DOI:
10.1039/C6RA27461G
(Paper)
RSC Adv., 2017,
7, 6780-6787
Exploration of metabolite signatures using high-throughput mass spectrometry coupled with multivariate data analysis†
Received
28th November 2016
, Accepted 27th December 2016
First published on 20th January 2017
Abstract
Disease impacts important metabolic pathways and the alteration of metabolites may serve as a potential biomarker for early-stage diagnosis. High-resolution mass spectrometry-based metabolomics have been used to discover new biomarker metabolites. Rheumatoid arthritis (RA) seriously affects the quality of life in patients, but its pathophysiology remains unclear. This study aimed to develop a high-throughput approach by screening potential biomarkers to facilitate the diagnosis using metabolomics. The alteration of the metabolic profile of RA was investigated in human urine samples based on high-resolution UPLC-QTOF/MS and multivariate statistical analysis. Furthermore, ingenuity pathway analysis (IPA) was performed for the bioinformatics analysis of the data. Variable importance for projection values was determined, and the t-test was conducted for selecting a biomarker panel for RA. Receiver operating characteristic analysis was used to evaluate diagnostic accuracy of metabolites. We found that the score plot of orthogonal partial least squares discriminant analysis showed significant discrimination between RA and healthy groups. Five metabolites were identified as potential biomarkers for RA. The values of AUC, ranging from 0.819 to 0.993, indicated the potential capacity of these metabolites to distinguish RA patients and demonstrated that the differentially expressed metabolites might be a useful tool for the effective diagnosis of RA. The most significantly altered networks included FXR/RXR activation and bile acid biosynthesis. This study demonstrates that a high-resolution mass spectrometry-based metabolomics approach could provide crucial insight into the pathogenesis mechanism of RA.
1. Introduction
Rheumatoid arthritis (RA) is a chronic autoimmune disease characterized by damage of the affected joints that causes pain, swelling, stiffness, and loss of function in joints.1 Early diagnosis of RA is important for early intervention. Several biochemical biomarkers, including proteoglycan aggrecan, type I collagen, type II collagen, hyaluronic acid, osteocalcin, and others, have been identified to be associated with RA.2–8 Presently, rheumatoid factor (RF), a well-known biomarker for RA, is not useful for the specific diagnosis of RA because it is also detected in various other rheumatic disorders.9,10 Therefore, more reliable biomarkers with diagnostic capabilities are still required for RA. Identification of the sensitive biomarkers of RA patients would reflect both diagnosis and disease severity and provide an important tool for maximizing patient care. Numerous omics studies have provided some biomarker candidates and an insight into the pathology of RA.11 Urine is an easy, inexpensive, safe, and noninvasive fluid and it has the potential to mirror health conditions.12
Biomarkers are important tools for disease diagnosis and stratification, treatment selection, monitoring disease progression, and establishing patients' responses to therapies.13–15 Metabolomics is a non-targeted analysis of global changes of the complete set of metabolites in organisms and may be a powerful tool for discovering new biomarkers for diseases. Recently, metabolomics technologies have been increasingly exploited for the discovery of disease biomarkers.16–18 The advantage of evaluating patients using a high-resolution mass spectrometry-based strategy is the possibility of providing insight that helps the development of a better understanding of the disease state.19–22 It is possible to describe patterns of metabolite biomarkers that are highly discriminatory for the perturbations in diseases. The diagnostic information can be obtained by quantifying biomarker metabolites and comparing them to normal samples.23 The characteristic metabolite profile associated with a disease and the metabolic phenotype can be obtained using a metabolomics approach. Herein, results of a metabolomics study that monitored metabolic changes in the urine composition of RA patients compared to those observed in healthy controls have been presented. Ingenuity pathway analysis was used to elucidate the differentiated altered metabolites and associated pathways. In this study, metabolic phenotype features and regulated metabolite signatures of RA were successfully characterized using integrated high-throughput metabolomics coupled with multivariate data analysis and ingenuity pathway analysis.
2. Experimental
2.1 Reagents and materials
HPLC grade acetonitrile was provided by Merck (Germany). Leucine-enkephalin was provided by Sigma-Aldrich (St. Louis, MO, USA). Water was produced by a Milli-Q ultra-pure water system (Millipore, Billerica, USA). Both methanol and formic acid (HPLC grade) were provided by Tedia (USA). The standards including kynurenic acid, hippuric acid, glycine, 3-methyluridine, and acetylcysteine were obtained from Sigma-Aldrich (St. Louis, MO, USA).
2.2 Patient enrollment
The study was approved by the Ethics Committee of the Heilongjiang University of Chinese Medicine and written informed consent was obtained from all participants involved in this study. A total of 139 patients together with 124 normal cases were recruited. All subjects gave written informed consent. RA patients were required to be positive for RF and to have a RA disease duration of 6.5 years. The healthy control participants were recruited from hospital and university staff. These healthy individuals had no disease symptoms and did not use any medication. Detailed baseline and histopathologic characteristics for these patients are listed in the ESI Table 1.† Participants involved in this study did not take any medications, surgery, radiotherapy or chemotherapy, and those suffering from metabolic diseases, liver diseases, kidney diseases or any other cancers were excluded. Participants did not receive any drugs before one week of enrollment. Urine samples from RA subjects on week 1 of enrollment were selected for metabolomic profiling. All the subjects were fasting before 10 p.m., and the samples were obtained in the morning between 8 and 9 a.m. All samples were processed in the same laboratory to avoid any bias.
2.3 Sample preparation
Sample preparation for the urine metabolomics analysis was conducted as follows: 400 μL of acetonitrile was added to 100 μL of urine, then vortexed for 1 min, and centrifuged for 10 min at a speed of 10
000 rpm at 4 °C. After this, 400 μL of supernatant was transferred in a freeze-dryer. Finally, the dried supernatant was dissolved in 100 mL water/acetonitrile (4
:
1, v/v) solution at 4 °C, and then the supernatant was filtered through a 0.22 μm membrane. Quality control (QC) was the mixture obtained from the equivalent volume in each sample. The urine was stored at −80 °C until analysis. Urine samples were thawed at room temperature before analysis.
2.4 UPLC analysis
Urine samples were prepared for fast ultra UPLC-QTOF/MS (Waters corp., Milford, USA) analysis.24 Chromatographic separation was performed using a BEH C8 column (2.1 × 100 mm, 1.7 μm, Waters corp., Milford, USA) with a Waters LC system. The column temperature was maintained at 40 °C and the injection temperature was 4 °C. The analysis was performed using a fast ultra LC/TOF-MS with gradient elution using 0.1% formic acid in water as mobile phase A and 0.1% formic acid in acetonitrile as mobile phase B. Gradient elution was performed with the following solvent system: (A) 0.1% formic acid–water and (B) acetonitrile (ACN). The gradient was as follows: 1–10% A at 0–2.0 min, 10–30% A at 2.0–6.0 min, 30–50% A at 6.0–10.0 min, 50–90% A at 10.0–11.0 min, and 90% A at 11.0–13.0 min. The flow rate was 2 mL min−1 and the injection volume was 3 mL. The technical variation of the analysis over time was monitored by a pooled QC sample.
2.5 High-resolution mass spectrometry
MS experiments were performed using a fast time of flight mass spectrometer (Waters corp., Milford, USA) equipped with an ESI source in the positive ion mode. The mass range was set at 50–1000 m/z in the full scan mode. The following parameters were used: capillary voltage, 3.0 kV; cone voltage, 25 V; collision energy, 5 eV; desolvation gas, 500 L h−1; cone gas, L h−1; desolvation temperature, 300 °C; and source temperature, 110 °C. Leucine-enkephalin was used as the lock mass.
2.6 Chemometrics analysis
The urine metabolome measurements resulted in a list of features after preprocessing the data using Masslynx 4.1 (Waters corp., Milford, USA). The urine data were processed by EZinfo software (V2, Waters corp., Milford, USA) for peak picking and peak alignment. The parameter of MZ width was set at 0.01 Da and RT width was set at 0.2 min. Principal component analysis (PCA) and orthogonal projection to least squares discriminant analysis (OPLS-DA) calculations were performed by EZinfo software (V2, Waters corp., Milford, USA) using the autofit function. Initially, PCA was first used to reduce the dimensionality of the multidimensional dataset, while giving a comprehensive view of the clustering trend for the multidimensional data. OPLS-DA was then advanced for global and targeted profiling from the converted spectral data. In addition, a quantitative estimation of the discriminatory power of each descriptor was evaluated using the VIP (variable importance for the projection) parameters provided by OPLS-DA. The VIP value was used to evaluate the variable contribution and find out the potential biomarkers. Metabolites were identified by automated comparison of the ion features that included retention time, m/z, adducts, and fragments.
2.7 Ingenuity pathways analysis
To explore the typical metabolic perturbations associated with the related metabolites, a pathway analysis using the IPA system (http://www.ingenuity.com/), which is a web-based software application that identifies biological pathways and functions relevant to biomolecules of interest, was performed. We uploaded the metabolite lists (with KEGG IDs) and the changes of the related metabolites onto an IPA server. Canonical pathways and molecular interaction networks were generated based on the IPA knowledge.
2.8 Statistical analyses
The t-test analyses of covariance were performed using SPSS software (version 17.0; SPSS, Inc., Chicago, IL), with p < 0.05 deemed to be significant. A further diagnostic property of important metabolites was deduced by receiver operating characteristic (ROC) curve analysis using MedCalc software (Broekstraat, Mariakerke, Belgium).
3. Results
3.1 Typical chromatograms
In this study, we used UPLC-QTOF/MS to collect the metabolite information of RA. Representative BPI of a urine sample is shown in Fig. 1A. The utilization of QC samples throughout the analytical run to monitor the data quality was a pragmatic solution for complex multi-component-based metabolomic research. As shown in Fig. 1B in the ESI,† the QC samples closely clustered together in the PCA score plots, which suggests that the analytical systems were stable and reliable.
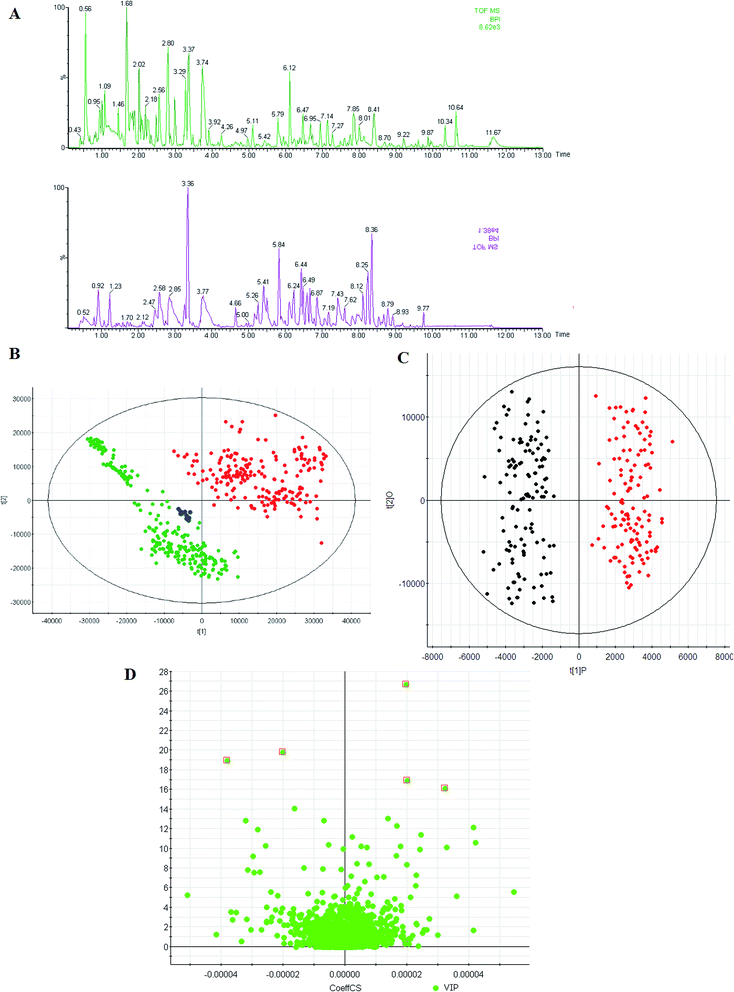 |
| Fig. 1 Metabolic profiles analysis. (A) The typical base peak chromatograms of control subjects (up) and RA patients (down) by UPLC-QTOF/MS, (B) score plot of the PCA model for control subjects (green), RA group (red), and QC samples (blue), (C) score plot of the OPLS-DA model of the UPLC-QTOF/MS data from RA patients (red) and controls (green) group, (D) VIP-plot for selection of interesting variables for patients with RA and age-matched healthy controls. | |
3.2 Metabolic profiles
Mean-centered MS data, normalized to total area, were subjected to unsupervised modeling by PCA that provided an overview of data and a means of detecting outliers. Thorough investigation of subsequent PC's revealed grouping according to the presence of RA. Scores plots showed separation between these two groups, highlighting the presence of variations related to the disease. To specify the metabolic variations related with the disease, an OPLS-DA model, which is a standard and well accepted technique used in the metabolomics field to discover characteristic differences between RA and healthy groups, was built for the urine data set to find the most discriminating features between the RA and healthy groups. OPLS-DA was applied in an effort to extract systematic parts of information discriminating patients and controls. The LC-MS data were subjected to PLS-DA. As shown in the score plots (Fig. 1C), the RA and healthy groups could be separated into two distinct clusters, which indicated that the state of metabolism was different between the RA and healthy groups. Fig. 1C shows that the separations of the two groups can be achieved with the model parameters R2Y = 0.91 and Q2 = 0.83 for LC-MS data. R2Y and Q2 show the explanative ability and predictive ability of the model, respectively.
3.3 Differential metabolites
Identification of potential biomarker candidates that account for the differentiation of diseases is a necessary step not only for diagnosis, but also for better understanding of the functional metabolism in clinical diseases. To screen putative biomarkers for RA, variable importance for projection (VIP) values from the OPLS-DA model were obtained. VIP values were used to rank the contribution of metabolites to discriminate between the RA and healthy groups, which are based on weighted coefficients of the OPLS-DA model (Fig. 1D). VIP analysis showed the order of metabolites according to their critical influence on clustering. The key metabolites, which were essential for distinguishing between the RA and healthy groups, were selected from the results of the important metabolites (VIP > 15). Then, the Student's t-test (p-value < 0.05) led to further testing of the selected metabolites with high VIP values as biomarker candidates for RA. VIP and the p-value confirmed the key metabolites for separating the groups from the other groups. Elemental compositions were calculated using Masslynx 4.1 software. Evidence for the identity of features was accumulated by integrating information on accurate mass of the ions and some fragments, retention time, HMDB database, and in house human urine compound lists. Then, the identification of some compounds was verified by authentic standards. In this study, 5 metabolites (VIP > 15 and P < 0.001) were found to have VIP values higher than 15, of which 4 metabolites were higher in the RA group, whereas 1 metabolite was higher in the healthy group. Note that the fold change of succinate was highest in the RA group, and the fold changes of taurocholic acid, hippurate, and L-asparagine were much higher than those of other metabolites in the RA group. The fold changes of the metabolite abundances increased in the RA group and ranged from 2.76 to 32.15 (Table 1).
Table 1 Information on biomarker metabolites analyzed by UPLC-QTOF/MS
No. |
VIP |
RT |
[M + H]+ |
Formula |
ppm |
Name |
HMDB |
Change |
(p) |
Trend |
AUC |
Sensitivity (%) |
Specificity (%) |
1 |
26.68 |
7.72 |
516.2921 |
C26H46NO7S |
−1.88 |
Taurocholic acid |
HMDB00036 |
32.15 |
0.0001 |
↑ |
0.993 |
98.7 |
95.7 |
2 |
19.81 |
6.59 |
179.0514 |
C9H9NO3 |
1.51 |
Hippurate |
HMDB00714 |
17.54 |
0.0002 |
↑ |
0.972 |
95.2 |
96.3 |
3 |
18.97 |
6.93 |
220.9994 |
C4H8N2O3 |
1.39 |
L-Asparagine |
HMDB00168 |
9.92 |
0.0002 |
↑ |
0.897 |
88.7 |
84.2 |
4 |
16.94 |
5.17 |
466.3082 |
C26H44NO6 |
1.14 |
Glycocholic acid |
HMDB00138 |
3.48 |
0.0009 |
↓ |
0.823 |
84.7 |
82.8 |
5 |
16.10 |
3.23 |
393.2948 |
C24H41O4 |
−1.30 |
Chenodeoxycholic acid |
HMDB00518 |
2.76 |
0.0012 |
↓ |
0.819 |
80.2 |
82.3 |
3.4 Network function analysis
Ingenuity Pathway Analysis (IPA) was applied with the related metabolites to explore pathway and network analysis of the differentially expressed metabolites in RA. The top six altered pathways were generated beyond the discriminating metabolites and are listed in Table S2.† In the network function analysis, the related metabolites in RA tended to gather into an integrated network (Fig. 2A). Bioinformatics analysis with the IPA software found a strong correlation between bile acid biosynthesis, hepatic cholestasis, asparagine degradation I, asparagine biosynthesis I, FXR/RXR activation, tRNA charging, and these metabolites (Fig. 2B).
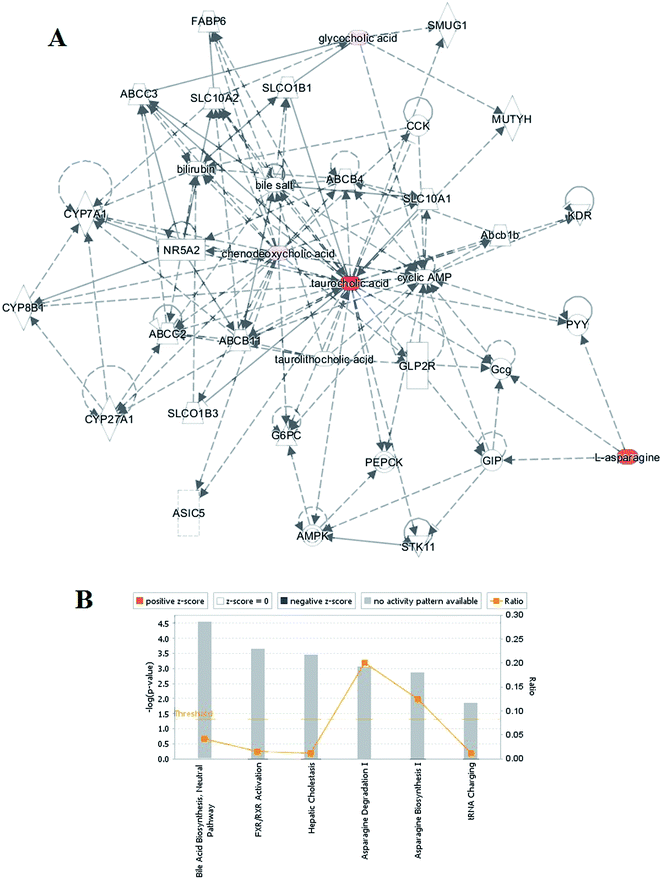 |
| Fig. 2 Ingenuity pathway analysis. (A) The merged network of the identified metabolites. Metabolite symbols in red were up-regulated, whereas those in pink were down-regulated. Solid lines between molecules indicate a direct physical relationship between molecules, whereas dotted lines indicate indirect functional relationships. (B) Network function analysis of the differential metabolites by ingenuity pathway analysis. | |
3.5 ROC analysis
The diagnostic potential of these metabolic biomarkers for RA was evaluated in the external validation data, as described in the methods section. The area under the ROC curve (AUC) provides a numerical value of the relationship between the specificity and sensitivity of a biomarker. Furthermore, the sensitivity and specificity indicate the probably tests for correctly identifying patients with RA and without RA (healthy groups), respectively. An AUC value of 1.0 indicates perfect prediction of the diagnostic test. Table S2† also shows the ROC curve analysis for the predictive power of the 5 biomarkers (taurocholic acid, hippurate, L-asparagine, glycocholic acid, and chenodeoxycholic acid) of the RA group to discriminate RA and healthy groups. The values of AUC ranged from 0.819 to 0.993 and indicated the potential capacity of these metabolites to distinguish RA patients from normal subjects (Table 1). Note that taurocholic acid had a sensitivity of 98.7% and a specificity of 95.7%, which were obtained from the ROC curve, and the value of AUC was 0.993, exhibited good diagnostic performance. To demonstrate the utility of urine biomarkers for the early diagnosis of RA, taurocholic acid and hippurate were selected to form a biomarker group, indicating a high predictive ability for RA patients.
4. Discussion
Metabolomic biomarkers are potentially related to the clinical manifestation of disease. However, to the best of our knowledge, in-depth study of the relationship between the urine metabolite profile and RA is still required. Mass spectrometry has been applied to identify metabolic signatures associated with disease.24–28 In the present study, we report a mass spectrometry-based metabolic phenotyping study to identify the global metabolic defects as well as distinct metabolic signatures of RA in comparison with healthy control subjects. We also aimed to further characterize metabolic signatures and potential biomarkers of RA. In this study, an untargeted metabolomics study based on an UPLC-QTOF/MS technique was performed to investigate dysregulated metabolic signatures in urine samples of RA patients. We first compared the metabolic characteristics of RA patients with those of healthy controls using a LC-MS platform combined with multivariate statistical analysis. The findings demonstrated good discrimination between RA and healthy controls. On the basis of the VIP threshold and Student's t-test, a total of 5 identified potential biomarkers were selected. Among these, taurocholic acid and hippurate were selected to form a biomarker group, indicating a high predictive ability for RA patients. The top canonical pathways were bile acid biosynthesis, hepatic cholestasis, asparagine degradation I, asparagine biosynthesis I, FXR/RXR activation, and tRNA charging. These results suggested that combining UPLC-MS and multivariate data analysis techniques can be used for a comprehensive urine metabolomics analysis and screening of biomarkers for the diagnosis of RA.
Taurocholic acid is a bile acid and is the product of conjugation of cholic acid with taurine. Bile acids are physiological detergents that facilitate excretion, absorption, and transport of fats and sterols in the intestine and liver.29 Bile acids are also steroidal amphipathic molecules derived from the catabolism of cholesterol. They modulate bile flow and lipid secretion, essential for the absorption of dietary fats and vitamins, and have been implicated in the regulation of all the key enzymes involved in cholesterol homeostasis.30 Hippurate is an acyl glycine formed by the conjugation of benzoic acid with glycine. Acyl glycines are produced by the action of glycine N-acyltransferase, which is an enzyme that catalyzes the chemical reaction.31 Moreover, L-asparagine is not an essential amino acid, which means that it can be synthesized from central metabolic pathway intermediates in humans and is not required in the diet. The precursor to asparagine is oxaloacetate, which is converted into aspartate using a transaminase enzyme.32 The enzyme transfers the amino group from glutamate to oxaloacetate producing alpha-ketoglutarate and aspartate. The enzyme asparagine synthetase produces asparagine, AMP, glutamate, and pyrophosphate from aspartate, glutamine, and ATP. In the asparagine synthetase reaction, ATP is used to activate aspartate, forming beta-aspartyl-AMP.33 Glycocholic acid is an acyl glycine and a bile acid–glycine conjugate. It is a secondary bile acid produced by the action of enzymes existing in the microbial flora of the colonic environment.34 In hepatocytes, both primary and secondary bile acids undergo amino acid conjugation at the C-24 carboxylic acid on the side chain, and therefore, almost all bile acids in the bile duct exist in a glycine conjugated form. Chenodeoxycholic acid is a bile acid. Bile acids are steroid acids found predominantly in the bile of mammals. Bile acids are physiological detergents that facilitate excretion, absorption, and transport of fats and sterols in the intestine and liver. They have been implicated in the regulation of all the key enzymes involved in cholesterol homeostasis.30 Currently, the application of metabolomics to urine samples has been successful in finding potential biomarkers for diseases.35–39 Our findings have defined a panel of molecules whose levels are altered in the urine of RA patients. The urine biomarkers identified in this study exhibited satisfactory diagnostic performance. The strength of this study is that urine analysis with metabolomics is simple and noninvasive. In conclusion, this is the first report on the identification of potential biomarkers for RA using urine of human RA patients. We also demonstrated that high-throughput metabolomics coupled with multivariate data analysis and ingenuity pathway analysis may be a useful tool to discover biomarkers.
5. Conclusion
In this study, we have reported the potential of metabolomics to more effectively and efficiently diagnose RA and discriminate between RA patients and healthy subjects. Urine metabolite profiling showed RA to be associated with a profound abnormality in metabolic phenotype. The levels of taurocholic acid, hippurate, L-asparagine, glycocholic acid, chenodeoxycholic acid in the RA cases were significantly different from those in the control subjects. The five most significantly different pathways were tRNA charging, bile acid biosynthesis, hepatic cholestasis, asparagine degradation I, asparagine biosynthesis I, and FXR/RXR activation. The biomarkers identified in this study exhibited satisfactory diagnostic performance. The discovery of candidate biomarkers in RA patients is an essential research area for the improvement of disease monitoring and the development of personalized medicine. The findings suggest high-throughput metabolomics coupled with multivariate data analysis, and the ingenuity pathway analysis provided a feasible way to discover regulated metabolite signatures of RA that possesses great potential for the diagnosis of RA patients.
Competing financial interests
The authors declare no competing financial interests.
Acknowledgements
This work was supported by grants received from theNatural Science Foundation of Heilongjiang Province of China (H201330), the Outstanding Innovative Talent Support Program of Heilongjiang University of Chinese Medicine (2012RCQ29), Key Program of Natural Science Foundation of State (Grant No. 81302905), Natural Science Foundation of Heilongjiang Province of China (H2015038), and Youth Innovative Talent Program of Heilongjiang Province of China (UNPYSCT-2015118).
References
- Y. Okada, D. Wu, G. Trynka, T. Raj, C. Terao and K. Ikari, et al., Genetics of rheumatoid arthritis contributes to biology and drug discovery, Nature, 2014, 506(7488), 376–381 CrossRef CAS PubMed.
- L. Vande Walle, et al., Negative regulation of the NLRP3 inflammasome by A20 protects against arthritis, Nature, 2014, 512(7512), 69–73 Search PubMed.
- Y. Ito, et al., Detection of T cell responses to a ubiquitous cellular protein in autoimmune disease, Science, 2014, 346(6207), 363–368 CrossRef CAS PubMed.
- I. Surolia, et al., Functionally defective germline variants of sialic acid acetylesterase in autoimmunity, Nature, 2010, 466(7303), 243–247 CrossRef CAS PubMed.
- X. Zhang, et al., The oral and gut microbiomes are perturbed in rheumatoid arthritis and partly normalized after treatment, Nat. Med., 2015, 21(8), 895–905 CrossRef CAS PubMed.
- W. Tang, et al., The growth factor progranulin binds to TNF receptors and is therapeutic against inflammatory arthritis in mice, Science, 2011, 332(6028), 478–484 CrossRef CAS PubMed.
- J. Bromberg, TNF-α trips up Treg cells in rheumatoid arthritis, Nat. Med., 2013, 19(3), 269–270 CrossRef CAS PubMed.
- S. Lefèvre, et al., Synovial fibroblasts spread rheumatoid arthritis to unaffected joints, Nat. Med., 2009, 15(12), 1414–1420 CrossRef PubMed.
- T. J. Wang, et al., Metabolite profiles and the risk of developing diabetes, Nat. Med., 2011, 17(4), 448–453 CrossRef CAS PubMed.
- P. Emery, et al., Sustained remission with etanercept tapering in early rheumatoid arthritis, N. Engl. J. Med., 2014, 371(19), 1781–1792 CrossRef CAS PubMed.
- L. Guo, et al., Plasma metabolomic profiles enhance precision medicine for volunteers of normal health, Proc. Natl. Acad. Sci. U. S. A., 2015, ii, 201508425 Search PubMed.
- T. J. Wang, et al., Metabolite profiles and the risk of developing diabetes, Nat. Med., 2011, 17(4), 448–453 CrossRef CAS PubMed.
- R. M. Onjiko, et al., Single-cell mass spectrometry reveals small molecules that affect cell fates in the 16-cell embryo, Proc. Natl. Acad. Sci. U. S. A., 2015, 112(21), 6545–6550 CrossRef CAS PubMed.
- X. Liu, et al., Sepsis biomarkers: an omics perspective, Front. Med., 2014, 8(1), 58–67 CrossRef PubMed.
- R. J. Langley, et al., Integrative “omic” analysis of experimental bacteremia identifies a metabolic signature that distinguishes human sepsis from systemic inflammatory response syndromes, Am. J. Respir. Crit. Care Med., 2014, 190(4), 445–455 CrossRef CAS PubMed.
- M. M. Khamis, D. J. Adamko and A. El-Aneed, Mass spectrometric based approaches in urine metabolomics and biomarker discovery, Mass Spectrom. Rev., 2015 DOI:10.1002/mas.21455.
- M. Smoluch, P. Mielczarek and J. Silberring, Plasma-based ambient ionization mass spectrometry in bioanalytical sciences, Mass Spectrom. Rev., 2016, 35(1), 22–34 CrossRef CAS PubMed.
- A. Zhang, H. Sun and X. Wang, Mass spectrometry-driven drug discovery for development of herbal medicine, Mass Spectrom. Rev., 2016 DOI:10.1002/mas.21529.
- R. J. Langley, et al., An integrated clinico-metabolomic model improves prediction of death in sepsis, Sci. Transl. Med., 2013, 5, 195ra95 Search PubMed.
- R. Priori, L. Casadei, M. Valerio, R. Scrivo, G. Valesini and C. Manetti, 1H-NMR-based metabolomic study for identifying serum profiles associated with the response to etanercept in patients with rheumatoid arthritis, PLoS One, 2015, 10(11), e0138537 Search PubMed.
- S. P. Young, S. R. Kapoor, M. R. Viant, J. J. Byrne, A. Filer, C. D. Buckley, G. D. Kitas and K. Raza, The impact of inflammation on metabolomic profiles in patients with arthritis, Arthritis Rheum., 2013, 65(8), 2015–2023 CrossRef CAS PubMed.
- S. R. Kapoor, A. Filer, M. A. Fitzpatrick, B. A. Fisher, P. C. Taylor, C. D. Buckley, I. B. McInnes, K. Raza and S. P. Young, Metabolic profiling predicts response to anti-tumor necrosis factor α therapy in patients with rheumatoid arthritis, Arthritis Rheum., 2013, 65(6), 1448–1456 CrossRef CAS PubMed.
- B. J. Blaise, et al., Metabolic phenotyping of traumatized patients reveals a susceptibility to sepsis, Anal. Chem., 2013, 85, 10850–10855 CrossRef CAS PubMed.
- P. Ràfols, D. Vilalta, J. Brezmes, N. Cañellas, E. Del Castillo, O. Yanes, N. Ramírez and X. Correig, Signal preprocessing, multivariate analysis and software tools for MA(LDI)-TOF mass spectrometry imaging for biological applications, Mass Spectrom. Rev., 2016 DOI:10.1002/mas.21527.
- R. Bandu, H. J. Mok and K. P. Kim, Phospholipids as cancer biomarkers: mass spectrometry-based analysis, Mass Spectrom. Rev., 2016 DOI:10.1002/mas.21510.
- Q. Liang, H. Liu, H. Xing, Y. Jiang and A. Zhang, UPLC-QTOF/MS based metabolomics reveals metabolic alterations associated with severe sepsis, RSC Adv., 2016, 6, 43293–43298 RSC.
- M. Wagner, D. Tonoli, E. Varesio and G. Hopfgartner, The use of mass spectrometry to analyze dried blood spots, Mass Spectrom. Rev., 2016, 35(3), 361–438 CrossRef CAS PubMed.
- W. D. Lehmann, A timeline of stable isotopes and mass spectrometry in the life sciences, Mass Spectrom. Rev., 2017, 36(1), 58–85 CrossRef CAS PubMed.
- M. V. St-Pierre, et al., Transport of bile acids in hepatic and non-hepatic tissues, J. Exp. Biol., 2001, 204(Pt 10), 1673–1686 CAS.
- T. Claudel, et al., The Farnesoid X receptor: a molecular link between bile acid and lipid and glucose metabolism, Arterioscler., Thromb., Vasc. Biol., 2005, 25(10), 2020–2030 CrossRef CAS PubMed.
- L. C. Amorim, et al., Determination of o-cresol by gas chromatography and comparison with hippuric acid levels in urine samples of individuals exposed to toluene, J. Toxicol. Environ. Health, 1997, 50(4), 401–407 CrossRef CAS.
- V. A. Nagaraj, et al., Asparagine requirement in Plasmodium berghei as a target to prevent malaria transmission and liver infections, Nat. Commun., 2015, 6, 8775 CrossRef PubMed.
- N. E. O'Connell, et al., Asparagine deamidation reduces DNA-binding affinity of the Drosophila melanogaster Scr homeodomain, FEBS Lett., 2015, ii: S0014–5793(15), 00869 Search PubMed.
- J. Y. Chiang, Bile acid regulation of hepatic physiology: III. Bile acids and nuclear receptors, Am. J. Physiol.: Gastrointest. Liver Physiol., 2003, 284(3), G349–G356 CrossRef CAS PubMed.
- Q. Liang, T. Zhang, Y. Jiang, H. Xing, C. Wang, B. Li and A. Zhang, Metabolomics of alcoholic liver disease: a clinical discovery study, RSC Adv., 2015, 5, 80381–80387 RSC.
- Q. Liang, H. Liu, H. Xing, Y. Jiang, T. Zhang and A. Zhang, High-resolution mass spectrometry for exploring metabolic signatures of sepsis-induced acute kidney injury, RSC Adv., 2016, 6, 29863–29868 RSC.
- A. Radionova, I. Filippov and P. J. Derrick, In pursuit of resolution in time-of-flight mass spectrometry: a historical perspective, Mass Spectrom. Rev., 2016, 35(6), 738–757 CrossRef CAS PubMed.
- Q. Liang, H. Liu, T. Zhang, Y. Jiang, H. Xing and A. Zhang, High-throughput metabolic profiling for discovering metabolic biomarkers of sepsis-induced acute lung injury, RSC Adv., 2016, 6, 11008–11013 RSC.
- Q. Liang, H. Liu, T. Zhang, Y. Jiang, H. Xing and A. Zhang, Potential urine biomarkers from a high throughput metabolomics study of severe sepsis in a large Asian cohort, RSC Adv., 2015, 5, 102204–102209 RSC.
Footnote |
† Electronic supplementary information (ESI) available. See DOI: 10.1039/c6ra27461g |
|
This journal is © The Royal Society of Chemistry 2017 |
Click here to see how this site uses Cookies. View our privacy policy here.