DOI:
10.1039/C7RA01305A
(Paper)
RSC Adv., 2017,
7, 37612-37626
Computational analysis revealing that K634 and T681 mutations modulate the 3D-structure of PDGFR-β and lead to sunitinib resistance†
Received
31st January 2017
, Accepted 14th July 2017
First published on 31st July 2017
Abstract
Platelet-derived growth factor receptor-beta (PDGFR-β) is expressed by endothelial cells (ECs) of tumor-associated blood vessels and regulates primarily early hematopoiesis. Human PDGFR-β is a novel therapeutic target for the treatment of glioblastoma. However, a major challenge of glioblastoma therapy is to overcome drug resistance, mostly initiated by missense mutations in the protein kinase catalytic domain. The present work aimed to carry out in silico structural studies on wild-type (WT) and some major mutant-type (MT) PDGFR-β complexes to elucidate the probable mechanism of its resistance related to the anti-angiogenic and anticancer drug sunitinib. In the absence of a crystal structure, the 3D structures of WT and MT PDGFR-β kinase were predicted using homology modeling followed by docking analysis with sunitinib. Molecular dynamics simulations of WT and MT PDGFR-β complexes with sunitinib were performed to understand the differential structural alterations in the PDGFR-β kinase structure, as well as its stability. Our results showed that the overall effect of mutations in the residues K634A, T681M, T681F, T681I, and T681A led to the destabilized 3D structures of PDGFR-β and altered their binding affinities with sunitinib. In particular, the mutation at the gatekeeper residue threonine 681 (T681M), present in the ATP binding pocket, majorly affected the protein stability, thus conferring resistance to the drug sunitinib. Our present findings utilizing in silico approaches show that the differential binding of sunitinib with WT and MT proteins leads to resistance being developed in sunitinib chemotherapy.
1. Introduction
Human PDGFR-β (platelet-derived growth factor receptor-beta) belongs to the receptor tyrosine kinase-III (RTK-III), which is originally assembled under the umbrella of the platelet-derived growth factor receptor (PDGFR) family,1 which includes a number of other members, such as PDGFR-α, CSF, Fms, Flt3, and Kit.2 The structural features of PDGFRs comprise an extracellular portion of five immunoglobulin (Ig) domain segments, a transmembrane domain, a juxtamembrane domain, and an intracellular portion, containing a split protein tyrosine kinase domain or a kinase insert domain, following a C-terminal cytoplasmic tail.3 In the RTK family, the human kinases share a large similarity in their three dimensional (3D) structures, particularly regarding the catalytic kinase domain, consisting of an amino or N-terminal lobe, including a five-stranded β sheet and one α helix. The larger carboxy or C-terminal lobe is mainly α-helical. The highly conserved amino acid residues in the kinases comprise several glycines in the nucleotide-binding loop (nb-loop), a lysine residue in the 3rd β-strand, a glutamic acid in the C-terminal α-helix, an asparagine and aspartic acid in the catalytic loop, and an Asp-Phe-Gly (DFG) motif region in the initiation region of the activation loop.4 The extremely conserved adenosine tri-phosphate (ATP) binding site lies in the cleft between the N- and C-terminal lobes.5–7
Extensive studies over the last two decades have shown that the PDGFs and PDGFRs are involved in human cancer, such as glioblastoma, by progression through the autocrine and paracrine stimulation of tumor cell growth.8 In addition to autocrine stimulation, PDGF signaling applies paracrine stimulation on stromal cells' tumor-associated angiogenesis.8 In human glioblastoma, PDGFR-α is expressed by glioma tumor cells of all grades, while PDGFR-β is reported to be expressed by endothelial cells (ECs) of tumor-associated blood vessels9 and is more pronounced in high grade, in comparison to low grade, glioblastoma.8 PDGFR-β plays a key role in early hematopoiesis and blood vessel formation,8 and is mainly expressed in vascular smooth muscle and pericytes.10 The increased expression of PDGF/PDGFRs in glial tumor cells and human tumors correlates with higher-grade tumors. This indicates both the autocrine and paracrine actions of PDGF and PDGFRs in tumor progression and angiogenesis. To date, the molecular mechanisms of angiogenesis triggered by PDGFB/PDGFR-β are not completely understood.9
Previous studies suggested that the essential residues required for the recognition of PDGFR-β antagonists are located in the tyrosine kinase domain. Targeting this catalytic region with ATP competitive inhibitors appears to offer a promising approach for drug intervention. However, access to the intracellular targets and inhibition selectivity remain major obstacles with this approach.11 Numerous anticancer or anti-angiogenic FDA-approved small-molecule inhibitors have been developed as PDGFR antagonists, which mainly differ in their selectivity and effectiveness.12 Moreover, the molecular structure of this receptor and the mechanism of ligand–receptor interactions remain poorly understood due to the absence of X-ray structural data of PDGFR-β.13 Therefore, taking the aforementioned reasoning into account, the 3D structure of PDGFR-β kinase domain was predicted in the present study using a molecular modeling approach, which was efficiently utilized for further elucidating the structural and functional information of the protein.14
Mutagenesis study in the binding site of the PDGFR-β kinase domain allowed determination of the amino acids predominantly involved in the ligand binding.15–17 This site-directed mutagenesis experiments study in PDGFR-β suggested the role of antagonist binding and the importance of the receptor subtype selectivity.18,19 The site-directed mutations study in the kinase domain of WT PDGFR-β was carried out to predict the mutant K634 and T681 in the PDGFR-β structure in order to understand the impact of mutations on protein function and its interactions with potential inhibitors. The molecular docking study of PDGFR-β was carried with the potential kinase inhibitor sunitinib to evaluate the modulation of the 3D structure due to mutation of the single amino acid residue K634 and T681. Furthermore, we performed MD simulations of WT and MT PDGFR-β to assess the biophysical impact on the function of the receptor after mutation, which ultimately leads to the development of sunitinib resistance.
2. Results and discussion
2.1 Homology modeling of WT and MT PDGFR-β
We selected the protein sequence of the kinase domain of human PDGFR-β having 363 residues (amino acids 600–962) from UniProtKB and predicted its structure to define the ATP binding site. The theoretical model of the kinase domain of PDGFR-β was predicted earlier (PDB ID: 1LWP) having 363 residues; however, the ATP binding site was not well established. The 1LWP model showed the crucial residues involved in ATP interaction that lie at the peripheral region of protein (Fig. S2A†).
The multiple template selection approach was used to improve the quality of the modeled protein.20,21 The three selected template structures, namely VEGFR2 (PDB ID: 1VR2), FGFR1 (PDB ID: 1FGI), and IGF (PDB ID: 1IRK), showed 55%, 54%, and 40% sequence homology with the PDGFR-β kinase domain (600–962), respectively. These templates were structurally aligned to define the structurally conserved regions (SCRs) and were then further aligned to the conserved residues with PDGFR-β (600–962), which were defined using Clustal-X (2.1), as shown in Fig. S1.†
MODELER 9v8 was used to carry out the homology modeling. We generated 100 models to maintain a high level of optimization and to sustain the spatial restraints in term of the protein density function (PDF). The final predicted model was selected on the basis of a high value of a negative DOPE score22 (Fig. 1A). The DOPE score indicated that the predicted model was almost similar in terms of the overall folding pattern of the templates. The overall folding pattern specifies the folds, loop regions, and mainly the binding site, which were almost similar between the target and template crystallographic structures, thus showing the conserved amino acid residues involved in the receptor–antagonists interactions (Fig. 1B). Noticeably, the structural comparison showed almost similar folding patterns as the ATP binding site domain (Fig. 1B).
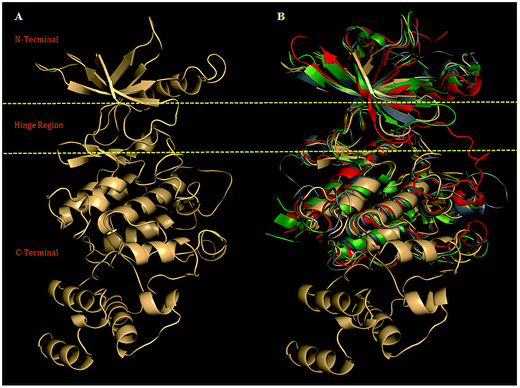 |
| Fig. 1 (A) Model (ribbon view) showing N-terminal, Hinge region, and C-terminal, was selected for refinement using biophysical filtration. (B) The structural alignment of PDGFR-subtypes with the crystal structure VEGFR-2 (PDB ID: 1VR2, green), FGFR-1 (PDB ID: 1FGI, red) and human insulin receptor (PDB ID: 1IRK, gray), with structurally superimposed rmsd values: (1.06 Å), (0.87 Å), and (2.0 Å) for PDGFR-β. | |
2.2 Stereochemical evaluation of PDGFR-β
The stereochemical properties of the modeled structure on the SAVS server exhibited that 89.0% of the PDGFR-β residues were present in the most favored regions (A, B, L) of the Ramachandran plot, while 0.6% of the residues of PDGFR-β were present in a disallowed region. The stereochemical results of the present PDGFR-β model showed improved parameters in comparison to the earlier reported modeled structure (PDB ID: 1LWP) (Fig. S3 and Table S1†).
2.3 Assessment of the stereochemical properties
The overall quality of the PDGFR-β model was further validated by considering the Z-score (ProSA-Web server), representing the total quality index compared to 1LWP, and the non-bonding interaction between each amino acid in the 3D structure, thus providing an overall quality factor compared to 1LWP via an ERRAT2 plot (<95% cutoff). We observed that the currently modeled structure of PDGFR-β is well within the range of a high-quality model in comparison to 1LWP. The result demonstrated that the total quality index (Z-score) was −6.71 for PDGFR-β and −5.66 for 1LWP (Fig. 2). Moreover, the ERRAT2 score was 82.566 for PDGFR-β (Fig. S4A†) and 34.648 for 1LWP (Fig. S4B†). The coordinates of the predicted human PDGFR-β structure (PMDB ID: PM0079890) protein was submitted to the Protein Model DataBase (PMDB) and can be accessed from (http://mi.caspur.it/PMDB).
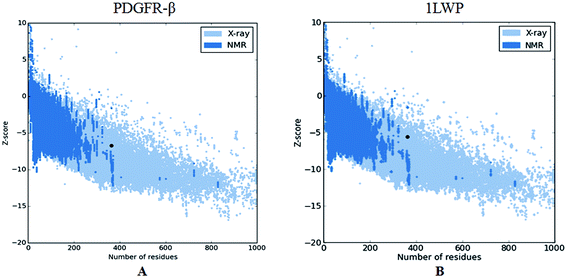 |
| Fig. 2 Overall model quality of PDGFR-β and 1LWP on the basis of Z-scores of all the experimentally determined protein chains in the current PDB structures solved by X-ray or NMR. The Z-score is −6.71 for PDGFR-β and −5.66 for 1LWP. This plot can be used to check whether the Z-score of these 3D structures is within the range of scores typically found for native proteins of a similar size. | |
2.4 Structural refinement of WT and MT PDGFR-β by MD simulations
In order to refine the WT and MT PDGFR-β models, MD simulations were carried out to access the dynamic stability and structural behavior of the protein. The data trajectory files for the MD simulations were collected over a simulation time period of 80 ns.
The analysis based on g_rms with Cα backbone atoms showed a stable conformation, which was maintained throughout the simulation time of 80 ns (Fig. 3A). PDGFR-β WT and MT (K634A) attained stable conformations in 10 ns, with an initial drift of ∼0.25 nm. The stable equilibrium of PDGFR-β was continuously maintained up to the end of the simulation time of 80 ns. The RMSD plot of mutant K634A showed a small drift of ∼0.15 nm as compared to the RMSD value of WT up to the end of the simulation. However, the other mutants T681M, T681F, T681I, and T681A did not show any significant deviation as compared to the WT model (Fig. 3A). The magnitude of fluctuation with a minor change in the average RMSD value of the Cα backbone atoms strongly suggests a stable dynamic behavior of the PDGFR-β WT and MT structures.
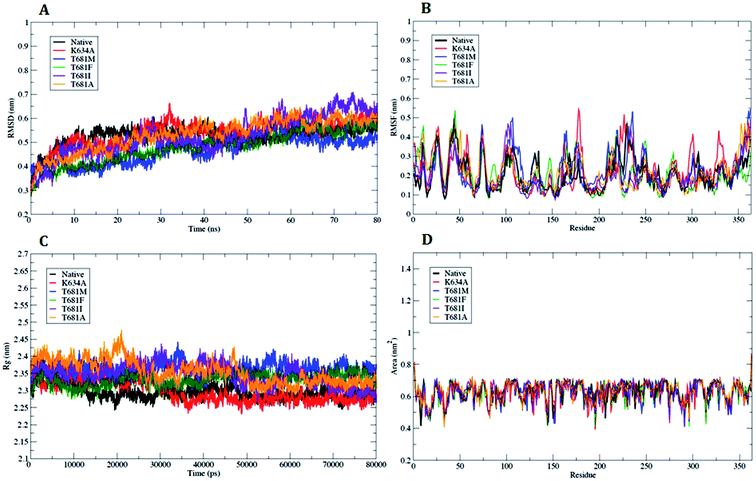 |
| Fig. 3 Analysis with RMSD, RMSF, Rg and SASA. (A) Plot of time vs. RMSD trajectory of WT and MT PDGFR-β protein models at 80 ns. (B) RMSF plot for WT and MTPDGFR-β. (C) Time evolution of the radius of gyration (Rg) value for WT and MT PDGFR-β. (D) Solvent accessible surface area (SASA) plot for WT and MT PDGFR-β. WT (black), mutant (MT) K634A (red), T681M (blue), T681F (green), T681I (violet), and T681A (orange). | |
Analysis of the flexible regions based on g_rmsf results showed the average position of fluctuations of all the Cα atoms of the amino acid residues with respect to the PDGFR-β WT and MT structures. The RMSF plot showed a higher fluctuation in mutant K634A as compared to WT protein, which occurred mainly in the loop regions of the protein, with a maximum peak at around 0.54 nm (Fig. 3B). However, in the case of mutants T681M, T681F, T681I, and T681A, no significant fluctuation was observed when compared to WT protein (Fig. 3B). In conclusion, the RMSF plot confirmed that the fluctuations of the amino acid residues are more pronounced in loop regions and less pronounced in the secondary structure conformations.
The radius of gyration (Rg) is an effective tool to measure the structural compactness, shape, and folding of the overall PDGFR-β WT and MT structures at different time points. It can be described as the mass weighted root-mean-square distance of a group of atoms from their common center of mass. We performed Rg analysis to observe the conformational alterations and dynamic stability of the PDGFR-β WT and MT structures. The average calculated value of Rg from the trajectory is shown in (Fig. 3C). Throughout the simulation, the WT and MT models (K634A, T681F, T681M, T681I, and T681A) exhibited almost a similar pattern in terms of the Rg values, suggesting there was no change in the overall structure and folding of the protein after mutation.
In addition, we also examined the solvent accessible surface area (SASA) to explore the behavior of the hydrophilic and hydrophobic residues of the PDGFR-β WT and MT structures. The SASA results showed that the amino acid residues of the MT structures (K634A, T681F, T681M, T681I, and T681A) have a similar or lower SASA value as compared to WT PDGFR-β protein, and they retained the accessibility during a simulation time of 80 ns (Fig. 3D).
However, there were no significant conformational changes registered during the whole simulation run time. These results were found to be consistent with the secondary structure analysis with the DSSP module using the do_dssp23 command (Fig. 4), and, consequently, this provides clear evidence that the secondary and tertiary structure conformation (α-helix and β-sheets) of the PDGFR-β WT and MT structures remained stable during the simulation.
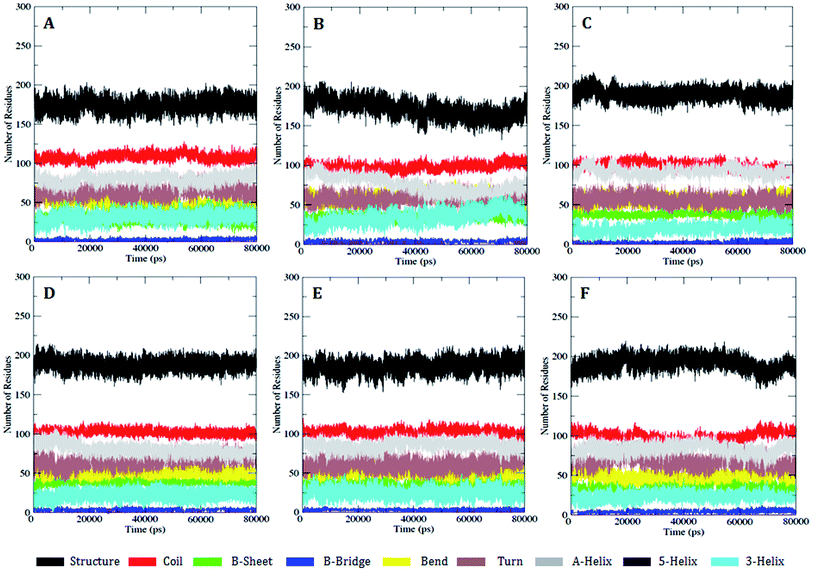 |
| Fig. 4 DSSP analysis for the secondary structure fluctuations as a function of time from 0 to 80 ns for WT and MT PDGFR-β at 300 K. (A) WT, (B) K634A, (C) T681M, (D) T681F, (E) T681I, and (F) T681A. | |
2.5 Prediction of the PDGFR-β binding site
All the protein kinases share a common catalytic domain, containing a cleft, where ATP binds and is thus recognized as the ATP binding site, and which is the major focus of small-molecule drug design for protein kinases.24 To validate the exact coordinates of the ATP binding site for PDGFR-β, which shares a key motive (i.e., hinge region), as shown in (Fig. 1), and contains similar features and is highly conserved in all the RTKs,24 the center of mass was calculated for the cavity to proceed as the active site for the docking analysis. Finally, on the basis of the minimum binding energy (ΔG) score and maximum residual interactions with sunitinib, we chose the ATP binding site for the competitive binding of sunitinib for the various MT proteins (Table S2†).
2.6 Docking analysis of WT and MT PDGFR-β
The residue surrounding the binding site of PDGFR-β comprised the residues Lys634 in the 3rd β-strand (ATP binding site), Thr681 (gatekeeper region), and Glu682, Tyr683, and Cys684 (hinge region) (Fig. 5). The importance of the residues located in the ATP binding site of PDGFR-β especially in the gatekeeper and hinge regions were confirmed by the in silico site-directed mutagenesis data for the PDGFR-β.15–17 We also considered the data from other diverse RTKs that shared a high homology, especially with the catalytic site of the tyrosine kinase domains.4 The reported data from the mutational studies with PDGFR-β suggest that Lys634 (ATP site) and Thr681 (gatekeeper) are essential in the PDGFR-β antagonist interaction.15–17
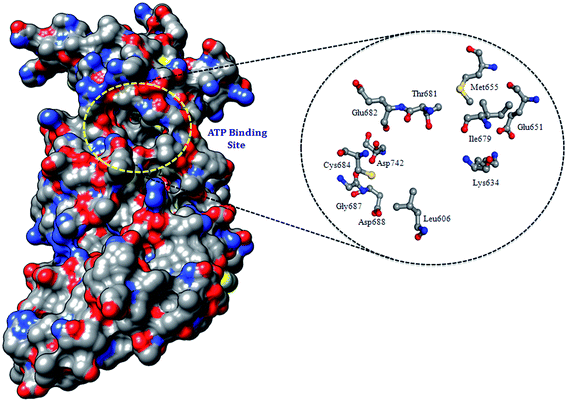 |
| Fig. 5 Surface view of the predicted structure of PDGFRB with the ATP binding site (highlighted by yellow circle); (inset) binding site amino acid residues are shown in a ball and stick format with standard atom colors. | |
The mutagenesis study of the WT and MT PDGFR-β for the residues K634A, T681F, T681A, T681M, and T681I and molecular docking analysis were used to validate the function. The binding energy (ΔG) and inhibition constant (Ki) for WT PDGFR-β were −12.94 Kcal mol−1 and 0.32 nM, respectively. A comparative binding study of WT and MT PDGFR-βwith sunitinib showed that the mutant T681M showed a weak interface interaction with ΔG = −5.80 Kcal mol−1 and Ki = 55.75 μM as compared to the mutants T681A (ΔG = −6.00 Kcal mol−1, Ki = 40.32 μM), T681F (ΔG = −6.24 Kcal mol−1, Ki = 26.55 μM), T681I (ΔG = −7.14 Kcal mol−1, Ki = 5.81 μM), and K634A (ΔG = −7.25 Kcal mol−1, Ki = 4.87 μM), respectively (Fig. 6).
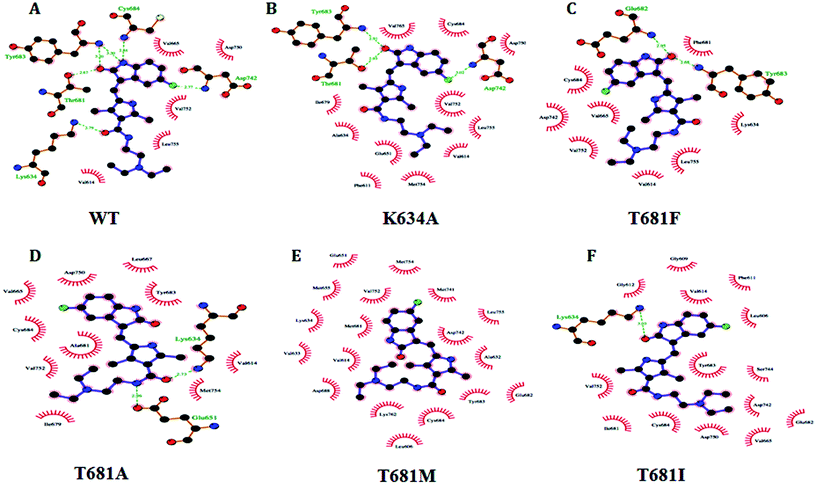 |
| Fig. 6 Amino acid residual interactions of the protein–ligand interface in PDGFR-β: (A) WT, (B) MT K634A, (C) MT T681F, (D) MT T681A, (E) MT T681M, and (F) MT T681I. The color-coding schemes are as follow: the residues of PDGFR-β (WT/MT) in green color, sunitinib in purple color. The hydrogen bond interactions are represented by dashed lines. The amino acid residues involved in the hydrophobic interactions are shown as starbursts. | |
The results from the molecular docking analysis of sunitinib with MT PDGFR-β showed a lower hydrogen bond interaction as compared to WT PDGFR-β. Similarly, the free binding affinity of sunitinib showed a higher value with WT as compared to MT. The results are summarized in detail in Table 1. Five mutant models (K634A, T681F, T681A, T681M, and T681I) were generated and residual interaction analysis was carried out with them and sunitinib. The docking analysis with sunitinib showed five different conformations and spatial orientation at the different sites of all five of the mutants (Fig. 6). The binding energy values in MT PDGFR-β with sunitinib were significantly lower than in the WT.
Table 1 Molecular docking analysis results of wild-type (WT) and mutant-type (MT) PDGFR-β with sunitinib by Autodock 4.2
Protein model |
Binding energy, ΔG (Kcal mol−1) |
Ki observed (nM μM−1) |
Hydrogen bonding |
H-bond variation (WT–MT) (Å) |
Residues |
Distance (Å) |
Wild type |
−12.94 |
0.32 (nM) |
K634 |
2.79 |
— |
T681 |
2.67 |
— |
Y683 |
3.20, 3.30 |
— |
C684 |
2.84 |
— |
D742 |
2.77 |
— |
K634A |
−7.25 |
4.87 (μM) |
T681 |
2.93 |
−0.26 |
Y683 |
2.91 |
0.38 |
D742 |
3.02 |
−0.25 |
T681F |
−6.24 |
26.55 (μM) |
E682 |
2.95 |
— |
Y683 |
2.68 |
0.61 |
T681A |
−6.00 |
40.32 (μM) |
K634 |
2.73 |
0.05 |
E651 |
2.96 |
— |
T681M |
−5.80 |
55.75 (μM) |
— |
— |
— |
T681I |
−7.14 |
5.81 (μM) |
K634 |
2.94 |
−0.15 |
The WT complexed with sunitinib formed five hydrogen bonds with the residues K634, T681, Y683, C684, and D742 (Table 1). These five hydrogen bonds were disrupted in T681M, resulting in an absence of hydrogen bond interactions being formed in the complex structure (Fig. 6E); whereas, K634A, T681F, T681A, and T681I formed three, two, two, and one hydrogen bonds, respectively. K634A showed interactions with the T681, Y683, and D742 residues, T681F with the E682 and Y683 residues, T681A with the K634 and E651 residues, while T681I showed only one interaction with the K634 residue, respectively. The overall changes in the neighboring amino acid residues of the WT and MT PDGFR-β complexes with sunitinib are depicted in Fig. S5.† These results demonstrated that sunitinib exhibited a stronger binding with WT PDGFR-β as compared to the MT proteins. The MT model T681M showed the least binding affinity with sunitinib, which was also confirmed by the docking studies (Fig. 6).
2.7 Molecular dynamic simulation analysis of WT and MT PDGFR-β
Based on the results of the docking analysis, MD simulations were performed with the WT and MT PDGFR-β models, and the dynamic behavior of the protein was analyzed. In the amino acid trajectories, no significant drift was seen to originate from the PDGFR-β structures in the MD simulations. The PDGFR-β protein structures WT and MT could be aligned with the backbone atoms' root-mean-square deviation (RMSD) below 3.5 Å. We observed that the trajectories were more stable under 3.5 Å from the initial point of a native or WT trajectory up to 80 ns. These trajectories were thus considered for further analysis. The mutant T681M showed the maximum deviation till the end of the simulation, resulting in a backbone RMSD of ∼0.20 to 0.65 nm (Fig. 7A), respectively. However, the mutants K634A, T681F, T681I, and T681A did not show a significant deviation from the WT model up to the end of the simulation period (Fig. 7A).
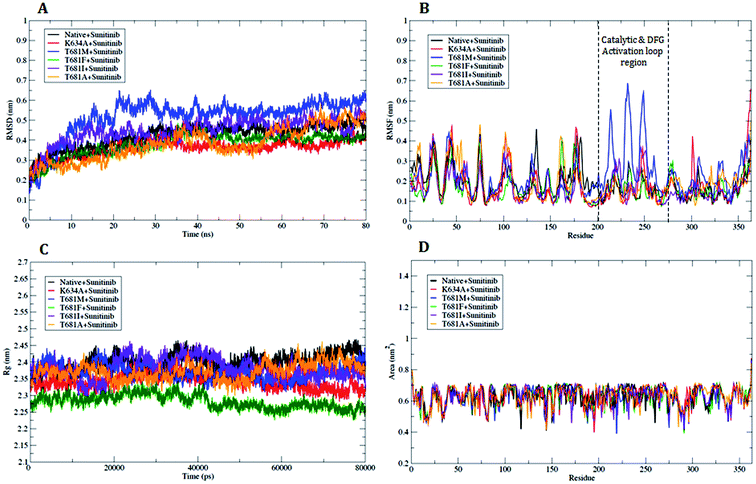 |
| Fig. 7 Analysis with RMSD, RMSF, Rg and SASA. (A) Plot of time vs. RMSD trajectory of WT and MT PDGFR-β protein models at 80 ns. (B) RMSF plot for WT and MT PDGFR-β. (C) Time evolution of the radius of gyration (Rg) value for WT and MT PDGFR-β, (D) solvent accessible surface area (SASA) plot for WT and MT PDGFR-β. WT (black), mutant (MT) K634A (red), T681M (blue), T681F (green), T681I (violet), and T681A (orange). | |
To understand the mutational effect on the flexible areas of the protein structure, all the Cα atom time evolution plots of the root-mean-square fluctuation (RMSF) were analyzed. The RMSF result indicated that there was a higher fluctuation in the MT protein T681M as compared to the WT protein. This observation was also consistent with the other MT models throughout the course of the simulation. The T681M mutation affected the fluctuations in the adjacent residues at a maximum distance of ∼0.642 nm. This result demonstrated a gain in flexibility due to the mutation (Fig. 7B). Also, the increased flexibility of the backbone of T681M significantly affected the overall binding of sunitinib with the MT PDGFR-β model. The RMSF distribution for sunitinib bound to the WT and MT models for K634A, T681F, T681I, and T681A showed a consistently distributed fluctuation (Fig. 7B). Overall, these results demonstrate that the structural deviations in the MT model T681M were significantly altered as compared to the WT and other MT models.
We further carried out a secondary structure analysis to define the structural stability of the WT and MT (Fig. 8). The results showed that the stable conformation of WT was maintained throughout the simulation (Fig. 8A). All the α-helices were found to be persistent and transient, with turns and bends in the first few primary residues, thus allowing us to elucidate the stability of protein. In the case of the MT models K634A, T681M, T681F, T681I, and T681A, they appeared to slightly increase in size compared to WT, resulting in a more flexible nature of the protein (Fig. 8B–F). This inspection was in complete favor with the results of the RMSF investigation.
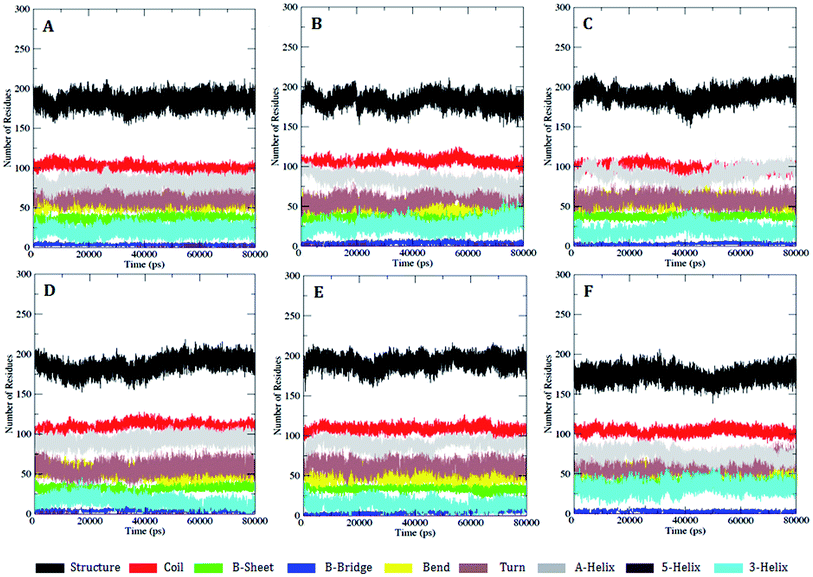 |
| Fig. 8 DSSP analysis for the secondary structure fluctuations as a function of time from 0 to 80 ns for WT and MT PDGFR-β at 300 K: (A) WT, (B) K634A, (C) T681M, (D) T681F, (E) T681I, and (F) T681A. | |
The radius of gyration (Rg) can be designated as the mass weighted root-mean-square distance of atoms from their center of mass. The competence, shape, and folding of the overall PDGFR-β structure at different time point scales throughout the trajectory can be seen in the plot of Rg (Fig. 7C). Throughout the simulation, the MT models K634A, T681F, T681M, T681I, and T681A displayed a similar pattern in terms of Rg value, out of which the mutant T681M showed the highest deviation with an Rg score of ∼2.44 nm (Fig. 7C).
Further, we also examined the compactness of the hydrophobic core region of the WT and MT models by investigating the change in solvent accessibility surface area (SASA). No significant change in SASA of the WT and MT protein model with time was observed (Fig. 7D). For the MT proteins, this suggests that there was no shift of hydrophobic contact between the amino acid residues from the solvent accessible area to the buried state. The T681M mutation had slightly lower values of SASA when compared to WT, and when compared to the K634A, T681F, T681I, and T681A mutations, it remained undistinguished throughout the simulation, thus explaining the influence of the mutation on the protein structure.
The number of hydrogen bonds pattern in the WT and MT structures during the MD simulations was also calculated by hydrogen bond (HB) plot analysis. The HB plot presents the characteristic patterns of the secondary structure elements, where helices can be recognized as strips directly adjacent to the diagonal, while antiparallel beta strands can be recognized by the strips perpendicular to the diagonal, and parallel beta strands by the off-diagonal strips parallel to the diagonal.25 As suggested by Bikadi et al., the tertiary interaction points connecting the perturbation of a hydrogen bond affect the close environment of the affected amino acids. To illustrate this perturbation, the tertiary H-bonds were joined in order to demonstrate the possible flow of information within a protein, whereby the points representing the tertiary hydrogen bonds in the HB plot were connected and used to investigate to which part of the secondary structure of the protein the information flows. By connecting the lines, it was suggested that effective and fast communication within distant parts of the protein can be accomplished if the perturbing effect reaches tertiary hydrogen bonds, while the perturbation effect finally dissipates at regions where interactions between proximal residues are present. The perturbation of a hydrogen bond will affect the close environment of the affected amino acid residues. It is reasonable to assume that in order to have a prompt effect of perturbation on the whole protein, the perturbation should go through the tertiary interactions (Fig. 9A–E). An examination of the HB plots for the WT and MT PDGFR-β model proteins indicated the change in hydrogen bonding interaction patterns in the mutant proteins in comparison with the wild-type proteins. The comparison of the H-bond map of the WT (Fig. 9A) and MT models showed that in the HB plots, the mutant T681M (Fig. 9C) had the maximum perturbation effects in the tertiary H-bonding pattern. Investigating the rearrangement of tertiary hydrogen bonds in the WT and MT form (Tables S3 and S4†), it can be seen that rearrangement of the tertiary hydrogen bonds can be found at two functionally important regions: first the catalytic loop region (His824–Asn831) and second, the DFG activation loop region (Cys843–Pro866). The H-bonding patterns from the residues Ser800–Arg920, including both the aforementioned functional regions in MT T681M have a different HB pattern due to the absence of tertiary hydrogen bonds (Fig. 9C) as compared to WT. However, the HB pattern in all the other MT models, i.e., K634A, T681F, T681I, and T681A, did not show any remarkable alterations in the HB pattern when compared to the WT model. This observation is in tune with the result of the RMSF plot.
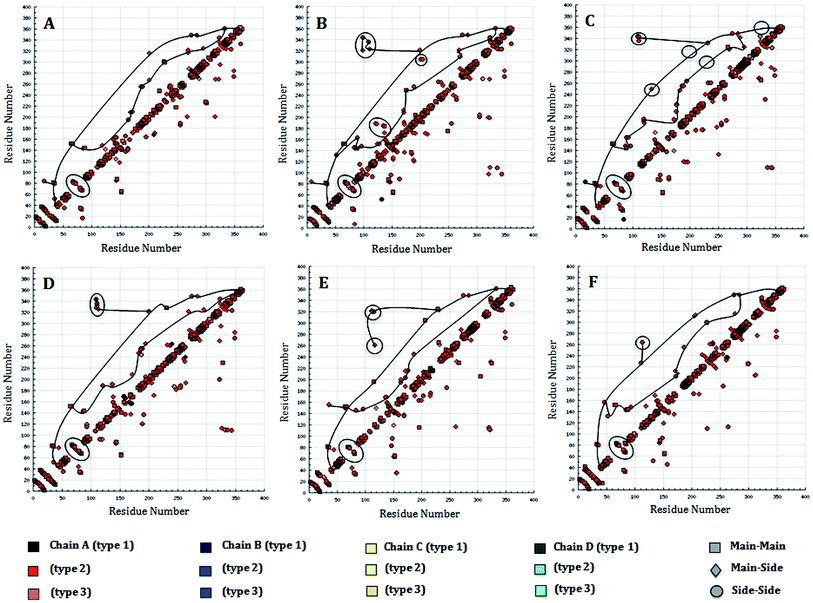 |
| Fig. 9 Hydrogen bond plot of: (A) WT structure of PDGFR-β and (B) MT structure K634A, (C) WT and MT T681M, (D) WT and T681F, (E) WT and T681I, (F) WT and T681A. The modeled PDGFR-β structure comprises 363 amino acid residues, with the numbering patterns of the residues i.e., 600–962 were used for the HB plot analysis based on the full-length sequence, which possess in total 1106 residues. | |
To examine the changes in the overall structure and dynamic stability in WT PDGFR-β and the MT structures throughout the simulation time, we visualized a snapshot of the catalytic site every 20 ns. Five snapshots per protein model (WT and MT) (0 ns, 20 ns, 40 ns, 60 ns, and 80 ns) of the MD trajectories were taken to examine the conformational alteration in their binding mode (Fig. 10). We clearly could see that the mutant T681M showed a significant alteration in the binding mode of sunitinib, which faced a severe steric clash with the larger hydrophobic amino acid residue at the gatekeeper site that terminated the effective ligand binding. It was observed that the conformational alteration was most drastic in the case of the T681M mutant, followed by the K634A, T681F, T681I, and T681A mutants.
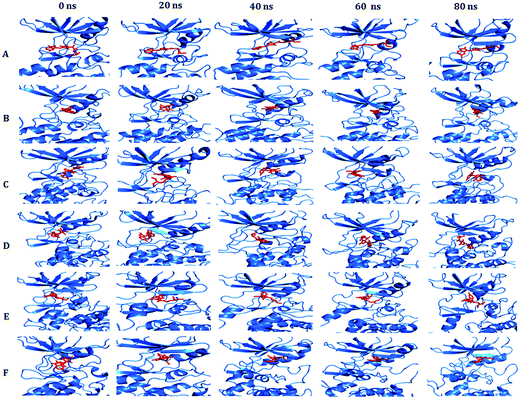 |
| Fig. 10 Conformational changes of the active sites in the PDGFR-β kinase domain associated with the WT and MT models. Observed conformational changes of the binding pattern of PDGFR-β on sunitinib during MD simulation of: (A) WT, (B) mutant K634A, (C) mutant T681M, (D) mutant T681F, (E) mutant T681I, and (F) mutant T681A. Time 0 ns represents the start of the production phase after equilibration and 80 ns represents the end of the production phase. | |
2.8 Binding free energy analysis by MM-GBSA
In order to further explore the free energy of the binding of both small molecules and biological macromolecules, the molecular mechanic energies were combined with MM-GBSA analysis.26 Most of the scoring functions have at least one of major limitation, such as how to handle the solvent effects. However, including physical approximations, such as molecular mechanics generalized born surface area (MM-GBSA), could help solve this problem. Despite comprising various approximations, MM-GBSA methods can assist validating and rationalizing the experimental findings and can be used to improve the results of the docking energies.26 The major objective of this approach is to find the difference between the free energies of the bound and unbound state of the protein–ligand complexes. All the thermochemical properties were calculated by the MM-GBSA method by applying the AMBER suite for each coordinate at every 10 ps sampling frequency throughout the MD trajectory for all the protein–ligand complexes. The complexes having the lowest binding energy were considered to be most stable (Table 2).
Table 2 Absolute free energy ΔGTOT = (ΔEGAS + ΔESOLV) − TΔS; ΔEGAS + ΔESOLV = enthalpy; TΔS = solute entropy; ΔEGAS = total energy of solute, ΔEGAS = ΔEVDW + ΔEELE; ΔEVDW = van der Waal's energy; ΔEELE = electrostatic/coulombic energy; ΔESOLV = total energy of solvation; ΔESOLV = ΔEPOL(GB) + ΔENPOL; ΔEPOL(GB) = polar solvation contribution, generalized born method; ΔENPOL = nonpolar contribution
Energies |
WT-sunitinib (Kcal mol−1) |
K634A-sunitinib (Kcal mol−1) |
T681M-sunitinib (Kcal mol−1) |
T681P-sunitinib (Kcal mol−1) |
T681I-sunitinib (Kcal mol−1) |
T681A-sunitinib (Kcal mol−1) |
ΔEVDW |
−60.83 |
−54.82 |
−42.05 |
−50.08 |
−50.07 |
−55.08 |
ΔEELE |
−34.98 |
−34.36 |
−32.37 |
−33.19 |
−33.43 |
−37.81 |
ΔEPOL (GB) |
12.57 |
19.92 |
16.88 |
17.15 |
16.24 |
16.55 |
ΔENPOL |
−7.87 |
−6.32 |
−5.21 |
−6.43 |
−6.37 |
−6.89 |
ΔEGAS |
−95.81 |
−89.18 |
−72.42 |
−83.27 |
−83.50 |
−92.89 |
ΔESOLV |
4.69 |
13.59 |
11.66 |
10.72 |
9.87 |
9.66 |
ΔGTOT |
−56.14 |
−41.22 |
−30.39 |
−39.35 |
−40.20 |
−45.42 |
Table 2 shows the binding free energy for every component for each WT and MT PDGFR-β bound to sunitinib. For each protein–ligand interaction, the gas phase energy (ΔGgas) contributed to the binding, while the solvation free energy (ΔGsolv) opposed the binding. The van der Waal's energy (ΔEVDW), electrostatic energy (ΔEELE), nonpolar contribution (ΔENPOL), and the total energy of solute (ΔEGAS) have negative values and showed a favorable contribution to the total free energy. However, the total energy of solvation (ΔESOLV) and the polar solvation contribution (ΔEPOL(GB)) possess positive values and therefore contributed unfavorably toward the total free energy. Further investigating the gas phase terms, we found that the van der Waals interactions (ΔEvdw) were more important than the electrostatic interactions (ΔEele), especially for the associations between the WT and MT PDGFR-β models with sunitinib.
The total free energies (ΔGTOT) obtained from MM-GBSA for the protein–ligand complexes show comparable values ranging from −56.14 kcal mol−1 to −30.39 kcal mol−1 (−56.14 kcal mol−1 for the WT PDGFR-β sunitinib complex and −30.39 kcal mol−1 for mutant T681M–sunitinib complex) (Table 2). ΔGTOT for the mutant protein T681M showed the least value for the T681M mutant and sunitinib complex.
2.9 Principal component analysis
For additional support of our MD simulation results, we collected the large-scale motions of the WT and MT PDGFR-β proteins using essential dynamics (ED) analysis. The existence of higher constrains in the MT as compared to the WT protein structure was further assessed by PCA analysis. The essential subspace is defined by the eigenvectors with the largest associated eigen values in which the maximum protein dynamics occurs. From these projections, we could conceive the cluster of stable states. Two features were very apparent from these plots. First, the clusters were more well defined in WT than in the MT models of protein. Second, all the MT models occupied a larger region of phase space, especially along the first principal component (PC1), as compared to that covered by WT (Fig. 11). The overall flexibility of the two protein models (i.e., WT and MT) was further investigated by a trace of the diagonalized covariance matrix of the Cα atomic positional fluctuations. We obtained the trace of the covariance matrix value for the WT protein as lower at 49.532 nm2 (Fig. 11A) as compared to the MT model T681M (Fig. 11B), which showed a higher value of 75.643 nm2. However, the mutants K634A, T681F, T681I, and T681A (Fig. 11C–E) did not show any significantly higher value as compared to WT. It is clear that the mutant T681M acted in a completely different manner, with the trace of the covariance matrix value being 75.643 nm2, when compared to the other mutant protein models, thus confirming the overall increased flexibility of this mutant variant over the WT protein at 300 K (Fig. 11A).
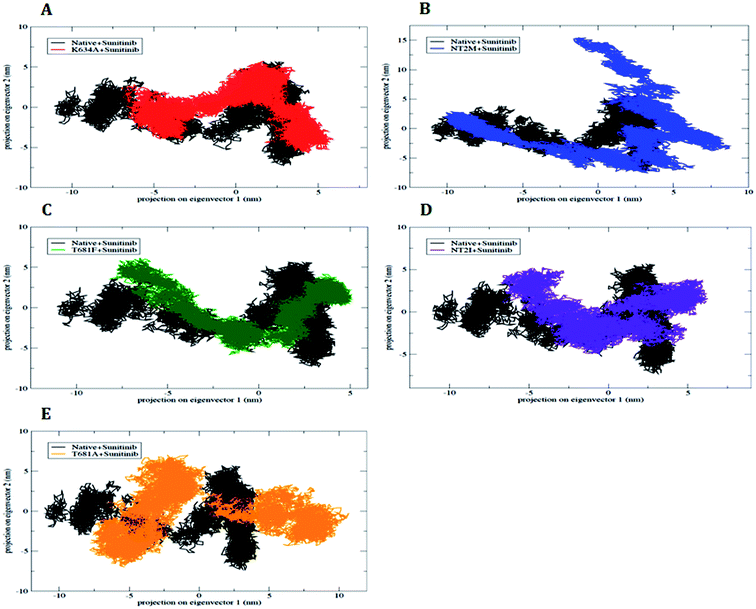 |
| Fig. 11 Projection of the motion of the protein in the phase space along the first two principal eigenvectors at 300 K: (A) WT (black) vs. K634A (red), (B) WT (black) vs. T681TM (blue), (C) WT (black) vs. T681F (green), (D) WT (black) vs. T681I (violet), and (E) WT (black) vs. T681A (orange.). | |
3. Conclusion
Human PDGFR-β is a novel therapeutic target for glioblastoma involving cell angiogenesis. We predicted the PDGFR-β kinase domain to determine the ATP binding site and validated it with an in silico mutagenesis study. A molecular dynamics simulation was precisely performed to refine the models (i.e., WT and MT) as well as to illustrate the residual interactions with the potential kinase inhibitor sunitinib. In the present work, we demonstrated that the mutants in PDGFR-β lead to developing resistance in anticancer drug, due to conformational changes in the protein. The K634A, T681M, M681F, M681I, and M681A site-directed mutations in the tyrosine kinase domain of the PDGFR-β are the key mutations that have been reported in the literature. Among all the MT proteins, T681M showed the most significant structural alterations. This was confirmed by docking and MD simulation studies that were used to explain the binding mechanism of the MT models, such as K634A, M681F, M681I, and M681A, which showed similar behavior in comparison to T681M with a drug complex. The key residues, such as Lys634, Thr681, Tyr683, Cys684, and Asp742 involved in hydrogen bond interactions with sunitinib were perturbed in the mutant receptor, leading to conformational changes and a loss of stability in the MT models. The residue T681M in PDGFR-β triggered significant conformational modulations, which led to a decrease in binding affinity of sunitinib (ΔG = −5.80; Ki = 55.75 μM) as compared to WT (ΔG = −12.94; Ki = 0.32 nM), respectively. Essentially, the present study provides significant insights into the structure-based development of a PDGFR-β kinase inhibitor in glioblastoma treatment.
4. Materials and methods
4.1 Structure prediction of human WT and MT PDGFR-β and validation of the models
The primary amino acid sequence of the human PDGFR-β kinase domain was obtained from the SWISS-PROT protein sequence Database (ID: P09619 – PDGFRB_HUMAN).27 The kinase domain of PDGFR-β (600–962) was considered for the present study. Sequence homologous templates to PDGFR-β were obtained from the Protein Data Bank (http://www.rcsb.org/pdb) by using a PSI-BLAST search.28 The templates crystal structures by overall sequence identity were: VEGFR-2 (PDB ID: 1vr2, chain A, resolution 2.4 Å);29 FGFR-1 (PDB ID: 1fgi, chain A, resolution 2.5 Å);30 insulin receptor kinase (PDB ID: 1irk, chain A, resolution 2.1 Å).31 The PDGFR-β sequence was aligned with the three template protein sequences using Clustal-X 2.1.32 The numbering pattern used for the amino acids measured in this study was based on the full-length sequence. The 3D model of PDGFR-β was built by a comparative modeling approach incorporated in the program MODELLER 9v8.33 We generated 100 models for the sequence-templates alignment. The best protein model was chosen on the basis of the DOPE (discrete optimized protein energy),22 assessment method as implemented in the modeler. The modeled structures were validated by the ProSA-web34 and SAVES servers (http://nihserver.mbi.ucla.edu/SAVES), which uses the PROCHECK, ERRAT, and WHAT CHECK programs.35–37 ProSA server was used to validate the quality of the modeled structure on the basis of the Z-scores, which specifies the overall quality of a model. The PROCHECK program elucidated the stereochemical quality by creating a Ramachandran plot.
4.2 Molecular dynamics simulations
MD simulation of the PDGFR-β kinase domain model was carried out for energy minimization and for analyzing the biophysical behavior in the dynamic system. All the MD simulations were performed with the GROMACS 4.6.5 package, using the GROMOS96 43A1 force field38 and spc216 water model. The coordinate of each system was energy minimized with the steepest descent algorithm to remove any bad contacts. The protein was placed centrally in a cubic box with a size of 1.0 nm and the systems were solvated with a simple point charge (SPC) water molecule. Finally, the protein was solvated in a cubic box having a size of nearly 70.5 × 70.5 × 70.5 Å, and five Na+ ions were added to neutralize the system by replacing the water molecules to confirm the overall charge neutrality, while periodic boundary conditions (PBC) were applied in all directions. A 1.4 nm cutoff for the van der Waals interactions and a 1.0 nm cutoff for the columbic interactions were adopted for the simulation study. The linear constraint solver (LINCS) algorithm39 was used to constrain all the bond lengths. For energy minimization of the protein, a two-step ensemble process (NVT and NPT) was used to equilibrate the system at a constant temperature of 300 K. Initially, the Berendsen thermostat method with no pressure coupling was used for the canonical ensemble, NVT and secondly involve Parrinello–Rahman method with pressure of 1 bar (P) for, isothermal–isobaric (NPT) ensemble.40 For computing the long-range electrostatic interactions, the Particle Mesh Ewald (PME)41 method was used. Finally, after equilibration with constant temperature and pressure, the system was submitted to 40 ns MD simulation at 300 K and 1 bar pressure to carry out the structural analyses on PDGFR-β. All the analyses of the trajectories were examined visually using the VMD software42 and UCSF Chimera.43
4.3 Molecular dynamics trajectory analysis
The structural properties of the native or WT and MT models of the PDGFR-β protein were computed from the molecular dynamics trajectory files with the built-in utilities of GROMACS 4.6.5. All the trajectory files were analyzed by the use of g_rmsd, g_rmsf, g_sas, and g_gyrate GROMACS utilities to obtain the graphs of the root-mean-square deviations (RMSD), the root-mean-square fluctuations (RMSF), the solvent accessibility surface area (SASA), and the radius of gyration (Rg). The total number of intermolecular hydrogen bonds (HB) formed between the protein and ligand throughout the simulation was calculated using an HB analysis tool.45 The secondary structural analyses were performed for both the WT and MT models over the whole simulation period by using the DSSP module.23 The total number of hydrogen bond was prominent on the basis of the donor–acceptor distance being smaller than 3.9 Å and the donor–hydrogen–acceptor angle being larger than 90°.44 The Graphing, Advanced Computation and Exploration program (GRACE)45 program was used to generate the 3D backbone RMSD, while the C-α (carbon-alpha) atoms of RMSF, Rg, and SASA analysis motion projection of the molecules in the phase space of the system were plotted for all six simulations.
4.4 Molecular docking
Docking simulations were performed with the program AutoDock 4.2.46,47 The Lamarckian genetic algorithm (LGA) was applied to deal with the protein–antagonists interactions.46 Polar hydrogen atoms were added geometrically, and Gasteiger charges to all the atoms were assigned to the protein PDGFR-β, and a PDBQ file was then created. The 3D affinity grid fields with a grid map of 50 × 40 × 40 points were created using the auxiliary program AutoGrid. A grid-point spacing of 0.375 Å, and a distance-dependent function of the dielectric constant were used for the calculation of the energetic map. The default settings were used for all the other parameters. The AutoDock tools utility was used to generate both the grid and docking parameter files (i.e., gpf and dpf).
For all dockings, 100 independent executed runs were carried with the step sizes of 0.2 Å for translations and 8° for orientations and torsions. For LGA, pseudo-Solis and Wets native search approaches were used, with an initial population of random individuals with a population size of 150 individuals; a maximum number of 2.5 × 106 energy assessments; a maximum number of generations of 2.7 × 106; an elitism value of 1; a mutation rate of 0.02; and a crossover rate of 0.8. The numbers of iterations per local search were 300. The possibility of performing a local search on an individual in the population was 0.06. The maximum number of sequential successes or failures before doubling or halving the local search step size was 4, and the termination criterion for the local search was 0.01. For the binding energy in the docking step, the van der Waals' interaction representing as Lennard–Jones 12–6 dispersion/repulsion, hydrogen bonding as a directional 12–10 term, and the coulombic electrostatic potential for the charges were used.
The docking simulations ended after several runs and a cluster analysis of the ligands was implemented with their corresponding docked energy. Docking solutions with a ligand all-atom rmsd within 1.0 Å of each other were clustered together and ranked by the lowest energy representative. The lowest energy solution of the lowest ligand all-atom rmsd cluster was accepted as the calculated binding energy. The whole system was minimized to convergence. Although the solvation energies could not be explicitly considered during the minimization, the energy calculations were performed with a distance-dependent dielectric constant of 5 to mimic the solvation effect of the inhibitors in the protein environment.48 All the calculations were carried out on PC-based machines running Unix operating systems. The resultant structure files for WT and MT PDGFR-β docked to sunitinib were analyzed using VMD 1.8.5 and PyMOL visualization programs.42,49
4.5 MD simulations: protein–drug complex
All the MD simulations were performed for the WT and MT models of the PDGFR-β protein in the presence of sunitinib. The complexes of PDGFR-β–sunitinib were obtained from the molecular docking analysis with the WT and MT models to evaluate the structural changes, binding affinity, and stability of the complex in the course of a dynamic system. The preliminary step in the complex MD simulation was the generation of a topology file for the ligand using the GlycoBioChem PRODRG2 server.50 The correlation between the ligand charges predicted by PRODRG2 and the quantum chemical calculations are shown in Fig. S6.† After defining the sunitinib topology, the same procedure revealed in the above section describing the MD simulations was followed. All the trajectory files were obtained from a 40 ns simulation run and were analyzed with GROMACS utilities: g_rms, g_rmsf, g_gyrate, g_sas and do_DSSP, while hydrogen bond analysis was carried out by HB plot software.
4.6 Binding free energy calculations
Binding free energy calculations are used for an extensive investigation of the energetic coefficients responsible for the molecular stability or binding affinity51 and provide a quantitative measurement of protein–ligand interactions.52 The binding free energies of PDGFR-β with sunitinib were analyzed using the molecular mechanics generalized born surface area (MM-GBSA)53,54 method as integrated in the AMBER suite. At first, we extracted the last 10 ns trajectory from the 80 ns MD simulation results and converted it to the crd format for AMBER through visual molecular dynamics (VMD).42 For calculation of all the energy components, a total of 1000 snapshots were taken from the trajectory, and finally, the single trajectory protocol was applied to calculate the MM-GBSA binding free energy. The binding free energy ΔG was calculated as the difference for three species as:
ΔGbind = Gcomplex − Gprotein − Gligand |
The free energy was calculated for the protein, ligand, and complex using a single trajectory approach for each snapshot.
4.7 Principal component analysis
To study the global motion of PDGFR-β WT and MT protein structures, we carried out a principal component analysis (PCA) or essential dynamics (ED) analysis. The calculation of the eigenvectors and eigen values, and their projection along the first two principal components, was carried out according to the standard protocol within the GROMACS software utilities.55 PCA or ED is a method that diminishes the complexity of the data and obtains the concerted motion in the MD simulations, which are essentially correlated and presumably significant for biological functions.55 In the ED analysis, a covariance matrix was formed after elimination of the rotational and translational movements from the trajectories. The set of eigenvectors and eigenvalues was recognized by diagonalizing the matrix. Eigenvalues represent the amplitude of the eigenvector along the multidimensional space, while the displacement of Cα atoms along each eigenvector shows the concerted motions of the protein along each direction. This method divided the protein into two conformational subspaces: an essential subspace and a physically constrained non-essential subspace. The essential subspace was defined by the unconstrained, anharmonic motion of the positional fluctuation of the atoms. In order to perform PCA, the trajectory files of the Cα backbone were first obtained and analyzed using g_covar and g_anaeig in GROMACS utilities.
Conflict of interest
Both the authors of this paper declare that they have no conflict of interest.
Acknowledgements
The authors wish to thanks Delhi University Computer Center (DUCC), University of Delhi, Delhi – 110007, India, for providing the computer outsourcing cluster facility, DBT-BIF (ACBR), and Centre for Development and Advanced Computing (C-DAC), Bioinformatics Resources & Applications Facility (BRAF), Pune, India, for providing supercomputing facility for Molecular Dynamic Simulation.
References
- Y. Yarden, J. Escobedo, W. Kuang, T. Yang-Feng, T. Daniel, P. Tremble, E. Chen, M. Ando, R. Harkins and U. Francke, Nature, 1986, 323, 226–232 CrossRef CAS PubMed.
- G. Manning, D. B. Whyte, R. Martinez, T. Hunter and S. Sudarsanam, Science, 2002, 298, 1912–1934 CrossRef CAS PubMed.
- J. Yu, C. Ustach and H.-R. C. Kim, J. Biochem. Mol. Biol., 2003, 36, 49–59 CAS.
- S. S. Taylor and E. Radzio-Andzelm, Structure, 1994, 2, 345–355 CrossRef CAS PubMed.
- B. Nolen, S. Taylor and G. Ghosh, Mol. Cell, 2004, 15, 661–675 CrossRef CAS PubMed.
- S. S. Taylor and A. P. Kornev, Trends Biochem. Sci., 2011, 36, 65–77 CrossRef CAS PubMed.
- S. W. Cowan-Jacob, W. Jahnke and S. Knapp, Future Med. Chem., 2014, 6, 541–561 CrossRef CAS PubMed.
- M. Hermanson, K. Funa, M. Hartman, L. Claesson-Welsh, C.-H. Heldin, B. Westermark and M. Nistér, Cancer Res., 1992, 52, 3213–3219 CAS.
- M. Raica and A. M. Cimpean, Pharmaceuticals, 2010, 3, 572–599 CrossRef CAS PubMed.
- C. Betsholtz, P. Lindblom, M. Bjarnegard, M. Enge, H. Gerhardt and P. Lindahl, Curr. Opin. Nephrol. Hypertens., 2004, 13, 45–52 CrossRef CAS PubMed.
- D. Fabbro, S. Ruetz, E. Buchdunger, S. W. Cowan-Jacob, G. Fendrich, J. Liebetanz, J. Mestan, T. O'Reilly, P. Traxler and B. Chaudhuri, Pharmacol. Ther., 2002, 93, 79–98 CrossRef CAS PubMed.
- A. E. El-Kenawi and A. B. El-Remessy, Br. J. Pharmacol., 2013, 170, 712–729 CrossRef CAS PubMed.
- C.-H. Heldin, Cell Commun. Signaling, 2013, 11, 1 CrossRef PubMed.
- C. DeWeese-Scott and J. Moult, Proteins: Struct., Funct., Bioinf., 2004, 55, 942–961 CrossRef CAS PubMed.
- A. Sorkin, B. Westermark, C.-H. Heldin and L. Claesson-Welsh, J. Cell Biol., 1991, 112, 469–478 CrossRef CAS PubMed.
- F. D. Böhmer, L. Karagyozov, A. Uecker, H. Serve, A. Botzki, S. Mahboobi and S. Dove, J. Biol. Chem., 2003, 278, 5148–5155 CrossRef PubMed.
- M. Azam, M. A. Seeliger, N. S. Gray, J. Kuriyan and G. Q. Daley, Nat. Struct. Mol. Biol., 2008, 15, 1109–1118 CAS.
- D. Srinivasan, D. M. Kaetzel and R. Plattner, Cell. Signalling, 2009, 21, 1143–1150 CrossRef CAS PubMed.
- R. Morphy, J. Med. Chem., 2009, 53, 1413–1437 CrossRef PubMed.
- P. Larsson, B. Wallner, E. Lindahl and A. Elofsson, Protein Sci., 2008, 17, 990–1002 CrossRef CAS PubMed.
- J. C. Mobarec, R. Sanchez and M. Filizola, J. Med. Chem., 2009, 52, 5207–5216 CrossRef CAS PubMed.
- M. Y. Shen and A. Sali, Protein Sci., 2006, 15, 2507–2524 CrossRef CAS PubMed.
- W. Kabsch and C. Sander, Biopolymers, 1983, 22, 2577–2637 CrossRef CAS PubMed.
- J. J.-L. Liao, J. Med. Chem., 2007, 50, 409–424 CrossRef CAS PubMed.
- Z. Bikadi, L. Demko and E. Hazai, Arch. Biochem. Biophys., 2007, 461, 225–234 CrossRef CAS PubMed.
- S. Genheden and U. Ryde, Expert Opin. Drug Discovery, 2015, 10, 449–461 CrossRef CAS PubMed.
- E. Gasteiger, A. Gattiker, C. Hoogland, I. Ivanyi, R. D. Appel and A. Bairoch, Nucleic Acids Res., 2003, 31, 3784–3788 CrossRef CAS PubMed.
- S. F. Altschul, T. L. Madden, A. A. Schäffer, J. Zhang, Z. Zhang, W. Miller and D. J. Lipman, Nucleic Acids Res., 1997, 25, 3389–3402 CrossRef CAS PubMed.
- M. A. McTigue, J. A. Wickersham, C. Pinko, R. E. Showalter, C. V. Parast, A. Tempczyk-Russell, M. R. Gehring, B. Mroczkowski, C.-C. Kan and J. E. Villafranca, Structure, 1999, 7, 319–330 CrossRef CAS PubMed.
- M. Mohammadi, G. McMahon, L. Sun, C. Tang, P. Hirth, B. K. Yeh, S. R. Hubbard and J. Schlessinger, Science, 1997, 276, 955–960 CrossRef CAS PubMed.
- S. R. Hubbard, L. Wei, L. Ellis and W. A. Hendrickson, Nature, 1994, 372, 746–754 CrossRef CAS PubMed.
- R. Chenna, H. Sugawara, T. Koike, R. Lopez, T. J. Gibson, D. G. Higgins and J. D. Thompson, Nucleic Acids Res., 2003, 31, 3497–3500 CrossRef CAS PubMed.
- A. Šali and T. L. Blundell, J. Mol. Biol., 1993, 234, 779–815 CrossRef PubMed.
- M. Wiederstein and M. J. Sippl, Nucleic Acids Res., 2007, 35, W407–W410 CrossRef PubMed.
- A. L. Morris, M. W. MacArthur, E. G. Hutchinson and J. M. Thornton, Proteins: Struct., Funct., Bioinf., 1992, 12, 345–364 CrossRef CAS PubMed.
- R. Liithy, J. Bowie and D. Eisenberg, Nature, 1992, 356, 83–85 CrossRef PubMed.
- R. A. Laskowski, M. W. MacArthur, D. S. Moss and J. M. Thornton, J. Appl. Crystallogr., 1993, 26, 283–291 CrossRef CAS.
- X. Daura, A. E. Mark and W. F. Van Gunsteren, J. Comput. Chem., 1998, 19, 535–547 CrossRef CAS.
- B. Hess, H. Bekker, H. J. Berendsen and J. G. Fraaije, J. Comput. Chem., 1997, 18, 1463–1472 CrossRef CAS.
- R. Martoňák, A. Laio and M. Parrinello, Phys. Rev. Lett., 2003, 90, 075503 CrossRef PubMed.
- T. Darden, D. York and L. Pedersen, J. Chem. Phys., 1993, 98, 10089–10092 CrossRef CAS.
- W. Humphrey, A. Dalke and K. Schulten, J. Mol. Graphics, 1996, 14, 33–38 CrossRef CAS PubMed.
- E. F. Pettersen, T. D. Goddard, C. C. Huang, G. S. Couch, D. M. Greenblatt, E. C. Meng and T. E. Ferrin, J. Comput. Chem., 2004, 25, 1605–1612 CrossRef CAS PubMed.
- E. Baker and R. Hubbard, Prog. Biophys. Mol. Biol., 1984, 44, 97–179 CrossRef CAS PubMed.
- P. Turner, Center for Coastal and Land-Margin Research, Oregon Graduate Institute of Science and Technology, Beaverton, OR, 2005 Search PubMed.
- D. S. Goodsell and A. J. Olson, Proteins: Struct., Funct., Bioinf., 1990, 8, 195–202 CrossRef CAS PubMed.
- G. M. Morris, D. S. Goodsell, R. S. Halliday, R. Huey, W. E. Hart, R. K. Belew and A. J. Olson, J. Comput. Chem., 1998, 19, 1639–1662 CrossRef CAS.
- E. L. Mehler and T. Solmajer, Protein Eng., 1991, 4, 903–910 CrossRef CAS PubMed.
- M. A. Lill and M. L. Danielson, J. Comput.-Aided Mol. Des., 2011, 25, 13–19 CrossRef CAS PubMed.
- A. W. SchuÈttelkopf and D. M. Van Aalten, Acta Crystallogr., Sect. D: Biol. Crystallogr., 2004, 60, 1355–1363 CrossRef PubMed.
- N. Homeyer and H. Gohlke, Mol. Inf., 2012, 31, 114–122 CrossRef CAS PubMed.
- W. Wang and P. A. Kollman, J. Mol. Biol., 2000, 303, 567–582 CrossRef CAS PubMed.
- T. Hou, J. Wang, Y. Li and W. Wang, J. Chem. Inf. Model., 2010, 51, 69–82 CrossRef PubMed.
- J. Wang, P. Morin, W. Wang and P. A. Kollman, J. Am. Chem. Soc., 2001, 123, 5221–5230 CrossRef CAS PubMed.
- A. Amadei, A. Linssen and H. J. Berendsen, Proteins: Struct., Funct., Bioinf., 1993, 17, 412–425 CrossRef CAS PubMed.
Footnote |
† Electronic supplementary information (ESI) available. See DOI: 10.1039/c7ra01305a |
|
This journal is © The Royal Society of Chemistry 2017 |
Click here to see how this site uses Cookies. View our privacy policy here.