was run using Progenesis QI software to find potential ion peaks. | |
Multivariate statistical analysis and identification of potential metabolites
Typically, the trajectory analysis of score plots from principal component analysis (PCA) was performed by EZinfo 2.0 software and, according to the results of PCA (Fig. 2A), we found that the metabolic profiles in the different groups could be separated clearly. The results of the corresponding VIP plot based on serum profiling data showed a significant difference in ions between the T2DM and healthy groups (Fig. 2B), and the ions furthest from the origin were regarded as potential biomarkers responsible for the differences between the T2DM and healthy groups. We selected the biomarkers that met a VIP threshold of 1.0 and had a value of p < 0.05 (Student's t-test) as candidates for the next steps. After highly accurate scanning of the MS and MS/MS signals and statistical screening, several biomarkers were imported into MassFragment™ application manager; for example, the chemical structure and mass fragment information of murocholic acid are illustrated in Fig. 2C. Via careful screening against network databases such as MassBank, ChemSpider and the Kyoto Encyclopedia of Genes and Genomes (KEGG) and using the earlier matched results from the HMDB, 33 novel biomarkers were finally tentatively identified, namely, phosphatidylcholines (PCs), sphingomyelins (SMs), lysolecithin phosphatidylcholines (LysoPCs), L-carnitine, cytidine, beta-tyrosine, beta-leucine, cinnamic acid, sorbitol-6-phosphate, 4-hydroxybenzaldehyde, acetyl-N-formyl-5-methoxykynurenamine, L-3-phenyllactic acid, azelaic acid, gentisate aldehyde, 2-phenylethanol glucuronide, taurodeoxycholic acid, deoxycholic acid 3-glucuronide, sphingosine-1-phosphate, cyclic inositol phosphate, 14,15-epoxy-5,8,11-eicosatrienoic acid, palmitic acid, chenodeoxycholic acid, murocholic acid, docosahexaenoic acid, cholesterol sulfate and 17a,21-dihydroxypregnenolone. A clustering heat map (Fig. 3A) illustrated the differences in relative values between the T2DM patients and healthy participants, and a correlation analysis (Fig. 3B) provided a further understanding of the tentatively identified biomarkers. To investigate the magnitudes of the changes in the tentatively identified markers, the relative intensities of the markers were compared between the two groups (Fig. 3C).
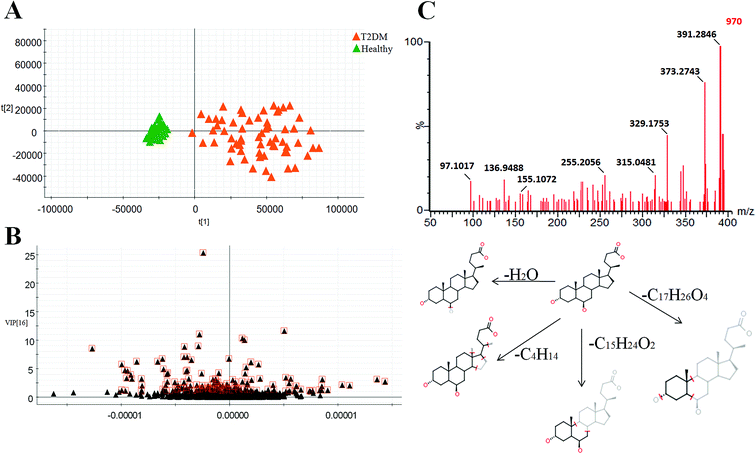 |
| Fig. 2 PCA score plot (A) based on the serum profiles of T2DM patients [orange color] and healthy participants [green color]. VIP plot of the selected biomarkers (B), in which the variables marked by a box are the markers selected as potential biomarkers. (C) Chemical structure and major mass fragments proposed for murocholic acid in negative ion mode. | |
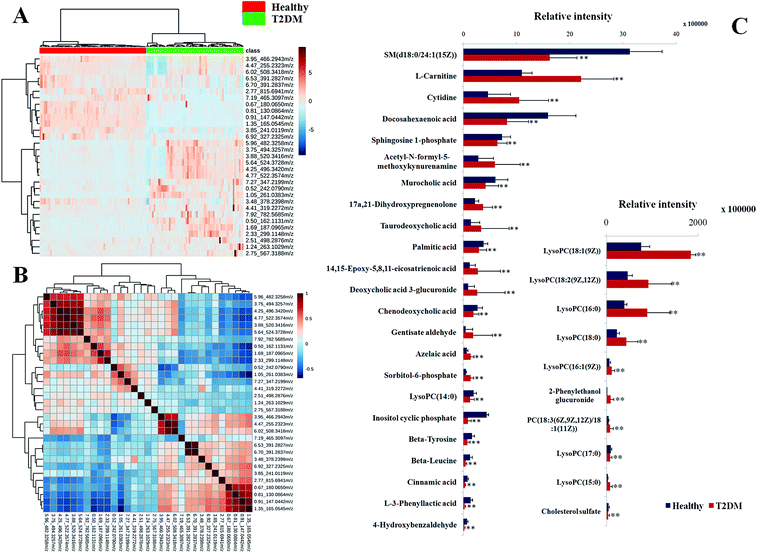 |
| Fig. 3 Heat map (A) of potential metabolites in healthy participants and T2DM patients. Correlation analysis (B) of tentatively identified metabolites in T2DM patients and healthy participants. Column diagram (C) of the relative intensity of potential biomarkers in T2DM patients and healthy participants. The heat map was constructed for potential candidates of importance, which were extracted by OPLS-DA analysis. The color of each section is proportional to the magnitude of the change in the metabolite (red: upregulated; blue: downregulated). | |
Pathway reconstruction
The tentatively identified biomarkers were further imported into the MetaboAnalyst 3.0 website (http://www.metaboanalyst.ca/) and the KEGG (http://www.kegg.jp/kegg/). We found that they were mainly involved in five classes of pathway, namely, lipid metabolism, amino acid metabolism, nucleotide metabolism, carbohydrate metabolism and bile acid metabolism (Fig. 4). An overview of the pathway analysis is shown in Fig. 5A. With impact indices of 0.1037, 0.0353, 0.0296, 0.0279 and 0.0223, the lipid metabolism pathways glycerophospholipid metabolism, sphingolipid metabolism, fatty acid metabolism, and arachidonic acid metabolism and the amino acid metabolism pathway tyrosine metabolism were regarded as the main disordered pathways that were suspected of triggering disordered metabolism in the case of T2DM. In addition, according to the pathway database in the KEGG, potential relationships that focus on the main disordered pathways are reconstructed in Fig. 5B and C. The disordered biomarkers present in these pathways may reflect relationships between different biomarkers and pathways.
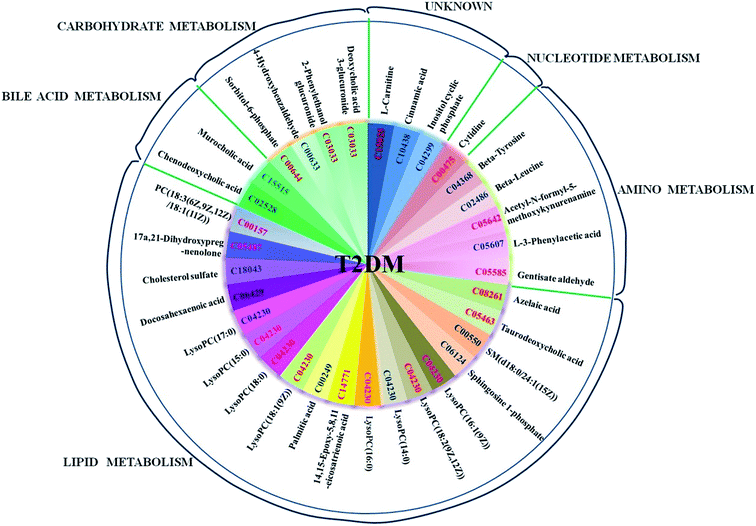 |
| Fig. 4 Distribution map of potential metabolites that are expressed abnormally in T2DM patients. The ensemble ID on each sector represents the KEGG number of the biomarkers that are differentially expressed. The metabolism categories are shown at the edge of the circle. | |
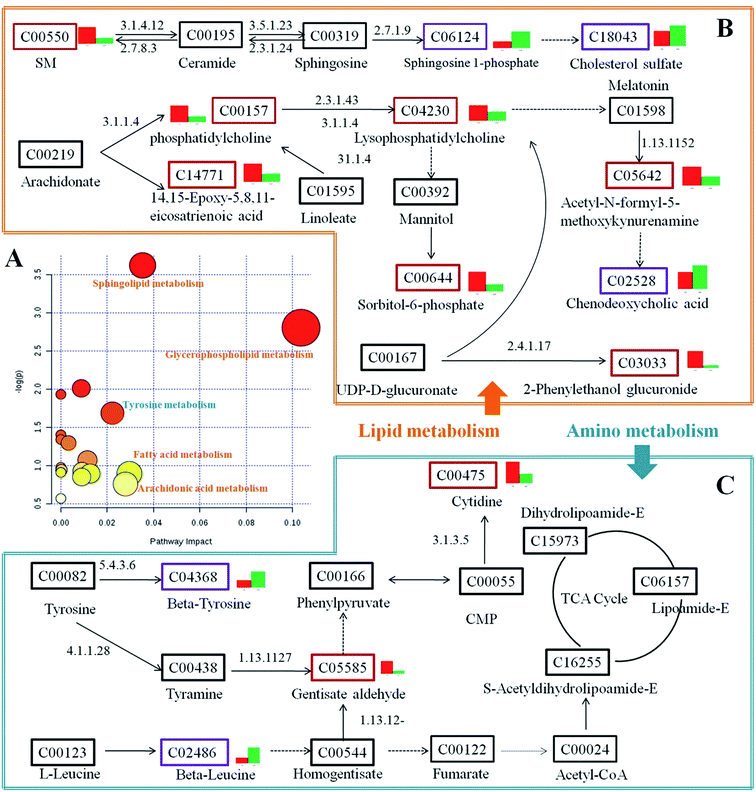 |
| Fig. 5 Reconstructed pathways associated with the biomarkers that are abnormally expressed in T2DM in this study. An overview of the pathway analysis is shown in (A), and the main disordered pathways of lipid metabolism are illustrated within the orange frame in (B), whereas the main disordered pathways of amino acid metabolism are illustrated within the blue frame in (C). In the pathways illustrated in the frames, the KEGG ID of each biomarker is shown. A red box around the KEGG ID indicates that the marker was upregulated in the T2DM patients, whereas a purple box indicates that the marker was downregulated and a black box indicates that the biomarker was not found in our experiment. The numbers on the arrows that connect the biomarkers are Enzyme Commission numbers, and the bar charts to the right of the KEGG boxes show the relative amounts that were expressed in the T2DM patients (red) and healthy participants (green). | |
Prediction of potential targets in type 2 diabetes by IPA
To further analyze detailed functional changes in the T2DM patients, Ingenuity Pathway Analysis (IPA) was utilized. The analysis of the disease and functional changes (Table S1†) revealed that upregulated differentially expressed metabolites were mainly involved in the synthesis of reactive oxygen species, apoptosis of tumor cell lines, concentration of lipids, synthesis of eicosanoids, and the generation and production of reactive oxygen species, whereas downregulated differentially expressed metabolites were mainly involved in the growth of epithelial tissue, cellular homeostasis, cell proliferation of tumor cell lines, synthesis of nucleotides and binding of DNA. According to canonical pathway analysis, seven related pathways (Fig. 6A) and two prediction networks (Fig. 6B and C) were identified. The seven pathways comprised palmitate biosynthesis I, L-carnitine biosynthesis, sphingosine and sphingosine-1-phosphate metabolism, the mitochondrial L-carnitine shuttle pathway, stearate biosynthesis I, PDGF signaling, and ceramide signaling. Among these, sphingosine and sphingosine-1-phosphate metabolism, L-carnitine biosynthesis, and palmitate biosynthesis I were the three main pathways, with p-values of 2.23, 2.20 and 2.04, respectively. According to the prediction networks, we found that 14,15-epoxyeicosatrienoic acid, azelaic acid, chenodeoxycholic acid, cytidine, palmitic acid, sphingosine-1-phosphate, cholesterol sulfate, taurodeoxycholic acid, and lysophosphatidylcholine were the main abnormal biomarkers in the potential disease networks in T2DM patients. Coincidentally, all these substances were abnormally upregulated in patients with T2DM, and these biomarkers could be regarded as predictive targets of T2DM.
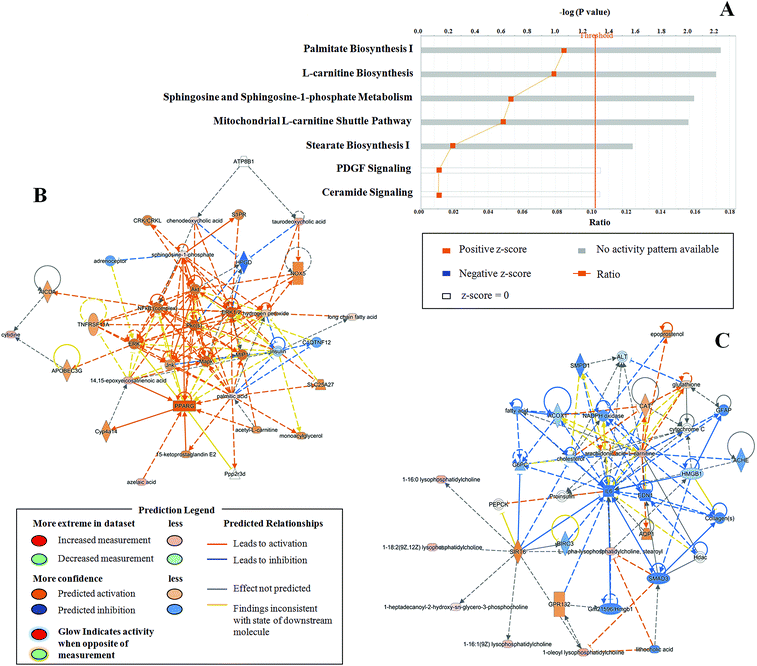 |
| Fig. 6 Most enriched pathways (A) found in this experiment in T2DM patients. IPA prediction networks (B) (C) associated with T2DM patients in this study. Note: orange lines indicate activation and blue lines indicate inhibition. | |
Predictive biomarkers of T2DM provided by metabolomics strategy
Some researchers have suggested that an increase in the area under the receiver operating characteristic (ROC) curve should be the main criterion for measuring the behavior of the key factors associated with the risk of developing disease.13 In our experiment, we tentatively identified 33 biomarkers that were highly correlated with T2DM and, by importing details of the behavior of these biomarkers, which we found in our patients' serum, into the MetaboAnalyst 3.0 website, a biomarker analysis was carried out. After a data integrity check and data normalization, we obtained results of classical univariate ROC curve analyses, and the AUC data of these curves are listed in Table S2.† The area under the ROC curve (AUC) is a summary measure that essentially averages diagnostic accuracy. ROC curves with values of AUC > 0.9 were obtained for several possible biomarkers, and the respective AUC values were 1.000, 0.976, 0.988, 0.999, 0.982, 1.000, 0.937 and 0.958 (Fig. 7). The ROC analysis showed the ability to discriminate T2DM patients from healthy participants and helped distinguish more valuable markers from tentatively identified markers.
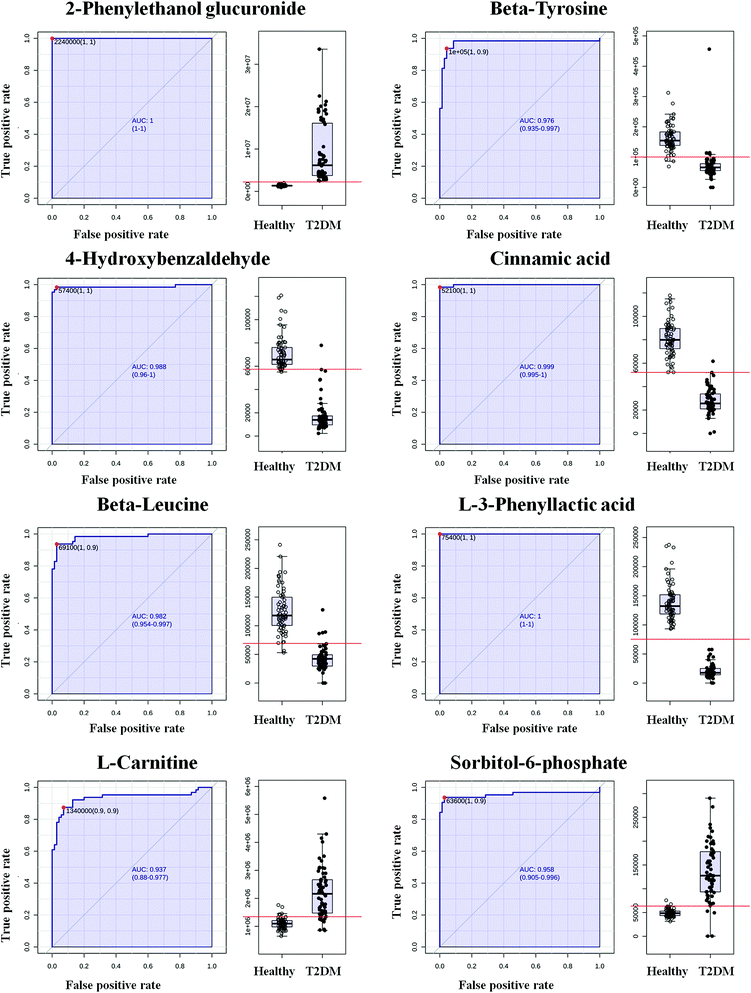 |
| Fig. 7 Evaluation of diagnostic efficacy using ROC curves for metabolites in serum differentially expressed in T2DM patients and healthy participants, as detected by UPLC-MS (AUC > 0.9). | |
Distinguishing diseases using biomarkers
To examine the suitability of our 33 tentatively identified biomarkers, we utilized these biomarkers as a basis for grouping 50 samples, which we randomly selected from all 134 candidates using EZinfo 2.0 software. This was a single-blind experiment, so we did not know whether the sample that we selected was from a T2DM patient or a healthy person. As a result, two clusters were obtained, in which 22 samples were assigned to group 1 and 28 samples were assigned to group 2. By mousing over each sample in a cluster, we obtained detailed information on the sample and found that group 1 was the cluster for T2DM patients and group 2 was the cluster for healthy participants, and the healthy and T2DM groups were sharply divided (Fig. 8). Using this method, we have preliminarily confirmed the usefulness of the selected markers.
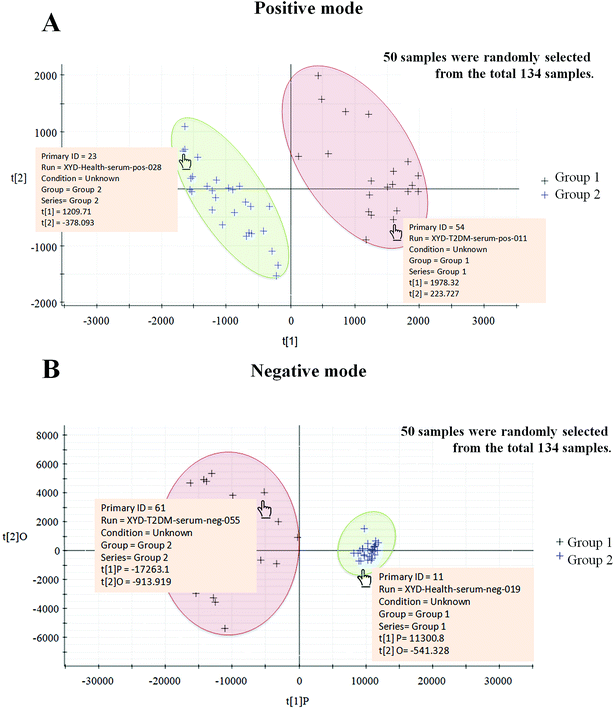 |
| Fig. 8 Thirty-three tentatively identified biomarkers were used as a basis for grouping 50 samples, which we randomly selected from all 134 candidates using EZinfo 2.0 software. The individual samples were unknown. By distinguishing our biomarkers the samples were sharply divided into two groups in both positive mode (A) and negative mode (B). By mousing over the cross corresponding to the sample, detailed information on the sample was presented. Group 1 is the T2DM group and group 2 is the healthy group. | |
Discussion
Type 2 diabetes mellitus is considered to be a complex chronic non-communicable disease that can lead to accelerating senility and premature death. The key criterion for intervening in or curing this disease may be an earlier specific diagnosis or the establishment of evaluation indicators based on the general state of this disease that could comprehensively reflect the characteristic features of T2DM. Here, with the assistance of advanced metabolomics technologies and the profiling of biomarkers of referable diseases that has been carried out in Chinese people,14 we enrolled 64 T2DM patients and 70 healthy participants, who were diagnosed by common criteria. Then, by the analysis of serum phenotypes in T2DM patients, we determined many metabolic biomarkers and disordered pathways, which could be regarded as new routes for discovering potential mechanisms of T2DM.
In patients with T2DM, this disease is often due to a deficiency or dysfunction of insulin, and the abnormally expressed hormone may correspondingly cause increases in blood sugar to a high level. The excess sugar then leads to a variety of functional lesions, in particular in the eyes, kidneys, heart, and blood vessels, nerve damage and chronic dysfunction.15,16 Insulin is a protein hormone secreted by β cells in pancreatic islets in response to stimulation by endogenous or exogenous substances such as glucose, lactose, ribose, arginine, glucagon and so on.17 As an externally secreted protein, it is synthesized in ribosomes18 with subsequent processing in the endoplasmic reticulum and Golgi apparatus. Communication between the endoplasmic reticulum and Golgi apparatus is facilitated by vesicular transport,19 and the final exocytosis from the Golgi apparatus to the extracellular environment also depends on vesicle trafficking.20 A vesicle is a small structure within a cell, which consists of fluid enclosed by a lipid bilayer.21 Depending on the structural origin of ketoacyl or isoprene groups, lipids may be divided into eight categories: fatty acids, glycerolipids, glycerophospholipids, sphingolipids, saccharolipids, polyketides, sterol lipids, and prenol lipids.22 The functional roles of lipids are diverse: they serve as regulatory agents in cell growth and adhesion, participate in the biosynthesis of other biomolecules, and serve to increase the enzymatic activities of enzymes.23 The amphiphilic nature of lipids enables them to act as the bases of structures such as membranes and vesicles. The arrangements of lipids and various proteins, which act as receptors and channel pores in membranes, control the entry and exit of other molecules and ions as part of the cell metabolism. Via our experiment, we concluded that lipid metabolism was a principal disordered pathway in our T2DM patients, and recent studies have found that irregular lipid levels related to diabetic dyslipidemia begin to develop prior to the clinical onset of T2DM at a time when blood glucose concentrations are still at a relatively normal level.24 We inferred that the development of T2DM in our experiment was highly correlated with disordered lipid metabolism in blood serum and that abnormal vesicle trafficking may be the cause of the inadequate production of insulin in T2DM patients, but the specific mechanism remains to be further studied.
Amino acids are collectively defined as organic compounds that contain amino and carboxyl groups. They are the basic units of biological functional macromolecular proteins and the fundamental substances that constitute proteins that are needed for animal nutrition. Several surveys have suggested that amino acids may play an important role in the tricarboxylic acid cycle (TCA cycle).25,26 During our experiment, disordered biomarkers were identified that were involved in amino acid metabolism and the TCA cycle in various ways, and amino acid metabolism was considered to be another principal disordered pathway in our T2DM patients. In the process of decomposition of amino acids into participants in the TCA cycle, they are first broken down into a nitrogen-containing fraction and a non-nitrogenous fraction. The nitrogen-containing fraction is eventually metabolized to urea and then eliminated into the environment, and the non-nitrogenous fraction is finally metabolized to form α-keto acids, which subsequently enter the TCA cycle. Disordered amino acid metabolism may reflect a disordered state of the TCA cycle, which is a physiological phenomenon that plays a central role in the process of the release of stored energy.27 Moreover, many surveys have suggested that insulin also plays an important role in the process of amino acid metabolism28 by promoting the assembly of branched chain amino acids into muscle proteins,29 inhibiting the oxidation of branched chain amino acids in muscle cells, decreasing the uptake of amino acids by the liver, and inhibiting gluconeogenesis.30 When the amount of insulin is insufficient, branched chain amino acids in muscle cells accelerate the oxidation and decrease the uptake of branched chain amino acids in muscle tissues, which leads to a decrease in glucose and protein tolerance in diabetes patients.31 Diabetes mellitus is a disease caused by the combined effects of the internal and external environment, and abnormal metabolism of amino acids can reflect disorders in the metabolism of glucose and lipids to some extent. Our results, which regarded amino acid metabolism and lipid metabolism as principal disordered pathways in T2DM patients, also supported this theory. So far, biomarkers such as lysolecithin phosphatidylcholines (LysoPCs), sphingomyelin (SM), phosphatidylcholine (PC), β-tyrosine, β-leucine, acetyl-N-formyl-5-methoxykynurenamine, and L-3-phenyllactic acid have continually been identified in numerous metabolomics studies of T2DM.32–34 In our experiment we confirmed the predictive function of these biomarkers in 134 human subjects and identified other biomarkers that had not been reported in previous experiments related to T2DM, namely, 4-hydroxybenzaldehyde and cyclic inositol phosphate. 4-Hydroxybenzaldehyde is one of the three isomers of hydroxybenzaldehyde and reacts with NAD+ and H2O to produce 4-hydroxybenzoate, NADH, and two protons. Under catalysis by benzoylformate decarboxylase, it can be generated as an intermediate in the aminobenzoate degradation pathway and is connected to tryptophan metabolism in an indirect way. Tryptophan is an essential amino acid needed in humans to prevent illness or death and, according to research into membrane proteins and model transmembrane peptides,35 residues of tryptophan also play special roles in “anchoring” membrane proteins within the membrane. During our experiment, we considered 4-hydroxybenzaldehyde to be a tentatively identified biomarker that could forecast the development of T2DM. During the ROC curve analyses in our experiment, the AUC value of the corresponding ROC curve was 0.988. This solid response reflects the ability of this marker to predict T2DM. Cyclic inositol phosphate is a substrate for annexin A3, which is a cellular protein and also a substrate for epidermal growth factor receptor tyrosine kinase. Many studies have suggested a subtle association between annexin and cancer.36 As a membrane protein, it acts as an intermediary for signaling, activates downstream signaling components, and acts as a calcium transporter. The disordered state of cyclic inositol phosphate may to a slight extent indicate a disturbance in cell signaling activities or a dysfunction in calcium transport. Chenodeoxycholic acid and murocholic acid are both bile acid metabolites that we found to be abnormally expressed in the serum of T2DM patients during this metabolomics study. They are predominantly found in the hepatoenteral circulation system of mammals and can play an essential role in fat metabolism.37,38 Bile acids are major steroid acids that are synthesized from cholesterol in the liver and are mostly excreted into the small intestine, then reabsorbed in the terminal ileum and finally returned to the liver.39 Bile acids have a wide range of pleiotropic effects, and disordered levels of bile acids indirectly indicate dysfunction of the liver, which could cause serious complications such as fatty liver, hepatomegaly, abnormal liver function, and cirrhosis.40,41 A series of studies have also shown that bile acid metabolism is altered in T2DM patients and, conversely, that manipulation of the bile acid pool can improve glycemic control in such patients.42,43 2-Phenylethanol glucuronide and deoxycholic acid 3-glucuronide are natural human metabolites of 2-phenylethanol and deoxycholic acid, which are generated in the liver by UDP-glucuronyl transferase, and we found them to be significantly upregulated in the T2DM patients in this study. This disordered condition may have links to liver dysfunction, and the liver is one of the most essential organs in the body as it plays key roles in the metabolism of carbohydrate, fat and protein and is also the main site of the action and catabolism of insulin.44,45
In this experiment, we repeated the data collection and processing stages three times and concluded that substances that were consistently present were definite biomarkers. Other biomarkers were also tentatively identified in the serum of T2DM patients in contrast to that of healthy participants, namely, L-carnitine, cytidine, cinnamic acid, sorbitol-6-phosphate, azelaic acid, gentisate aldehyde, taurodeoxycholic acid, and 17a,21-dihydroxypregnenolone. All these markers could act as a reference for the clinical diagnosis or mechanistic study of T2DM, and we tested their usability in a single-blind study using EZinfo. Using our 33 biomarkers as a basis for grouping 50 randomly selected samples, these were sharply divided into clusters corresponding to T2DM patients and healthy participants, which suggests that these biomarkers that we selected could be used as diagnostic criteria in indistinguishable cases.
Conclusions
Diabetes mellitus is a metabolic disease with multiple complications such as diabetic retinopathy, diabetic chronic kidney disease, diabetic neuropathy, hypertension, coronary atherosclerotic heart disease, cerebrovascular disease, and vascular disease of the lower extremities. The earlier we diagnose its incidence, the more effective is the treatment that we can apply. Metabolic profiling of small molecules provides a snapshot of physiological processes, and by using a metabolite profiling platform based on mass spectrometry coupled with pattern recognition analysis, a panel of 33 biomarkers involved in the metabolism of lipids, carbohydrates, and amino acids were tentatively identified in this study. Our experiment yielded substantial new insights into serum metabolism in type 2 diabetes mellitus patients, and these metabolites will be targets for future mechanistic research to help understand the clinical significance of these metabolic abnormalities. Although the precise mechanism of T2DM has not been clearly illustrated at present, the future risk of diabetes was elevated to a greater extent in subjects with such metabolic disorders.
Conflicts of interest
The authors declare no competing financial interests.
Acknowledgements
This work was supported by grants from the Key Program of Natural Science Foundation of State (Grant No. 81430093, 81373930, 81673586, 81302905), National Key Subject of Drug Innovation (Grant No. 2015ZX09101043-005, 2015ZX09101043-011), TCM State Administration Subject of Public Welfare (Grant No. 2015468004), Specialized Research Fund for the Doctoral Program of Higher Education (20132327130001, 20122327120006), Application Technology and Development of Youth Talents Project in Harbin (2014RFQXJ116), and University Nursing Program for Young Scholars with Creative Talents in Heilongjiang Province (UNPYSCT-2015118).
References
- D. J. Hunter and K. S. Reddy, Noncommunicable diseases, N. Engl. J. Med., 2013, 369(14), 1336–1343 CrossRef CAS PubMed.
- R. L'Heveder, T. Nolan and International Diabetes Federation, Diabetes Res. Clin. Pract., 2013, 101(3), 349–351 CrossRef PubMed.
- L. Guariguata, D. Whiting and C. Weil, et al., The International Diabetes Federation diabetes atlas methodology for estimating global and national prevalence of diabetes in adults, Diabetes Res. Clin. Pract., 2011, 94(3), 322–332 CrossRef PubMed.
- J. Weng and P. Pozzilli, Hot topics on diabetes in China, Diabetes/Metab. Res. Rev., 2015, 31(8), 779 CrossRef PubMed.
- The Chinese medical association, Complications Chinese type 2 diabetes prevention guide (2013 edition), Chinese endocrine metabolism, 2014, 30(10), 26–89 Search PubMed.
- J. J. Chamberlain, A. S. Rhinehart and C. F. Shaefer, et al., Diagnosis and Management of Diabetes: Synopsis of the 2016 American Diabetes Association Standards of Medical Care in Diabetes, Ann. Intern. Med., 2016, 164(8), 542 CrossRef PubMed.
- Zhang, A. Zhang and S. Qiu, et al., High-throughput metabolomics approach reveals new mechanistic insights for drug response of phenotypes of geniposide towards alcohol-induced liver injury by using liquid chromatography coupled to high resolution mass spectrometry, Mol. BioSyst., 2016, 13(1), 73–82 RSC.
- A. Zhang, G. Yan and X. Zhou, et al., High resolution metabolomics technology reveals widespread pathway changes of alcoholic liver disease, Mol. BioSyst., 2016, 12(1), 262–273 RSC.
- X. Wang, S. Zhang and A. Zhang, et al., Metabolomics study of type 2 diabetes and therapeutic effects of Tianqijiangtang-capsule using ultra-performance liquid chromatography/electrospray ionization quadruple time-of-flight mass spectrometry, Anal. Methods, 2013, 5(9), 2218–2226 RSC.
- A. Zhang, H. Wang and H. Sun, et al., Metabolomics strategy reveals therapeutical assessment of limonin on nonbacterial prostatitis, Food Funct., 2015, 6(11), 3540–3549 CAS.
- Y. Wang, L. Klarić and X. Yu, et al., The Association Between Glycosylation of Immunoglobulin G and Hypertension: A Multiple Ethnic Cross-Sectional Study, Medicine, 2016, 95(17), e3379 CrossRef CAS PubMed.
- M. Song, F. Zhao and L. Ran, et al., The Uyghur population and genetic susceptibility to type 2 diabetes: potential role for variants in CDKAL1, JAZF1, and IGF1 genes., OMICS: J. Integr. Biol., 2015, 19(4), 230 CrossRef CAS PubMed.
- J. A. Hanley and B. J. Mcneil, The meaning and use of the area under a receiver operating characteristic (ROC) curve, Radiology, 1982, 143(1), 29–36 CrossRef CAS PubMed.
- J. Xie, A. Zhang and X. Wang, Metabolomic applications in hepatocellular carcinoma: toward the exploration of therapeutics and diagnosis through small molecules, RSC Adv., 2017, 7(28), 17217–17226 RSC.
- C. Weyer, C. Bogardus and D. M. Mott, et al., The natural history of insulin secretory dysfunction, and insulin resistance in the pathogenesis of type 2 diabetes mellitus, J. Clin. Invest., 1999, 104(6), 787–794 CrossRef CAS PubMed.
- R. A. Defronzo, Lilly lecture 1987. The triumvirate: beta-cell, muscle, liver. A collusion responsible for NIDDM, Diabetes, 1988, 37(6), 667 CrossRef CAS PubMed.
- J. H. Koeslag, P. T. Saunders and E. Terblanche, A reappraisal of the blood glucose homeostat which comprehensively explains the type 2 diabetes mellitus-syndrome X complex, J. Physiol., 2003, 549(2), 333–346 CrossRef CAS PubMed.
- A. Lernmark, S. J. Chan and R. Choy, et al., Biosynthesis of insulin and glucagon: a view of the current state of the art, Ciba Found. Symp., 1976, 41(2), 7 CAS.
- A. V. Bryksin and P. P. Laktionov, Role of glyceraldehyde-3-phosphate dehydrogenase in vesicular transport from golgi apparatus to endoplasmic reticulum, Biochemistry Biokhimiia, 2008, 73(6), 619–625 CrossRef CAS PubMed.
- M. Mueckler, Insulin resistance and the disruption of Glut4 trafficking in skeletal muscle, J. Clin. Invest., 2001, 107(10), 1211–1213 CrossRef CAS PubMed.
- J. S. Bonifacino and B. S. Glick, The mechanisms of vesicle budding and fusion, Cell, 2004, 116(2), 153–166 CrossRef CAS PubMed.
- E. Fahy, S. Subramaniam and R. C. Murphy, et al., Update of the LIPID MAPS comprehensive classification system for lipids, J. Lipid Res., 2009, 50(suppl), S9–S14 CrossRef PubMed.
- V. S. Sokolov and Y. A. Chizmadzhev, Biomembranes: Molecular Structure and Function, J. Electroanal. Chem., 1989, 276(3), 424–425 CrossRef.
- I. Bo, P. Almgren and T. Tuomi, et al., Cardiovascular Morbidity and Mortality Associated With the Metabolic Syndrome, Diabetes Care, 2001, 24(4), 683–689 CrossRef.
- J. M. Berg, J. L. Tymoczko and L. Stryer, Amino Acids Are Made from Intermediates of the Citric Acid Cycle and Other Major Pathways, W. H. Freeman, 2002 Search PubMed.
- P. Joanny, H. Hillman and J. Corriol, The effect of some amino acids, glycolytic intermediates and citric acid cycle intermediates on the swelling, and the potassium and sodium concentrations, of guinea pig cerebral cortex slices in vitro, J. Neurochem., 2010, 13(5), 371–374 CrossRef.
- B. Futcher, Metabolic cycle, cell cycle, and the finishing kick to Start, Genome Biol., 2006, 7(4), 1–5 CrossRef PubMed.
- P. Felig, Plasma Amino Acid Levels and Insulin Secretion in Obesity, Laboratory Medicine, 1970 Search PubMed.
- M. Monirujjaman and A. Ferdouse, Metabolic and Physiological Roles of Branched-Chain Amino Acids, Adv. Mol. Biol., 2014, 2014 Search PubMed.
- H. Liu, R. Liu and Y. Xiong, et al., Leucine facilitates the insulin-stimulated glucose uptake and insulin signaling in skeletal muscle cells: involving mTORC1 and mTORC2, Amino Acids, 2014, 46(8), 1971–1979 CrossRef CAS PubMed.
- W. W. Winder and B. F. Holmes, Insulin stimulation of glucose uptake fails to decrease palmitate oxidation in muscle if AMPK is activated, J. Appl. Physiol., 2000, 89(6), 2430–2437 CAS.
- F. Xu, S. Tavintharan and C. F. Sum, et al., Metabolic signature shift in type 2 diabetes mellitus revealed by mass spectrometry-based metabolomics, J. Clin. Endocrinol. Metab., 2013, 98(6), 1060–1065 CrossRef PubMed.
- Y. Yan, Q. Wang and W. Li, et al., Discovery of potential biomarkers in exhaled breath for diagnosis of type 2 diabetes mellitus based on GC-MS with metabolomics, RSC Adv., 2014, 4(48), 25430–25439 RSC.
- D. M. Van, J. Vogels and A. Tas, et al., Evaluation of metabolite profiles as biomarkers for the pharmacological effects of thiazolidinediones in Type 2 diabetes mellitus patients and healthy volunteers, Br. J. Clin. Pharmacol., 2010, 63(5), 562–574 Search PubMed.
- J. D. Schaechter and R. J. Wurtman, Serotonin release varies with brain tryptophan levels, Brain Res., 1990, 532(1), 203–210 CrossRef CAS PubMed.
- M. Schostak, G. P. Schwall and S. Poznanović, et al., Annexin A3 in urine: a highly specific noninvasive marker for prostate cancer early detection, J. Urol., 2009, 181(1), 343–353 CrossRef CAS PubMed.
- B. Angelin, K. Einarsson and K. Hellström, et al., Effects of cholestyramine and chenodeoxycholic acid on the metabolism of endogenous triglyceride in hyperlipoproteinemia, J. Lipid Res., 1978, 19(8), 1017 CAS.
- P. Lefebvre, B. Cariou and F. Lien, et al., Role of bile acids and bile acid receptors in metabolic regulation, Physiol. Rev., 2009, 89(1), 147–191 CrossRef CAS PubMed.
- D. W. Russell, The Enzymes, Regulation, and Genetics of Bile Acid Synthesis, Annu. Rev. Biochem., 2003, 72(1), 137 CrossRef CAS PubMed.
- Y. Li, K. Jadhav and Y. Zhang, Bile acid receptors in non-alcoholic fatty liver disease, Biochem. Pharmacol., 2013, 86(11), 1517 CrossRef CAS PubMed.
- R. E. Poupon, Y. Chrétien and R. Poupon, et al., Serum bile acids in primary biliary cirrhosis: effect of ursodeoxycholic acid therapy, Hepatology, 1993, 17(4), 599–604 CrossRef CAS PubMed.
- B. Staels and F. Kuipers, Bile acid sequestrants and the treatment of type 2 diabetes mellitus, Drugs, 2007, 67(10), 1383 CrossRef CAS PubMed.
- J. J. Holst and M. A. Mcgill, Potential new approaches to modifying intestinal GLP-1 secretion in patients with type 2 diabetes mellitus: focus on bile acid sequestrants, Clin. Drug Invest., 2012, 32(1), 1–14 CrossRef CAS PubMed.
- R. Carlisle, J. T. Galambos and W. D. Warren, The relationship between conventional liver tests, quantitative function tests, and histopathology in cirrhosis, Dig. Dis. Sci., 1979, 24(5), 358 CrossRef CAS PubMed.
- M. Kobayashi, H. Ikegami and T. Fujisawa, et al., Prevention and treatment of obesity, insulin resistance, and diabetes by bile acid-binding resin, Diabetes, 2007, 56(1), 239–247 CrossRef CAS PubMed.
Footnote |
† Electronic supplementary information (ESI) available. See DOI: 10.1039/c7ra05722a |
|
This journal is © The Royal Society of Chemistry 2017 |