DOI:
10.1039/C6RA27247A
(Paper)
RSC Adv., 2017,
7, 58-66
Identification and analysis of promiscuity cliffs formed by bioactive compounds and experimental implications
Received
23rd November 2016
, Accepted 9th December 2016
First published on 22nd December 2016
Abstract
Multi-target activities of small molecules must be distinguished from apparent promiscuity resulting from assay artifacts. The molecular origins of specific multi-target activities are currently poorly understood. Compounds from the medicinal chemistry literature with available high-confidence activity data were systematically searched for ‘promiscuity cliffs’, defined as pairs of structural analogs with large differences between the number of targets they are active against. During the search, compounds with detectable aggregator properties, pan-assay interference characteristics, or other possible chemical liabilities were eliminated. A large number of promiscuity cliffs remained, many of which were centered on a limited number of highly promiscuous compounds, as revealed by network representations. The analysis of promiscuity cliffs often suggested follow-up experiments to further explore the molecular basis of promiscuity and assess the influence of data sparseness. Therefore, promiscuity cliffs identified herein are made freely available to support follow-up investigations.
Introduction
Compound promiscuity is often associated with non-specific interactions, aggregation effects, or other assay artifacts and as such is highly undesired.1–4 On the other hand, promiscuity is also rationalized as the ability of small molecules to specifically interact with multiple targets.5,6 Defined multi-target activities depart from the specificity (single target) paradigm that has ruled drug discovery efforts for a long time during the experimental reductionism era7,8 when target-based approaches took center stage. However, such activities become highly relevant in the context of polypharmacology, an emerging concept in drug discovery.9–11 Polypharmacology refers to functional effects (including undesired side effects) elicited by physiologically relevant multi-target activities of small molecules, as exemplified by kinase inhibitors that are successfully used in oncology.12 These compounds are known by now to inhibit multiple kinases (and potentially other targets as well).
A number of studies have attempted to identify structural features and molecular properties that may give rise to false-positive assay results or undesirable promiscuity associated with non-specific interactions.3,4,13,14 By contrast, molecular origins of polypharmacology-relevant promiscuity (specific multi-target interactions) mostly remain to be understood. Only a few studies have addressed related issues. For example, it was shown that many pharmaceutical target proteins are capable of recognizing structurally diverse small molecules.15 Furthermore, most promiscuous publicly available screening hits were identified16 and it was shown that many – but not all – of these molecules were associated with chemical liabilities such as those of pan-assay interference compounds (PAINS)3,4 or other potential reactivities and sources of assay artifacts.17 Furthermore, structural features or binding site characteristics that might give rise to promiscuity have been addressed in a few studies.18–20
We have attempted to further explore multi-target activities of small molecules. Therefore, the concept of ‘promiscuity cliffs’ was applied that was first introduced for the analysis of compound array experiments.21 In this study, large differences in apparent promiscuity of compounds from diversity-oriented synthesis were detected under the specific conditions of compound array experiments. We identified structural analogs with PD differences of 50 to more than 90 targets and assembled pair-wise promiscuity cliffs to represent selected examples.21 Therefore, promiscuity cliffs were defined as pairs of structural analogs with large differences in the number of targets they were active against. The analysis was focused on screening data and provided proof-of-concept for promiscuity cliffs. The same compound array data were also used in another investigation to explore large-magnitude differences in compound promiscuity and associate significantly different PDs of related compounds.22 Herein, for the first time we have systematically searched compounds from the medicinal literature for promiscuity cliffs. Care was taken to exclusively consider compounds for which high-confidence activity data were available and that were not associated with detectable chemical liabilities, as discussed above. A significant number of promiscuity cliffs was identified and these cliffs were analyzed in detail. The analysis also provided suggestions for further biological assays of cliff-forming compounds and the design of analogs to further explore possible origins of multi-target activities.
Material and methods
Compounds with high-confidence activity data
From ChEMBL23,24 (version 22), the main public repository of compounds from the medicinal chemistry literature, molecules with high-confidence activity data25 were extracted. For each qualifying compound, the presence of direct interactions (i.e., assay relationship type “D”) with human single-protein targets at the highest confidence level (i.e., assay confidence score 9) was required. As potency measurements, only explicitly specified equilibrium constants (Ki) and IC50 values were considered and compounds with activity records including comments such as ‘inactive’, ‘inconclusive’, or ‘not active’ were discarded. For compounds with multiple Ki or IC50 values for the same target, the geometric mean of the values was calculated as the final potency annotation if all values fell into the same order of magnitude. Otherwise the values were discarded. Furthermore, all compounds that originated from PubChem screening assays26 were omitted. This was done to predominantly focus the analysis on compound optimization data from medicinal chemistry. Because high-confidence activity data were exclusively considered, no potency threshold value was applied. In fact, for the analysis of promiscuity cliffs, weak activities should be taken into consideration to derive additional target hypotheses for structural analogs, as further discussed below, provided the measurements are reliable.
Promiscuity cliffs
For each qualifying compound, the PD (number of targets) was determined. Pairwise structural relationships were established based on the matched molecular pair (MMP) formalism.27,28 An MMP represents a pair of structural analogs that share a core structure (MMP-core) and are only distinguished by a structural modification at a single site, i.e. the exchange of a pair of substructures, termed a chemical transformation.28 Transformation-size restrictions were applied to limit structural changes to those typically observed in analog series.26 For the generation of transformation size-restricted MMPs, single-, dual, and triple-cut fragmentation of exocyclic bonds was carried out.29 Thus, MMPs might contain non-contiguous cores.
MMPs were systematically generated using an in-house implementation of the Hussain and Rea algorithm25 and with the aid of OpenEye Chemistry toolkit.30
For our analysis, a promiscuity cliff was defined as a pair of compounds that formed a transformation-size restricted MMP with a PD difference of at least 10 targets. Accordingly, a highly promiscuous cliff compound was required to have a PD of at least 11. From all pairs of analogs, promiscuity cliffs were selected on the basis of these criteria. Fig. 1 shows an exemplary promiscuity cliff.
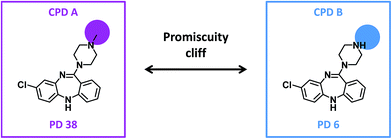 |
| Fig. 1 Promiscuity cliff. Shown are two compounds forming a promiscuity cliff. For each compound, the promiscuity degree (PD) is given. Structural modifications are highlighted. | |
Promiscuity cliffs were visualized in a network representation. In this network, nodes represented cliff compounds and edges connecting pairs of nodes the formation of promiscuity cliffs. Nodes were colored according to their PD (gray, 1; blue, 2–10; magenta, ≥11). The promiscuity cliff network was generated using Cytoscape.31
Pan-assay interference compounds and aggregators
Promiscuous cliff compounds were screened for PAINS3,4 and aggregators,1,2 which frequently give rise to artifacts under assay conditions, using public PAINS filters24,32,33 and the aggregation advisor.34
Results and discussion
Identification of promiscuity cliffs
The identification of promiscuity cliffs is summarized in Fig. 2. Initially, ChEMBL compounds with available high-confidence activity data were selected. Screening data containing active and inactive compounds were originally used to establish the presence of promiscuity cliffs.21 The systematic search for promiscuity cliffs and their analysis reported herein was intentionally focused on compounds from the medicinal chemistry literature and high-confidence activity. The identification of promiscuity cliffs with reliable target annotations including weak activities was considered an essential part of this study.
 |
| Fig. 2 Identification of promiscuity cliffs. The flowchart summarizes the steps involved in the identification of promiscuity cliffs formed by ChEMBL compounds. In addition, compound and cliff statistics are reported. HP stands for highly promiscuous. | |
Molecular scaffolds associated with different levels of promiscuity on the basis of the compounds they represented were identified previously.35 With the search for promiscuity cliffs, a transition was facilitated from compound scaffolds to analog pairs.
The initial selection set contained 1260 promiscuity cliffs formed by a total of 1207 compounds, which were then screened for PAINS and aggregators. Compounds with detected PAINS/aggregator alerts were removed, which reduced the number of promiscuity cliffs to 1111. The remaining cliffs were formed by 117 compounds with a PD ≥ 11 and 1000 with a smaller PD. The 117 most promiscuous compounds were then subjected to careful visual inspection to identify other potential chemical liabilities not detected as PAINS that might also cause artificial assay readouts, as reported previously.17 As summarized in Fig. 3, 42 compounds were classified as potentially reactive or fluorescent on the basis of visual inspection and also removed from further consideration. Thus, the final selection consisted of 784 promiscuity cliffs that were formed by 75 highly promiscuous compounds and 763 cliff partners. The PD of the most promiscuous compounds ranged from 11 to 38.
 |
| Fig. 3 Compounds with potential liabilities. A total of 42 highly promiscuous compounds, not detected as PAINS or aggregators using public filters, were omitted from further consideration on the basis of visual inspection, due to potential reactivity or other chemical liabilities that might give rise to assay artifacts. The pie chart reports the distribution of these compounds over seven assigned liability categories. For each category, the number of compounds is given and an exemplary compound is shown. | |
Transformations constituting these promiscuity cliffs were extracted and compared to those of promiscuity cliffs originating from compound array screening data.21 Only minute overlap i.e. (two shared transformations) was detected, indicating the uniqueness of cliff-forming transformations from different compound sources. Furthermore, transformations that yielded promiscuity cliffs with high frequency across different targets were not detected.
Distribution of promiscuity degrees over cliffs
Fig. 4 reports the frequency of promiscuity cliffs formed by compounds with different PDs. The majority of cliffs (71%) combined a compound with a single target annotation and another with 11 or more annotations. The three most frequent PD combinations were 1/11 (158 cliffs), 1/12 (114), and 1/16 (90). Nine promiscuity cliffs each with PD combinations 1/38 and 2/38 were found. In addition, there were 25 instances of cliffs with PD combination 3/15 and 10 with combination 3/19. By contrast, promiscuity cliffs involving compounds with PD > 3 and PD > 15, respectively, were rare. For compounds at these promiscuity levels, four cliffs with PD combination (6/16) were detected, which was the largest number per combination. The two cliffs combining most promiscuous compounds represented PD combination 6/32 and 6/38, respectively. Thus, most promiscuity cliffs were formed by target-specific and promiscuous compounds, whereas cliffs only involving promiscuous compounds with PD > 3 were only rarely detected. We note that medicinal chemistry literature records do not provide information about how frequently compounds might have been tested against different targets. Thus, apparent target specificity may – or may not – result from data incompleteness.36 Therefore, analysis of promiscuity cliffs offers the opportunity to examine other potential targets of compounds with apparent specificity by considering promiscuous structural analogs, as further discussed below.
 |
| Fig. 4 Promiscuity degrees of cliff compounds. For each promiscuity cliff, the PD of the two participating compounds is compared. Cells contain the number of cliffs with the corresponding PD combination. In addition, cells are colored according to the number of cliffs they represent applying a spectrum from green (largest number of cliffs) over yellow to red (single cliff). Empty cells represent PD combinations that were not detected. | |
Target distribution
Fig. 5a reports to how many families the targets of promiscuous compounds with PD ≥ 11 belong. Targets were assigned to families on the basis of the UniProt classification scheme.37 Target families representing multiple compounds included the protein kinase family (35 compounds), the G protein coupled receptor (GPCR) family 1 (27 compounds), and the sodium neurotransmitter symporter (TC 2.A.22) family (19 compounds). For 33 compounds with a maximal PD of 31, all targets belonged to the same family. In addition, targets of 31 highly promiscuous compounds belonged to two families. Among others, these target combination included GPCR family 1 and the sodium neurotransmitter symporter family (13 compounds) or protein kinase family and the selectin/LECAM family (5 compounds). By contrast, only 11 compounds were identified with activity against targets from three or more families. As an exception, the single most promiscuous compound (PD 38) was annotated with targets from seven families. The distribution in Fig. 5a reveals that many promiscuous compounds were active against related targets, as one might expect. For the exploration of promiscuity patterns, compounds with activity against two or more target families are also of considerable interest. In Fig. 5b the 30 protein families are given for 42 compounds whose targets belonged to multiple families. A protein family network was generated in which each of these 30 families was represented by a node. Edges connected nodes if the corresponding families shared at least one highly promiscuous (HP) compound. On the basis of protein–HP compound relationships, five groups (individual clusters) of related protein families were identified. The largest group (consisting of 15 protein families) included, among others, G protein coupled receptors, sodium neurotransmitter symporter, and kinases. Furthermore, two smaller groups of five closely related target families were identified (families 16–20 and 21–25, respectively) which shared a single compound. Fig. 5c shows exemplary compounds that were exclusively active against targets from a single family or targets from different families.
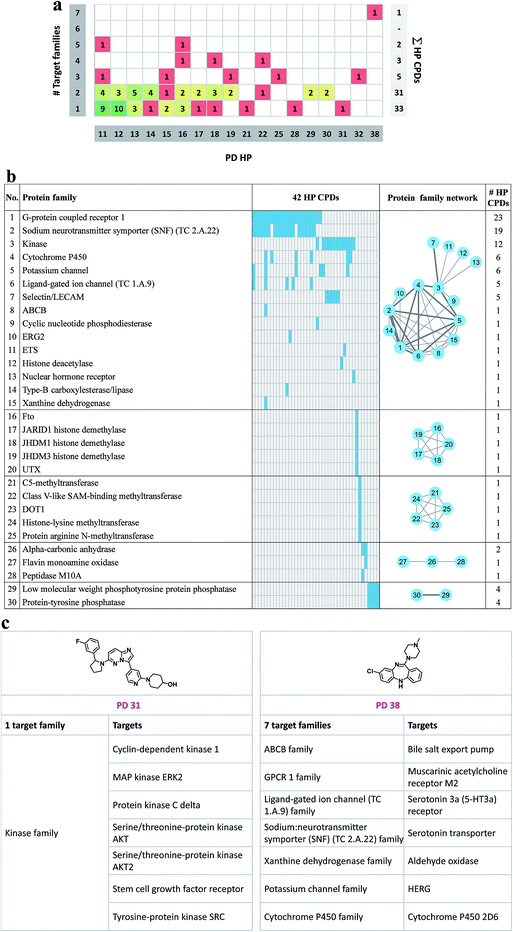 |
| Fig. 5 Target families of highly promiscuous compounds. (a) On the horizontal axis, highly promiscuous compounds are ordered according to their PD (ranging from 11 to 38). On the vertical axis on the left, the number of different families is reported to which the corresponding targets belong. Cells contain the number of compounds representing a given target family/PD combination. In addition, cells are colored according to the number of compounds they represent applying a spectrum ranging from green (largest number) over yellow to red (single compound). On the vertical axis on the right, the sum of highly promiscuous compounds is given for each number of target families. (b) Given are the protein target families (numbered from 1 to 30) of highly promiscuous compounds (42 HP CPDs). For each family, the total number of HP compounds is given. Protein–HP compound relationships are shown in a matrix format in which cells represent protein–HP compound combinations. Cells are colored blue if the HP compound is active against a target from this family. Otherwise, cells are colored gray. In addition, a protein family network is shown in which nodes represent families (labeled with the family number). Two nodes are connected by an edge if the corresponding families share an HP compound. Edge thickness scales with the number of shared compounds. (c) Two examples of highly promiscuous compounds are shown whose targets belong to the same family or are distributed across different families. Target families and exemplary targets are designated. | |
Promiscuity cliff network
A network representation was generated to determine how promiscuity cliffs were arranged across participating compounds, as shown in Fig. 6. In this network, nodes represent compounds and edges pairwise promiscuity cliffs. A striking feature of the network was its extensive cluster structure. A variety of compound clusters with “star” topology (i.e. including a central densely connected node) was observed including several large clusters. Thus, many promiscuity cliffs were centered on individual highly promiscuous compounds for which multiple structural analogs with low PDs were available. In such cases, targets associated with analog relationships can be further explored. In addition, smaller clusters with different topologies were observed that were often predominantly formed by promiscuous compounds. Fig. 7 shows representative promiscuity cliffs from different cluster environments, which are also highlighted in Fig. 6. These promiscuity cliffs represent small chemical modifications of analogs having large PD differences.
 |
| Fig. 6 Promiscuity cliff network. Shown is a promiscuity cliff network. Nodes represent cliff compounds and edges indicate the formation of promiscuity cliffs. In addition, nodes are colored according to their PD (gray, 1; blue, 2–10; magenta, ≥11). Selected clusters containing highly promiscuous compounds are labeled (1–6). | |
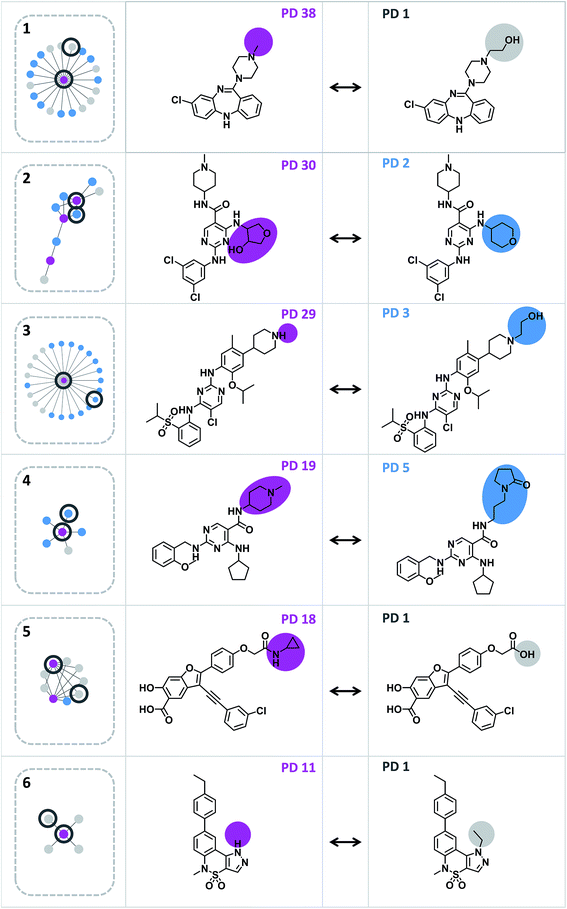 |
| Fig. 7 Exemplary promiscuity cliffs. For each of the six clusters in Fig. 6 (1–6), an exemplary cliff is shown (represented according to Fig. 1). | |
Experimental design
Given their characteristic features discussed above, promiscuity cliffs often provide immediate suggestions for follow-up experiments. For example, Fig. 8 shows two highly promiscuous compounds with modifications at a single site, leading to the formation of multiple cliffs. In such instances, cliff compounds with low PDs can be tested against additional targets associated with their highly promiscuous cliff partner. Hence, additional targets of analogs may be confirmed, thereby directly addressing the issue of data incompleteness. In addition, structural modifications may be identified that render compounds more promiscuous (if such modifications exit). Furthermore, Fig. 9 shows a series of promiscuity cliffs that involve chemical modification of a highly promiscuous compound at different sites. In such instances, analogs can be designed that probe contributions of specific modifications at different sites and their combinations to promiscuity, as also illustrated in Fig. 9. Thus, promiscuity cliffs often suggest follow-up experiments to further explore multi-target activities of small molecules.
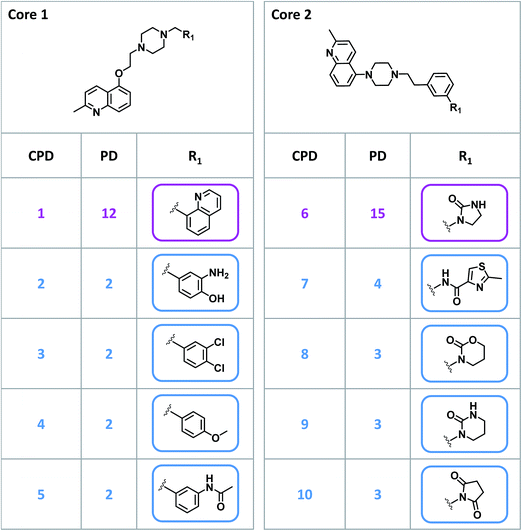 |
| Fig. 8 Promiscuity cliffs with single-site variations. For two highly promiscuous compounds (CPD 1 and CPD 6), four promiscuity cliff partners (CPDs 2–5 and CPDs 7–10, respectively) are depicted in an R-group table format. The invariant core structures (core 1 and core 2) are shown at the top. These cliffs exclusively involve chemical modifications at a single site (R1). In addition, PDs of all compounds are provided. | |
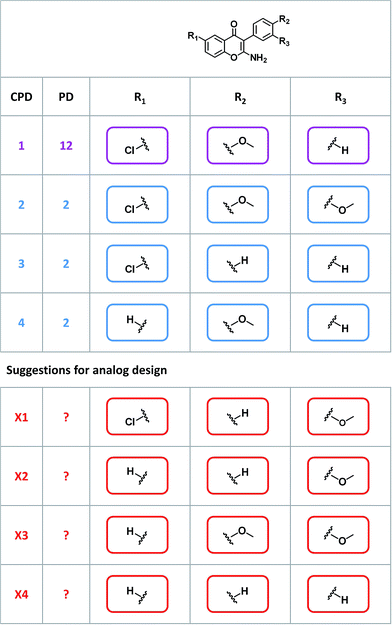 |
| Fig. 9 Promiscuity cliffs with variations at different sites. For a highly promiscuous compound, three promiscuity cliff partners are depicted in an R-group table format. The invariant core structure is shown at the top. These cliffs involve chemical modifications at three sites (R1, R2, and R3). For all compounds, PDs are reported. Compounds X1–X4 represent analogs with novel combinations of R-groups designed to further explore possible origins of promiscuity. | |
Data availability
To support follow-up studies, all promiscuity cliffs reported herein and their target information are made freely available in an organized form as an open access deposition.38
Concluding remarks
In this study, we have systematically searched for promiscuity cliffs formed by compounds from medicinal chemistry sources. Given the stringent selection criteria, these promiscuity cliffs are anticipated to have a high level of authenticity. A significant number of cliffs was obtained by applying the matched molecular pair concept and the composition and target distribution of these cliffs was analyzed in detail. A promiscuity cliff network revealed that many cliffs were centered on limited numbers of highly promiscuous compounds and formed disjoint cliff clusters. Comparison of these compounds and their cliff partners frequently suggested additional experiments to explore the role of data sparseness and further analyze structural features that might contribute to promiscuity.
Acknowledgements
The use of OpenEye's toolkits was made possible by their free academic licensing program.
References
- S. L. McGovern, E. Caselli and N. A. Grigorieff, J. Med. Chem., 1996, 45, 1712–1722 CrossRef.
- B. K. Shoichet, Drug Discovery Today, 2006, 11, 607–615 CrossRef CAS PubMed.
- J. B. Baell and G. A. Holloway, J. Med. Chem., 2010, 53, 2719–2740 CrossRef CAS PubMed.
- J. Baell and M. A. Walters, Nature, 2014, 513, 481–483 CrossRef CAS PubMed.
- Y. Hu and J. Bajorath, Drug Discovery Today, 2013, 18, 644–650 CrossRef CAS PubMed.
- Y. Hu and J. Bajorath, F1000Research, 2013, 2, 144 Search PubMed.
- P. Nurse, Nature, 1997, 387, 657 CrossRef CAS PubMed.
- D. H. Roukos, Pharmacogenomics, 2011, 12, 695–698 CrossRef PubMed.
- G. V. Paolini, R. H. Shapland, W. P. van Hoorn, J. S. Mason and A. L. Hopkins, Nat. Biotechnol., 2006, 24, 805–815 CrossRef CAS PubMed.
- A. D. Boran and R. Iyengar, Curr. Opin. Drug Discovery Dev., 2010, 13, 297–309 CAS.
- A. Anighoro, J. Bajorath and G. Rastelli, J. Med. Chem., 2014, 57, 7874–7887 CrossRef CAS PubMed.
- Z. A. Knight, H. Lin and K. M. Shokat, Nat. Rev. Cancer, 2010, 10, 130–137 CrossRef CAS PubMed.
- J.-U. Peters, P. Schnider, P. Mattein and M. Kansy, ChemMedChem, 2009, 4, 680–686 CrossRef CAS PubMed.
- R. F. Bruns and I. A. Wilson, J. Med. Chem., 2012, 55, 9763–9772 CrossRef CAS PubMed.
- Y. Hu and J. Bajorath, PLoS One, 2015, 10, e0126838 Search PubMed.
- S. Jasial, Y. Hu and J. Bajorath, PLoS One, 2016, 11, e0153873 Search PubMed.
- E. Gilberg, S. Jasial, D. Stumpfe, D. Dimova and J. Bajorath, J. Med. Chem., 2016, 59, 10285–10290 CrossRef CAS PubMed.
- A. Kahraman, R. J. Morris, R. A. Laskowski and J. M. Thornton, J. Mol. Biol., 2007, 368, 283–301 CrossRef CAS PubMed.
- A. Kahraman, R. J. Morris, R. A. Laskowski, A. D. Favia and J. M. Thornton, Proteins, 2010, 78, 1120–1136 CrossRef CAS PubMed.
- S. Barelier, T. Sterling, M. J. O'Meara and B. K. Shoichet, ACS Chem. Biol., 2015, 10, 2772–2784 CrossRef CAS PubMed.
- D. Dimova, Y. Hu and J. Bajorath, J. Med. Chem., 2012, 55, 10220–10228 CrossRef CAS PubMed.
- A. B. Yongye and J. L. Medina-Franco, J. Chem. Inf. Model., 2012, 52, 2454–2461 CrossRef CAS PubMed.
- A. Gaulton, L. J. Bellis, A. P. Bento, J. Chambers, M. Davies, A. Hersey, Y. Light, S. McGlinchey, D. Michalovich, B. Al-Lazikani and J. P. Overington, Nucleic Acids Res., 2012, 40, D1100–D1107 CrossRef CAS PubMed.
- A. P. Bento, A. Gaulton, A. Hersey, L. J. Bellis, J. Chambers, M. Davies, F. A. Krüger, Y. Light, L. Mak, S. McGlinchey, M. Nowotka, G. Papadatos, R. Santos and J. P. Overington, Nucleic Acids Res., 2014, 42, D1083–D1090 CrossRef CAS PubMed.
- Y. Hu and J. Bajorath, J. Chem. Inf. Model., 2014, 54, 3056–3066 CrossRef CAS PubMed.
- Y. Wang, J. Xiao, T. O. Suzek, J. Zhang, J. Wang, Z. Zhou, L. Han, K. Karapetyan, S. Dracheva, B. A. Shoemaker, E. Bolton, A. Gindulyte and S. H. Bryant, Nucleic Acids Res., 2012, 40, D400–D412 CrossRef CAS PubMed.
- E. Griffen, A. G. Leach, G. R. Robb and D. J. Warner, J. Med. Chem., 2011, 54, 7739–7750 CrossRef CAS PubMed.
- J. Hussain and C. Rea, J. Chem. Inf. Model., 2010, 50, 339–348 CrossRef CAS PubMed.
- X. Hu, Y. Hu, M. Vogt, D. Stumpfe and J. Bajorath, J. Chem. Inf. Model., 2012, 52, 1138–1145 CrossRef CAS PubMed.
- OEChem TK, OpenEye Scientific Software, Inc., Sante Fe, NM, USA, 2012 Search PubMed.
- P. Shannon, A. Markiel, O. Ozier, N. S. Baliga, J. T. Wang, D. Ramage, N. Amin, B. Schwikowski and T. Ideker, Genome Res., 2003, 13, 2498–2504 CrossRef CAS PubMed.
- RDKit, Cheminformatics and Machine Learning Software, 2013, http://www.rdkit.org Search PubMed.
- T. Sterling and J. J. Irwin, J. Chem. Inf. Model., 2015, 55, 2324–2337 CrossRef CAS PubMed.
- J. J. Irwin, D. Duan, H. Torosyan, A. K. Doak, K. T. Ziebart, T. Sterling, G. Tumanian and B. K. Shoichet, J. Med. Chem., 2015, 58, 7076–7087 CrossRef CAS PubMed.
- Y. Hu and J. Bajorath, J. Chem. Inf. Model., 2010, 50, 2324–2337 Search PubMed.
- J. Mestres, E. Gregori-Puigjane, S. Valverde and R. V. Sole, Nat. Biotechnol., 2008, 26, 983–984 CrossRef CAS PubMed.
- UniProt consortium, Nucleic Acids Res., 2015, 43, D204–D212 CrossRef PubMed.
- http://https://zenodo.org/record/200393.
|
This journal is © The Royal Society of Chemistry 2017 |
Click here to see how this site uses Cookies. View our privacy policy here.