DOI:
10.1039/C6MT00202A
(Paper)
Metallomics, 2017,
9, 112-123
Defining the human copper proteome and analysis of its expression variation in cancers†
Received
17th September 2016
, Accepted 1st December 2016
First published on 1st December 2016
Abstract
Copper (Cu) is essential for living organisms, and acts as a cofactor in many metabolic enzymes. To avoid the toxicity of free Cu, organisms have specific transport systems that ‘chaperone’ the metal to targets. Cancer progression is associated with increased cellular Cu concentrations, whereby proliferative immortality, angiogenesis and metastasis are cancer hallmarks with defined requirements for Cu. The aim of this study is to gather all known Cu-binding proteins and reveal their putative involvement in cancers using the available database resources of RNA transcript levels. Using the http://Uniprot.org database along with manual curation, we identified a total of 54 Cu-binding proteins (named the human Cu proteome). Next, we retrieved RNA expression levels in cancer versus normal tissues from the TCGA database for the human Cu proteome in 18 cancer types, and noted an intricate pattern of up- and downregulation of the genes in different cancers. Hierarchical clustering in combination with bioinformatics and functional genomics analyses allowed for the prediction of cancer-related Cu-binding proteins; these were specifically inspected for the breast cancer data. Finally, for the Cu chaperone ATOX1, which is the only Cu-binding protein proposed to have transcription factor activities, we validated its predicted over-expression in patient breast cancer tissue at the protein level. This collection of Cu-binding proteins, with RNA expression patterns in different cancers, will serve as an excellent resource for mechanistic–molecular studies of Cu-dependent processes in cancer.
Significance to metallomics
Copper is an essential metal ion that facilitates many biological functions. This work adopts a global approach and first summarizes all known human copper-binding proteins and then investigates their putative importance in cancer using a recognized database of RNA expression information for an extended set of cancer types. The expanding functional repertoire of metalloproteins justifies further studies of metalloproteins in order to address non-traditional functions in cell signaling and diseases, such as cancer. Here, we provide new information that may be used by others to explore cancer mechanisms related to copper-ion dependence.
|
Introduction
Cu is essential to living organisms and acts as a cofactor in key enzymes, but the redox properties that allow the metal to impart activities to proteins also imply the potential for toxicity.1 To minimize the toxic effects and strictly regulate the temporal and spatial distributions of Cu, organisms have evolved elaborate systems for the uptake, intracellular transport, protein loading and storage of Cu. In human cells, after internalization of Cu by the Cu importer CTR1,2 Cu is distributed in the cytoplasm via at least three pathways:3 in the secretory path, the cytoplasmic Cu chaperone ATOX1 delivers Cu to the Cu-transporting P1B-type ATPases ATP7A and ATP7B, also called Wilson and Menkes disease proteins, in the trans-Golgi network. Once transferred to ATP7A/B, Cu is channeled to the lumen and, upon ATP hydrolysis, loaded onto many target Cu-dependent enzymes, e.g., ceruloplasmin (CP) and lysyl oxidase (LOX).4 CP constitutes the major Cu transporter in blood plasma, where it acts as a ferroxidase, oxidizing iron, and delivering Cu to other cells.5–8 LOX is also a secreted Cu-dependent enzyme and crosslinks extracellular matrix proteins. In addition to the secretory pathway, there are two other paths for Cu transport in the cytoplasm. In one, the Cu chaperone for superoxide dismutase 1 (CCS) directs Cu to cytoplasmic Cu/Zn superoxide dismutase 1 (SOD1); in the other, Cu is transported to mitochondria by COX17, and with help from additional proteins (i.e., SCOs and COX11), Cu is incorporated into cytochrome c oxidase (COX1 and COX2).
Since Cu is a key component in many essential enzymes,1,9,10 it is not surprising that Cu is required for at least three characteristic phenomena involved in cancer progression: proliferative immortality, angiogenesis, and metastasis.11,12 In support of increased Cu demand, cancer tissue and cancer patients' serum have increased Cu levels, whereas serum levels of other metals (i.e., iron, zinc) are often lower than normal.11 Cancer progression involves uncontrolled growth of cells, followed by cancer cell invasion, dissemination and secondary tumor formation at local and distant sites. For a tumor to grow larger than a few mm, angiogenesis is needed. Cu influences several molecular pathways that induce a pro-angiogenic response,13 including direct binding to, and promoting the expression of, angiogenic factors.14,15 Cu also influences the ability of cancerous cells to metastasize through activation of metabolic and proliferative enzymes.11 For example, LOX is secreted by cancer cells to create a pro-metastatic niche.16,17
Recent unprecedented findings suggest that ATOX1 has transcription factor activities connected to cancer progression.18 ATOX1 acts as a Cu-dependent transcription factor19 that promotes the expression of cyclin D1, a key protein involved in the cell cycle and cell proliferation,20 and the Cu-dependent enzyme SOD3, a major extracellular antioxidant protein and a protectant against hypertension.21,22 ATOX1 also promotes inflammatory neovascularization by acting as a Cu-dependent transcription factor for NADPH oxidase,23 and is essential for platelet-derived growth factor-induced Cu-dependent cell migration, thus potentially regulating malignant angiogenesis and vascular remodeling.24 In some analogy with ATOX1, CCS transports Cu to the nucleus and regulates the formation of the hypoxia-inducible factor 1 (HIF-1) transcriptional complex, which in turn promotes the expression of the vascular endothelial growth factor (VEGF) and, thereby, tumor growth.25 Our research group recently confirmed the presence of ATOX1 in the nucleus of mammalian cells, but found no binding to the proposed DNA promoter sequence in vitro.26 Nonetheless, from an extensive yeast two hybrid screening, using ATOX1 as a bait, several new human protein partners were detected:27 interestingly, among the confident hits, several proteins are related to cancer (e.g., CPEB4, DNMT1, and PPM1A that regulate RNA transcription, DNA methylation, and phosphorylation, respectively28–31).
To get a global view, we established here the human copper proteome (i.e., the collection of all identified human Cu-binding proteins) and then analyzed their RNA transcript level changes in different cancer tissues using information taken from the publicly-available TCGA database. The Cancer Genome Atlas, or TCGA, is a collaboration between the National Cancer Institute (NCI) and the National Human Genome Research Institute (NHGRI) that has generated comprehensive, multi-dimensional maps of genomic changes in many different cancers. It was recently updated to include RNA transcript levels for 25 different cancer types (GDC data portal accessed on 22 Aug 2016). Based on functional genomics and bioinformatics analysis of the extracted expression level data, we identified Cu-binding proteins that appear important in cancer and, finally, in a proof-of-principle experiment, we confirmed the upregulation of the ATOX1 protein in patient breast cancer tissue.
Methods
Data mining
Cu-binding proteins were identified using http://Uniprot.org, literature review, and manual curation. As a first screening, genes were extracted from http://Uniprot.org using the search terms ‘human + copper’. We then manually checked each gene in Uniprot and selected only the ones defined as Cu-binding or Cu-transport. Although not defined as Cu-binding in Uniprot, we added SPARC, MEMO1 and MAP2K1 as Cu-binding proteins, based on literature reports.32–34 This resulted in a total of 54 proteins that can be classified as Cu-binding. Subcellular localization of the 54 Cu-binding proteins was retrieved from the section ‘Localization’ in http://Genecards.org. Protein function was extracted from http://Uniprot.org and literature.
RNA-seq data analysis
The normalized gene expression quantification data for 54 copper-binding genes and 18 tumor types were downloaded from the GDC data portal. The values are upper quartile normalized FPKM count estimates for sequence reads mapping to the genes. The log
2-fold change (lfc = log
2(tumor/normal)) and p-values (t-test) were calculated from the normalized expression data for each gene and tumor type. To visualize the similarity in the gene expression patterns, a heat map was created with R version 3.3.0 (http://www.r-project.org) using the heat map.2 function in R's ‘gplot’ package (version 3.0.1). The genes and tumors were classified based on the Pearson correlation coefficients as a similarity measure in gene expression and Ward2's hierarchical clustering method. The classifications for interpretation of the strengths of the Pearson's correlations were implemented according to Cohen.35 The proteo-genomics heat map in Fig. 4 is based on data presented by Mertins et al.36 following PAM50-defined molecular subtypes.37
Cluster and GO (gene ontology) term enrichment analysis
The Cytoscape software (http://www.cytoscape.org)38 was used together with GeneMania App39 to generate interaction networks for all clusters of Cu-binding proteins identified in the heat map. The global parameters were: (i) data source, selected considering the known co-expression, and physical (protein–protein) and genetic interactions; (ii) maximum resultant genes, set to double the size of each cluster; and (iii) an automatic weighting method to assign values to each node. Genes in query get the maximum node size in the Cytoscape graph, whereby the size of a related gene is inversely proportional to the rank of that gene in the list, sorted using the score assigned by GeneMANIA. In a separate (top-down) approach, we analyzed the overall GO term enrichment by calculation of the weighted average log
2-fold change of the expression of all genes in cancer, with a cut-off value of 0.6, followed by ranking of the genes by the absolute value of their log
2-fold change (i.e., over- and underrepresented genes above the cut-off taken together). Then, we used this ranked and non-redundant list of gene identifiers as input for the GO enrichment analysis tool Gorilla (http://cbl-gorilla.cs.technion.ac.il/),40 with the p-value threshold set to 10−3 and retrieving the most represented biological processes. The resulting table, ranked by the FDR q-value (i.e., a correction of the above p-value using the Benjamini and Hochberg method), was fed to the ReviGO platform (http://revigo.irb.hr/)41 to visualize a semantic similarity-based scatterplot. The circles' color represents the log
10 p-value.
Patient material
The breast cancer material consists of tissue microarrays (TMAs) of biopsies originating from a cohort of post-menopausal breast cancer patients enrolled in a randomized clinical trial between 1976 and 1990. Briefly, breast cancer patients with a tumor diameter ≤30 mm and no lymph node involvement were included. The patients were randomized to receive tamoxifen for two years or no endocrine treatment. Nottingham histological grade and immunohistochemical staining of ER, PR and HER2 were performed retrospectively according to standardized methods used in clinical routine.42 Retrospective studies of biomarkers were approved by the regional ethical review board at the Karolinska Institute, Stockholm, Sweden (KI 97-451, with amendment 030201). The four molecular breast cancer subtypes were defined as follows: luminal A, ER+/PR+/HER2−/low mitotic count; luminal B, ER+/(high mitotic count and/or PR− and/or HER2+); HER2-subtype (ER−/HER2+); and triple negative breast cancer (TNBC; ER−, PR− and HER2−).
Immunohistochemical (IHC) staining of ATOX1 in breast tissue
The paraffin embedded sections were incubated at 65 °C for 2 h, followed by deparaffinization in Aqua de Par 10X Ancillary reagent (Biocare Medical, Concord California) for 20 min. The sections were then subjected to treatment with Borg Decloaker RTU antigen retrieval solution (Biocare Medical, Concord California) for heat induced epitope retrieval (HIER) using a Decloaking Chamber NxGen pressure cooker (Biocare Medical, Concord California) programed in a temperature cycle to reach a maximum of 110 °C for 5 min. After allowing cooling down, the slides were rinsed in tap water prior to washing in TBS (Tris-buffered saline). After blocking endogenous peroxidase by incubation in Peroxidazed 1 (Biocare Medical, Concord California) for 5 min, the slides were washed in TBS and incubated with the mouse monoclonal anti-ATOX1 primary antibody (ab54865, Abcam) for 30 min at room temperature (RT) followed by incubation with the secondary antibody MACH4 Universal HRP (horseradish peroxidase)-Probe and the tertiary antibody MACH4 Universal HRP-Polymer (Biocare Medical, Concord California) for 10 min each at RT. After HRP detection, the tissue section was rinsed in TBS. For visualization, one drop (32 μl) of DAB chromogen per 1.0 ml of DAB substrate buffer (Biocare Medical, Concord California) was applied to the tissue sections followed by incubation for 5 min at RT. After rinsing in deionized water, the sections were counterstained with Mayer's haematoxylin staining solution (Histolab Products AB, Göteborg Sweden) and mounted with Pertex mounting media (Histolab Products AB, Göteborg Sweden). The immunostaining was scored by two independent observers based on the intensity in the epithelial cells without knowledge of clinicopathological and biological information. The staining intensity was scored according to the following criteria: negative (no brown), weak (light brown), moderate (brown), and strong (dark brown) staining. In total, 67 breast cancer tissue samples were reliably analyzed along with four normal breast tissue samples. (Due to the limited sample number, a statistical comparison between the scoring data for the subtypes and the control tissue, calculated using Fisher's exact test, resulted in no statistical significance.) Notably, matching samples of normal and cancerous breast tissue from the same patient are desired to obtain higher statistical power (not available for this dataset).
Results and discussion
I. The human Cu proteome
To gather all human proteins known to bind Cu, we used http://Uniprot.org as a source and identified all proteins classified as Cu binding or Cu transporting (Table S1, ESI† with proposed functions of each protein, if reported). To the list, we added SPARC, MEMO1 and MAP2K1 as Cu-binding proteins, based on literature reports,32–34 although they are not yet described as such in UniProt. Next, we used stringent criteria in http://Genecards.org with assignment by both UniProtKB and COMPARTMENTS (confidence levels 4 or 5) to separate the proteins according to their subcellular localizations. Subcellular localizations for 46 of the 54 identified Cu-binding proteins were indicated following these stringent criteria. ATOX1, MEMO1, MT3, LTF and S100A5 were localized according to less stringent criteria with assignment by COMPARTMENTS (confidence level 4 or 5) only, and CUTA and MT4 according to even less stringent criteria with assignment by COMPARTMENTS (confidence level 2). MOXD2P was the only Cu protein that remained unassigned. As MOXD2P is a pseudogene of MOXD1, we speculated that it occupies the same subcellular site as MOXD1, i.e., the endoplasmic reticulum (Fig. 1 and Table S1, ESI†).
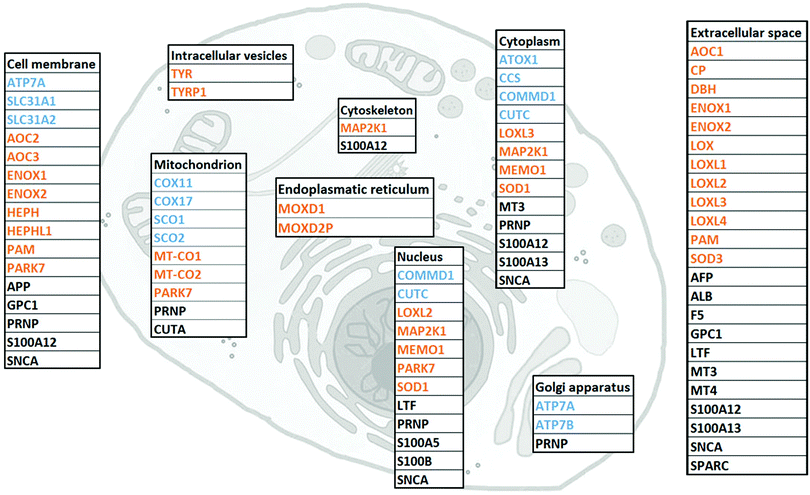 |
| Fig. 1 Cellular localization of the 54 identified Cu-binding proteins. Subcellular localizations for 46 of the 54 copper-binding proteins are indicated following the stringent criteria in http://Genecards.org with assignment by both UniProtKB and COMPARTMENTS (confidence levels 4 or 5). ATOX1, MEMO1, MT3, LTF and S100A5 were localized according to the less stringent criteria with assignment by COMPARTMENTS (confidence level 4 or 5) only, and CUTA and MT4 according to even less stringent criteria with assignment by COMPARTMENTS (confidence level 2). MOXD2P was the only Cu protein that remained unassigned. As MOXD2P is a pseudogene of MOXD1, we speculated that it occupies the same subcellular site as MOXD1, i.e., the endoplasmic reticulum. Colors indicate a protein's function, with blue for the transporter; orange for the enzyme; and black for the protein with other or unknown function. | |
The list of 54 Cu-binding proteins (Fig. 1 and Table S1, ESI†) corresponds to less than 0.5% of the whole proteome (which consists of at least 10
000 different proteins). This fraction is expected based on earlier estimates using a bioinformatics approach.43 There are two important points: first, there may be more proteins to be discovered as Cu-binding proteins. Thus, the list will grow with time. Second, some of the proteins defined as Cu-binding proteins may not use Cu for their function. For example, the three amyloidogenic proteins SNCA (α-synuclein), APP (amyloid β precursor protein) and PRNP (prion protein) are reported to bind Cu, but neither the role of Cu nor the exact functions are determined for these proteins.44–47
We found that human cells have 12 proteins classified as Cu transporters. For import there are SLC31A1 and SLC31A2 (CTR1 and CTR2), for cytoplasmic transport there are CCS for Cu delivery to SOD1, ATOX1 for Cu delivery to ATP7A/B in the Golgi, and then COX11, COX17, SCO1, and SCO2 that provide Cu to COX1 and COX2 in the mitochondria. COMMD1 is an enigmatic protein that may be involved in the regulation of exocytosis of Cu-loaded vesicles,48,49 perhaps via modulation of ATP7B protein stability.50 Whether CUTC is truly a Cu transporter, as reported here, or a Cu-dependent enzyme instead, is an open question.51
We observed that about half of the identified Cu-binding proteins are Cu-dependent enzymes (Fig. 1 and Table S1, ESI†). Many are found extracellular and in the plasma membrane, but also in all (but Golgi) intracellular compartments. In addition to many well-characterized Cu-dependent enzymes, PARK7 is classified as a Cu-dependent enzyme. It is a peptidase that may also function as a sensor for oxidative stress and, notably, mutations in this gene are the cause of early-onset Parkinson's disease.52,53 In addition to Cu homeostasis and enzymatic activity, we functionally classified the remaining 15 proteins in the Cu proteome as ‘other’ which corresponds to either non-enzymatic or unknown functions (Table S1, ESI†). Interesting proteins in this group are four S100 proteins, typically EF-hand calcium-binding proteins, which have Cu-binding sites and Cu-dependent functions.54 Notably, none (but ATOX1, see Introduction) of the identified Cu-binding proteins are transcription factors.
In addition to LOX (collagen cross-linking) and ATOX1/CCS (putative transcription factor roles), only a few other proteins in the Cu proteome have yet been shown to play roles in cancer. MAP2K1 (MEK1) is a kinase involved in the mitogen-activated protein kinase signaling pathway, and directly related to tumor growth,18 invasion and metastasis.55,56 MEMO1 is a Cu-dependent redox enzyme that facilitates tumor cell migration and in vivo metastasis57via, for example, production of reactive oxygen species, regulation of transcriptional pathways related to the epithelial to mesenchymal transition,58 and interaction with the RhoA–mDia1 signaling complex.59 SPARC, a collagen-binding glycoprotein, plays a role in tumor invasion and metastasis via modulation of cell–cell and cell–matrix interactions.32,33 Interestingly, in certain types of cancer, SPARC is associated with highly aggressive tumor phenotypes, whilst in others SPARC may function as a tumor suppressor.60 In addition to extracellular mechanisms already mentioned, LOX also regulates cancer progression within cells, here via actin polymerization promoting migratory phenotypes.61 Whereas LOX obtains Cu from the secretory pathway (i.e., ATOX1–ATP7A), it is not known how SPARC, MEMO1 and MEK1 are loaded with Cu.
II. RNA transcript levels of Cu-binding proteins in cancer
To derive if the assigned Cu-binding proteins are involved in cancer, which subsequently may be used to elucidate new (druggable) pathways, we used the TCGA data (http://cancergenome.nih.gov/) to extract RNA transcript levels of the Cu proteome. Of the data for 25 different cancers in TCGA, 18 contained information on all the 54 Cu-binding proteins and, thus, these 18 cancer types were used in the analysis. In each case, the RNA transcript level in cancer tissues was compared to the RNA transcript level in matching normal tissues. In Fig. 2, we show the resulting heat map with log
2-fold changes for the 54 proteins (on the x axis) in 18 cancer types (on the y axis) (red, upregulation; blue, downregulation; and white, no change). In this plot, genes placed close to each other on the y axis have more comparative transcript levels than genes far away in the list, in the different cancer types.
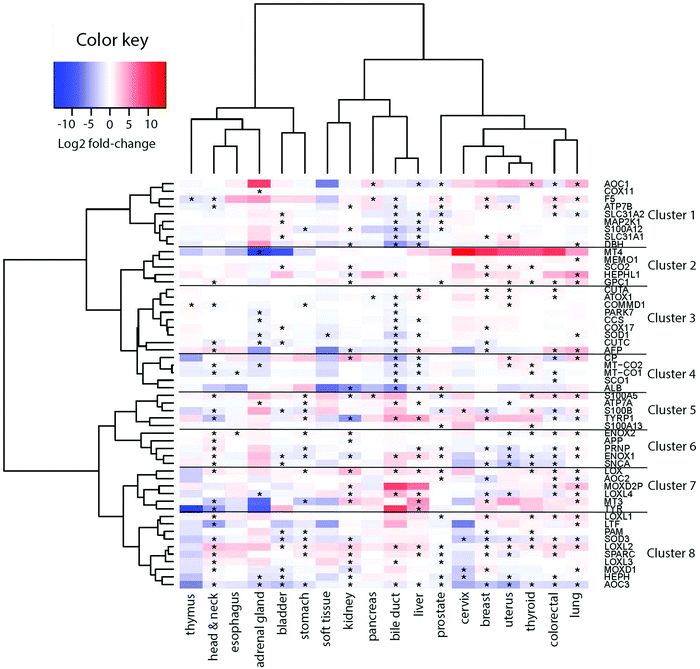 |
| Fig. 2 Heat map of expression patterns for the human Cu proteome in cancers. Expression patterns of 54 copper-binding genes across 18 tumor types are given. The color scale indicates the degree of change in expression (log 2-fold change), with blue for downregulation and red for upregulation. Genes showing a log 2-fold change of at least 0.4 and a p-value below 0.05 were considered to be significant and are marked with an asterisk (*). Both the row and column dendrograms indicate unsupervised hierarchical clustering of 54 copper-binding genes and 18 cancer types, respectively. The data were classified using the Ward2's hierarchical clustering method with a dissimilarity matrix based on the Pearson correlation as the distance metric. | |
Visual inspection revealed that many of the proteins in the human Cu proteome are up- or downregulated in the different cancers in nontrivial patterns. For example, LOX, LOXL1-2, SPARC and ENOX2 are upregulated, and S100B, PRNP, ENOX1, SNCA, SOD3, HEPHL1 and AOC3 are downregulated in many cancers (≥6 out of 18). With respect to ATOX1, it is upregulated in breast, colorectal, uterus and liver tumors, but downregulated in bile duct and pancreatic tumors (Fig. S1, ESI†).
III. Clustering of Cu-binding proteins
Next, building on the hierarchical clustering of the RNA expression data we divided the Cu-binding proteins on the y-axis, in Fig. 2, into 8 clusters, with each cluster containing the genes with the most similar expression patterns throughout the different cancers. Cluster 1 ranges from AOC1 to DBH, cluster 2 from MT4 to GPC1, cluster 3 from CUTA to AFP, cluster 4 from CP to ALB, cluster 5 from S100A5 to S100A13, cluster 6 from ENOX2 to SNCA, cluster 7 from LOX to TYR, and cluster 8 from LOXL1 to AOC3 (Fig. 2). Also the 18 tumor types can be divided into 3 groups based on the hierarchical clustering results. Group 1 contains thymus, head and neck, esophagus, adrenal gland, bladder, and stomach cancers; group 2 contains soft tissue, kidney, pancreas, bile duct and liver cancers; and group 3 contains prostate, cervix, breast, uterus, thyroid, colorectal, and lung cancers (Fig. 2).
To reveal the functional aspects of the eight clusters of Cu-binding proteins derived from cancer expression patterns, the clusters were analyzed with the GeneMANIA tool using the Cytoscape software. This tool combines the available bioinformatics information regarding the co-expression pattern, and physical and genetic interactions of the genes within each cluster, including here one extra level of interaction partners, to derive what is known about functional relations between the genes. Based on the distance between the gene representations and the number of interactions between the genes within each cluster plot, we detected that the genes in clusters 1, 3 and 8 are closely related in terms of known functional parameters (many interactions and short distances between the gene representations), whereas those in clusters 2 and 4 seem to be more distant to each other with regard to known functions (few interactions and genes spread apart). In addition, Cu-binding proteins assigned to one cluster based on the heat map (blue/red/black circles) sometimes appear as partner proteins (grey circles) in other clusters (Fig. 3). This observation suggests that the clusters have a high level of inter-connectivity.
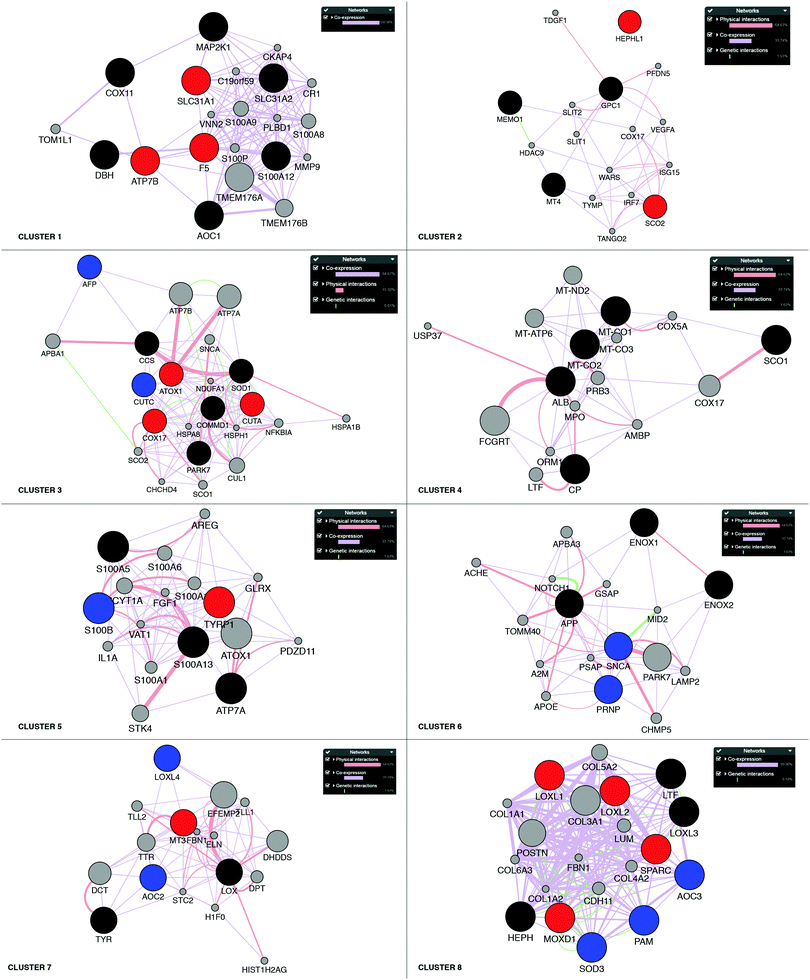 |
| Fig. 3 Network analysis of the eight clusters of Cu-binding protein genes. Networks of interactions for each of the gene clusters (red, blue and black circles) identified in the RNA expression heat map shown in Fig. 2. Red circles denote upregulation in breast cancer; blue circles, downregulation in breast cancer; and black circles, no change in breast cancer. Each network shows the first-level partners (grey circles) in physical interactions (red connectors), co-expression correlation (purple connectors) and genetic interactions (green connectors) for each gene in a cluster. The close proximity of the genes in the network indicates a high level of interaction. | |
IV. Exploration of breast cancer RNA transcript data
To further analyze the roles of the Cu-binding proteins, we focused on the RNA transcript data for one cancer: breast cancer. For the breast cancer TCGA data, we observed the upregulation of F5, ATP7B and SLC31A1 in cluster 1, SCO2 and HEPHL1 in cluster 2, CUTA, ATOX1, and COX17 in cluster 3, TYRP1 in cluster 5, MT3 in cluster 7, and LOXL1-2, SPARC and MOXD1 in cluster 8 (red circles in Fig. 3). Because several Cu-binding proteins are upregulated in breast cancer (corresponding to 26% of the Cu proteome proteins), clearly, Cu-dependent processes are of importance for breast cancer development. Moreover, we found downregulated CUTC and AFP in cluster 3, S100B in cluster 5, PRNP and SNCA in cluster 6, AOC2 and LOXL4 in cluster 7, and PAM, SOD3 and AOC3 in cluster 8 in breast cancer (blue circles in Fig. 3). One may imagine that even if cancer cells have a higher demand for Cu than normal cells (which should correlate with increased Cu uptake and/or decreased Cu export), some Cu-binding proteins must likely be downregulated in order to facilitate increased Cu loading of upregulated (and thereby, cancer-promoting) Cu-binding proteins.
Of the upregulated Cu-binding proteins in breast cancer, five were Cu transporters: SLC31A1, ATOX1, ATP7B, COX17 and SCO2. This suggests that there is an increased Cu flow via SLC31A1-mediated cell uptake followed by loading onto Cu-dependent enzymes via the ATOX1–ATP7B path, and delivery of Cu to mitochondria via COX17 and SCO2 in breast cancer. Notably, the COX1 and COX2 genes, normally obtaining Cu via COX17/COX11/SCO1/SCO2 were not upregulated in the breast cancer data. This may hint at other purposes of increased Cu delivery to mitochondria. For example, Cu import into mitochondria can regulate the mitochondrial redox status as reversible changes in the redox state of cysteines in Cu-transport proteins occur in parallel with Cu transfer.62,63 Five Cu-binding enzymes were upregulated in breast cancer, namely, HEPHL1, TYRP1, LOXL1, LOXL2, and MOXD1. Since ATP7B, but not ATP7A, was upregulated in breast cancer, it appears that these enzymes are loaded with Cu via ATP7B under these conditions.
The expression of the CUTA gene is also upregulated in breast cancer and found in the same gene cluster as ATOX1 and COX17 (cluster 3). Since CUTA is predicted to be a mitochondrial protein (Fig. 1), COX17 may be the chaperone that delivers Cu to CUTA in mitochondria. However, in the functional network analysis in Fig. 3, the nearest neighbors of CUTA are COMMD1 and SOD1, both of which are cytoplasmic proteins, posing the question in which compartment is CUTA really present. Like LOX1 and LOX2, SPARC is an extracellular protein upregulated in breast cancer that is found in cluster 8. Therefore, we speculate that SPARC, like LOX1 and LOX2, is loaded with Cu via the secretory pathway, i.e., through the ATOX1–ATP7A/B path, where ATP7B is the likely ATPase as is upregulated in breast cancer.
To test these hypotheses, molecular studies investigating the roles of COX17 and/or ATOX1 in providing Cu to CUTA, CUTA localization, and the ATOX1–ATP7B path for loading Cu onto SPARC are desired and, importantly, should be assessed as a function of breast cancer molecular subtype. Similar analyses of upregulated RNA transcripts of Cu-binding proteins in each of the remaining 17 cancer types will likely result in a number of unprecedented predictions of Cu-dependent processes important in different cancer types.
V. ATOX1 protein upregulation in breast cancer tissues
We note that changes in RNA transcript levels not always reflect the corresponding changes in protein levels as many Cu-binding proteins may be regulated at the translational and/or post-translational level. To address this possible caveat, we turned to the recently-reported proteogenomics data36 on four PAM50-defined intrinsic breast cancer subtypes (29 luminal A, 33 luminal B, 18 HER2 (ERBB2)-enriched, and 25 basal-like) that contained RNA and protein data for 36 of the here identified 54 Cu-binding proteins. Analysis of the reported information revealed that 28 of the 36 Cu-binding proteins (78%) have high-to-moderate positive correlations between RNA transcript and protein levels (Fig. 4). ATOX1 is one of the proteins with the highest positive correlation (r = 0.67) indicating that if the RNA transcript level is up, protein amount is up too. Thus, as a first approximation, RNA data may be used as a measure of protein levels in this set of genes. Nonetheless, proteomic studies are always desired to support RNA transcript analysis because of the sensitivity of high-resolution accurate-mass tandem mass spectrometry (MS/MS) for determining protein levels.36
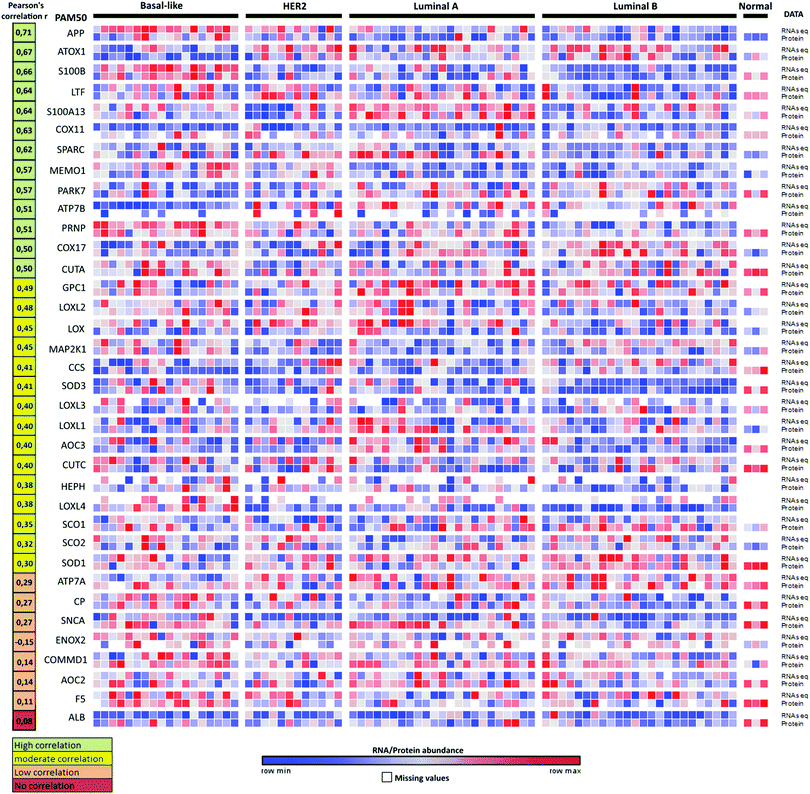 |
| Fig. 4 Proteogenomic data for breast cancer subtypes. Heat map of correlations between protein (high-resolution accurate-mass tandem mass spectrometry, MS/MS) and RNA-seq data for 36 Cu-binding proteins in breast cancer samples36 clustered according to the PAM50-defined subtypes (labeled basal-like, HER2, luminal A and luminal B). Correlations between the protein and transcript levels were calculated using the Pearson correlation coefficient, r (from ref. 35). The genes were ordered according to r (shown in the left column) from high (top) to low (bottom) correlation. RNA/protein abundance is depicted in a color scale from blue (low) to red (high) scaled within each row according to ref. 36. | |
As a first step towards protein expression analysis, we focused on ATOX1 because of its proposed transcription factor role.26,27,64 Using immunohistochemistry we investigated the amount and distribution of ATOX1 protein in 67 breast cancer sections in tissue microarrays (TMAs). In agreement with the RNA transcript data from TCGA (i.e., upregulation of ATOX1 in breast cancer; Fig. 2), we found ATOX1 protein levels to appear higher in cancerous than in normal breast tissues (examples in Fig. 5 and Fig. S2, ESI†). This observation parallels the data from the Human Protein Atlas that report ATOX1 protein in cancerous but not in normal breast tissues (http://www.proteinatlas.org; version 15, last accessed on 17 November 2016). ATOX1 scoring data for the 67 breast cancer samples (consisting of negative, weak and moderate ATOX1 staining intensities) were categorized into four molecular subtypes (i.e., luminal A, luminal B, HER2, and triple negative) (Fig. 6). Inspection reveals that the highest ATOX1 intensities were found in all cancer subtypes but HER2 and, interestingly, the least proliferative subtype (luminal A) was the one with the highest frequency of ATOX1 negatives. In order to draw molecular conclusions, additional samples are needed; more quantitative detection methods must be used (e.g., MS/MS, Western blot) and matching normal and cancer tissue samples from the same patient are desired. With respect to cellular distribution, ATOX1 was localized in both the cytoplasm and the nucleus, with apparent increased nuclear localization in tissue samples with the highest ATOX1 levels. This protein distribution parallels the reported data from cell line studies and emphasizes the prospect of ATOX1 activities in the nucleus.26,27,64 In future studies, we will investigate the functional roles of ATOX1 in established breast cancer cell lines (work in progress).
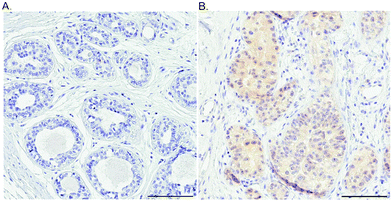 |
| Fig. 5 Probing the ATOX1 protein in normal and breast cancer tissues. An illustration of the IHC staining of ATOX1 protein in benign (A) and cancerous (B) ductal breast tissue. Scale bars = 100 μm. | |
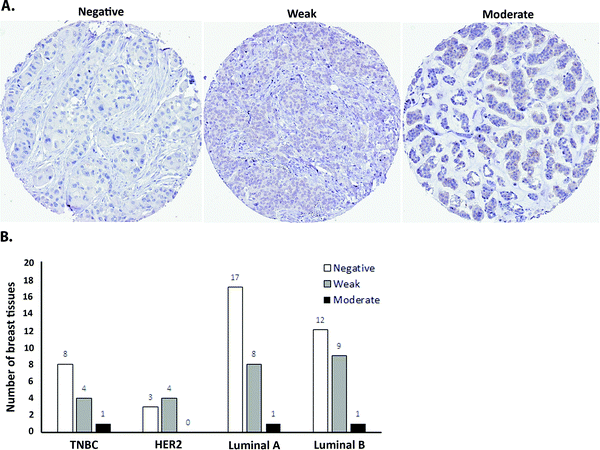 |
| Fig. 6 ATOX1 expression in breast cancer tissue of different molecular subtypes. (A) Legend for the manual scoring of ATOX1 expression levels (i.e., staining intensity) in microarrays of breast cancer tissue sections. (B) Scoring results for ATOX1 expression levels in cancerous breast tissues (67 samples) of the following molecular subtypes: triple negative (TNBC), HER2, luminal A, and luminal B. | |
Conclusions
Our study brings together all known Cu-binding proteins into, what we term, the Cu proteome, followed by extraction of their expression levels in different cancers using RNA transcript data available in the TCGA database. The hierarchical clustering of this data collection (shown as a heat map in Fig. 2) constitutes an excellent resource for researchers aiming to test new hypotheses around cancer progression. Cu is a necessary component of most cancer cells,11,12 but the possible participation of Cu-binding proteins in various steps of cancer is an underexplored topic. For the breast cancer data, we performed an initial inspection of Cu-binding proteins that are upregulated at the RNA transcript level, and thereby we proposed mechanisms by which SPARC and CUTA obtain Cu. We also compared the RNA transcript and protein levels for most Cu-binding proteins as a function of molecular subtypes of breast cancer (Fig. 4). Mechanistic studies using a combination of animal, cell culture and biophysical experiments are desired to test the hypotheses based on the presented proteogenomic data.
As a top-down approach, we analyzed the expression patterns of all human genes in cancer versus normal tissues from the TCGA database using the ReviGO software and the GOseq tool, Gorilla. This procedure condenses the extensive and complicated information provided by expression patterns of individual genes into an enrichment graph of biological attributes (GO terms) that can be visualized (Fig. S3, ESI†). Notably, only a few overrepresented GO terms in the plot include Cu-binding proteins. AOC3, MEMO1, LTF and SPARC are the four Cu-binding proteins found in the plot, and they are connected to the following enriched GO terms: response to metal ion (AOC3), cell division (SPARC), ERBB2–ERBB3 signaling (MEMO1), regeneration (AOC3), catabolism (AOC3) and regulation of cell proliferation (LTF). With more studies assessing the roles of Cu-binding proteins in cancer, the database of information will expand, and we predict that GO terms related to Cu-dependence will become of increased significance in the future.
List of abbreviations
Cu | Copper |
RNA-seq | RNA sequencing |
HIF-1 | Hypoxia-inducible factor 1 |
NBIS | National Bioinformatics Infrastructure Sweden |
NCI | National Cancer Institute |
NHGRI | National Human Genome Research Institute |
TCGA | The Cancer Genome Atlas |
VEGF | Vascular endothelial growth factor |
MS/MS | High-resolution accurate-mass tandem mass spectrometry |
GO | Gene ontology |
Acknowledgements
Juan Carlos Fierro-González is supported by grants from the Swedish Society for Medical Research, Folke and Marianne Edler Research Fund and Lars Hierta Memorial Foundation. Pernilla Wittung-Stafshede is supported by Knut and Alice Wallenberg Foundation, the Swedish Research Council, and Chalmers Foundation. Support from NBIS (National Bioinformatics Infrastructure Sweden) is gratefully acknowledged.
References
- A. Grubman and A. R. White, Copper as a key regulator of cell signalling pathways, Expert Rev. Mol. Med., 2014, 16, e11 CrossRef PubMed.
- H. Ohrvik and D. J. Thiele, How copper traverses cellular membranes through the mammalian copper transporter 1, Ctr1, Ann. N. Y. Acad. Sci., 2014, 1314, 32–41 CrossRef CAS PubMed.
- S. Puig and D. J. Thiele, Molecular mechanisms of copper uptake and distribution, Curr. Opin. Chem. Biol., 2002, 6, 171–180 CrossRef CAS PubMed.
- K. A. Koch, M. M. Pena and D. J. Thiele, Copper-binding motifs in catalysis, transport, detoxification and signaling, Chem. Biol., 1997, 4, 549–560 CrossRef CAS PubMed.
- E. D. Harris and S. S. Percival, Copper transport: insights into a ceruloplasmin-based delivery system, Adv. Exp. Med. Biol., 1989, 258, 95–102 CAS.
- M. C. Linder, J. R. Moor and K. Wright, Ceruloplasmin assays in diagnosis and treatment of human lung, breast, and gastrointestinal cancers, J. Natl. Cancer Inst., 1981, 67, 263–275 CAS.
- M. C. Linder, R. R. Bryant, S. Lim, L. E. Scott and J. E. Moor, Ceruloplasmin elevation and synthesis in rats with transplantable tumors, Enzyme, 1979, 24, 85–95 CAS.
- C. H. Campbell, R. Brown and M. C. Linder, Circulating ceruloplasmin is an important source of copper for normal and malignant animal cells, Biochim. Biophys. Acta, 1981, 678, 27–38 CrossRef CAS.
- M. Matson Dzebo, C. Arioz and P. Wittung-Stafshede, Extended functional repertoire for human copper chaperones, Biomol. Concepts, 2016, 7, 29–39 CAS.
- M. L. Turski and D. J. Thiele, New roles for copper metabolism in cell proliferation, signaling, and disease, J. Biol. Chem., 2009, 284, 717–721 CrossRef CAS PubMed.
- D. Denoyer, S. Masaldan, S. La Fontaine and M. A. Cater, Targeting copper in cancer therapy: ‘Copper That Cancer’, Metallomics, 2015, 7, 1459–1476 RSC.
- D. Hanahan and R. A. Weinberg, Hallmarks of cancer: the next generation, Cell, 2011, 144, 646–674 CrossRef CAS PubMed.
- D. C. Rigiracciolo, A. Scarpelli, R. Lappano, A. Pisano, M. F. Santolla, P. De Marco, F. Cirillo, A. R. Cappello, V. Dolce, A. Belfiore, M. Maggiolini and E. M. De Francesco, Copper activates HIF-1alpha/GPER/VEGF signalling in cancer cells, Oncotarget, 2015, 6, 34158–34177 Search PubMed.
- Q. Pan, C. G. Kleer, K. L. van Golen, J. Irani, K. M. Bottema, C. Bias, M. De Carvalho, E. A. Mesri, D. M. Robins, R. D. Dick, G. J. Brewer and S. D. Merajver, Copper deficiency induced by tetrathiomolybdate suppresses tumor growth and angiogenesis, Cancer Res., 2002, 62, 4854–4859 CAS.
- N. S. Kenneth, G. E. Hucks, Jr., A. J. Kocab, A. L. McCollom and C. S. Duckett, Copper is a potent inhibitor of both the canonical and non-canonical NFkappaB pathways, Cell Cycle, 2014, 13, 1006–1014 CrossRef CAS PubMed.
- J. T. Erler, K. L. Bennewith, T. R. Cox, G. Lang, D. Bird, A. Koong, Q. T. Le and A. J. Giaccia, Hypoxia-induced lysyl oxidase is a critical mediator of bone marrow cell recruitment to form the premetastatic niche, Cancer Cell, 2009, 15, 35–44 CrossRef CAS PubMed.
- Siddikuzzaman, V. M. Grace and C. Guruvayoorappan, Lysyl oxidase: a potential target for cancer therapy, Inflammopharmacology, 2011, 19, 117–129 CrossRef CAS PubMed.
- D. C. Brady, M. S. Crowe, M. L. Turski, G. A. Hobbs, X. Yao, A. Chaikuad, S. Knapp, K. Xiao, S. L. Campbell, D. J. Thiele and C. M. Counter, Copper is required for
oncogenic BRAF signalling and tumorigenesis, Nature, 2014, 509, 492–496 CrossRef CAS PubMed.
- S. Itoh, H. W. Kim, O. Nakagawa, K. Ozumi, S. M. Lessner, H. Aoki, K. Akram, R. D. McKinney, M. Ushio-Fukai and T. Fukai, Novel role of antioxidant-1 (Atox1) as a copper-dependent transcription factor involved in cell proliferation, J. Biol. Chem., 2008, 283, 9157–9167 CrossRef CAS PubMed.
- E. A. Klein and R. K. Assoian, Transcriptional regulation of the cyclin D1 gene at a glance, J. Cell Sci., 2008, 121, 3853–3857 CrossRef CAS PubMed.
- S. Itoh, K. Ozumi, H. W. Kim, O. Nakagawa, R. D. McKinney, R. J. Folz, I. N. Zelko, M. Ushio-Fukai and T. Fukai, Novel mechanism for regulation of extracellular SOD transcription and activity by copper: role of antioxidant-1, Free Radical Biol. Med., 2009, 46, 95–104 CrossRef CAS PubMed.
- K. Ozumi, V. Sudhahar, H. W. Kim, G. F. Chen, T. Kohno, L. Finney, S. Vogt, R. D. McKinney, M. Ushio-Fukai and T. Fukai, Role of copper transport protein antioxidant 1 in angiotensin II-induced hypertension: a key regulator of extracellular superoxide dismutase, Hypertension, 2012, 60, 476–486 CrossRef CAS PubMed.
- G. F. Chen, V. Sudhahar, S. W. Youn, A. Das, J. Cho, T. Kamiya, N. Urao, R. D. McKinney, B. Surenkhuu, T. Hamakubo, H. Iwanari, S. Li, J. W. Christman, S. Shantikumar, G. D. Angelini, C. Emanueli, M. Ushio-Fukai and T. Fukai, Copper Transport Protein Antioxidant-1 Promotes Inflammatory Neovascularization via Chaperone and Transcription Factor Function, Sci. Rep., 2015, 5, 14780 CrossRef CAS PubMed.
- T. Kohno, N. Urao, T. Ashino, V. Sudhahar, R. D. McKinney, T. Hamakubo, H. Iwanari, M. Ushio-Fukai and T. Fukai, Novel role of copper transport protein antioxidant-1 in neointimal formation after vascular injury, Arterioscler., Thromb., Vasc. Biol., 2013, 33, 805–813 CrossRef CAS PubMed.
- L. Qiu, X. Ding, Z. Zhang and Y. J. Kang, Copper is required for cobalt-induced transcriptional activity of hypoxia-inducible factor-1, J. Pharmacol. Exp. Ther., 2012, 342, 561–567 CrossRef CAS PubMed.
- D. Kahra, T. Mondol, M. S. Niemiec and P. Wittung-Stafshede, Human Copper Chaperone Atox1 Translocates to the Nucleus but does not Bind DNA in Vitro, Protein Pept. Lett., 2015, 22, 532–538 CrossRef CAS PubMed.
- H. Ohrvik and P. Wittung-Stafshede, Identification of New Potential Interaction Partners for Human Cytoplasmic Copper Chaperone Atox1: Roles in Gene Regulation?, Int. J. Mol. Sci., 2015, 16, 16728–16739 CrossRef PubMed.
- E. Ortiz-Zapater, D. Pineda, N. Martinez-Bosch, G. Fernandez-Miranda, M. Iglesias, F. Alameda, M. Moreno, C. Eliscovich, E. Eyras, F. X. Real, R. Mendez and P. Navarro, Key contribution of CPEB4-mediated translational control to cancer progression, Nat. Med., 2012, 18, 83–90 CrossRef CAS PubMed.
- I. Callebaut, J. C. Courvalin and J. P. Mornon, The BAH (bromo-adjacent homology) domain: a link between DNA methylation, replication and transcriptional regulation, FEBS Lett., 1999, 446, 189–193 CrossRef CAS PubMed.
- A. K. Das, N. R. Helps, P. T. Cohen and D. Barford, Crystal structure of the protein serine/threonine phosphatase 2C at 2.0 A resolution, EMBO J., 1996, 15, 6798–6809 CAS.
- X. Lin, X. Duan, Y. Y. Liang, Y. Su, K. H. Wrighton, J. Long, M. Hu, C. M. Davis, J. Wang, F. C. Brunicardi, Y. Shi, Y. G. Chen, A. Meng and X. H. Feng, PPM1A functions as a Smad phosphatase to terminate TGFbeta signaling, Cell, 2006, 125, 915–928 CrossRef CAS PubMed.
- S. A. Arnold and R. A. Brekken, SPARC: a matricellular regulator of tumorigenesis, J. Cell Commun. Signaling, 2009, 3, 255–273 CrossRef PubMed.
- G. P. Nagaraju, R. Dontula, B. F. El-Rayes and S. S. Lakka, Molecular mechanisms underlying the divergent roles of SPARC in human carcinogenesis, Carcinogenesis, 2014, 35, 967–973 CrossRef CAS PubMed.
- M. S. Weaver, G. Workman and E. H. Sage, The copper binding domain of SPARC mediates cell survival in vitro via interaction with integrin beta1 and activation of integrin-linked kinase, J. Biol. Chem., 2008, 283, 22826–22837 CrossRef CAS PubMed.
-
L. Cohen, in Life events and psychological functioning: theoretical and methodological issues, ed. C. LH, Sage, Newsbury Park, CA, 1988, pp. 11–30 Search PubMed.
- P. Mertins, D. R. Mani, K. V. Ruggles, M. A. Gillette, K. R. Clauser, P. Wang, X. Wang, J. W. Qiao, S. Cao, F. Petralia, E. Kawaler, F. Mundt, K. Krug, Z. Tu, J. T. Lei, M. L. Gatza, M. Wilkerson, C. M. Perou, V. Yellapantula, K. L. Huang, C. Lin, M. D. McLellan, P. Yan, S. R. Davies, R. R. Townsend, S. J. Skates, J. Wang, B. Zhang, C. R. Kinsinger, M. Mesri, H. Rodriguez, L. Ding, A. G. Paulovich, D. Fenyo, M. J. Ellis, S. A. Carr and C. Nci, Proteogenomics connects somatic mutations to signalling in breast cancer, Nature, 2016, 534, 55–62 CrossRef CAS PubMed.
- J. S. Parker, M. Mullins, M. C. Cheang, S. Leung, D. Voduc, T. Vickery, S. Davies, C. Fauron, X. He, Z. Hu, J. F. Quackenbush, I. J. Stijleman, J. Palazzo, J. S. Marron, A. B. Nobel, E. Mardis, T. O. Nielsen, M. J. Ellis, C. M. Perou and P. S. Bernard, Supervised risk predictor of breast cancer based on intrinsic subtypes, J. Clin. Oncol., 2009, 27, 1160–1167 CrossRef PubMed.
- P. Shannon, A. Markiel, O. Ozier, N. S. Baliga, J. T. Wang, D. Ramage, N. Amin, B. Schwikowski and T. Ideker, Cytoscape: a software environment for integrated models of biomolecular interaction networks, Genome Res., 2003, 13, 2498–2504 CrossRef CAS PubMed.
- D. Warde-Farley, S. L. Donaldson, O. Comes, K. Zuberi, R. Badrawi, P. Chao, M. Franz, C. Grouios, F. Kazi, C. T. Lopes, A. Maitland, S. Mostafavi, J. Montojo, Q. Shao, G. Wright, G. D. Bader and Q. Morris, The GeneMANIA prediction server: biological network integration for gene prioritization and predicting gene function, Nucleic Acids Res., 2010, 38, W214–W220 CrossRef CAS PubMed.
- E. Eden, R. Navon, I. Steinfeld, D. Lipson and Z. Yakhini, GOrilla: a tool for discovery and visualization of enriched GO terms in ranked gene lists, BMC Bioinf., 2009, 10, 48 CrossRef PubMed.
- F. Supek, M. Bosnjak, N. Skunca and T. Smuc, REVIGO summarizes and visualizes long lists of gene ontology terms, PLoS One, 2011, 6, e21800 CAS.
- P. L. Jerevall, A. Jansson, T. Fornander, L. Skoog, B. Nordenskjold and O. Stal, Predictive relevance of HOXB13 protein expression for tamoxifen benefit in breast cancer, Breast Cancer Res., 2010, 12, R53 CrossRef PubMed.
- C. Andreini, L. Banci, I. Bertini and A. Rosato, Occurrence of copper proteins through the three domains of life: a bioinformatic approach, J. Proteome Res., 2008, 7, 209–216 CrossRef CAS PubMed.
- A. Prakash, G. K. Dhaliwal, P. Kumar and A. B. Majeed, Brain biometals and Alzheimer's disease – boon or bane?, Int. J. Neurosci., 2016, 1–10, DOI:10.3109/00207454.2016.1174118.
- A. Binolfi, A. A. Valiente-Gabioud, R. Duran, M. Zweckstetter, C. Griesinger and C. O. Fernandez, Exploring the structural details of Cu(I) binding to alpha-synuclein by NMR spectroscopy, J. Am. Chem. Soc., 2011, 133, 194–196 CrossRef CAS PubMed.
- J. Stockel, J. Safar, A. C. Wallace, F. E. Cohen and S. B. Prusiner, Prion protein selectively binds copper(II) ions, Biochemistry, 1998, 37, 7185–7193 CrossRef CAS PubMed.
- M. L. Kramer, H. D. Kratzin, B. Schmidt, A. Romer, O. Windl, S. Liemann, S. Hornemann and H. Kretzschmar, Prion protein binds copper within the physiological concentration range, J. Biol. Chem., 2001, 276, 16711–16719 CrossRef CAS PubMed.
- Y. Wang, V. Hodgkinson, S. Zhu, G. A. Weisman and M. J. Petris, Advances in the understanding of mammalian copper transporters, Adv. Nutr., 2011, 2, 129–137 CrossRef CAS PubMed.
- C. A. Phillips-Krawczak, A. Singla, P. Starokadomskyy, Z. Deng, D. G. Osborne, H. Li, C. J. Dick, T. S. Gomez, M. Koenecke, J. S. Zhang, H. Dai, L. F. Sifuentes-Dominguez, L. N. Geng, S. H. Kaufmann, M. Y. Hein, M. Wallis, J. McGaughran, J. Gecz, B. Sluis, D. D. Billadeau and E. Burstein, COMMD1 is linked to the WASH complex and regulates endosomal trafficking of the copper transporter ATP7A, Mol. Biol. Cell, 2015, 26, 91–103 CrossRef PubMed.
- P. de Bie, B. van de Sluis, E. Burstein, P. V. van de Berghe, P. Muller, R. Berger, J. D. Gitlin, C. Wijmenga and L. W. Klomp, Distinct Wilson's disease mutations in ATP7B are associated with enhanced binding to COMMD1 and reduced stability of ATP7B, Gastroenterology, 2007, 133, 1316–1326 CrossRef CAS PubMed.
- Y. Li, J. Du, P. Zhang and J. Ding, Crystal structure of human copper homeostasis protein CutC reveals a potential copper-binding site, J. Struct. Biol., 2010, 169, 399–405 CrossRef CAS PubMed.
- K. Hedrich, A. Djarmati, N. Schafer, R. Hering, C. Wellenbrock, P. H. Weiss, R. Hilker, P. Vieregge, L. J. Ozelius, P. Heutink, V. Bonifati, E. Schwinger, A. E. Lang, J. Noth, S. B. Bressman, P. P. Pramstaller, O. Riess and C. Klein, DJ-1 (PARK7) mutations are less frequent than Parkin (PARK2) mutations in early-onset Parkinson disease, Neurology, 2004, 62, 389–394 CrossRef CAS PubMed.
- F. Billia, L. Hauck, D. Grothe, F. Konecny, V. Rao, R. H. Kim and T. W. Mak, Parkinson-susceptibility gene DJ-1/PARK7 protects the murine heart from oxidative damage in vivo, Proc. Natl. Acad. Sci. U. S. A., 2013, 110, 6085–6090 CrossRef CAS PubMed.
- B. A. Gilston, E. P. Skaar and W. J. Chazin, Binding of transition metals to S100 proteins, Sci. China: Life Sci., 2016, 59, 792–801 CrossRef CAS PubMed.
- E. Lemieux, S. Bergeron, V. Durand, C. Asselin, C. Saucier and N. Rivard, Constitutively active MEK1 is sufficient to induce epithelial-to-mesenchymal transition in intestinal epithelial cells and to promote tumor invasion and metastasis, Int. J. Cancer, 2009, 125, 1575–1586 CrossRef CAS PubMed.
- M. L. Turski, D. C. Brady, H. J. Kim, B. E. Kim, Y. Nose, C. M. Counter, D. R. Winge and D. J. Thiele, A novel role for copper in Ras/mitogen-activated protein kinase signaling, Mol. Cell. Biol., 2012, 32, 1284–1295 CrossRef CAS PubMed.
- G. MacDonald, I. Nalvarte, T. Smirnova, M. Vecchi, N. Aceto, A. Dolemeyer, A. Frei, S. Lienhard, J. Wyckoff, D. Hess, J. Seebacher, J. J. Keusch, H. Gut, D. Salaun, G. Mazzarol, D. Disalvatore, M. Bentires-Alj, P. P. Di Fiore, A. Badache and N. E. Hynes, Memo is a copper-dependent redox protein with an essential role in migration and metastasis, Sci. Signaling, 2014, 7, ra56 CrossRef PubMed.
- A. V. Sorokin and J. Chen, MEMO1, a new IRS1-interacting protein, induces epithelial–mesenchymal transition in mammary epithelial cells, Oncogene, 2013, 32, 3130–3138 CrossRef CAS PubMed.
- K. Zaoui, S. Honore, D. Isnardon, D. Braguer and A. Badache, Memo-RhoA-mDia1 signaling controls microtubules, the actin network, and adhesion site formation in migrating cells, J. Cell Biol., 2008, 183, 401–408 CrossRef CAS PubMed.
- P. Bhoopathi, C. S. Gondi, M. Gujrati, D. H. Dinh and S. S. Lakka, SPARC mediates Src-induced disruption of actin cytoskeleton via inactivation of small GTPases Rho-Rac-Cdc42, Cell. Signalling, 2011, 23, 1978–1987 CrossRef CAS PubMed.
- S. L. Payne, M. J. Hendrix and D. A. Kirschmann, Lysyl oxidase regulates actin filament formation through the p130(Cas)/Crk/DOCK180 signaling complex, J. Cell. Biochem., 2006, 98, 827–837 CrossRef CAS PubMed.
- A. Voronova, W. Meyer-Klaucke, T. Meyer, A. Rompel, B. Krebs, J. Kazantseva, R. Sillard and P. Palumaa, Oxidative switches in functioning of mammalian copper chaperone Cox17, Biochem. J., 2007, 408, 139–148 CrossRef CAS PubMed.
- L. Banci, I. Bertini, S. Ciofi-Baffoni, T. Hadjiloi, M. Martinelli and P. Palumaa, Mitochondrial copper(I) transfer from Cox17 to Sco1 is coupled to electron transfer, Proc. Natl. Acad. Sci. U. S. A., 2008, 105, 6803–6808 CrossRef CAS PubMed.
- P. Wittung-Stafshede, Unresolved questions in human copper pump mechanisms, Q. Rev. Biophys., 2015, 48, 471–478 CrossRef CAS PubMed.
Footnote |
† Electronic supplementary information (ESI) available: Fig. S1–S3 and Table S1. See DOI: 10.1039/c6mt00202a |
|
This journal is © The Royal Society of Chemistry 2017 |
Click here to see how this site uses Cookies. View our privacy policy here.