DOI:
10.1039/C6RA27531A
(Paper)
RSC Adv., 2017,
7, 8581-8588
Exploiting intrinsic fluorescence spectroscopy to discriminate between Acinetobacter calcoaceticus–Acinetobacter baumannii complex species
Received
29th November 2016
, Accepted 14th January 2017
First published on 26th January 2017
Abstract
Several spectroscopic techniques, such as infrared, Raman, fluorescence and mass spectrometry, have been tested in the context of bacterial typing. The degree of success is highly dependent on the taxonomic level. Intrinsic fluorescence spectroscopy, detecting natural bacterial fluorophores, has been claimed to be a reliable alternative to the standard typing methods, with some published works reporting its success. In this work we evaluate the ability of this technique to discriminate between four closely related species belonging to the so-called Acinetobacter calcoaceticus–Acinetobacter baumannii complex. Single-point and two-dimensional fluorescence spectral data were acquired at room temperature and 25 °C. Spectra were analysed by partial least squares discriminant analysis and soft independent modelling of class analogy. The percentages of correct species assignments, ranging from 4.2–97.7%, were highly dependent on the experimental conditions and the method of data analysis. It seems that the results benefit from strict temperature control being those achieved with two-dimensional data slightly better than with single-point data. Nevertheless, it was impossible to achieve a satisfactory percentage of correct assignments for the four species simultaneously, pointing to several limitations of this technique for such bacterial species typing purposes.
1. Introduction
In the last 20 years there has been a boom in published works reporting the ability of spectroscopy to execute bacterial typing at different taxonomic levels.1,2 Most of these studies have been focused on the comparison between spectroscopy and laborious, expensive, gold standard molecular biology techniques, aiming to replace the latter with faster and cheaper techniques. Infrared, Raman, fluorescence and mass spectrometry are among the most commonly reported spectroscopic techniques. Successful implementation seems to be highly dependent on the microorganism, the taxonomic level and/or the spectroscopic technique.3–7 Due to such a great diversity of results, spectroscopic techniques are still not commonly accepted for bacterial typing, and more studies are required for full validation. Also, the discrimination and/or identification of some bacterial species remain a challenge for molecular biology due to their phenotypic and genotypic similarities. An example is the Acinetobacter calcoaceticus–Acinetobacter baumannii (Acb) complex. This complex encompasses several species (A. baumannii, Acinetobacter nosocomialis, Acinetobacter pittii, A. calcoaceticus, Acinetobacter seifertii and Acinetobacter genomic species “Between 1 and 3”) and most of the studies aiming at its identification reported very high rates of misidentification.8,9 These species are frequently involved in severe nosocomial infections with high mortality rates,10 possess multidrug resistance11,12 and are often associated with different clinical outcomes,13 highlighting the need for accurate identification. Bacteria possess natural fluorophores such as aromatic amino acids (AAA), nucleic acids (NA) and co-enzymes (FAD, NADH, NADPH) that can undergo fluorescence following excitation at specific wavelengths. Therefore, bacteria are good candidates for fluorescence-based spectroscopic studies. Intrinsic fluorescence spectroscopy (IFS) was first applied in the microbiology context in 1970 for the monitoring of microorganism cultures.14 Since then, only a few studies have reported the potential of this technique as a diagnostic tool for bacterial typing. Leblanc and Dufour reported correct genus and species assignments in more than 80% cases.15 Giana et al. correctly discriminated between Escherichia coli, Enterococcus faecalis, and Staphylococcus aureus.16 Ammor and co-workers reported 98.3% and 100% of correct assignments regarding the discrimination between lactic acid bacteria at genus and species level, respectively.17 Sohn et al. correctly discriminated among Escherichia coli, Salmonella and Campylobacter,18 and Tourkya et al. reported Pseudomonas correct species assignments above 90%.19 Walsh and co-workers also correctly discriminated between bacteria in blood cultures at Gram, family and species level (correct assignments above 96%).20 Also, we have been unable to find comparative studies between IFS and other spectroscopic techniques.
In this work we explore the potential of IFS coupled to appropriate chemometric methods for bacterial typing of very closely related species belonging to the Acb complex (A. baumannii, A. nosocomialis, A. pittii and A. calcoaceticus). The typing performance of IFS was compared with those of competing spectroscopic techniques, namely Fourier-transform infrared spectroscopy (FTIRS) and matrix-assisted laser desorption/ionization time-of-flight mass spectrometry (MALDI-TOF MS).
2. Materials and methods
2.1. Bacterial isolates
This work included a collection of 71 isolates (essentially clinical isolates) belonging to four species of the Acb complex: A. baumannii (n = 27), A. pittii (n = 18), A. nosocomialis (n = 16), and A. calcoaceticus (n = 10). The isolates were selected from a larger collection8,21–23 to reflect the currently known diversity within the Acb complex. All isolates were previously identified at the species level by rpoB sequencing while most of them were also characterized by other taxonomic methods such as multilocus sequence typing or amplified fragment length polymorphism (AFLP). Details of the collection are given in Table 1.
Table 1 Epidemiological characteristics of the Acinetobacter calcoaceticus–Acinetobacter baumannii complex isolates included in this worka
Acinetobacter species |
Strain no. |
Human specimen |
Country of isolation |
Year of isolation |
STb |
Note |
CZ-Czech Republic; NL-Netherlands; UK-United Kingdom; SE-Sweden; DK-Denmark; CN-China; HU-Hungary; US-United States of America, CH-Switzerland, DE-Germany. MLST scheme of Diancourt L., Passet V., Nemec A., Dijkshoorn L., Brisse S., PLoS ONE, 2010, 5(4), e10034. The STs designated “New” are not included in the current version of the Institute Pasteur database; N.D.-not determined. |
A. baumannii |
NIPH 60 |
Sputum |
CZ |
1992 |
34 |
|
NIPH 67 |
Tracheal secretion |
CZ |
1992 |
35 |
|
NIPH 70 |
Tracheal secretion |
CZ |
1992 |
36 |
|
NIPH 80 |
IV cannula |
CZ |
1993 |
37 |
|
RUH 134 (=NIPH 528) |
Urine |
NL |
1982 |
2 |
EU clone II reference strain |
NIPH 146 |
Wound |
CZ |
1993 |
New 1 |
|
NIPH 190 |
Tracheal secretion |
CZ |
1993 |
9 |
|
NIPH 201 |
Nasal swab |
CZ |
1992 |
38 |
|
NIPH 290 |
Urine |
CZ |
1994 |
1 |
EU clone I |
NIPH 329 |
Tracheal secretion |
CZ |
1994 |
11 |
|
NIPH 335 |
Sputum |
CZ |
1994 |
10 |
|
NIPH 410 |
Cannula |
CZ |
1996 |
39 |
|
NIPH 501T |
Urine |
Not known |
<1949 |
52 |
Type strain |
RUH 875 (=NIPH 527) |
Urine |
NL |
1984 |
1 |
EU clone I reference strain |
NIPH 601 |
Urine |
CZ |
1993 |
40 |
|
NIPH 615 |
Tracheal secretion |
CZ |
1994 |
12 |
|
NIPH 1362 |
Tracheal aspirate |
CZ |
2000 |
47 |
EU clone II |
NIPH 1734 |
Sputum |
CZ |
2001 |
15 |
|
NIPH 2061 |
IV cannula |
CZ |
2003 |
2 |
EU clone II |
NIPH 2390 |
Sputum |
NL |
1987 |
27 |
|
NIPH 2393 |
Pharynx |
NL |
1987 |
55 |
|
NIPH 2778 |
Sputum |
NL |
2002 |
48 |
|
NIPH 2783 |
Wound |
NL |
2002 |
18 |
|
ANC 4097 |
Sputum |
CZ (import from Egypt) |
2011 |
1 |
EU clone I |
ANC 4201 |
Sputum |
CZ |
2011 |
2 |
EU clone II |
ANC 4373 |
Wound swab |
CZ (import from Cyprus) |
2012 |
N.D. |
EU clone I |
NIPH 1669 (=LUH 5875) |
Blood |
NL |
1997 |
3 |
EU clone III reference strain |
A. nosocomialis |
NIPH 12 |
Burn |
CZ |
1991 |
N.D. |
|
NIPH 106 |
Tracheal secretion |
CZ |
1993 |
N.D. |
|
NIPH 258 |
Throat |
CZ |
1993 |
N.D. |
|
NIPH 386 |
Sputum |
CZ |
1996 |
New 3 |
|
NIPH 523 (=LMG 993) |
Not known |
Not known |
<1950 |
74 |
|
NIPH 2119T |
Sputum |
NL |
1987 |
76 |
Type strain |
NIPH 2134 |
Urine |
NL |
1984 |
68 |
|
NIPH 2140 |
Blood |
NL |
1975–1980 |
N.D. |
|
NIPH 2265 |
Urine |
SE |
1980–1981 |
N.D. |
|
RUH 2284 (=LMG 10622) |
Bronchus |
NL |
1987 |
N.D. |
|
RUH 2624 (=LMG 10617) |
Skin |
NL |
1987 |
71 |
|
RUH 2627 (=LMG 10623) |
Rectum |
NL |
1987 |
N.D. |
|
NIPH 2813 |
Sputum |
NL |
2000 |
71 |
|
ANC 3803 |
Skin |
CN |
2001 |
N.D. |
|
ANC 3805 |
Sputum |
NL |
2003 |
N.D. |
|
ANC 3806 |
Bronchial secretion |
UK |
2000 |
N.D. |
|
A. pittii |
NIPH 14 |
Burn |
CZ |
1991 |
N.D. |
|
RUH 37 (=LMG 10560) |
Blood |
NL |
1981 |
N.D. |
|
NIPH 76 |
Urine |
CZ |
1992 |
N.D. |
|
NIPH 95 |
Urine |
CZ |
1993 |
N.D. |
|
NIPH 119 |
Burn |
CZ |
1993 |
N.D. |
|
NIPH 336 |
Urine |
CR |
1993 |
N.D. |
|
RUH 468 (=LMG 10562) |
Urine |
NL |
1984 |
N.D. |
|
NIPH 519T |
Cerebrospinal fluid |
Not known |
<1967 |
63 |
Type strain |
NIPH 789 |
Trachea |
HU |
1994 |
N.D. |
|
RUH 1163 (=LMG 10555) |
Toe web |
NL |
1985 |
75 |
|
NIPH 2133 |
Bronchus |
NL |
1984 |
72 |
|
NIPH 2135 |
Drain |
NL |
1984 |
N.D. |
|
NIPH 2141 |
Not known |
CH |
1984 |
N.D. |
|
NIPH 2255 |
Wound |
SE |
1980 |
N.D. |
|
NIPH 2258 |
Wound |
SE |
1980 |
73 |
|
NIPH 2805 |
Urine |
NL |
1986 |
70 |
|
ANC 3678 |
Water with mud |
CZ |
2008 |
N.D. |
|
ANC 3870 (=SH024) |
Axilla |
DE |
1993 |
93 |
|
A. calcoaceticus |
NIPH 13 (=CCM 4665) |
Burn |
CZ |
1991 |
New 4 |
|
RUH 582 (=LMG 10516) |
Soil |
NL |
1984 |
N.D. |
|
RUH 944 (=LMG 10515) |
IV cannula |
NL |
1984 |
N.D. |
|
RUH 2202 (=LMG 10517) |
Wound |
SE |
1980–1981 |
92 |
|
RUH 2203 (=LMG 10518) |
Wound |
SE |
1980–1981 |
N.D. |
|
NIPH 2706 |
Sputum |
CZ |
2006 |
N.D. |
|
NIPH 2814 |
Urinary catheter |
NL |
2004 |
61 |
|
ANC 3801 |
Eye |
N |
2006 |
N.D. |
|
ANC 3804 |
Amputation stump |
N |
1994 |
N.D. |
|
ANC 4104 |
Well water |
CZ |
2011 |
N.D. |
|
2.2. Sample preparation
Isolates were grown from frozen stocks in Tryptic Soy Agar (TSA) at 37 °C for 16 hours. Prior to the measurement of the fluorescence spectra, cells of the third quadrant were suspended in saline solution ([NaCl] = 9 g L−1) ensuring an optical density of 0.300 ± 0.005 measured at 600 nm (Microplate absorbance reader, Sunrise Basic Tecan). For the experiments at controlled temperature, the suspensions were stabilized in a water bath at 25 °C prior to spectral acquisition.
2.3. Acquisition of intrinsic fluorescence spectra
Intrinsic fluorescence spectra were acquired on a Horiba Aqualog 800 equipped with an integrated agitation system. Single-point fluorescence (SPF) spectra were recorded from 305–400 nm with an excitation wavelength of 270 nm (for tryptophan) and from 280–450 nm with an excitation wavelength of 250 nm (for NADH + AAA) (slit size 5.0 nm). Two-dimensional fluorescence (2DF) spectra were recorded from 150–927 nm (slit size 5.0 nm) with excitation from 240–800 nm (in increments of 3 nm). The integration time for both modes was set to 0.1 s. SPF and 2DF spectra were acquired at room temperature and at the strictly controlled temperature of 25 °C. Spectra were acquired in triplicate (instrumental replicates) on three distinct days from independent culture cells (biological replicates), yielding a total of 71 (isolates) × 2 (temperatures) × 3 (instrumental replicates) × 3 (biological replicates) × 2 (fluorophores; NADH + AAA and Trp) = 2556 spectra for SPF data. Similarly 1278 spectra were acquired for 2DF data (spectra were no longer acquired at two discrete wavelength ranges corresponding to the two different fluorophores). Of note, 2DF data were stored after the removal of the 1st order of the Rayleigh scatter effect and also after the removal of both the 1st and 2nd orders of the Rayleigh scatter effect. Data were organized in eight distinct data sets (Table 2). Due to the large amount of generated data, spectra from the instrumental and biological replicates were averaged prior to chemometric analysis.
Table 2 Spectral data setsa
Data set |
Fluorescence method |
Temperature |
Fluorophore |
Rayleigh scatterb |
SPF-single-point fluorescence; 2DF-two-dimensional fluorescence; R.T.- room temperature; NADH + AAA-reduced form of nicotinamide adenine dinucleotide + aromatic amino acids; Trp tryptophan; n.a.-not applicable. Order of the Rayleigh scatter effect removed. |
#1 |
SPF |
R.T. |
NADH + AAA |
n.a. |
#2 |
Trp |
#3 |
25 °C |
NADH + AAA |
#4 |
Trp |
#5 |
2DF |
R.T. |
n.a. |
1st |
#6 |
1st and 2nd |
#7 |
25 °C |
1st |
#8 |
1st and 2nd |
2.4. Multivariate data analysis
All data sets were modelled with partial least squares discriminant analysis (PLSDA)24,25 and soft independent modelling by class analogy (SIMCA).26,27 The PLSDA model is based on the PLS-2 regression algorithm and was optimized by the leave-one-sample-out cross-validation procedure in order to prevent overfitting.27,28 Isolates were randomly divided into two sets: 70% of the data set was used for the cross-validation method (to define the optimal number of latent variables to use) and 30% of the data set was used for external testing. The preserved number of latent variables was defined according to the minimum misclassification rate for the cross-validation data set. Results, in terms of the proportion of correct predictions for each species (the confusion matrix), were obtained by applying the optimized model to the external data set. More information regarding the PLSDA model optimization and its application in a similar context is described elsewhere.29 The SIMCA model is based on the identification of local models built from defined groups and aims to predict a class membership for each observation.26,27 In SIMCA, multiple principal component analysis models are performed on the data corresponding to each species. A sufficient number of principal components are retained to account for most of the variation within each species. New samples can be projected onto the developed models and the class membership is evaluated according to the distance to each model. A measure of that distance can be based on the Hotelling's T2 distribution and squared prediction error (SPE) statistics.26 These statistics measure the distance of the projected sample within the model and the residuals. A probability of class membership can also be estimated based on these distances so that an assignment can be decided. Therefore, isolates can be assigned to one class or to multiple classes or they can be not assigned. In this work a threshold of probability equal to 0.99 was set for deciding on class membership. More details on the implementation of SIMCA for modelling spectral data from bacteria can be found in Preisner et al.30 Model predictions for each data set were obtained following a similar strategy as for the PLSDA model. Details can be found in Næs et al.26 Pre-processing spectra should be applied in order to remove unwanted data features such as noise, baseline drifts or others. For the SPF data, a few spectral pre-processing methods were applied to model each data set. The pre-processing method yielding the lower cross-validation misidentification rate for each data set was preserved. Models were attempted using no pre-processing at all, standard normal variate, multiplicative scatter correction and Savitzky–Golay filtering with first and second derivative (considering different polynomial orders (2 to 3) and filter widths (uneven size from 9 to 17)). For the 2DF data, removal of the 1st order and the 1st + 2nd orders of the Rayleigh scatter effect were attempted.31 The original three-mode 2DF data arrangement (excitation × emission × signal intensity) was unfolded to a two-mode array preserving the sample mode and yielding a matrix with dimensions samples × excitation × emission. Due to the high collinearity between the columns and the existence of columns with constant values, only those with standard deviations above the threshold 200 were preserved for further analysis. For all situations, before building each PLSDA and SIMCA model, data were mean-centred. All calculations were carried out using Matlab version 8.6 (R2015b) (Mathworks, Natick, MA) and the PLS Toolbox version 8.1 for Matlab (Eigenvector Research, Manson, WA).
3. Results
3.1. Spectral analysis
Fig. 1A presents the average emission spectra, obtained at 25 °C following excitation at 250 nm (corresponding to the NADH + AAA fluorescence), for each of the four Acinetobacter species included in this work. The spectra present fluorescence maxima between 330 and 336 nm.32 The high level of spectral similarity among the four species should be noted and emphasizes the need for appropriate chemometric methods to discriminate between them. The most similar species seem to be A. baumannii and A. pittii whilst the most dissimilar one is A. calcoaceticus, which is in agreement with their phenotypic and genotypic characteristics. The average emission spectra corresponding to the tryptophan residues, obtained at 25 °C, (Fig. 1B) present absorbance maxima between 320 and 330 nm.32 Again, the four species present a very high spectral similarity showing a similar pattern to the one predicted through the NADH + AAA fluorescence spectra. Spectra for both NADH + AAA and tryptophan present the typical excitation maximum peaks (data not shown) respectively at 290 and 280 nm which is in accordance with work by Ammor et al.32 Fig. 2 presents an example of the excitation–emission matrices obtained for all the isolates considered in this work. No significant differences were noted among the isolates of the four species. It is possible to observe two main peaks: (i) between 300 and 400 nm; and (ii) between 425 and 550 nm. The first one mostly includes the fluorescence emissions of tryptophan (350 nm) and tyrosine (300 nm) and the second one includes the fluorescence of NADH (440 and 460 nm), NADPH (nicotamide adenine dinucleotide phosphate; 464 nm) and FAD (flavin adenine dinucleotide; 535 nm).32 Notably, no spectral differences were observed between spectra collected at room temperature and those collected at 25 °C.
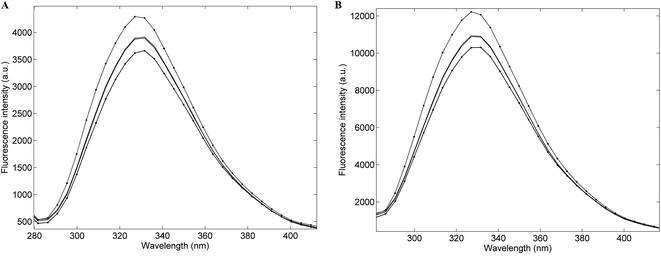 |
| Fig. 1 Average single-point fluorescence intensity emission spectra obtained at room temperature following excitation at 250 nm (A) and 270 nm (B) of: –– Acinetobacter baumannii; – – Acinetobacter pittii; •––• Acinetobacter nosocomialis and • – – • Acinetobacter calcoaceticus. | |
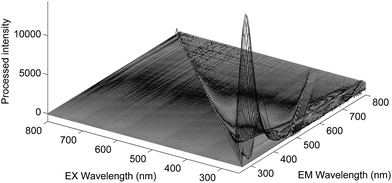 |
| Fig. 2 Excitation–emission matrix of one Acinetobacter baumannii isolate after the removal of the 1st and 2nd orders of the Rayleigh scatter effect at room temperature. | |
3.2. Single-point fluorescence data
Table 3 presents the global percentage of correct species assignments as well as the individual values for each species achieved through the SPF data. The values were obtained from the confusion matrices of the PLSDA and SIMCA models, considering both fluorophores and temperature conditions (sets 1 to 4). The percentages of total correct species assignments clearly indicate that the method benefits from temperature control. Generally, the percentage of correct assignments for each species is higher for the temperature controlled experiments although there are exceptions. A. calcoaceticus was predicted slightly more accurately in the room temperature experiments and A. pittii presents a very similar percentage of correct species assignments at both temperature conditions (considering the same chemometric model and fluorophore). Globally, the best result from SPF data was obtained with the SIMCA model considering tryptophan fluorescence at 25 °C (64.8% of total correct species assignments; A. baumannii: 70.4%; A. pittii: 61.1%; A. nosocomialis: 62.5% and A. calcoaceticus: 60.0%). The exception was A. calcoaceticus which was better predicted by the PLSDA models.
Table 3 Percentages of correct Acinetobacter calcoaceticus–Acinetobacter baumannii complex species predictions from the PLSDA and SIMCA models considering both temperatures and fluorophores for single-point fluorescence dataa
T |
Fluorophore |
Model |
Correct species predictions (%) |
Total |
A. baumannii |
A. pittii |
A. nosocomialis |
A. calcoaceticus |
T-temperature; R.T.-room temperature; NADH + AAA-reduced form of nicotinamide adenine dinucleotide + aromatic amino acids; Trp-tryptophan. |
R.T. |
NADH + AAA |
PLSDA |
54.6 |
59.5 |
54.3 |
30.1 |
82.6 |
SIMCA |
47.9 |
33.3 |
55.6 |
37.5 |
40.0 |
Trp |
PLSDA |
43.2 |
45.6 |
38.5 |
23.6 |
79.1 |
SIMCA |
49.3 |
51.9 |
27.8 |
68.8 |
50.0 |
25 °C |
NADH + AAA |
PLSDA |
55.0 |
57.0 |
64.1 |
30.5 |
71.5 |
SIMCA |
47.9 |
51.9 |
66.7 |
31.3 |
30.0 |
Trp |
PLSDA |
53.8 |
50.0 |
68.6 |
25.8 |
80.8 |
SIMCA |
64.8 |
70.4 |
61.1 |
62.5 |
60.0 |
3.3. Two-dimensional fluorescence data
Similarly to the predictions from the SPF data, the total percentages of correct species predictions from 2DF as well as the corresponding values for each species benefit from strict temperature control (Table 4). Exceptions were noted especially for A. pittii and A. nosocomialis for which there were extremely low percentages of correct species predictions at 25 °C using the PLSDA model after the removal of both orders of the Rayleigh scatter effect. Also, A. calcoaceticus species were better predicted at room temperature, with the highest percentage of correct species predictions (97.4%) achieved in this work. Regarding the Rayleigh scatter effect, the best predictions were achieved with the removal of both orders of the scatter effect except for the already noted exceptions of A. pittii and A. nosocomialis. Similarly to the SPF data, the highest percentage of correct species predictions globally was achieved with the SIMCA model at 25 °C (64.8% of total correct species assignments; A. baumannii: 66.7%; A. pittii: 66.7%; A. nosocomialis: 62.5% and A. calcoaceticus: 60.0%). The exception was A. calcoaceticus which was always better predicted by the PLSDA model.
Table 4 Percentages of correct Acinetobacter calcoaceticus–Acinetobacter baumannii complex species predictions from the PLSDA and SIMCA models considering both temperatures and the removal of either the 1st or the 1st and 2nd orders of the Rayleigh scatter effect for two-dimensional fluorescence dataa
T |
Rayleigh scatterb |
Stdc |
Model |
Correct predictions (%) |
Total |
A. baumannii |
A. pittii |
A. nosocomialis |
A. calcoaceticus |
T-temperature; R.T.-room temperature; Std-standard deviation. Order of the Rayleigh scatter effect removed. The standard deviation selected corresponds to the one that allowed the higher percentage of correct species predictions globally. |
R.T. |
1st |
300 |
PLSDA |
42.2 |
37.2 |
42.7 |
26.0 |
76.0 |
SIMCA |
45.1 |
40.7 |
61.1 |
50.0 |
20.0 |
1st and 2nd |
370 |
PLSDA |
45.2 |
41.8 |
31.8 |
35.4 |
97.7 |
SIMCA |
47.9 |
44.4 |
44.4 |
50.0 |
60.0 |
25 °C |
1st |
210 |
PLSDA |
56.5 |
62.5 |
44.6 |
43.4 |
81.9 |
SIMCA |
59.2 |
55.6 |
61.1 |
50.0 |
80.0 |
1st and 2nd |
200 |
PLSDA |
38.4 |
71.1 |
4.4 |
4.2 |
76.1 |
SIMCA |
64.8 |
66.7 |
66.7 |
62.5 |
60.0 |
4. Discussion
Fluorescence spectroscopy is claimed to be a very promising tool in the medical microbiology field32,33 namely for monitoring fungal and viral infections,33 for bacterial typing purposes at genus18 and species level17,19,20 and for the fluorescence characterization of microorganisms.34,35 However, this spectroscopic technique has been barely explored with respect to bacterial typing of very closely related species and it has not been used within the Acinetobacter genus. In this context, this work was developed to evaluate the ability of IFS to discriminate between Acinetobacter calcoaceticus–Acinetobacter baumannii complex species. For this purpose, SPF and 2DF spectra were acquired under controlled (25 °C) and uncontrolled (room) temperatures and analysed using different pre-processing methods and chemometric models. The percentages of correct species assignments obtained herein vary from 4.2–97.7% according to the spectroscopy methodology (SPF or 2DF) and the chemometric tool applied and seem to benefit from strict temperature control. Despite some very satisfactory individual results, it was not possible to select an optimum set of experimental conditions that could be combined with an appropriate chemometric model to allow the simultaneous and successful discrimination of the four species. As a spectroscopic technique, IFS competes with other spectroscopic techniques such as FTIRS and MALDI-TOF MS. According to previous results obtained by us, and considering nearly the same bacterial collection, it was possible to successfully discriminate six species from the Acb complex with MALDI-TOF MS (100% of correct species identifications for intact bacterial cells).36 However, this is quite an expensive technique due to the cost of equipment acquisition and maintenance. Using FTIRS, it was possible to successfully discriminate between the six species of the Acb complex through a very simple flowchart (although a few isolates were wrongly clustered).37 As a cost effective and environmentally friendly technique, FTIRS clearly possesses a high potential to discriminate between very closely related species. Together these findings suggest that IFS lacks the ability to discriminate between very closely related species such as those belonging to the Acb complex under typical experimental conditions. The controversial results found in the literature, which point to IFS as a promising potential technique for bacterial typing, probably suffer from a lack of robustness of the bacterial collections included. For example, Ammor et al. achieved 100% correct Lactobacillus species discrimination mostly by considering one isolate of each species.17 Also, Tourkya et al.19 reported 91.67% of correct Pseudomonas species identification with a single isolate of each species. The best results were achieved by Walsh et al. considering a very consistent and representative bacterial collection,20 however, using a previously constructed database. No satisfactory species discrimination has been found in the literature considering robust bacterial collections, meaning that some caution has to be used when claiming IFS as a technique with very high potential for bacterial typing at the species level.
5. Conclusions
This work reports the results obtained from an attempt to use intrinsic fluorescence (single-point and two-dimensional) to discriminate between four Acb complex species (A. baumannii, A. pittii, A. nosocomialis and A. calcoaceticus). Fluorescence spectra of a large bacterial collection belonging to the Acb complex were acquired at room temperature and at a controlled temperature. Both fluorescence methodologies (SPF and 2DF) combined with two chemometric methods (PLSAD and SIMCA) and the considered fluorophores (NADH + AAA and tryptophan) yielded average rates of correct assignments of between 38% and 65%. The rates of correct species assignments were superior when experiments were conducted under the controlled temperature (25 °C), as expected. However, the obtained rates of correct assignments were significantly lower when compared with the available literature claims for the high efficiency of this technique for species discrimination. The results obtained herein, considering different fluorescence methods, fluorophores and experimental conditions, reveal that extreme caution should be taken when using this method under these conditions for discriminating between very closely related species.
Conflict of interest
The authors have no competing interests.
Acknowledgements
This work received financial support from the European Union (FEDER funds POCI/01/0145/FEDER/007265) and National Funds (FCT/MEC, Fundação para a Ciência e Tecnologia and Ministério da Educação e Ciência) under the Partnership Agreement PT2020 UID/QUI/50006/2013. Cristina Quintelas was supported by a postdoctoral grant (SFRH/BPD/101338/2014). Clara Sousa was funded through the NORTE-01-0145-FEDER-000024 – “New Technologies for three Health Challenges of Modern Societies: Diabetes, Drug Abuse and Kidney Diseases”. Thanks are due to Alexandr Nemec from the Laboratory of Bacterial Genetics, National Institute of Public Health, Prague – Czech Republic, for providing all the bacterial isolates used in this work.
References
- P. Zarnowiek, L. Lechowicz, G. Czerwonka and W. Kaca, Curr. Med. Chem., 2015, 22(14), 1710–1718 CrossRef.
- S. Angeletti, J. Microbiol. Methods, 2016 DOI:10.1016/j.mimet.2016.09.003.
- K. Maquelin, C. Kirschner, L. P. Choo-Smith, N. Braak, H. P. Endtz, D. Naumann and G. J. Puppels, J. Microbiol. Methods, 2012, 51, 255–271 CrossRef.
- M. W. Wulf, D. Willemse-Erix, C. M. Verduin, G. Puppels, A. van Belkum and K. Maquelin, Clin. Microbiol. Infect., 2012, 18, 147–152 CrossRef CAS PubMed.
- T. R. Sandrin, J. E. Goldstein and S. Schumaker, Mass Spectrom. Rev., 2013, 32, 188–217 CrossRef CAS PubMed.
- C. Sousa, L. Silva, F. Grosso, J. Lopes and L. Peixe, J. Photochem. Photobiol., B, 2014a, 133, 108–114 Search PubMed.
- C. Sousa, J. Botelho, F. Grosso, L. Silva, J. Lopes and L. Peixe, Frontiers in Microbiology, 2015, 6, 481 Search PubMed.
- A. Nemec, L. Krizova, M. Maixnerova, T. J. van der Reijden, P. Deschaght, V. Passet, M. Vaneechoutte, S. Brisse and L. Dijkshoorn, Res. Microbiol., 2011, 162, 393–404 CrossRef CAS PubMed.
- A. Nemec, L. Krizova, M. Maixnerova, O. Sedo, S. Brisse and P. G. Higgins, Int. J. Syst. Evol. Microbiol., 2015, 65, 934–942 CrossRef CAS PubMed.
- K. H. Park, J. H. Shin, S. Y. Lee, S. H. Kim, M. O. Jang, S. J. Kang, S. I. Jung, E. K. Chung, K. S. Ko and H. C. Jang, PLoS One, 2013, 8, e65026 CAS.
- Y. M. Lim, K. S. Shin and J. Kim, J. Clin. Microbiol., 2007, 45, 902–905 CrossRef CAS PubMed.
- F. Grosso, S. Quinteira and L. Peixe, Journal of Hospital Infection, 2010, 75, 82–83 CrossRef CAS PubMed.
- C. C. Lai, H. L. Hsu, C. K. Tan, H. Y. Tsai, A. Cheng, C. Y. Liu, Y. T. Huang, C. H. Liao, W. H. Sheng and P. R. Hsueh, J. Clin. Microbiol., 2012, 50, 2982–2986 CrossRef CAS PubMed.
- D. E. F. Harrison and B. Chance, Appl. Microbiol., 1970, 19, 446–450 CAS.
- L. Leblanc and E. Dufour, FEMS Microbiol. Lett., 2002, 221, 147 CrossRef.
- H. E. Giana, L. Silveira, R. A. Zangaro and M. T. Pacheco, J. Fluoresc., 2003, 13, 489S CrossRef.
- S. Ammor, K. Yaakoubi, I. Chevallier and E. Dufour, J. Microbiol. Methods, 2004, 59, 271–281 CrossRef CAS PubMed.
- M. Sohn, D. S. Himmelsbach, F. E. Barton and P. J. Fedorka-Cray, Appl. Spectrosc., 2009, 63, 1251–1255 CrossRef CAS PubMed.
- B. Tourkya, T. Boubellouta, E. Dufour and F. Leriche, Curr. Microbiol., 2009, 58, 39–46 CrossRef CAS PubMed.
- J. D. Walsh, J. M. Hyman, L. Borzhemskaya, A. Bowen, C. McKellar, M. Ullery, E. Mathias, C. Ronsick, J. Link, M. Wilson, B. Clay, R. Robinson, T. Thorpe, A. van Belkum and W. M. Dunne Jr, mBio, 2013, 4, e00865 CrossRef PubMed.
- A. Nemec, L. Janda, O. Melter and L. Dijkshoorn, J. Med. Microbiol., 1999, 48(3), 287–296 CrossRef CAS PubMed.
- A. Nemec, L. Dolzani, S. Brisse, P. van den Broek and L. Dijkshoorn, J. Med. Microbiol., 2004, 53, 1233–1240 CrossRef CAS PubMed.
- L. Krizova, R. A. Bonnin, P. Nordmann, A. Nemec and L. Poirel, J. Antimicrob. Chemother., 2012, 67(6), 1550–1552 CrossRef CAS PubMed.
- P. Geladi and B. R. Kowalsky, Anal. Chim. Acta, 1986, 185, 11–17 Search PubMed.
- B. K. Alsberg, D. B. Kell and R. Goodacre, Anal. Chem., 1998, 70, 4126–4133 CrossRef CAS PubMed.
- A user-friendly guide to multivariate calibration and classification, ed. T. Næs, T. Isaksson, T. Fearn and T. Davies, NIR Publications, Chichester, UK, 2002 Search PubMed.
- S. Wold, Pattern Recogn., 1976, 8, 127–139 CrossRef.
- R. De Maesschalck, A. Candolfi, D. L. Massart and S. Heuerding, Chemom. Intell. Lab. Syst., 1999, 47, 65–77 CrossRef CAS.
- C. Sousa, F. Grosso, L. Meirinhos-Soares, L. Peixe and J. Lopes, J. Biophotonics, 2014b, 7, 287–294 Search PubMed.
- O. Preisner, J. A. Lopes and J. C. Menezes, Chemom. Intell. Lab. Syst., 2008, 94, 33–42 CrossRef CAS.
- J. R. Lakowicz, in Principles of Fluorescence Spectroscopy, Springer Science & Business Media, 3rd edn, 2007, p. 954 Search PubMed.
- M. S. Ammor, J. Fluoresc., 2007, 17, 455–459 CrossRef CAS PubMed.
- A. Shahzad, G. Köhler, M. Knapp, E. Gaubitzer, M. Puchinger and M. Edetsberger, J. Transl. Med., 2009, 7, 99 CrossRef PubMed.
- L. R. Dartnell, T. A. Roberts, G. Moore, J. M. Ward and J.-P. Muller, PLoS One, 2013, 8, e75270 CAS.
- H. Zhu, W. Lao, Q. Chen, Q. Zhang and H. Chen, Int. J. Clin. Exp. Med., 2015, 8, 3651–3661 CAS.
- C. Sousa, J. Botelho, L. Silva, F. Grosso, A. Nemec, J. Lopes and L. Peixe, Int. J. Med. Microbiol., 2014, 304, 669–677 CrossRef CAS PubMed.
- C. Sousa, L. Silva, F. Grosso, A. Nemec, J. Lopes and L. Peixe, Eur. J. Clin. Microbiol. Infect. Dis., 2014, 33, 1345–1353 CrossRef CAS PubMed.
|
This journal is © The Royal Society of Chemistry 2017 |
Click here to see how this site uses Cookies. View our privacy policy here.