DOI:
10.1039/C7RA00473G
(Paper)
RSC Adv., 2017,
7, 9989-9997
Rapid identification of dual p53-MDM2/MDMX interaction inhibitors through virtual screening and hit-based substructure search†
Received
12th January 2017
, Accepted 27th January 2017
First published on 3rd February 2017
Abstract
Multi-target agents have garnered great interest over the past decade for their favorable therapeutic efficacy and drug resistance profiles. Recently, dual inhibition of the p53 tumor suppressor interaction with its two negative regulators MDM2 and MDMX has become an attractive anticancer approach as it can induce sustained MDM2/MDMX antagonism and robust p53 activation. However, small molecule inhibitors with dual specificity against MDM2 and MDMX are difficult to design and are still scarce. To identify novel scaffolds for dual inhibition of the p53-MDM2/MDMX interactions, we developed two five-point pharmacophore models for filtering the 2012 National Cancer Institute database, from which molecular docking was conducted to identify dual inhibitors. We found 38 virtual hits and subjected them to a fluorescence polarization-based competitive binding assay, resulting in 10 active compounds of different scaffolds. To further expand the chemical diversity of the initial hits, we performed a hit-based substructure search and identified NSC148171 from pharmacophore 1 as the most potent dual-specificity inhibitor with Ki values for MDM2 and MDMX at 0.62 and 4.6 μM. All hits were subjected to inhibition assay of cancer cellular vitality and showed anti-proliferative activity roughly correlated with their Ki values. This work not only yields several novel scaffolds for further structural and functional optimization of dual-specificity inhibitors of the p53-MDM2/MDMX interactions, but also showcases the power of our computational methods for small molecule anticancer drug discovery.
Introduction
Multi-target drugs have attracted considerable attention for the past decade as viable therapeutic solutions to complex diseases and growing incidences of drug resistance.1 While a single-target strategy has proven useful to treat some single gene disorders, it fails to alleviate many complex ones involving multiple factors.2 Due to the compensatory mechanisms and redundant functions, biological systems can tolerate single-point disturbance.3 From this aspect, complex diseases are often caused by the breakdown of physiological systems owing to multiple genetic and/or environmental factors, thus they are more possibly healed or alleviated though simultaneous adjustment of multiple targets.4 Moreover, a dual inhibitor has great advantages over the combination of two single-target drugs as it can eliminate the necessity of optimizing individual inhibitors' doses for efficacy and potential complications of drug–drug interactions.5 Therefore, the design of multi-target compounds is recently an area of great interest in the pharmaceutical industry, especially for the treatment of cancers.6
p53 is a tumor suppressor, which transcriptionally regulates the expression of various target genes that mediate cell-cycle arrest, DNA repair or apoptosis in response to cellular stresses such as DNA damage or oncogene activation.7 All these cellular responses are designed to prevent damaged cells from proliferating and passing mutations on to the next generation.8 Not surprisingly, in almost all human cancers the p53 pathway is defective due to either loss-of-function mutations in the DNA-binding domain of p53 or functional inhibition of wild-type p53 by its two negative regulators, i.e., MDM2 and MDMX.9,10 Ample evidence shows that MDM2 primarily controls p53 stability through ubiquitination to target the tumor suppressor protein for constitutive degradation by the proteasome, whereas MDMX mainly acts as a significant p53 transcriptional antagonist independently of MDM2.7,11–14 Simultaneous disruption of the p53-MDM2/MDMX interactions has been shown to achieve sustained and robust p53 activation, promising a highly attractive strategy for anticancer therapy.15
Although multi-target drugs have advantages, they are difficult to identify and design. Recently, several laboratories conducted in silico screening to identify dual inhibitors targeting the p53-MDM2/MDMX interactions,16,17 and all reported hits showed weak activity. In this study, we aim to develop a virtual screening method coupled with a hit-based substructure search strategy for identifying more potent dual inhibitors of the p53-MDM2/MDMX interactions. Virtual hits were subjected to a fluorescence polarization (FP) based competitive binding assay.18 Our strategy will likely provide a new and efficient method for the identification of novel dual inhibitors of the p53-MDM2/MDMX interactions with therapeutic potential.
Materials and methods
Data set of dual inhibitors of MDM2 and MDMX
1712 chemical structures of MDM2 and/or MDMX inhibitors were retrieved from the ChEmBL database (https://www.ebi.ac.uk/chembl/), of which 63 entries with bioactivities (Kd, Ki, or IC50) measured against both MDM2 and MDMX were deemed “dual inhibitors” used in our study for their scaffold and R-group analysis. The analysis of scaffold was performed using “Scaffold Hunter”19 and “ChemAxon's Fragmenter” (http://www.chemaxon.com/), that implemented the RECAP20 algorithm. The module “Find molecules from a library with similar fingerprints to the reference compound” in Discovery studio 2.5 was used to analyze R-groups. Minimum similarity is set to 0.6. The structure and bioactivity information on these compounds is listed in Table S1 in the ESI.† The molecular weight (MW) and hydrophobicity parameter A
log
P (Ghose–Crippen–Viswanadhan octanol–water partition coefficient) of 63 inhibitors were acquired by using “Calculate Various Molecular Properties for Ligands” module in Discovery studio 2.5. The density plots were made by the software R 3.2.3 (https://www.r-project.org/).
Structural selection and energy minimization
The structures of MDM2-RO-2443 (PDB CODE: 3VBG), MDMX-RO-2443 (PDB CODE: 3U15), MDM2-K23 (PDB CODE: 3LBK), and MDMX-WW8 (PDB CODE: 3LBJ) were downloaded from the Protein Data Bank (http://rcsb.org). We chose the above structures because both MDM2 and MDMX proteins can bind to the same inhibitor (RO-2443) or inhibitors of highly similar scaffolds (K23 and WW8). Discovery studio 2.5 was used to prepare the above structures through residual repair and energy minimization as previously described.21
Molecular docking for the ligand-MDM2 and -MDMX interactions
Molecular docking of a series of compounds was conducted for MDM2 and MDMX. The “define and edit binding site” module in Discovery studio 2.5 was used to define the binding pockets of MDM2 and MDMX. Co-crystallized compounds were used to define the pocket of protein. CDOCKER, a docking module in Discovery studio 2.5, was used to generate the detailed ligand–protein interactions. All docking parameters are default in CDOCKER module.
Pharmacophoric filtering for MDM2/MDMX
Structural details from known dual inhibitors of MDM2/MDMX were used to generate pharmacophoric filters. After structural analysis, two skeletons were chosen to form two pharmacophore models. The reasons for choosing these two skeletons were discussed in the section “Pharmacophore model and Virtual Screening”. The pharmacophore module in discovery studio 2.5 was used to construct these two five-point pharmacophore models. The NCI database was further filtered with above models. We used default parameters in the pharmacophore module in Discovery studio.
Virtual screening for dual inhibitors of p53-MDM2/MDMX interactions
After filtering the NCI database, we performed further virtual screening against MDM2 and MDMX by molecular docking. The CDOCKER program in Discovery studio 2.5 was used to further screen the NCI database. Default parameters were used during the docking process, followed by the “Analyze Ligand Poses” process and computation of hydrogen (H)-bonds between receptor and ligand poses. We only chose ligands that can form H-bonds with the same residues as seen in the crystal structure.
FP based competitive binding assay
In order to determine quantitatively the binding affinities of the compounds for MDM2 and MDMX, we used the previously established FP-based competitive binding assay.18 MDM2(25-109) and MDMX(24-108) proteins were chemically synthesized and structurally and functionally characterized as described previously.7 A phage-selected dual-specificity peptide antagonist of both MDM2 (Kd = 3.2 nM) and MDMX (Kd = 8.5 nM), termed PMI (TSFAEYWNLLSP),7 was fluorescently labeled. Succinimidyl ester-activated carboxytetramethylrhodamine (TAMRA-NHS) was covalently conjugated to the N-terminus of PMI, yielding TAMRA-PMI. The Kd values of TAMRA-PMI with MDM2 and MDMX were determined to be 0.62 and 0.72 nM, respectively. The specificity of binding was confirmed by competitive displacement of TAMRA-PMI from MDM2/MDMX by unlabeled PMI. Dose-dependent, competitive binding experiments were performed with serial dilution of test compound in DMSO. The test compound, MDM2/MDMX protein (50 nM) and TAMRA-PMI peptide (10 nM) in PBS (pH 7.4) were added to a Costar 96-well, black, round-bottom plate (Corning #3993) to produce a final volume of 125 μL. The polarization values were measured after 30 min of incubation at room temperature on a Tecan Infinite M1000 plate reader at λex = 530 nM, λem = 580 nM. Curve fitting was performed using GRAPHPAD PRISM4 software. Ki values were calculated as described previously for FP-based assays.18
In vitro antiproliferative activity
The cellular growth inhibitory activity was evaluated by a human primary glioblastoma cell line U87 (wild-type p53), which was obtained from American Type Culture Collection (ATCC). Cells were maintained in DMEM supplemented with 10% heat-inactivated FBS and 100 units per mL penicillin/streptomycin at 37 °C in a humidified atmosphere of 5% CO2. An amount of 3000–5000 cells per well was transferred to 96-well plates. After culturing for 12 h, the test compounds were added to triplicate wells at 100 μM and 0.1% DMSO for control. After 72 h of incubation, 20 μL per well MTS Reagent was added into each well and incubate for 2 hours at 37 °C in standard culture conditions. Shake the plate briefly on a shaker and measure absorbance of treated and untreated cells using a Tecan Infinite M1000 plate reader at OD with 490 nm. Wells containing no drugs were used as blanks. In order to avoid colorimetric interference, we used each compound (100 μM) dissolved in cell culture without cells as a negative control, and its absorbance value was deducted from that of sample well.22 Nutlin-3 (Sigma-Aldrich, 50 μM) was used as a positive control to validate the assay.
PAINS (pan-assay interference compounds) predictions
To eliminate false positive hits, we used PAINS-remover (http://www.cbligand.org/PAINS)23 as an additional filter to exclude potential promiscuous binders.
Results and discussion
Scaffolds and R-groups of known dual inhibitors of MDM2 and MDMX
The 63 dual inhibitors of MDM2 and MDMX (Table S1†) can be mainly categorized into 6 different scaffolds (Fig. 1). Further structural analysis identified 4 major R-groups found predominantly in 46 dual inhibitors of MDM2 and MDMX, with p-halogen substituted phenyl being the most abundant. The analysis of structural properties of dual inhibitors may provide useful information for the identification of new skeletons in small molecule drug design.
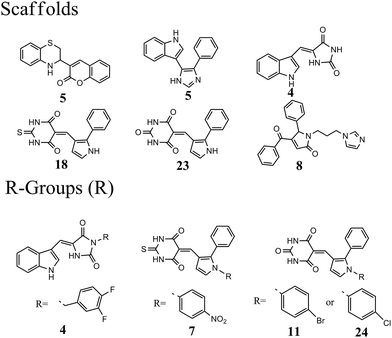 |
| Fig. 1 Analysis of scaffolds and R-groups for 63 dual inhibitors. The numbers represented the counts that were involved in 63 dual inhibitors. | |
Compound database preparation
Molecular properties such as molecular weight (MW) and hydrophobicity parameter A
log
P have been used extensively in modern drug discovery.24 We made a density plot of MW and A
log
P for 63 known dual inhibitors of MDM2 and MDMX retrieved from ChEMBL (https://www.ebi.ac.uk/chembl/). As shown in Fig. 2, the MW distribution of all known dual inhibitors ranged from 300 to 800, and their A
log
P distribution spanned from 0 to 9. These two rules or parameters were then applied to the NCI database to reduce the number of compounds in it from 265242 to 130105.
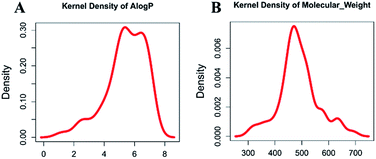 |
| Fig. 2 Distribution of molecular weight and A log P of known dual inhibitors. | |
Pharmacophore model and virtual screening
The pharmacophore module in Discovery studio 2.5 was used to construct two five-point pharmacophore models. The first model was based on RO-2443 and RO-5963,10 and the second one on K23, WW8, 4t and nutlin-3.10,25 We selected these compounds as templates for two reasons. First, these compounds are dual inhibitors. While RO-2443 and RO-5963 share the same skeleton, K23, WW8, 4t and nutlin-3 possess similar pharmacophores. Second, several crystal structures of some of these compounds in complex with MDM2 and/or MDMX have been reported,25,26 showing two different binding modes of MDM2/MDMX at the inhibitor–protein interface. MDM2 differs in binding mode between its complexes with RO-2443 (PDB CODE: 3VBG) and K23 (PDB CODE: 3LBK), whereas MDMX shows a difference in binding between its complexes with RO-2443 (PDB CODE: 3U15) and WW8 (PDB CODE: 3LBJ). For clarity, we chose RO-2443 and 4 t as representative structures in respective models to highlight pharmacophores at the binding site (Fig. 3). 4t was chosen because it comprises all common pharmacophores contained within K23, WW8 and nutlin-3 (PDB CODE: 5C5A).
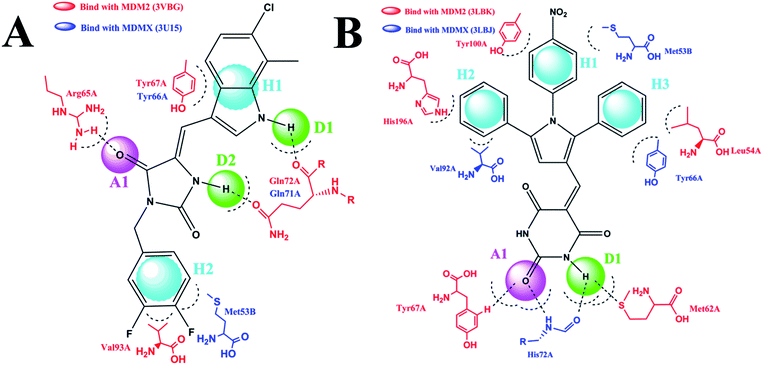 |
| Fig. 3 Two-dimensional pharmacophore models. (A) This model was based on two dual inhibitors, including RO-2443 and RO-5963. RO-2443 was used as a representative structure to highlight pharmacophores at the binding site. H2A1D2 showed two hydrophobic or hydrophobic aromatic centers (H), one H-bond acceptor (A) and two H-bond donors (D). (B) For K23, WW8, 4t, and nutlin-3, 4t was chosen as a representative structure to show the pharmacophore model. We used a five-point pharmacophore match allowing H3A1D1. | |
Shown in Fig. 3A is RO-2443 in complex with MDM2 (red) and MDMX (blue), where five pharmacophores in the first model recognized by both proteins are composed of one H-bond acceptor (A), two H-bond donors (D) and two hydrophobic or aromatic centers (H). Docking studies of 4t in the context of the complex structures of K23-MDM2 and WW8-MDMX led to the identification of five pharmacophores comprising three hydrophobic centers, one H-bond acceptor and one H-bond donor (Fig. 3B). Residues involved in interactions with RO-2443 were consistent with subsite interactions found in the known crystal structures of MDM2 and MDMX complexed with small inhibitors. These two models were applied to further filter the NCI database. Pharmacophore 1 (Fig. 3A) shrunk 130105 compounds into 32412 and pharmacophore 2 (Fig. 3B) narrowed down 130105 compounds to 29344 compounds.
A virtual docking screening was carried out on the optimized 3D chemical compound library. We validated the docking method CDOCKER by re-dock the co-crystallized ligand to its crystal structure, results showed that the original binding pose could be reproduced, which demonstrated the reliability of our docking method. Compounds screened on the basis of the first pharmacophore model (Fig. 3A) should have H-bond interactions with Arg65A and Gln72A in MDM2 (3VBG), and Gln71A in MDMX (3U15). For compounds derived from the second pharmacophore model (Fig. 3B), they should at least form one H-bond with MDM2 (3LBJ) or MDMX (3LBK). We obtained 567 compounds that satisfied the criteria defined by both models, 38 of which with sufficiently different scaffolds were available from the NCI for our subsequent studies. It is worth noting that the CDOCKER scoring is another useful criterion for virtual screening.27 We used hydrogen bonding as the criterion in our work because it has been shown by others that H-bonding makes critical energetic contributions to the binding of small molecule compounds to MDM2 and MDMX.28
Our results appear to validate the selection of H-bonding as a viable criterion for screening with respect to these proteins.
Potential inhibitors from in silico screening
We measured the binding affinities (Ki) of the 38 compounds for MDM2 and MDMX in a FP-based competitive binding assay, and ten of them exhibited moderate binding activity against MDM2 and/or MDMX (Table 1). The previously identified dual-specificity peptide inhibitor PMI and Nutlin-3a were used as positive controls, which yielded respective Ki values for MDM2 of 3.0 and 5.1 nM, and for MDMX of 4.9 nM and 1.54 μM, similar to the values reported in the literature.23,29 Two compounds, NSC142515 (S1) and NSC623731 (S2) (Table 1), derived from the two different models, emerged as the best dual inhibitors tested. NSC142515 bound to MDM2 and MDMX with respective Ki values of 2.13 and 4.50 μM, and for NSC623731 the Ki values were 6.57 and 11.25 μM, respectively (Fig. 4).
Table 1 Chemical structures and MDM2/MDMX inhibitory activities of the hits from virtual screening
Compound ID |
Structure |
Pharmacophore model |
NSC number |
A log P |
MW |
Ki (μM) MDM2 |
Ki (μM) MDMX |
S1 |
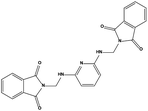 |
1 |
142515 |
3.16 |
427.41 |
2.13 ± 0.21 |
4.50 ± 0.54 |
S2 |
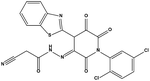 |
2 |
623731 |
3.8 |
500.31 |
6.42 ± 1.37 |
11.25 ± 2.27 |
S3 |
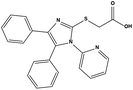 |
2 |
289919 |
5.13 |
387.45 |
17.36 ± 3.51 |
72.20 ± 5.86 |
S4 |
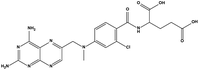 |
2 |
149506 |
1.5 |
488.88 |
38.82 ± 2.26 |
23.06 ± 1.58 |
S5 |
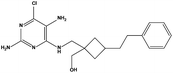 |
2 |
676363 |
3.09 |
361.87 |
30.98 ± 3.25 |
>200 |
S6 |
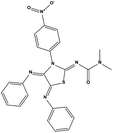 |
2 |
671360 |
4.39 |
472.52 |
48.90 ± 4.20 |
>200 |
S7 |
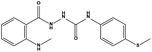 |
1 |
270117 |
1.91 |
330.4 |
56.03 ± 4.73 |
>200 |
S8 |
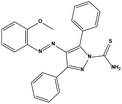 |
2 |
128646 |
6.45 |
413.49 |
77.15 ± 6.16 |
>200 |
S9 |
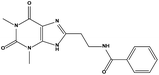 |
1 |
14143 |
0.61 |
327.34 |
92.97 ± 8.46 |
>200 |
S10 |
 |
1 |
282763 |
2.88 |
317.34 |
135.58 ± 6.97 |
>200 |
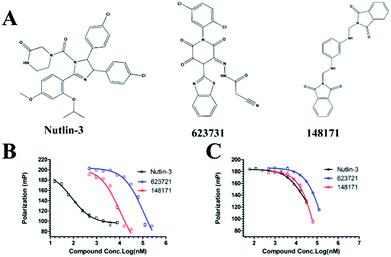 |
| Fig. 4 Chemical structures of potential inhibitors from in silico screening. A FP-based binding assay using SynMDM2 (25-109)/SynMDMX (24-108) and a p53-based peptide labeled with a fluorescence tag was used to quantitate the binding affinities of the compounds with MDM2/MDMX and to disrupt the interaction between MDM2 and p53. Nutlin-3, NSC623721 and NSC148171 yielded Ki values for MDM2 competitive antagonism of 0.0053 ± 0.0013, 6.42 ± 0.006 and 0.62 ± 0.014 μM, respectively. Nutlin-3, NSC623721 and NSC148171 inhibited TAMRA-PMI binding to MDMX with Ki values of 1.23 ± 0.010, 11.25 ± 0.006 and 4.6 ± 0.011 μM, respectively. Nutlin-3 is a positive control. Values represent the mean ± SEM of triplicate experiments. | |
Hit-based substructure search
The two scaffolds (NSC142515 and NSC623731) representing novel chemotypes for dual inhibition of the p53-MDM2/MDMX interactions were selected for hit-based substructure search in the NCI database, yielding three analogs of NSC142515 (HS1-3) and five analogs of NSC623731 (HS4-8) available for bioactivity evaluation (Table 2). In the first set of three analogs, only HS1 showed improved binding to MDM2 by 3-fold (Ki = 0.62 μM) while maintaining the same binding affinity for MDMX as NSC142515 (Fig. 4). NSC142515 differs from HS1 by a single atom in the scaffold, with a pyridine ring in the former replaced by a phenyl structure in the latter (Table 2). Interestingly, HS2, where the pyridine ring of NSC142515 is replaced by a carbonyl structure, displayed little binding to MDMX at 200 μM and reduced activity against MDM2 (10.4 μM), indicative of the importance of an aromatic center in the compound for maintaining its optimal interactions with MDM2 and MDMX. Although the substructure search turned up 5 active analogs of NSC623731 against MDM2 and/or MDMX, none of them showed improved binding to either protein compared with the parent compound. It should be pointed out that due to the limited availability from the NCI of the analogs of both NSC142515 and NSC623731, structure-to-activity relationship studies of more compounds from commercial sources or custom-synthesis may be needed to obtain more potent dual inhibitors of MDM2 and MDMX (Table 3).
Table 2 Inhibitory Activity of Compounds HS1–HS3 for MDM2/MDMX competitive binding
Compound ID |
Structure |
NSC number |
A log P |
MW |
Ki (μM) MDM2 |
Ki (μM) MDMX |
S1 |
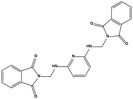 |
142515 |
3.16 |
427.41 |
2.13 ± 0.21 |
4.50 ± 0.54 |
HS1 |
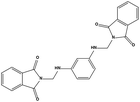 |
148171 |
3.07 |
426.42 |
0.62 ± 0.21 |
4.60 ± 1.17 |
HS2 |
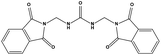 |
179406 |
1.23 |
378.34 |
10.41 ± 2.37 |
>200 |
HS3 |
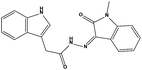 |
111575 |
1.64 |
332.36 |
20.48 ± 3.22 |
>200 |
Table 3 Inhibitory activity of compounds HS4–HS8 for MDM2/MDMX competitive binding
Compound ID |
Structure |
NSC number |
A log P |
MW |
Ki (μM) MDM2 |
Ki (μM) MDMX |
S2 |
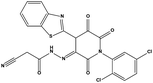 |
623731 |
3.8 |
500.31 |
6.42 ± 1.37 |
11.25 ± 2.27 |
HS4 |
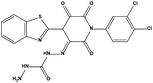 |
623044 |
3.02 |
491.31 |
14.14 ± 1.19 |
>200 |
HS5 |
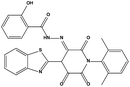 |
631520 |
4.97 |
512.54 |
28.05 ± 1.64 |
>200 |
HS6 |
 |
623047 |
5.81 |
537.55 |
32.74 ± 2.85 |
143.80 ± 8.17 |
HS7 |
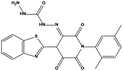 |
623043 |
2.78 |
450.47 |
40.82 ± 3.37 |
73.14 ± 5.61 |
HS8 |
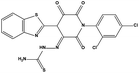 |
631519 |
3.83 |
492.36 |
70.14 ± 8.12 |
25.20 ± 2.28 |
Insight into the binding modes of active compounds against MDM2/MDMX
The detailed interactions of HS1 (NSC148171) and NSC623731 with MDM2/MDMX were modeled (Fig. 5). Compared with the known dual inhibitors, these two compounds share high similarities in their bound states. As shown in Fig. 5A, both NSC148171 (derived from pharmacophore model 1) and RO-2443 (co-crystallized ligand) form strong H-bonds with Gln72A and Arg65A in MDM2. RO-2443 forms two H-bonds with Gln72A, whereas NSC148171 only forms one H-bond with Gln72A. This may explain the difference in activity between RO-2443 (IC50 = 33 nM)26 and NSC148171 (Ki = 620 nM) (Fig. 5A and B). Although both NSC148171 and RO-2443 form H-bonds with Gln71A in MDMX (PDB CODE: 3U15), NSC148171 only forms one H-bond with Gln71A, likely leading to a lower binding activity of NSC148171 (IC50 = 41 nM for RO-2443 versus Ki = 4.6 μM for NSC148171). Of note, NSC148171 forms an additional H-bond with Tyr-66A, but its energetic importance remains unclear.
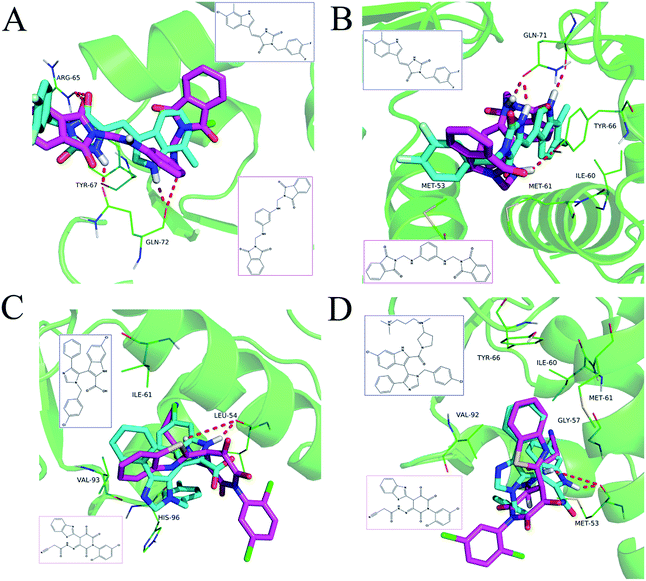 |
| Fig. 5 Detailed binding modes of dual inhibitors with MDM2 and MDMX. (A) The comparative binding modes of NSC148171 (magenta) and RO-2443 (crystalized ligand, cyan) with MDM2 (PDB-ID: 3VBG). ARG65 and GLN72 can both form strong hydrogen bonds with NSC148171 and RO-2443. However, TYR-67 can only form a hydrogen bond with RO-2443. (B) Detailed comparative binding modes of NSC148171 (magenta) and RO-2443 (crystalized ligand, cyan) with MDMX (PDB-ID: 3U15). GLN71 formed strong hydrogen bonds with both NSC148171 and RO-2443. While RO-2443 can form a second hydrogen bond with GLN71, NSC148171 can form a hydrogen bond with TYR66. (C) The comparative binding modes of NSC623731 (magenta) and K23 (crystalized ligand, cyan) with MDM2 (PDB-ID: 3LBK). (D) Detailed comparative binding modes of NSC623731 (magenta) and WW8 (crystalized ligand, cyan) with MDMX (PDB-ID: 3LBJ). To simplify the binding site, some atoms in WW8 were deleted. Two residues, LEU54 (MDM2) and MET53 (MDMX), formed strong hydrogen bonds with both NSC623731 and crystalized ligands. | |
As shown in Fig. 5C, Leu54 in MDM2 (PDB CODE: 3LBK) forms a strong H-bond with NSC623731 (derived from pharmacophore model 2) and K23 (co-crystallized ligand), likely contributing to the activity of NSC623731 (Ki = 6.57 μM) to some extent. Unlike K23, NSC623731 lacks critical hydrophobic interactions with Leu54A and His96A of MDM2, a possible reason for its lower activity than K23 (Ki = 0.96 μM). Both NSC623731 and WW8 (co-crystallized ligand) form an H-bond with Met53 of MDMX (PDB CODE: 3LBJ) (Fig. 5D), and project hydrophobic groups toward Met61, Ile60, Gly57, Val92 and Tyr66, thereby explaining their similar activities (Ki = 11.25 μM for NSC623731, Ki = 11 μM for WW8). Taken together, our modeling studies demonstrate that site-specific H-bonding and hydrophobic interactions contribute to the binding of our inhibitors to MDM2 and MDMX. These structural features of interaction may facilitate further screening for more potent dual inhibitors of the p53-MDM2/MDMX interactions.
In vitro antiproliferative activity
To investigate the in vitro antiproliferative activity of all hits, a human primary glioblastoma cell line, U87 (wild-type p53) was used for assaying with nutlin-3 as a positive control. The percent cell viabilities of compounds at 100 μM versus control were shown in Fig. 6A. In general, these dual inhibitors displayed varying activity against U87. As shown in Fig. 6B, with decreased competitive binding affinity against MDM2 and MDMX from S1 to S4, there exist rough decreased inhibitory effect against U87 cell line, which reflected the negative correlation between Ki value and inhibitory activity. Similar phenomenon can also be noticed in same series of compounds, such as from S6 to S9 (selectively against MDM2), from HS1 to HS3 and from HS4 to HS8 (against MDM2). Whereas, the S5, S10 and HS4 were an exception, we inferred that there were other target proteins of these compounds to cause the antiproliferative activity. Of note, the highly active S6 compound contains a nitro group, which can be cytotoxic in vitro.30 Whether or not the nitro group contributes to the strong activity of S6 remains to be further investigated.
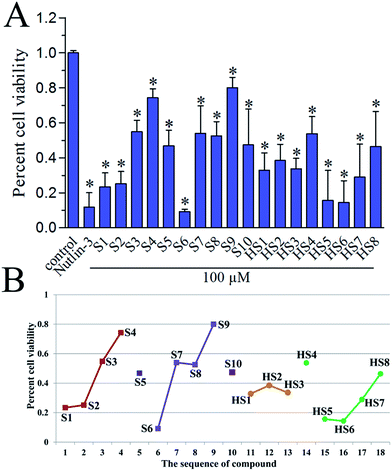 |
| Fig. 6 (A) In vitro antiproliferative activity of S1–S10 and HS1–HS8 against U87 cell line (wild-type p53), as determined by the standard MTS cell viability assay. Nutlin-3 (50 μM) was used as a positive control. Each column is the average of two separate experiments. (B) The correlation of the sequence of compounds' Ki value with their percent cell viability. | |
PAINS remover
PAINS risks of our validated hits were predicted as described.23 S8 (128646) and HS3 (111575) were identified as potential PAINS, suggesting that S8 and HS3 may be false positive hits and not ideal scaffolds for further structural optimization.
Conclusions
In the present study, we developed a virtual screening method coupled with hit-based substructure search strategy to identify dual inhibitors of the p53-MDM2/MDMX interactions, and obtained a series of novel scaffolds with moderate inhibitory activity against both target proteins. The most active compounds from different pharmacophores, NSC623721 and NSC148171, showed low micromolar binding affinity for both MDM2 and MDMX, significantly lower than that of known dual inhibitors discovered by in silico screening methods reported.16,17 Molecular docking analysis of the binding of NSC623721 and NSC148171 with MDM2 and MDMX has yielded structural information useful for improved in silico screening and chemical modification. All hits were demonstrated to possess anti-proliferative activity of human primary glioblastoma cell line U87 (wild-type p53) and the activities roughly correlated with their Ki values. Additional SAR studies of both compounds are necessary to further augment their activity against MDM2 and MDMX, leading ultimately to the discovery of potent dual inhibitors with therapeutic efficacy for cancer treatment. Our new strategy based on pharmacophore models and molecular docking will facilitate future virtual screening for novel dual inhibitors of the p53-MDM2/MDMX interactions for anticancer therapy.
Acknowledgements
This work was financially supported by the National Natural Science Foundation of China (No. 81573396, No. 21402235).
Notes and references
- A. Talevi, Front. Pharmacol., 2015, 6, 205 CrossRef PubMed.
- Z. Tan, R. Chaudhai and S. Zhang, ChemMedChem, 2016, 11, 1211–1218 CrossRef CAS PubMed.
- A. L. Hopkins, Nat. Chem. Biol., 2008, 4, 682–690 CrossRef CAS PubMed.
- M. A. Yildirim, K. I. Goh, M. E. Cusick, A. L. Barabasi and M. Vidal, Nat. Biotechnol., 2007, 25, 1119–1126 CrossRef CAS PubMed.
- C. Zhuang, C. Sheng, W. S. Shin, Y. Wu, J. Li, J. Yao, G. Dong, W. Zhang, Y. Y. Sham, Z. Miao and W. Zhang, Oncotarget, 2014, 5, 10830–10839 CrossRef PubMed.
- A. Petrelli and S. Giordano, Curr. Med. Chem., 2008, 15, 422–432 CrossRef CAS PubMed.
- M. Pazgier, M. Liu, G. Zou, W. Yuan, C. Li, C. Li, J. Li, J. Monbo, D. Zella, S. G. Tarasov and W. Lu, Proc. Natl. Acad. Sci. U. S. A., 2009, 106, 4665–4670 CrossRef CAS PubMed.
- X. Chen, L. Tai, J. Gao, J. Qian, M. Zhang, B. Li, C. Xie, L. Lu, W. Lu and W. Lu, J. Controlled Release, 2015, 218, 29–35 CrossRef CAS PubMed.
- C. Prives and S. W. Lowe, Nature, 2015, 525, 199–200 CrossRef CAS PubMed.
- A. Lemos, M. Leao, J. Soares, A. Palmeira, M. Pinto, L. Saraiva and M. E. Sousa, Med. Res. Rev., 2016, 36, 789–844 CrossRef CAS PubMed.
- Y. Haupt, R. Maya, A. Kazaz and M. Oren, Nature, 1997, 387, 296–299 CrossRef CAS PubMed.
- M. Liu, M. Pazgier, C. Li, W. Yuan, C. Li and W. Lu, Angew. Chem., Int. Ed., 2010, 49, 3649–3652 CrossRef CAS PubMed.
- M. Liu, C. Li, M. Pazgier, C. Li, Y. Mao, Y. Lv, B. Gu, G. Wei, W. Yuan, C. Zhan, W. Y. Lu and W. Lu, Proc. Natl. Acad. Sci. U. S. A., 2010, 107, 14321–14326 CrossRef CAS PubMed.
- F. Toledo, K. A. Krummel, C. J. Lee, C. W. Liu, L. W. Rodewald, M. Tang and G. M. Wahl, Cancer Cell, 2006, 9, 273–285 CrossRef CAS PubMed.
- D. Pei, Y. Zhang and J. Zheng, Oncotarget, 2012, 3, 228–235 CrossRef PubMed.
- S. Golestanian, A. Sharifi, G. M. Popowicz, H. Azizian, A. Foroumadi, A. Szwagierczak, T. A. Holak and M. Amanlou, Life Sci., 2016, 145, 240–246 CrossRef CAS PubMed.
- A. Zaytsev, B. Dodd, M. Magnani, C. Ghiron, B. T. Golding, R. J. Griffin, J. Liu, X. Lu, I. Micco, D. R. Newell, A. Padova, G. Robertson, J. Lunec and I. R. Hardcastle, Chem. Biol. Drug Des., 2015, 86, 180–189 CAS.
- C. Zhan, L. Zhao, X. Wei, X. Wu, X. Chen, W. Yuan, W. Y. Lu, M. Pazgier and W. Lu, J. Med. Chem., 2012, 55, 6237–6241 CrossRef CAS PubMed.
- S. Wetzel, K. Klein, S. Renner, D. Rauh, T. I. Oprea, P. Mutzel and H. Waldmann, Nat. Chem. Biol., 2009, 5, 581–583 CrossRef CAS PubMed.
- X. Q. Lewell, D. B. Judd, S. P. Watson and M. M. Hann, J. Chem. Inf. Comput. Sci., 1998, 38, 511–522 CrossRef CAS PubMed.
-
(a) S. Chen, H. Jiang, Y. Cao, Y. Wang, Z. Hu, Z. Zhu and Y. Chai, Sci. Rep., 2016, 6, 24245 CrossRef PubMed;
(b) S. Chen, S. Wu, W. Li, X. Chen, X. Dong, G. Tan, H. Zhang, Z. Hong, Z. Zhu and Y. Chai, Mol. BioSyst., 2014, 10, 3310–3321 RSC.
- A. H. Cory, T. C. Owen, J. A. Barltrop and J. G. Cory, Cancer Commun., 1991, 3, 207–212 CAS.
- J. B. Baell and G. A. Holloway, J. Med. Chem., 2010, 53, 2719–2740 CrossRef CAS PubMed.
- H. A. Zhong, V. Mashinson, T. A. Woolman and M. Zha, Curr. Top. Med. Chem., 2013, 13, 1290–1307 CrossRef CAS PubMed.
- Y. Lu, Z. Nikolovska-Coleska, X. Fang, W. Gao, S. Shangary, S. Qiu, D. Qin and S. Wang, J. Med. Chem., 2006, 49, 3759–3762 CrossRef CAS PubMed.
- C. Zhuang, Z. Miao, L. Zhu, G. Dong, Z. Guo, S. Wang, Y. Zhang, Y. Wu, J. Yao, C. Sheng and W. Zhang, J. Med. Chem., 2012, 55, 9630–9642 CrossRef CAS PubMed.
- Q. A. Al-Balas, H. A. Amawi, M. A. Hassan, A. M. Qandil, A. M. Almaaytah and N. M. Mhaidat, Pharmaceuticals, 2013, 6, 700–715 CrossRef CAS PubMed.
-
(a) B. Graves, T. Thompson, M. Xia, C. Janson, C. Lukacs, D. Deo, P. Di Lello, D. Fry, C. Garvie, K. S. Huang, L. Gao, C. Tovar, A. Lovey, J. Wanner and L. T. Vassilev, Proc. Natl. Acad. Sci. U. S. A., 2012, 109, 11788–11793 CrossRef CAS PubMed;
(b) G. M. Popowicz, A. Czarna, S. Wolf, K. Wang, W. Wang, A. Domling and T. A. Holak, Cell Cycle, 2010, 9, 1104–1111 CrossRef CAS PubMed.
- B. Graves, T. Thompson, M. Xia, C. Janson, C. Lukacs, D. Deo, P. Di Lello, D. Fry, C. Garvie, K. S. Huang, L. Gao, C. Tovar, A. Lovey, J. Wanner and L. T. Vassilev, Proc. Natl. Acad. Sci. U. S. A., 2012, 109, 11788–11793 CrossRef CAS PubMed.
- D. E. Beck, M. Abdelmalak, W. Lv, P. N. Reddy, G. S. Tender, E. O'Neill, K. Agama, C. Marchand, Y. Pommier and M. Cushman, J. Med. Chem., 2015, 58, 3997–4015 CrossRef CAS PubMed.
Footnotes |
† Electronic supplementary information (ESI) available. See DOI: 10.1039/c7ra00473g |
‡ Si Chen and Xiang Li contributed equally to this work. |
|
This journal is © The Royal Society of Chemistry 2017 |
Click here to see how this site uses Cookies. View our privacy policy here.