DOI:
10.1039/C6RA27608C
(Paper)
RSC Adv., 2017,
7, 13947-13956
Inhibitory effect of hydrophobic fullerenes on the β-sheet-rich oligomers of a hydrophilic GNNQQNY peptide revealed by atomistic simulations†
Received
1st December 2016
, Accepted 17th February 2017
First published on 2nd March 2017
Abstract
Increasing numbers of experiments show that carbon nanoparticles can significantly influence protein fibrillation, a process associated with many human amyloidosis. Our previous studies demonstrate that fullerenes and carbon nanotubes can markedly inhibit the formation of β-sheet-rich oligomers and amyloid fibrils of the hydrophobic KLVFFAE peptide, the central hydrophobic core fragment of Alzheimer's amyloid-β protein, mostly through hydrophobic and aromatic-stacking interactions. In this study, the effect of hydrophobic fullerene C60 on the oligomeric structures of the hydrophilic GNNQQNY peptide, the key amyloid-forming fragment of yeast prion protein Sup35, is investigated by extensive replica exchange molecular dynamics simulations. Our simulations, starting from eight random chains, show that fullerene can greatly impede the β-sheet formation of the GNNQQNY peptide and induce oligomer shifting from various β-sheet-rich structures to disordered coil conformations. Strikingly, fullerenes completely prevent fibril-like bilayer β-sheets, formed by GNNQQNY peptides alone. We find that C60 molecules interact strongly with the nonpolar aliphatic groups of polar residues N3, Q4 and Q5 and increase the water exposure of the peptide backbone, thus block the inter-peptide N3–Q4, Q4–Q4 and Q4–Q5 interactions which are crucial for oligomerization and β-sheet formation. These results, together with our previous studies on the KLVFFAE peptide, suggest that hydrophobic fullerenes could inhibit the aggregation of both hydrophobic and hydrophilic peptides. This finding may offer new clues for the design of therapeutic agents against different types of amyloidosis.
Introduction
Many proteins have an intrinsic tendency to aggregate into amyloid deposits that are hallmarks of numerous fatal diseases such as Alzheimer's, Parkinson's, Huntington's and Creutzfeldt–Jakob diseases.1–3 In spite of different protein composition, the main components of these amyloid deposits are amyloid fibrils that display a common cross-β structure with the β-strands perpendicular to and the inter-strand hydrogen bonds parallel to the fibril axis.4 This finding indicates that a general principle may govern amyloid fibril formation.5 Most importantly, increasing evidence suggests that small oligomers formed in the early stage of aggregation, rather than the fibrils themselves, are the most cytotoxic species in neurodegenerative diseases.6,7 However, due to the transient nature of oligomers, their atomic-level structures and the molecular mechanisms of formation, toxicity, and inhibition are still not fully understood.8 Numerous experimental studies demonstrated that hydrophobic fragments (such as KLVFFAE in the full length amyloid-β (Aβ),9 VQIVYK in tau protein10 and GVIGIAQ in superoxide dismutase (SOD1)11) are usually the important triggers for the fibrillation of full-length proteins, while polar segments (such as GNNQQNY in yeast prion Sup35 protein12 and polyglutamine sequence in Huntingtin protein13) also play key roles on protein aggregation. Most interestingly, these segments themselves can also form amyloid fibrils that displays many of the common characteristics as the fibrils formed by the full-length proteins.9–12,14 Among them, GNNQQNY peptide serves as a good model for studying the early stage of aggregation due to its availability of the atomic fibril-like structure determined by Eisenberg et al. using X-ray microcrystallography.15 The fibril-like structure from the GNNQQNY microcrystal contains a double β-sheet, with each sheet formed by main chain hydrogen bonds, and a dry, tightly self-complementing steric zipper formed by side chains protruding from the sheets.15 Solid state NMR experiments indicate significant structural differences between the structures of crystalline and fibrillary forms of this peptide, with a clearly increased complexity in the fibril structure.16–19 Very recently, two forms of high-resolution structures of GNNQQNY nanocrystals were obtained by Eisenberg et al. using cryo-EM method20 and they display different space groups: P21 and P212121.
Complementary to experiments, molecular dynamics (MD) simulations can provide the oligomerization dynamics and thermodynamics of GNNQQNY peptides at the atomic level. By using coarse-grained or atomistic MD simulations, several research groups investigated the structural stability of different sizes of preformed fibril-like oligomers,21–23 the oligomerization dynamics/kinetics of the peptide,24–31 the growth and assembly dynamics of preformed protofibrils,32,33 and the energetics and thermodynamics of oligomers (from dimers to hexamers).34–36 Two common findings from these studies are: (1) for preformed fibril-like oligomers, interstrand backbone–backbone and side chain–side chain hydrogen bonds are the main forces to stabilize a β-sheet, and steric zipper interactions between the side chains of N2, Q4, and N6 in two different sheets hold the sheets together; (2) irrespective of the force field used, antiparallel β-sheet dimers and trimers are populated in the early stage of aggregation, although β-strands in GNNQQNY fibrils are in parallel alignment.15,20 These computational studies have enhanced our understanding of the physical forces stabilizing the preformed fibrils and the formation pathway of small oligomers. However, the conformational ensembles of oligomers larger than hexamers remain to be determined at the atomic level.
Finding effective inhibitors that prevent the formation of toxic β-sheet-rich aggregates and revealing the inhibitory mechanism are important for the development of therapeutic agents. Researchers have found that nanoparticles (such as polymeric nanoparticles,37 gold nanoparticles38 and carbon nanoparticles39), peptides,40,41 small molecules,41–43 and antibodies41 can significantly interfere with the aggregation process of proteins. Among them, carbon nanoparticles (including fullerenes, carbon nanotubes, and graphene oxides) have attracted considerable attention,39,44,45 due to their good biocompatibility, low cytotoxicity and high capacity to cross blood brain barriers.46–48 Fullerene C60 with its 0.7 nm wide cage, presents the suitable size and shape for the formation of stable complexes with proteins.49 The presence of both five and six-membered rings in C60 (one C60 molecule containing 12 pentagons and 20 hexagons) suggests strong fullerene–protein interaction through aromatic stackings.50 Increasing experimental studies have demonstrated that fullerene C60 and its derivatives have remarkable anti-amyloid capacity and neuroprotective potentials.51–57 On the computational side, it has been reported that fullerene inhibits protein fibrillation or disrupts preformed fibrils by mostly binding to regions consisting of hydrophobic or aromatic residues.58–61 In particular, our recent work suggests that fullerenes prevent the fibrillation of hydrophobic KLVFFAE (Aβ(16–22)) peptide (the central hydrophobic core fragment of Aβ protein) by inhibiting the formation of β-sheet oligomers.50 However, the effect of the fullerene C60 on the aggregation of hydrophilic peptides remains elusive.
In this work, as a first step to understand the influence of hydrophobic fullerene on the aggregation of hydrophilic peptides, we investigated the conformational ensemble of GNNQQNY oligomers in the absence and presence of C60 molecules by performing extensive atomistic replica exchange molecular dynamics (REMD) simulations starting from multiple random peptide chains. Similar to our recent REMD study of Aβ(16–22) system,50 eight GNNQQNY peptide chains and three C60 molecules were simulated in the GNNQQNY system. Our simulations show that in the absence of C60 molecules, GNNQQNY octamers adopt a diverse ensemble of conformations, including predominant disordered coil-rich states, low-populated disordered β-sheet-rich states and ordered bilayer β-sheets. However, in the presence of C60, β-sheet-rich conformations are greatly reduced, in particular, the ordered bilayer β-sheets are completely suppressed. Binding probability and binding energy analyses demonstrate that C60 interacts strongly with the nonpolar aliphatic groups of polar residues N3, Q4 and Q5, thus interferes with the inter-peptide N3–Q4, Q4–Q4 and Q4–Q5 interactions which are important for β-sheet formation and aggregation of GNNQQNY peptide. The role of water molecules on peptide–peptide interactions are also discussed.
Models and methods
Initial conformations of GNN and GNN + C60 systems
We investigated two different systems: GNNQQNY octamer alone (GNN for short) and GNNQQNY octamer in the presence of three C60 molecules (GNN + C60 for short). The two termini of each GNNQQNY peptide chain are charged (NH3+ and COO−) to mimic the neutral experimental pH condition.62 Each system consists of eight peptide chains with random coil conformation for each chain. The eight GNNQQNY chains were placed randomly in a 6.7 × 6.7 × 6.7 nm3 box filled with SPC water molecules. Three C60 molecules were randomly displaced in the peptide system. The peptide concentrations in the two systems are 36.9 mg ml−1, which is close to the experimental peptide concentration for GNNQQNY fibril formation.63 The starting states of the two systems are given in Fig. S1 (ESI†).
REMD simulations
We conducted one 250 ns REMD64 simulation for each system using the GROMACS-4.5.3 software package,65 following the procedure adopted for our previous REMD simulations.50,66–68 The GROMOS96 43a1 force field69 was used, in accordance with previous computational studies of GNNQQNY aggregation.22–24,26 As demonstrated by a number of computational studies,27,35,70–73 REMD method is useful for investigating the aggregation of a series of peptides such as GNNQQNY27,35 and KLVFFAE.68 Moreover, our previous works on Aβ(16–22) aggregation showed that the predictions from REMD simulations were confirmed by experiments.50,74 Thus, we employed the REMD method to investigate the octameric structures of GNNQQNY peptide and the influence of C60 nanoparticles. The REMD simulations were performed in the NPT ensemble using 48 replicas (250 ns per replica) at temperatures ranging from 310 K to 411 K. Electrostatic interactions were calculated using particle mesh Ewald algorithm75 with a real space cut-off of 1.0 nm. van der Waals interactions were calculated using a cutoff of 1.4 nm. Constrains were applied for bond lengths of peptides using Linear Constraint Solver (LINCS) algorithm76 and for those of water molecules using SHAKE algorithm,77 allowing an integration time step of 2 fs. The solute and solvent were separately coupled to external temperature and pressure baths using velocity rescaling78 and Parrinello–Rahman79 coupling methods. The temperature- and pressure-coupling constants are 0.1 and 1.0 ps, respectively. Conformations were saved every 2 ps. The swap time between two neighboring replicas is 2 ps. The average acceptance ratio is ∼21%, as shown in Fig. S2 (ESI†).
Analysis methods
The DSSP program was used to calculate the secondary structure. The probability distribution of β-sheet size was calculated to characterize the structural feature of aggregated states. For an i-stranded β-sheet, the size of this β-sheet is the same as the number of β-strand in the sheet. For example, the β-sheet size of a three-stranded β-sheet is three. Two peptide chains are considered to form a β-sheet if (i) at least two consecutive residues in each chain visit the β-sheet state and (ii) the two chains form at least two backbone hydrogen bonds (H-bonds).68,80 One H-bond is considered as formed if the N⋯O distance is less than 0.35 nm and the N–H⋯O angle is greater than 150°. The percentage Pi of the β-sheet with size = i is calculated using the following formula.
here, ni is the number of β-sheet with size = i and N is the total number of chains (N = 8). Following our previous work,68,80 we used the connectivity length (CL) to characterize the structural orderness of the octamers. For each octamer, the CL is defined as the sum over the square root of the β-sheet size and the number of chains with a random coil conformation, i.e.
. For example, the CL of an octamer consisting of one four-stranded β-sheet and four random chains without β-sheets is
, and the CL of an octamer consisting of two four-stranded β-sheets is
. Thus, the larger the connectivity length is, the more disordered the octamer becomes. We also calculated the ratio of parallel/antiparallel (P/AP) alignments in a β-sheet. The two β-sheet-forming chains are considered to form an antiparallel (parallel) β-sheet if the scalar product of their end-to-end unit vector is less than −0.7 (greater than 0.7).
Potential of mean force (PMF) was constructed using the relation – RT
ln
H(x, y), where H(x, y) is the histogram of two selected reaction coordinates: the radius of gyration (Rg) of the octamer and the number of hydrogen bonds (number of H-bonds). Here, the H-bond number denotes the total number of intra- and inter-peptide H-bonds. The Daura cluster analysis method81 was used to classify the conformations sampled in the REMD simulations with a Cα-root-mean-square-deviation (Cα-RMSD) cutoff of 0.3 nm. The inter-peptide interactions and the peptide–C60 interactions were estimated respectively by the contact probability of residue–residue and residue–C60 pairs. Here, a contact is defined when the aliphatic carbon atoms of two nonsequential side chains (or main chains) come within 0.54 nm or any other atoms of two nonsequential side chains (or main chains) lie within 0.46 nm.68
We estimated the binding free energy between each amino acid residue and a C60 molecule using molecular mechanics/linear Poisson–Boltzmann surface area (MM/PBSA) method implemented for the GROMACS package (g_mmpbsa tool).82 It is challenging to calculate the conformational entropy for systems with large conformational changes using MM/PBSA.82,83 In this study, the binding free energy is the relative binding energy in which the molecular mechanics potential energy in a vacuum and the solvation free energy were included, while the contribution of conformational entropy was ignored, in accordance with a number of previous computational studies.43,50,61,84 Namely, the binding free energy (ΔGbinding) was calculated as: ΔGbinding = ΔEMM + ΔGsol. The ΔEMM is the potential energy in vacuum and ΔGsol (=ΔGpolar + ΔGnonpolar) is the solvation free energy that is required to transfer a solute from vacuum into the solvent. The ΔGpolar and ΔGnonpolar are respectively the polar and non-polar contributions to the solvation free energy. The ΔGpolar was calculated by the PB model and the Gnonpolar was estimated by the solvent accessible surface area. For comparison, we also calculated the contact probability between each residue and C60. The VMD program85 was used for trajectory visualization and for graphical structure analysis.
Results and discussion
Convergence check of the REMD simulations
We examined the convergence of the two 250 ns REMD simulations by comparing the following parameters within two different time intervals using the 100–175 ns and 175–250 ns data at 310 K for the two systems. As shown in Fig. S3,† the probabilities of dominant secondary structure (coil, β-sheet and β-bridge) of each residue are quite similar between the two time periods. The distribution of total number of H-bonds and that of side chain contact number overlap very well within the two independent time intervals in Fig. S4(a–d).† The Rg of octamers in the two systems also display similar distributions within the two intervals in Fig. S4(e–f).† For comparison, we also calculated the three quantities using the first 100 ns data and added the results in Fig. S4.† Their distributions (black curves) exhibit significant differences from those within the last 150 ns. Taken together, these results suggest that our REMD simulation for the two systems are reasonably converged after 100 ns. Unless specified, all the REMD simulation results presented below are based on the last 150 ns (t = 100–250 ns) simulation data generated at 310 K.
Fullerene C60 markedly reduces the β-sheet content and enhances the coil propensity of GNNQQNY peptide
The influence of C60 on the secondary structure propensity was monitored by calculating the average probability of each secondary structure (coil, β-sheet, β-bridge, bend, turn and helix) over all residues and the residue-based secondary structure probability of GNNQQNY aggregates in the GNN and GNN + C60 systems. As shown in Fig. 1(a), coil and β-sheet contents are dominant, but both of them are quite different in the two systems. Without C60, the coil and β-sheet probabilities are 62.7% and 29.1%, respectively. In the presence of C60, the coil content markedly increases to 75.4%, while the β-sheet content significantly decreases to 13.5%. The β-bridge and bend contents are quite similar in the two systems. Note that turn and helix contents (<1%) are negligible (data not shown). These results indicate that fullerene C60 can significantly inhibit β-sheet formation and promote coil formation of hydrophilic GNNQQNY peptide.
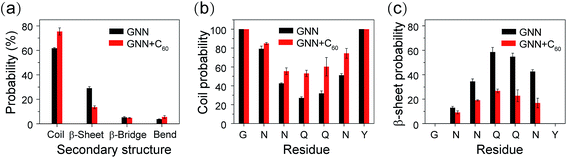 |
| Fig. 1 Secondary structure analysis of GNNQQNY oligomers in GNN and GNN + C60 systems. (a) The average probability of each secondary structure (including coil, β-sheet, β-bridge and bend) over all residues. The coil (b) and β-sheet (c) probability as a function of amino acid residue. | |
Fig. 1(b and c) shows the propensities of coil and β-sheet as a function of amino acid residue. In the GNN system, residues G1, N2, N3, N6 and Y7 predominantly adopt coil conformations, while middle residues Q4 and Q5 have a high β-sheet propensity (58.6% for Q4 and 54.7% for Q5). We also note that N6 adjacent to Y7 exhibits a significantly higher β-sheet probability than other Asn residues (N2 and N3). This observation indicates that Y7 has a positive effect on the β-sheet formation of its adjacent residues. In GNN + C60 system, the introduction of fullerene C60 into GNNQQNY octamers decreases the β-sheet probability of residues N3, Q4, Q5 and N6 from 34.6–58.6% to 16.8–26.7%, while increases the coil percentages from 27.1–42.5% to 53.1–74.4%. These results demonstrate that C60 nanoparticles can reduce effectively the β-sheet propensity of residues N3, Q4, Q5 and N6.
GNNQQNY octamers adopt predominantly disordered conformations and to a much lesser extent ordered bilayer β-sheet structures, while the presence of C60 completely suppresses the ordered bilayer β-sheets
We further investigated the influence of C60 on the atomic structures of GNNQQNY oligomers. We first calculated the probabilities of different sizes of β-sheets. As shown in Fig. 3(a), in the GNN system, the majority of the β-sheets are two-, three- and four-stranded β-sheets (having a probability of 32.2%, 10.9% and 9.5%, respectively). In the presence of C60, these three sizes of β-sheets have a significantly reduced probability (19.8%, 3.5% and 3.5%). Differences are more apparent for larger sizes of β-sheets. The probabilities of five-, six-, and seven-stranded β-sheets are respectively 4.9%, 1.3%, and 1.2% in GNN system, while they almost disappear in the GNN + C60 system.
Experimental studies by Eisenberg et al. showed that β-strands in GNNQQNY fibrils are in parallel alignment.15,20 It is interesting to examine whether the β-strands in GNNQQNY oligomers are also in parallel association. To this aim, we calculated the angle between two neighboring strands in all sizes of β-sheets and the parallel/antiparallel (P/AP) ratio as a function of β-sheet sizes in GNN system. As shown in Fig. 2(b), the P/AP ratio in two-stranded β-sheets is quite small (0.04), revealing that strands in two-stranded β-sheets are mostly in antiparallel association, consistent with previous simulation results.23,24,27,31,35 Interestingly, we find that the P/AP ratio becomes much larger in β-sheets with sizes larger than 2, implying that parallel arrangement of β-strands becomes favored when β-sheet sizes increase.
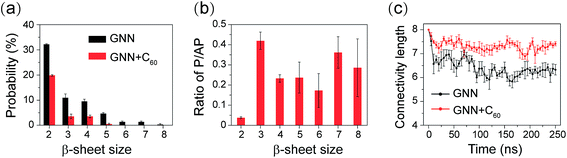 |
| Fig. 2 Analysis of different sizes of β-sheets and characterization of the structural orderness of GNNQQNY octamers in GNN and GNN + C60 systems. (a) Probability of different sizes of β-sheet. (b) The P/AP ratio as a function of β-sheet size in GNN system. (c) The connectivity length as a function of simulation time. | |
We then examined the structural orderness of GNNQQNY octamers in GNN and GNN + C60 systems by monitoring the time evolution of the connectivity length (CL) (Fig. 2(c)). The CLs of the octamers in the two systems decrease rapidly from the initial value of 8.0 respectively to 6.2 and 7.2 within the first 100 ns of the simulations and fluctuate around these two values during t = 100–250 ns. The large CL value in the GNN + C60 system compared to that in GNN system reveals that GNNQQNY octamers become much more disordered in the presence of C60 nanoparticles.
We also preformed cluster analysis on the GNNQQNY octamers in GNN and GNN + C60 systems. With a Cα-RMSD cutoff of 0.3 nm, the GNNQQNY conformations at 310 K were separated into 139 clusters in GNN system and 150 clusters in GNN + C60 system. The center conformations of the first eight most-populated cluster are shown in Fig. 3(a) and (b). These clusters represent 46.6% and 43.5% of all conformation of GNN and GNN + C60 systems. Without C60, as shown in Fig. 3(a), the first seven clusters are in mixed coil and β-sheet conformations, while cluster-8 is a bilayer β-sheet. Both parallel and antiparallel β-strand alignments are seen in these eight clusters. In the presence of C60, a majority of the eight clusters are in coil-rich conformations (Fig. 3(b)). These results indicate that C60 induces GNNQQNY to form disordered coil-rich aggregates, consistent with the CL analysis shown in Fig. 2(c).
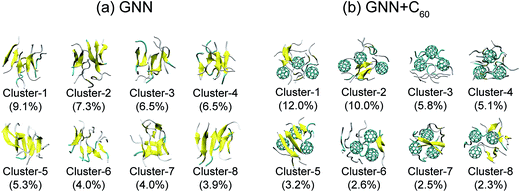 |
| Fig. 3 Representative structures for the first eight most-populated clusters of GNNQQNY octamer alone (a) and GNNQQNY octamer with three C60 molecules (b). | |
To have an overall view of the conformational distribution of GNNQQNY octamers in the two systems, we constructed in Fig. 4 the potential of mean force as a function of Rg and number of H-bonds of GNNQQNY octamers. It is noted that the free energy landscape is a hypersurface function of all the degrees of freedom of the system, while the two-dimensional PMF is a projection of the high-dimensional free energy landscape on two different reaction coordinates. Usually, the PMF is highly degenerate for a complex system involving peptide aggregation35,68,70,86 and it cannot discriminate between ordered and disordered conformations. As seen from Fig. 4, the global minimum energy basins of GNNQQNY octamers in the GNN and GNN + C60 systems are located at (Rg, number of H-bonds) values of (0.97 nm, 65) and (1.07 nm, 55), respectively. A decreased number of H-bonds and an increased value of Rg imply that C60 nanoparticles impede the formation of H-bonds and induce the formation of loosely packed aggregates, thus alter the whole free energy surface. We also constructed a PMF as a function of β-sheet probability and Rg of the octamers (Fig. S5†) and found that fullerenes result in a decreased β-sheet probability and an increased Rg of GNNQQNY oligomers.
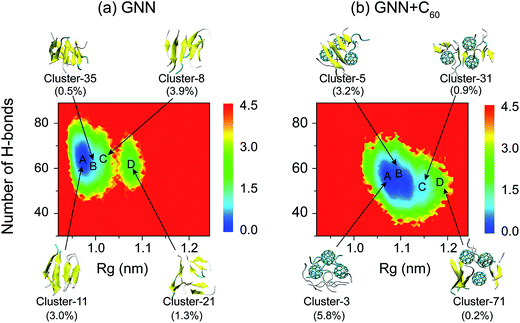 |
| Fig. 4 Potential of mean force (PMF) for GNNQQNY octamers in GNN (a) and GNN + C60 (b) systems. The PMF (in kcal mol−1) of GNNQQNY octamers plotted as a function of Rg and the number of H-bonds of GNNQQNY octamer. The top four most ordered conformations of octamers in GNN system and representative disordered coil-rich/β-sheet-rich conformations in GNN + C60 system are also given, along with their probabilities. | |
We also show in Fig. 4 the top four most ordered conformations of GNNQQNY octamers in GNN system and representative disordered coil-rich/β-sheet-rich conformations in GNN + C60 system as well as their locations. In the GNN system, the most ordered clusters are cluster-8, cluster-11, cluster-21 and cluster-35. Cluster-8 (3.9%) and cluster-11 (3.0%) correspond to bilayer β-sheet structures, although their β-strand arrangements are different (see Fig. S6(a–e)†). Cluster-35 corresponds to β-barrel structure with a very low probability (0.5%). Cluster-21 (1.3%) contains loosely packed β-sheet-rich conformations whose Rgs are larger than those of bilayer β-sheets and β-barrels. For GNN + C60 system, we present four representative disordered clusters: one cluster (cluster-3) corresponding to fully disordered coil conformations and the other three clusters (cluster-5, cluster-51 and cluster-71) corresponding to disordered β-sheet-rich conformations. The fully disordered coil conformations (cluster-3) are located in the global minimum energy basin. Different sizes of single layer β-sheets are seen in the disordered β-sheet-rich conformations, but they are separated by C60 molecules. This finding indicate that C60 molecules play the role as a breaker to block the formation of the fibril-like bi-layer β-sheet structures.
The GNNQQNY peptides in octamers display a hydration tendency similar to those in fibrils, while the addition of C60 molecules into GNN system increases the water exposure of backbone atoms
Experimental studies of GNNQQNY nanocrystals by Eisenberg et al. using X-ray microcrystallography15 and cryo-EM methods20 reported that, in the fibril-like nanocrystals, the polar groups of the side chains of residues N2, N3, Q5 and Y7 and the backbone of G1 and Y7 are hydrated by water molecules, while the side chains of residues Q4 and N6 are protected from exposing to water molecules. Thus, it is interesting to probe the hydration tendency of these residues in the early formed GNNQQNY oligomers and the influence of fullerenes. To that end, we calculated the number of water molecules within 0.35 nm from the backbone atoms or side chain atoms of each residue per peptide chain. The side chain of a glycine consists of a single hydrogen atom and this hydrogen atom is included implicitly in the Cα atom in the GROMOS force field. Thus, in the calculation, for residue G1, we considered the number of water molecules contacting with Cα atoms as the number of water molecules that have atomic contacts with the side chains of G1. Fig. 5 shows that, in the absence of fullerenes, the backbone atoms of G1 and Y7 have relatively larger number of contacting water molecules than those of other residues and the side chains of residues N2, Q5 and Y7 are more solvent-exposed than those of other residues. This finding indicates that the relative hydration propensity of amino acid residues of GNNQQNY peptide in early formed octamers is similar to those in amyloid fibrils, the final products of aggregation, as reported by Eisenberg et al.15,20 In the presence of C60 molecules, Fig. 5(a) shows that the number of water molecules around the backbone atoms has a net increase for each residue. Although this net increase looks small for each residue per peptide chain, it would be large when considering all of the eight peptide chains. It can be seen from Fig. 5(b) that for side chain atoms, the number of water molecules around some of the residues increases, while that around other residues decreases, indicating that the number of water molecules around side chain atoms changes slightly. The C60-induced water exposure of peptide backbone atoms would strengthen peptide–water interaction and weaken peptide–peptide interaction, thus interfering with the formation of inter-peptide backbone H-bonds.
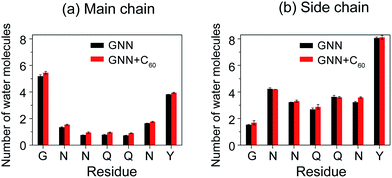 |
| Fig. 5 The number of water molecules within 0.35 nm of the backbone (a) and side chain (b) atoms of each residue per peptide chain in GNN and GNN + C60 systems. | |
Fullerenes block inter-peptide interactions by interacting strongly with the nonpolar aliphatic groups of polar residues N3, Q4 and Q5
It is of great importance to reveal the binding sites and the physical interactions that fullerenes inhibit the formation of β-sheet-rich GNNQQNY octamers. To this aim, we first estimated the inter-peptide main chain–main chain (MC–MC) and side chain–side chain (SC–SC) interactions by calculating the contact probability between each pair of residues. The calculated MC–MC and SC–SC contact probability maps (Fig. 6(a and c)) show that the Q4–Q4 pair has the highest MC–MC and SC–SC contact probabilities, reflecting the important role of Q4 in the formation of octamers. It can be seen from Fig. 6(a) that residue pairs along the left diagonal of the contact map have relatively high MC–MC contact probabilities, indicating that GNNQQNY peptides are aligned predominantly in antiparallel orientation. Interestingly, as shown in Fig. 6(c), the SC–SC contact probability of the Y7–Y7 pair (9.1%) is higher than that of the N2–Y7 pair (6.9%), implying that GNNQQNY peptides have a propensity to be aligned in a parallel orientation. These data suggest that the orientation between GNNQQNY peptides is modulated by a subtle balance among the aromatic-stacking interactions of the tyrosine rings, the MC–MC and SC–SC interactions of hydrophilic residue pairs. The observation of dominant antiparallel orientation for peptide chains in GNNQQNY octamers elucidates that, in small oligomers, the stacking interaction between the side chains of Y7 residues is less important than the interactions between hydrophilic residue pairs for the alignment orientation of peptide chains, consistent with previous MD simulations.24 By comparing Fig. 6(b and d) with (a and c), we find that fullerenes greatly weaken both the MC–MC and SC–SC interactions that are important for GNNQQNY oligomerization.
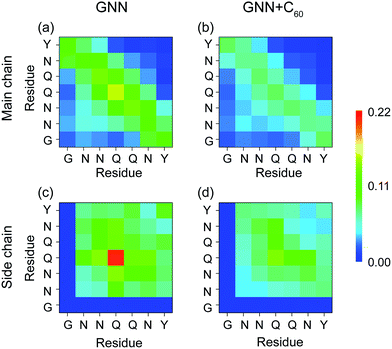 |
| Fig. 6 Inter-peptide MC–MC and SC–SC contact probability maps for GNNQQNY octamers in GNN (a and c) and GNN + C60 (b and d) systems. | |
H-Bonding interactions are considered to be crucial to the fibril formation of the hydrophilic GNNQQNY peptide.21,34,35 To identify the key residues for H-bond and β-sheet formation, we presented in Fig. 7 the MC–MC, SC–SC, and SC–MC H-bond formation maps for GNNQQNY peptides in GNN and GNN + C60 systems. In the GNN system, Fig. 7(a–c) shows that H-bonds are formed between MC–MC, SC–SC and MC–SC atom pairs. The C-terminal residues Y7 form more MC–MC H-bonds with N-terminal residues N2 and N3 than with other residues, which facilitates antiparallel β-strand association. Relatively large number of MC–MC H-bonds are also formed among the middle residues N3, Q4 and Q5, reflecting the important role of those three residues in β-sheet formation. Interestingly, large number of SC–SC H-bonds are also seen among residues N3, Q4 and Q5. As mentioned above, in the absence of C60, the coil probability is 62.7% and the majority of octamers are in disordered coil-rich conformations. The formation of large number of SC–SC H-bonds indicates that inter-peptide SC–SC H-bonding interactions play an important role on the oligomerization of GNNQQNY peptides. However, when C60 nanoparticles were added into the GNN system, both the number of MC–MC and SC–SC H-bonds are greatly reduced, although the MC–SC H-bond map is slightly affected. These results imply that C60 molecules markedly impede the formation of inter-peptide MC–MC and SC–SC H-bonds which are crucial for both β-sheet formation and oligomerization of GNNQNY peptides.
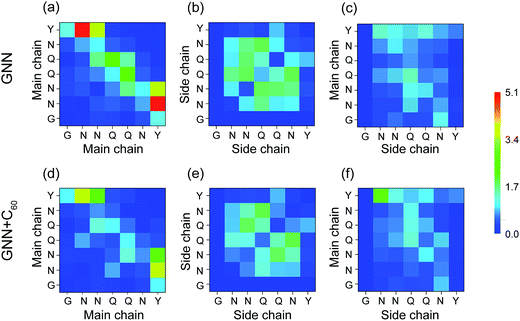 |
| Fig. 7 Inter-peptide H-bond formation maps between each pair of residue in GNN and GNN + C60 systems. MC–MC, SC–SC, and MC–SC H-bond formation maps are shown respectively in (a and d), (b and e) and (c and f). The number of H-bond is averaged over eight peptide chains. | |
To further understand the molecular mechanism that C60 nanoparticles inhibit GNNQQNY oligomerization and β-sheet formation, we calculated the contact probability and binding free energy between a C60 molecule and each amino acid residue. According to these calculations, we identified the dominant binding sites between C60 molecules and GNNQQNY peptides. As shown in Fig. 8(a), C60 molecules have the highest probabilities (>30%) to interact with the hydrophilic residues N3, Q4 and Q5, while have relatively low probability to bind with the aromatic residues Y7. The calculated binding free energy in Fig. 8(b) gives quantitatively the same results as the contact probability does in Fig. 8(a). These data demonstrate that hydrophobic C60 molecules preferentially bind to the middle hydrophilic residues N3, Q4 and Q5 rather than to the aromatic residues Y7. The binding modes of C60 molecules to each of the three residues are shown in Fig. 8(c). It can be seen from Fig. 8(c) that C60 molecules bind to the nonpolar aliphatic groups of N3, Q4 and Q5, revealing hydrophobic interactions between C60 and hydrophilic residues, rather than the π–π stacking interactions between C60 and Y7, are crucial to the binding of C60 to GNNQQNY peptides. Similarly, strong interactions between carbon nanotubes and hydrophilic residues were reported in previous experimental87 and computational88 studies on the adsorption of proteins to the surface of carbon nanotubes. As we demonstrated above, inter-peptide SC–SC H-bonding interactions among the three residues N3, Q4 and Q5 are crucial to the oligomerization of GNNQQNY peptides. The strong interactions between C60 molecules and these three residues would hinder the inter-peptide interactions responsible for GNNQQNY oligomerization, thus inhibits the fibrillation process. In our previous REMD studies, we found that carbon nanotubes68 and fullerenes50 can inhibit effectively the formations of β-sheet-rich oligomers of the hydrophobic KLVFFAE peptide via hydrophobic and aromatic-stacking interactions and we proposed that carbon nanoparticles may impede the fibrillation of KLVFFAE peptide.50,68 Interestingly, this prediction was confirmed by atomic force microscopy experiments.50,74 Taken together, we propose that hydrophobic fullerenes could inhibit the aggregation of both hydrophobic and hydrophilic peptides.
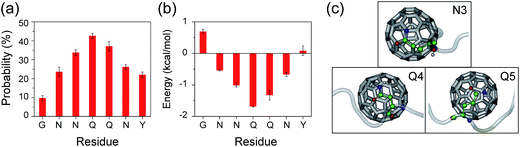 |
| Fig. 8 C60–peptide interaction analyses in GNN + C60 system. Contact probabilities (a) and the binding energy (b) between each amino acid residue and a C60 molecule. (c) Representative snapshots showing the binding of a C60 molecule with the nonpolar aliphatic groups of residues N3, Q4 and Q5. The GNNQQNY peptide is in semitransparent light grey cartoon with residues N3, Q4 and Q5 shown in colored ball-and-stick representations to distinguish different type of atoms: C (green), N (blue), O (red), H (gray). The C60 molecule is in silver sticks. | |
Conclusions
In summary, we investigated the octamerization of GNNQQNY peptide, an amyloid-like fibril-forming fragment of the yeast prion Sup35, and the inhibitory effect of fullerenes on GNNQQNY oligomerization by preforming two 250 ns atomistic REMD simulations starting from a disordered states. Our simulations show that, in the absence of C60 molecules, GNNQQNY octamers adopt mostly compact disordered conformations including a majority of coil-rich conformations and a minority of β-sheet-rich conformations. Interestingly, ordered bilayer β-sheet structures consisting of two four-stranded β-sheets are also populated, albeit with a low probability (6.9%). In the presence of C60 molecules, the coil percentage increases from 62.7% (without C60) to 75.4%, whereas the β-sheet probability decrease from 29.1% (without C60) to 13.5%. More importantly, C60 molecules greatly enhance the formation of disordered coil-rich conformations, while inhibit β-sheet-rich conformations and completely suppress ordered fibril-like bilayer β-sheet structures.
Inter-peptide contact probability maps and H-bond formation maps between all pairs of residues suggest that Q4–Q4 interactions and the SC–SC H-bonding interactions among residues N3, Q4 and Q5 play an important role in the oligomerization of GNNQQNY peptide and the MC–MC H-bonding interactions among residues N3, Q4 and Q5 are crucial for β-sheet formation. C60 molecules markedly impede the formation of inter-peptide MC–MC and SC–SC H-bonds by binding to the nonpolar aliphatic groups of residues N3, Q4 and Q5 and by exposing peptide backbone atoms to water molecules. The binding energy and binding probability calculation reveals that the hydrophobic interactions between C60 and hydrophilic residues N3, Q4 and Q5, rather than the π–π stacking interactions between C60 and residues Y7, are crucial to the fullerene–peptide interaction. Our computational study suggests that hydrophobic C60 nanoparticles are likely to inhibit the fibrillation of the hydrophilic GNNQQNY peptide.
Acknowledgements
This work was supported by the NSF of China (Grant No. 11274075 and 11674065) and the MOST of China (Grant No. 2016YFA0501702). Simulations were performed at National High Performance Computing Center of Fudan University.
References
- W. Peelaerts, L. Bousset, A. Van der Perren, A. Moskalyuk, R. Pulizzi, M. Giugliano, C. Van den Haute, R. Melki and V. Baekelandt, Nature, 2015, 522, 340–344 CrossRef CAS PubMed.
- F. Chiti and C. M. Dobson, Annu. Rev. Biochem., 2006, 75, 333–366 CrossRef CAS PubMed.
- T. P. Knowles, M. Vendruscolo and C. M. Dobson, Nat. Rev. Mol. Cell Biol., 2014, 15, 384–396 CrossRef CAS PubMed.
- L. C. Serpell, M. D. Benson, G. A. Tennent, M. B. Pepys and P. E. Fraser, J. Mol. Biol., 2000, 300, 1033–1039 CrossRef CAS PubMed.
- D. Thirumalai, D. K. Klimov and R. I. Dima, Curr. Opin. Struct. Biol., 2003, 13, 146–159 CrossRef CAS PubMed.
- D. M. Walsh, I. Klyubin, J. V. Fadeeva, W. K. Cullen, R. Anwyl, M. S. Wolfe, M. J. Rowan and D. J. Selkoe, Nature, 2002, 416, 535–539 CrossRef CAS PubMed.
- R. Kayed, E. Head, J. L. Thompson, T. M. McIntire, S. C. Milton, C. W. Cotman and C. G. Glabe, Science, 2003, 300, 486–489 CrossRef CAS PubMed.
- I. Benilova, E. Karran and B. De Strooper, Nat. Neurosci., 2012, 15, 349–357 CrossRef CAS PubMed.
- J. J. Balbach, Y. Ishii, O. N. Antzutkin, R. D. Leapman, N. W. Rizzo, F. Dyda, J. Reed and R. Tycko, Biochemistry, 2000, 39, 13748–13759 CrossRef CAS PubMed.
- M. von Bergen, P. Friedhoff, J. Biernat, J. Heberle, E. M. Mandelkow and E. Mandelkow, Proc. Natl. Acad. Sci. U. S. A., 2000, 97, 5129–5134 CrossRef CAS.
- M. I. Ivanova, S. A. Sievers, E. L. Guenther, L. M. Johnson, D. D. Winkler, A. Galaleldeen, M. R. Sawaya, P. J. Hart and D. S. Eisenberg, Proc. Natl. Acad. Sci. U. S. A., 2014, 111, 197–201 CrossRef CAS PubMed.
- M. Balbirnie, R. Grothe and D. S. Eisenberg, Proc. Natl. Acad. Sci. U. S. A., 2001, 98, 2375–2380 CrossRef CAS PubMed.
- S. L. Hands and A. Wyttenbach, Acta Neuropathol., 2010, 120, 419–437 CrossRef CAS PubMed.
- M. F. Perutz, T. Johnson, M. Suzuki and J. T. Finch, Proc. Natl. Acad. Sci. U. S. A., 1994, 91, 5355–5358 CrossRef CAS.
- R. Nelson, M. R. Sawaya, M. Balbirnie, A. O. Madsen, C. Riekel, R. Grothe and D. Eisenberg, Nature, 2005, 435, 773–778 CrossRef CAS PubMed.
- P. C. van der Wel, J. R. Lewandowski and R. G. Griffin, J. Am. Chem. Soc., 2007, 129, 5117–5130 CrossRef CAS PubMed.
- K. E. Marshall, M. R. Hicks, T. L. Williams, S. V. Hoffmann, A. Rodger, T. R. Dafforn and L. C. Serpell, Biophys. J., 2010, 98, 330–338 CrossRef CAS PubMed.
- P. C. van der Wel, J. R. Lewandowski and R. G. Griffin, Biochemistry, 2010, 49, 9457–9469 CrossRef CAS PubMed.
- J. R. Lewandowski, P. C. van der Wel, M. Rigney, N. Grigorieff and R. G. Griffin, J. Am. Chem. Soc., 2011, 133, 14686–14698 CrossRef CAS PubMed.
- M. R. Sawaya, J. Rodriguez, D. Cascio, M. J. Collazo, D. Shi, F. E. Reyes, J. Hattne, T. Gonen and D. S. Eisenberg, Proc. Natl. Acad. Sci. U. S. A., 2016, 113, 11232–11236 CrossRef CAS PubMed.
- J. Zheng, B. Ma, C. J. Tsai and R. Nussinov, Biophys. J., 2006, 91, 824–833 CrossRef CAS PubMed.
- L. Esposito, C. Pedone and L. Vitagliano, Proc. Natl. Acad. Sci. U. S. A., 2006, 103, 11533–11538 CrossRef CAS PubMed.
- L. Vitagliano, L. Esposito, C. Pedone and A. De Simone, Biochem. Biophys. Res. Commun., 2008, 377, 1036–1041 CrossRef CAS PubMed.
- Z. Zhang, H. Chen, H. Bai and L. Lai, Biophys. J., 2007, 93, 1484–1492 CrossRef CAS PubMed.
- J. Wang, C. Tan, H. F. Chen and R. Luo, Biophys. J., 2008, 95, 5037–5047 CrossRef CAS PubMed.
- A. S. Reddy, M. Chopra and J. J. de Pablo, Biophys. J., 2010, 98, 1038–1045 CrossRef CAS PubMed.
- J. Nasica-Labouze, M. Meli, P. Derreumaux, G. Colombo and N. Mousseau, PLoS Comput. Biol., 2011, 7, e1002051 CAS.
- J. Nasica-Labouze and N. Mousseau, PLoS Comput. Biol., 2012, 8, e1002782 CAS.
- B. Barz, D. J. Wales and B. Strodel, J. Phys. Chem. B, 2014, 118, 1003–1011 CrossRef CAS PubMed.
- Y. Sugita, A. Kitao and Y. Okamoto, J. Chem. Phys., 2000, 113, 6042–6051 CrossRef CAS.
- A. Srivastava and P. V. Balaji, J. Struct. Biol., 2015, 192, 376–391 CrossRef CAS PubMed.
- G. Reddy, J. E. Straub and D. Thirumalai, Proc. Natl. Acad. Sci. U. S. A., 2009, 106, 11948–11953 CrossRef CAS PubMed.
- D. Thirumalai, G. Reddy and J. E. Straub, Acc. Chem. Res., 2012, 45, 83–92 CrossRef CAS PubMed.
- J. Gsponer, U. Haberthur and A. Caflisch, Proc. Natl. Acad. Sci. U. S. A., 2003, 100, 5154–5159 CrossRef CAS PubMed.
- B. W. Strodel, C. S. Whittleston and D. J. Wales, J. Am. Chem. Soc., 2007, 16005–16014 CrossRef CAS PubMed.
- K. L. Osborne, M. Bachmann and B. Strodel, Proteins, 2013, 81, 1141–1155 CrossRef CAS PubMed.
- C. Cabaleiro-Lago, F. Quinlan-Pluck, I. Lynch, S. Lindman, A. M. Minogue, E. Thulin, D. M. Walsh, K. A. Dawson and S. Linse, J. Am. Chem. Soc., 2008, 130, 15437–15443 CrossRef CAS PubMed.
- Y. H. Liao, Y. J. Chang, Y. Yoshiike, Y. C. Chang and Y. R. Chen, Small, 2012, 8, 3631–3639 CrossRef CAS PubMed.
- M. Mahmoudi, O. Akhavan, M. Ghavami, F. Rezaee and S. M. Ghiasi, Nanoscale, 2012, 4, 7322–7325 RSC.
- T. Takahashi and H. Mihara, Acc. Chem. Res., 2008, 41, 1309–1318 CrossRef CAS PubMed.
- B. Cheng, H. Gong, H. Xiao, R. B. Petersen, L. Zheng and K. Huang, Biochim. Biophys. Acta, 2013, 1830, 4860–4871 CrossRef CAS PubMed.
- D. E. Ehrnhoefer, J. Bieschke, A. Boeddrich, M. Herbst, L. Masino, R. Lurz, S. Engemann, A. Pastore and E. E. Wanker, Nat. Struct. Mol. Biol., 2008, 15, 558–566 CAS.
- Q. Wang, X. Yu, K. Patal, R. Hu, S. Chuang, G. Zhang and J. Zheng, ACS Chem. Neurosci., 2013, 4, 1004–1015 CrossRef CAS PubMed.
- F. De Leo, A. Magistrato and D. Bonifazi, Chem. Soc. Rev., 2015, 44, 6916–6953 RSC.
- D. Lin, R. Qi, S. Li, R. He, P. Li, G. Wei and X. Yang, ACS Chem. Neurosci., 2016, 7, 1232–1240 CrossRef CAS PubMed.
- M. Zhang, X. Mao, Y. Yu, C. X. Wang, Y. L. Yang and C. Wang, Adv. Mater., 2013, 25, 3780–3801 CrossRef CAS PubMed.
- C. Li and R. Mezzenga, Nanoscale, 2013, 5, 6207–6218 RSC.
- L. Wang, S. Zhu, T. Lu, G. Zhang, J. Xu, Y. Song, Y. Li, L. Wang, B. Yang and F. Li, J. Mater. Chem. B, 2016, 4, 4913–4921 RSC.
- S. H. Friedman, D. L. DeCamp, R. P. Sijbesma, G. Srdanov, F. Wudl and G. L. Kenyon, J. Am. Chem. Soc., 1993, 115, 6506–6509 CrossRef CAS.
- L. Xie, Y. Luo, D. Lin, W. Xi, X. Yang and G. Wei, Nanoscale, 2014, 6, 9752–9762 RSC.
- L. L. Dugan, D. M. Turetsky, C. Du, D. Lobner, M. Wheeler, C. R. Almli, C. K. Shen, T. Y. Luh, D. W. Choi and T. S. Lin, Proc. Natl. Acad. Sci. U. S. A., 1997, 94, 9434–9439 CrossRef CAS.
- J. E. Kim and M. Lee, Biochem. Biophys. Res. Commun., 2003, 303, 576–579 CrossRef CAS PubMed.
- Y. Ishida, T. Fujii, K. Oka, D. Takahashi and K. Toshima, Chem.–Asian J., 2011, 6, 2312–2315 CrossRef CAS PubMed.
- A. G. Bobylev, A. B. Kornev, L. G. Bobyleva, M. D. Shpagina, I. S. Fadeeva, R. S. Fadeev, D. G. Deryabin, J. Balzarini, P. A. Troshin and Z. A. Podlubnaya, Org. Biomol. Chem., 2011, 9, 5714–5719 CAS.
- E. G. Makarova, R. Y. Gordon and I. Y. Podolski, J. Nanosci. Nanotechnol., 2012, 12, 119–126 CrossRef CAS PubMed.
- V. Vorobyov, V. Kaptsov, R. Gordon, E. Makarova, I. Podolski and F. Sengpiel, JAD, J. Alzheimer's Dis., 2015, 45, 217–233 CAS.
- Z. Bednarikova, P. D. Huy, M. M. Mocanu, D. Fedunova, M. S. Li and Z. Gazova, Phys. Chem. Chem. Phys., 2016, 18, 18855–18867 RSC.
- S. A. Andujar, F. Lugli, S. Hofinger, R. D. Enriz and F. Zerbetto, Phys. Chem. Chem. Phys., 2012, 14, 8599–8607 RSC.
- X. Zhou, W. Xi, Y. Luo, S. Cao and G. Wei, J. Phys. Chem. B, 2014, 118, 6733–6741 CrossRef CAS PubMed.
- P. D. Huy and M. S. Li, Phys. Chem. Chem. Phys., 2014, 16, 20030–20040 RSC.
- Y. Sun, Z. Qian and G. Wei, Phys. Chem. Chem. Phys., 2016, 18, 12582–12591 RSC.
- M. Haratake, T. Takiguchi, N. Masuda, S. Yoshida, T. Fuchigami and M. Nakayama, Colloids Surf., B, 2016, 149, 72–79 CrossRef PubMed.
- P. C. van der Wel, J. R. Lewandowski and R. G. Griffin, J. Am. Chem. Soc., 2007, 129, 5117–5130 CrossRef CAS PubMed.
- Y. Sugita and Y. Okamoto, Chem. Phys. Lett., 1999, 314, 141–151 CrossRef CAS.
- D. Van Der Spoel, E. Lindahl, B. Hess, G. Groenhof, A. E. Mark and H. J. Berendsen, J. Comput. Chem., 2005, 26, 1701–1718 CrossRef CAS PubMed.
- R. Qi, Y. Luo, B. Ma, R. Nussinov and G. Wei, Biomacromolecules, 2014, 15, 122–131 CrossRef CAS PubMed.
- J. Lei, R. Qi, G. Wei, R. Nussinov and B. Ma, Phys. Chem. Chem. Phys., 2016, 18, 8098–8107 RSC.
- H. Li, Y. Luo, P. Derreumaux and G. Wei, Biophys. J., 2011, 101, 2267–2276 CrossRef CAS PubMed.
- W. F. van Gunsteren, S. R. Billeter, A. A. Eising, P. H. Hünenberger, P. Krüger, A. E. Mark, W. R. P. Scott and I. G. Tironi, Biomolecular Simulation: The GROMOS96 Manual and User Guide, Vdf Hochschulverlag AG an der ETH Zürich, Zürich, Switzerland, 1996, pp. 1–1042 Search PubMed.
- Z. A. Levine, L. Larini, N. E. LaPointe, S. C. Feinstein and J. E. Shea, Proc. Natl. Acad. Sci. U. S. A., 2015, 112, 2758–2763 CrossRef CAS PubMed.
- A. Morriss-Andrews and J. E. Shea, Annu. Rev. Phys. Chem., 2015, 66, 643–666 CrossRef CAS PubMed.
- J. Nasica-Labouze, P. H. Nguyen, F. Sterpone, O. Berthoumieu, N. V. Buchete, S. Cote, A. De Simone, A. J. Doig, P. Faller, A. Garcia, A. Laio, M. S. Li, S. Melchionna, N. Mousseau, Y. Mu, A. Paravastu, S. Pasquali, D. J. Rosenman, B. Strodel, B. Tarus, J. H. Viles, T. Zhang, C. Wang and P. Derreumaux, Chem. Rev., 2015, 115, 3518–3563 CrossRef CAS PubMed.
- Y. Zou, Y. Sun, Y. Zhu, B. Ma, R. Nussinov and Q. Zhang, ACS Chem. Neurosci., 2016, 7, 286–296 CrossRef CAS PubMed.
- L. Xie, D. Lin, Y. Luo, H. Li, X. Yang and G. Wei, Biophys. J., 2014, 107, 1930–1938 CrossRef CAS PubMed.
- T. Darden, D. York and L. Pedersen, J. Chem. Phys., 1993, 98, 10089 CrossRef CAS.
- B. Hess, H. Bekker, H. J. Berendsen and J. G. E. M. Fraaije, J. Comput. Chem., 1997, 18, 1463–1472 CrossRef CAS.
- S. Miyamoto and P. A. Kollman, J. Comput. Chem., 1992, 13, 952–962 CrossRef CAS.
- G. Bussi, D. Donadio and M. Parrinello, J. Chem. Phys., 2007, 126, 014101 CrossRef PubMed.
- M. Parrinello and A. Rahman, Phys. Rev. Lett., 1980, 45, 1196–1199 CrossRef CAS.
- Y. Lu, P. Derreumaux, Z. Guo, N. Mousseau and G. Wei, Proteins: Struct., Funct., Bioinf., 2009, 75, 954–963 CrossRef CAS PubMed.
- X. Daura, K. Gademann, B. Jaun, D. Seebach, W. F. van Gunsteren and A. E. Mark, Angew. Chem., Int. Ed., 1999, 38, 236–240 CrossRef CAS.
- R. Kumari, R. Kumar, C. Open Source Drug Discovery and A. Lynn, J. Chem. Inf. Model., 2014, 54, 1951–1962 CrossRef CAS PubMed.
- N. Homeyer and H. Gohlke, Mol. Inf., 2012, 31, 114–122 CrossRef CAS PubMed.
- W. M. Berhanu and U. H. Hansmann, Proteins, 2013, 81, 1542–1555 CrossRef CAS PubMed.
- W. Humphrey, A. Dalke and K. Schulten, J. Mol. Graphics, 1996, 14, 33–38 CrossRef CAS PubMed.
- W. Song, G. Wei, N. Mousseau and P. Derreumaux, J. Phys. Chem. B, 2008, 112, 4410–4418 CrossRef CAS PubMed.
- S. Brown, T. S. Jespersen and J. Nygard, Small, 2008, 4, 416–420 CrossRef CAS PubMed.
- G. Zuo, S. G. Kang, P. Xiu, Y. Zhao and R. Zhou, Small, 2013, 9, 1546–1556 CrossRef CAS PubMed.
Footnote |
† Electronic supplementary information (ESI) available. See DOI: 10.1039/c6ra27608c |
|
This journal is © The Royal Society of Chemistry 2017 |
Click here to see how this site uses Cookies. View our privacy policy here.