Studying physisorption processes and molecular friction of cycloparaphenylene molecules on graphene nano-sized flakes: role of π⋯π and CH⋯π interactions
Received
3rd April 2017
, Accepted 30th May 2017
First published on 30th May 2017
Abstract
We theoretically study, by means of dispersion-corrected and cost-effective methods, the strength of non-covalent interactions between cyclic organic nanorings (i.e. [8]cycloparaphenylene molecule) and nano-sized (e.g. C96H24) graphene flakes acting as substrates. Both CH⋯π and π⋯π driven interactions are investigated, according to the relative orientation between the two weakly interacting monomers, whose potential energy profiles are accurately calculated in both cases. These configurations provide different physisorption curves, with the CH⋯π interaction leading to a larger well depth, and are found to slightly depend on edge effects of the nano-sized graphene flakes. Additionally, we fit the energy profiles to a compact (analytical) potential function, and study the atomic-scale friction between the molecule and the surface in the search of mechanisms for new molecular machines.
Design, System, Application
Organic molecules of cyclic topology are envisioned as chemical precursors for the controlled growth of SWCNTs of the desired (i.e. armchair or zigzag) type. Here we exploit, taken the case of [8]cycloparaphenylene and graphene nanoflakes as example, how the physisorption of these molecular templates might assist the process through their physical immobilization on substrates, which might induce an epitaxial nanochannel-like layer-by-layer growth, facilitating thus achieving that initial target. The molecular design strategy is based thus on challenging supramolecular forces, which are accurately estimated by state-of-the-art theoretical methods. Depending of the relative orientation between the adsorbate and the substrate, we can concomitantly estimate the strength of both π⋯π and CH⋯π interactions, as well as to disentangle the locality and additivity of the non-covalent forces driven by these processes, and fit the results to a simple yet accurate analytical potential function for further simulations at the nanoscale. In a further step, we also study the atomic-scale adsorbate–substrate friction, providing insights in how these systems may also act as molecular nanomachines.
|
1 Introduction
Cyclic organic nanorings are emerging carbon nanoforms with many envisioned applications.1–5 This family of molecules can be viewed as the cyclic analogues of linear conjugated oligomers, for instance [n]cycloparaphenylenes ([n]CPPs, see Fig. 1) are formed upon bending n phenylene units in para position until closing the corresponding nanoring, with their versatile, size-selective and gram-scale synthesis already achieved by a few groups6–9 and for systems of variable size up to n = 18 units. Among those promising chemical functions, these organic nanorings might constitute ideal templates, or building blocks, for the controlled growth of uniform single-walled carbon nanotubes (SWCNTs) of defined diameter, according to the number of units of the molecules acting as precursors, or with pre-defined borders, armchair or zigzag, according to the nature of the oligomer to be employed.10 However, despite some successful recent attempts in the search of new synthetic routes paving the way to the targeted SWCNTs, like the functionalization of [n]CPPs with fused polycyclic aromatic hydrocarbons (PAHs) or with heteroarenes,11–14 some open questions about the detailed molecular mechanism and the optimal experimental conditions are still not fully resolved, and thus additional research is still needed before viable applications of these molecules for that ambitious goal materialize.
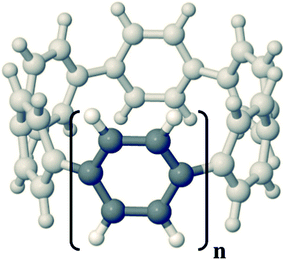 |
| Fig. 1 Chemical structure of the [n]CPP compounds. | |
The crystalline structure of solid-state samples of [n]CPPs, in which quasi-onedimensional but disjoint nanochannels are known to coexist with the herringbone pattern typical of PAHs crystals,15 might help to understand and then further engineer possible synthetic routes fuelling the aforementioned synthesis. We have recently rationalized the energy stability for the complete set of self-assembled dimers found in the crystalline structure of [6–12]CPPs, and how their different orientations could drive from a thermodynamical point of view (i.e. via their relative contribution to the cohesive energy) the formation of crystals depending on the system size.16 This supramolecular order and morphology of the samples might facilitate the growing mechanism of SWCNTs, via some Diels–Alder mechanism with C2H4 or by some C2H radical attacks, and subsequent dehydrogenation reactions,17,18 which could benefit from immobilizing the samples on some substrates to induce a fully directional growth, an issue we would thus like to consistently explore here.
The aromatic surface of graphene19,20 makes it an ideal substrate for immobilization of these organic nanorings through CH⋯π and π⋯π non-covalent interactions. Graphene nano-sized flakes (or nanoislands on other substrates) are extremely useful models to study these highly local interactions in adsorption processes,21–23 as they can also be easily functionalized24 and produced by STM experiments through mechanical contact between the tip and the surface or through electro-exfoliation.25 Once a reasonable nano-sized graphene flake is selected one can choose the corresponding nanoring size to avoid pronounced edge effects. Actually, according to the two possible orientations of the [n]CPP molecular backbone with respect to the flat graphene-like surface, one can also study the strength and equilibrium distance of the CH⋯π and of the π⋯π involved non-covalent interactions, similarly to the driven interactions found between parallel-displaced (face-to-face) and T-shaped benzene-graphene models. These interactions can be characterized by the corresponding energy profile corresponding to the physisorption process, disclosing thus useful information and structure–property relationships for the creation of new supramolecular functional materials. Furthermore, investigating the rotation of the cyclic nanoring against the surface would also allow to characterize the corresponding energy profiles for this molecular friction.
Note that the aforementioned strategy demands the use of theoretical methods being both cost-effective, due to the size of the weakly bound systems to be tackled, as well as highly accurate, able to account for all the concurring electronic and/or dispersion interactions needed to disclose the routes for a fine-tuned use of [n]CPPs in substrate-mediated chemical processes. We will thus first present the set of density functional theory (DFT) models selected for it, underlining the way in which the non-covalent interactions are safely introduced, before applying them to the calculation of well depths and distances for the physisorption processes tackled. We will also simultaneously explore if other recently developed low-cost methods (e.g. HF-3c) can accurately reproduce these results, paving thus the way towards extensions to larger systems and/or further molecular engineering studies.
2 Theoretical framework
The dispersion interactions are expected to become the strongest component of the physisorption energy profiles in these (non-polar) molecules. It is known that DFT might suffer from some drawbacks to incorporate these interactions in standard treatments, due to the semi-local nature of the exchange–correlation kernels used, and thus being unable to fully capture the long-range correlation acting between electrons situated at separated points r and r′ in space. Therefore, we will rely on the D3(BJ) and NL methods (vide infra) coupled with the B3LYP exchange–correlation functional26,27 to incorporate these effects both accurately and cost-effectively.
The former D3(BJ) method employs pair-wise interactions between all atoms, A and B, separated by the internuclear distance RAB = |RA − RB|, as a function of the set of nth-order interatomic dispersion coefficients (CABn) and a damping function fn = (a1R0AB + a2)n to switch the energy from medium- to short-distances, with
. The model is truncated at second order and it takes the final form:28,29
|
 | (1) |
with the
sn and
ai (
i = 1,2) the standard parameters fitted for the functional specifically selected.
30–32 This method is dubbed as B3LYP-D3(BJ) after appending the suffix of the dispersion correction to the original B3LYP model.
Another approach makes uses of a non-local (NL) kernel33 with information from densities at both points in space, ρ (r) and ρ (r′), coupled through an interaction function Φ (r,r′) with the correct asymptotic behaviour |r − r′|−6:
|
 | (2) |
where the parameters
b and
C are also known for the functional selected.
34 This method is correspondingly named as B3LYP-NL, and it has been shown before to behave very accurately for all kind of weak chemical interactions.
35,36
We finally benchmark the recently developed (low-cost) HF-3c method37–39 in the sense that a minimal basis set is used, largely reducing the final cost of a calculation, and with the appendix-3c meaning three pairwise corrections in the form:
|
EHF−3c = EHF/MINIX + ED3(BJ) + EgCPBSSE + EcRAB, | (3) |
where
EHF/MINIX is the Hartree–Fock (HF) energy with the MINIX basis set, E
D3(BJ) is merely a re-parameterized version of the D3(BJ) correction presented previously,
EgCPBSSE denotes a geometrical counterpoise correction (gCP) to account for the expected basis sets superposition error (BSSE), and
EcRAB corrects the systematically overestimated covalent bond lengths (
RAB) for electronegative elements A and B. Despite its simplicity, the method has been shown before to reliably capture the main physics behind intermolecular interactions of [
n]CPPs compounds.
40
2.1 Other technical details
We always employ adequate basis sets (i.e., cc-pVDZ for geometry optimizations and def2-TZVP for single-point energy calculations) and the release 3.0.3 of the ORCA package41 including the corresponding auxiliary (def2-TZVP/JK) basis sets for the more demanding calculations after invoking the ‘chain-of-spheres’ (COSX) technique42 to alleviate the associated computational cost. The numerical integration thresholds for both the exchange–correlation functional and the non-local, if any, correction were always strengthened with respect to the default values, to avoid any numerical limitation or noise for non-covalently bound complexes.
3 Results and discussion
3.1 Optimized monomers and built-in model for the weakly bound complexes
The computational protocol followed starts with the gas-phase optimization, at the B3LYP/cc-pVDZ level, of the monomers presented in Fig. 2. The minima nature of the monomers was confirmed by all (3N-6) positive frequencies obtained. These optimized structures are subsequently used for the rest of single-point energy calculations reported here. As a representative geometrical magnitude for assessing the reliability of the method used, we choose for the [8]CPP molecule (C48H32) the diameter defined as the distance between the opposite ipso-carbon atoms, being calculated here to be 11.1 Å compared with the experimental (X-ray) value of 10.9 Å,15 and the bond length of the benzenoid rings, calculated to be 1.405 Å compared again with the experimental value of 1.40 Å. Note also the inter-ring and alternating dihedral angles of 30.1° found between neighbouring benzene rings, in agreement with other studies.40
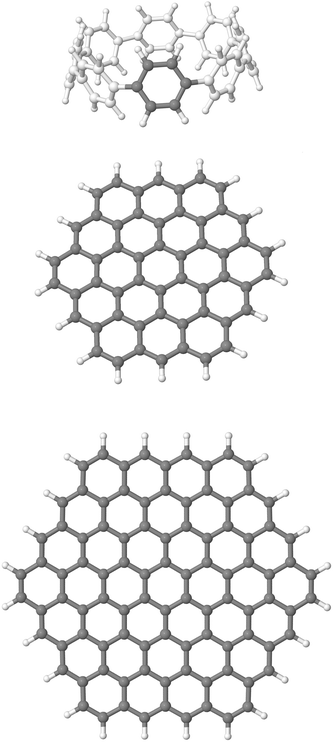 |
| Fig. 2 Top: Chemical structure of the [8]CPP oligomer; with the C atoms of the repeating unit along the backbone dark grey coloured. Middle and bottom: Chemical structure of the C54H18 and C96H24 compounds, respectively, used as finite models for graphene nanoflakes. | |
Note that, to model the graphene nanoflakes, we employ the finite-sized molecules CxxHyy, with xx/yy being 54/18 and 96/24, also known as circumcoronene and circumcircumcoronene, respectively, with the size of the latter being large enough to correctly represent bulk effects, according to recent benchmark studies.43 The use of finite molecular models, with the edges capped necessarily with H atoms, bring some advantages since they can be studied with a variety of highly performing theoretical methods, actually those developed for molecular systems and their nanoaggregates. The averaged optimized CC distances for C54H18 and C96H24 are 142.1 and 143.1 pm, respectively, compared with that of 142 pm for bulk graphene/graphite, and thus within the expected uncertainty when comparing theoretical and experimental results.44 More significant differences are found at the periphery rings, with CC distances ranging between 136.5 and 143.8 pm (137.4 and 144.9) for C54H18 (C96H24) due to pronounced edge effects. Actually, according to the relative sizes between the [8]CPP and both CxxHyy monomers, we will also try to concomitantly investigate the influence, if any, of edge effects of the graphene nanoflakes on the physisorption process.
We then build a dimer in which we situate the origin of the coordinate system at the center of mass of the graphene nanoflake, with the z axis being normal to its surface, and with the center of mass of the [8]CPP molecule on top of it. The intermolecular distance R thus corresponds to that between the center of masses of both monomers, and is aligned correspondingly with the z axis. The association or interaction energies for the weakly bound complexes at all distances are calculated as ΔE(R) = E([8]CPP⋯CxxHyy) − E([8]CPP) − E(CxxHyy), in steps of ΔR = 0.2 Å, except in the vicinity of the well depth for which ΔR is reduced to 0.05 Å. Note that: (i) the adsorption energies for benzene on graphene are fairly insensitive to the adsorption site;45 and (ii) we also neglect herein the deformation energy, the energy change when the isolated monomers are forced to readapt their geometry to that optimal for the dimer; however, these induced geometrical changes in the monomers upon formation of weakly bound complexes are sufficiently small, actually 0.3 (0.4) kcal mol−1 for the [8]CPP (C96H24) monomers, and are thus not expected to affect the conclusions reached here.
3.2 Exploration of computational methods: the case of [8]CPP⋯C54H18
We first present in Fig. 3 the energy profiles for the interaction energy between the [8]CPP and the C54H18 graphene nanoflake, for both configurations dominated by CH⋯π and π⋯π interactions. The B3LYP-based curves, despite using the large def2-TZVP basis set, were further corrected by the CounterPoise (CP) method to take into account and thus correct any residual basis set superposition error (BSSE). We also extract from these energy profiles the distance Re at which the well depth ΔE(Re) takes its minimum energy, gathering the values consequently in Table 1. We can easily see, independently of the method employed, how for the CH⋯π interactions: (i) the energy values for ΔE(Re) are twice as large than those computed for the π⋯π interactions, probably arising from the much larger surface contact between both monomers in this case;46 and (ii) the interaction energy per ring,
, is comprised between 3.8 and 4.1 kcal mol−1. Furthermore, the distance for the π⋯π interaction lies in the expected range of 3.30–3.40 Å, once one discounts half of the diameter (i.e. 11.1 Å) of the nanoring to the Re distances contained in Table 1.
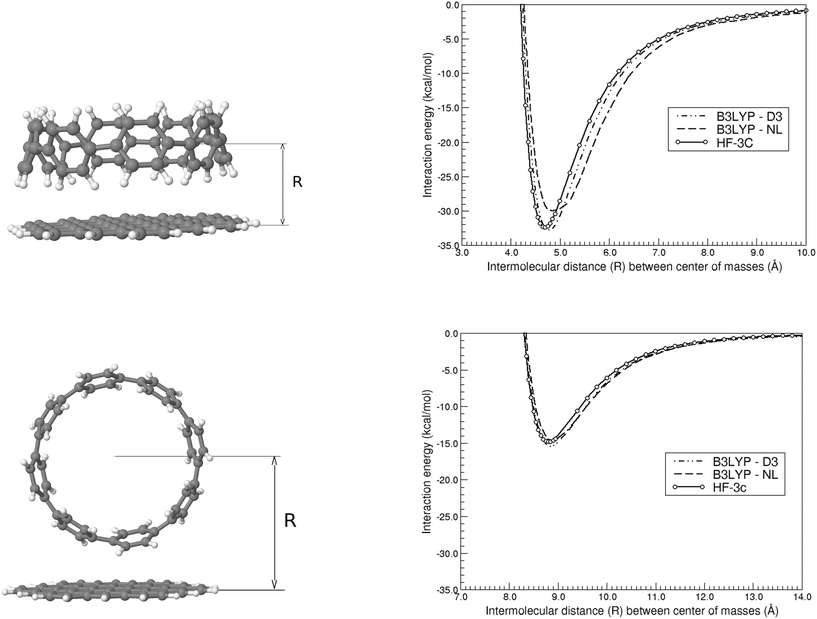 |
| Fig. 3 Interaction energy of the weakly bound [8]CPP⋯C54H18 complex as a function of the intermolecular (center-of-masses) distance, for both CH⋯π (top) and π⋯π (bottom) configurations and with the B3LYP-D3(BJ), B3LYP-NL, and HF-3c methods. The structures shown correspond to the minimum energy (at Re) situation. | |
Table 1 Interaction energies (kcal mol−1) and distances (Å) for the minimum energy configuration of [8]CPP and CxxHyy complexes
Complex |
Method |
CH⋯π |
π⋯π |
R
e
|
ΔE(Re) |
R
e
|
ΔE(Re) |
[8]CPP⋯C54H18 |
B3LYP-D3(BJ) |
4.75 |
−32.85 |
8.85 |
−15.45 |
|
B3LYP-NL |
4.80 |
−30.04 |
8.90 |
−14.69 |
|
HF-3c |
4.70 |
−32.42 |
8.80 |
−14.83 |
[8]CPP⋯(C54H18)2 |
HF-3c |
4.75 |
−32.94 |
— |
— |
[8]CPP⋯C96H24 |
HF-3c |
4.65 |
−42.59 |
8.75 |
−17.11 |
Keeping in mind the additivity nature of the non-covalent interactions, we also modelled within the B3LYP-D3(BJ), B3LYP-NL, and HF-3c methods, a simplified system in which the pristine C54H18 graphene nanoflake interacts with a unique benzene ring, with the plane formed by the C atoms of the latter residing either perpendicular (i.e. mimicking the CH⋯π interactions) or parallel (i.e. mimicking the π⋯π interactions) to the surface. First of all, we find well depth between −5.1 and −5.7 kcal mol−1 for the former case, which means an overestimation (i.e. more negative values) of around 24–28% with respect to the
obtained before for the whole [8]CPP. In the case of π⋯π interactions, the well depth are comprising between −10.0 and −11.2 kcal mol−1, and thus representing approximately 70% of the interaction energies for the whole [8]CPP⋯C54H18 complex. The Re distances obtained for the C6H6⋯C54H18 complex were found to differ only by 0.05 Å with respect to the previous cases. If we compare now with the C6H6⋯C6H6 T-shaped and parallel displaced configurations, see for instance reference values at the CCSD(T)/aug-cc-pVQZ level from ref. 47, we obtain higher values as it was expected from the larger number of pairwise non-local interactions.
3.3 Selection of the theoretical method
As regards the performance of the different methods assessed in the previous section, the HF-3c results are always close and comprised between those of B3LYP-D3(BJ) and B3LYP-NL, approaching in fact the latter but keeping an excellent trade-off between accuracy and computational cost. Due to the slight overestimation of interaction energies usually provided by the B3LYP-D3(BJ) method,48 which it has been shown to also happen for CH⋯π and π⋯π benzene–benzene interactions,49 and the quality of the B3LYP-NL method for a large variety of non-covalently bound systems,50 we will employ in the following the HF-3c method for dealing with the larger systems tackled along the rest of the study. Note that it would be possible to further decrease the computational cost by using a dispersion-corrected semiempirical method (e.g. PM6-DH2) but it has also been shown that this leads to root-mean-squared errors of up to 1 kcal mol−1 in the case of benzene–benzene interactions in a set of benchmark systems.51 Thus, taking into account all these facts, we select the HF-3c as the most reliable cost-effective alternative to explore here the existing interactions between larger graphitic and cyclic carbon nanoforms.
3.4 Extending the size of graphene flakes: the cases of [8]CPP⋯(C54H18)2 and [8]CPP⋯C96H24
We will further consider if a second sheet of nano-sized graphene flakes, with the relative orientation between the stacked sheets as it is exactly found in graphite, would have any influence on the aforementioned values. Taking into account the relative strength of the CH⋯π and π⋯π interactions, we will exclusively focus on the former. Table 1 includes the new Re and ΔE(Re) values, which just differ by only 1–2% with respect to the values obtained before using only one sheet. This shows how the conclusions reached here will also hold for an infinite stack of nano-sized graphene sheets.
We now extend the 2D dimensions of the nano-sized graphene flake considered, going from C54H18 to C96H24, to disentangle the possible influence of edge effects on the previously calculated energy profiles. Note that the dimensions of the latter nano-sized graphene flake, 15.7 × 17.4 Å, are sufficiently large (compare the structures shown in Fig. 3 and 4) to accommodate a [8]CPP molecule with a diameter of 11.1 Å. Table 1 shows how the Re distances are not significantly modified in this case, although the interaction energies are stabilized by a considerable amount. Actually, the strength for the CH⋯π interactions is 2.5 times higher than that found for the π⋯π case, thus underlying the viability of some epitaxial nanochannel-like growth of the samples.
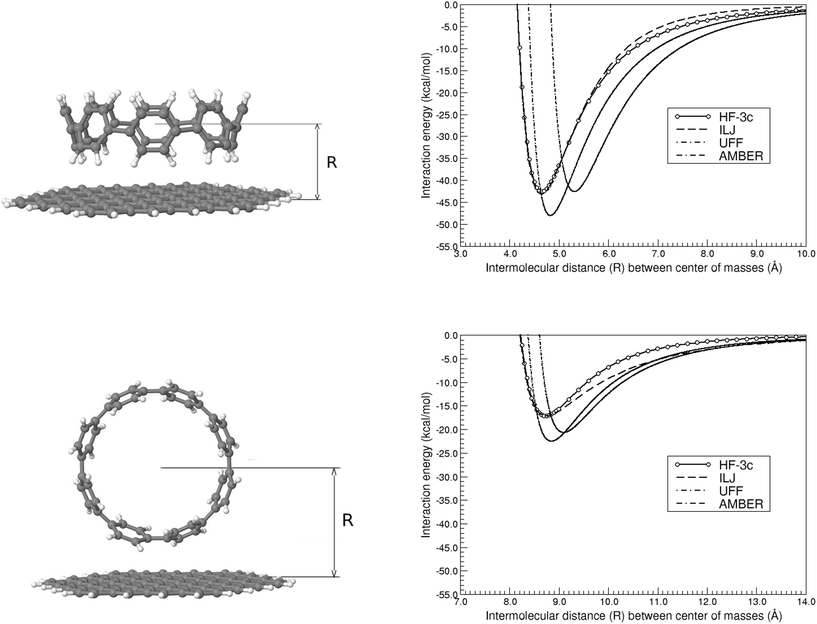 |
| Fig. 4 Interaction energy of the weakly bound [8]CPP⋯C96H24 complex as a function of the intermolecular (center-of-masses) distance, for both CH⋯π (top) and π⋯π (bottom) configurations, and with the HF-3c method, the ILJ potential, and the AMBER and UFF force fields. The structures shown correspond to the minimum energy (at Re) situation. | |
Actually, if we repeat the same exercise than before for the CH⋯π interactions, that is, comparing the interaction energy per ring
of −5.3 kcal mol−1 with that for a single T-shaped benzene ring of −5.5 kcal mol−1, we can see how these two values perfectly match now indicating much weaker edge effects in this case. This suggests an approximate linear relationship in the form ΔE(Re){[n]CPP⋯C96H24} ≈ nΔE(Re){C6H6⋯C96H24}. In the case of π⋯π interactions, we get a interaction energy for benzene with C96H24 of −10.85 kcal mol−1, to be compared with an experimental estimate of −11.5 kcal mol−1 for the interaction energy between benzene and infinite graphene,52 and thus showing again negligible edge effects, and a distance Re of 3.35 Å, to be compared to that of 3.25 Å in the case of the whole [8]CPP molecule, the latter thus fully representative of the expected π⋯π nature of the interactions involved.
We finally explore the impact of estimating the repulsive three-body effects through the form approximated by:53
|
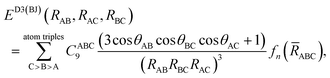 | (4) |
where
CABC9 is the corresponding
nth-order interatomic dispersion coefficient
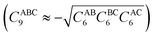
and
θIJ are the internal angles of the triangle formed by the internuclear distances
RAB −
RBC –
RAC, with
ABC the geometric mean of
RAB,
RBC, and
RAC, and
fn(
ABC) a damping function. This correction, when applied to the [8]CPP⋯C
96H
24 system, slightly shifts the physisorption curves and modifies the interaction energies given in
Table 1 by only 4.1 and 1.9 kcal mol
−1, for the CH⋯π and π⋯π interactions, respectively, in line with previous findings for polycyclic conjugated hydrocarbons
54 and without significantly affecting the conclusions reached here.
3.5 Improved Lennard-Jones potential function
In classical force field expressions, the intermolecular forces are represented by an electrostatic and a Lennard-Jones (LJ) or similar term, with the latter relying usually on two parameters, the LJ radii and well depths. A correct description of condensed phases, soft matter, or interfacial phenomena needs often the reparameterization of this term for quantitative accuracy55–57 before attempting to perform any reliable molecular dynamics simulation. However, although this simple model is able to reproduce the main features of non-covalent interactions around equilibrium distances, it might overestimate the short-range interactions, and thus possibly the shape of any energy profile for physisorption.
We will complementarily explore here the use of an improved LJ (ILJ) potential function to express the physisorption profiles in a compact way, recently described in the literature and providing great accuracy for the pairwise atom-atom interactions of rare gas molecules in gas-phase:58
|
 | (5) |
with
ε and
Re the depth of the potential well and the corresponding distance,
m = 6 for neutral–neutral systems, and the
n(
R) function given by:
|
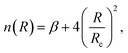 | (6) |
introducing the
β parameter as a measure of the hardness/softness of the interacting systems since
n(
R → 0) =
β. This
n(
R) dependence is expected to lead to a correct behavior in a wide range of intermolecular distances. We collect in
Table 2 the values of
β obtained by a leasts-squares fitting of
eqn (5)–(6) to the HF-3c curves, for the CH⋯π and π⋯π interactions and for both nano-sized graphene flakes considered. The depth and location of the potential well is perfectly reproduced by the model, see
Table 2, in agreement with previous studies of physisorption processes of small molecules on coronene.
59Fig. 4 shows the whole repulsive and long-range attractive regions, with the largest deviations occurring for the π⋯π stacking and restricted to the intermediate region between 4–7 Å. We add the physisorption profiles obtained with the highly used UFF
60 and AMBER
61 force fields, with the G09 package
62 and assigning charges with the QEq method,
63 to compare these results with the ILJ and the HF-3c expression. Note also that this ILJ expression has also been successfully applied to the energy ordering of nanoaggregates of up to 20–25 benzene molecules,
64 thus showing a great potential for further applications within the field.
Table 2 Interaction energies (kcal mol−1), distances (Å), and values of β for the minimum energy configuration of [8]CPP and CxxHyy complexes, as calculated with the ILJ potential fitted for the HF-3c energy curves
|
CH⋯π |
π⋯π |
Complex |
β
|
R
e
|
ΔE (Re) |
β
|
R
e
|
ΔE (Re) |
[8]CPP⋯C54H18 |
9.6333 |
4.70 |
−32.42 |
32.5856 |
8.80 |
−14.83 |
[8]CPP⋯C96H24 |
9.5626 |
4.65 |
−43.11 |
29.7393 |
8.75 |
−17.11 |
3.6 [8]CPP⋯C96H24 friction studies
Finally, we would like to investigate how the molecular friction, depending generally speaking on the roughness and the chemistry of the surface, might impact on the dynamical evolution of the physisorption process. Note that only recently atomic-scale (STM and/or AFM) experiments are able to probe this highly directional effect65,66 and theoretical studies are still scarce. Furthermore, the interfacial energy for adsorbates sliding or rotating over a graphene-like surface is expected to complement the current understanding about the friction mechanism in pristine and functionalized graphene flakes,22 as well as to participate in the design of molecular machines and motors operating at the nanoscale.67
We present in Fig. 5 the effect of rotating the [8]CPP molecule against the nano-sized (C96H24) graphene surface, again using the HF-3c method and in the range
. We explore both possibilities, either with the molecule frictioning the surface through the CH bonds (CH⋯π interactions) or through the twisted benzene rings closest to the surface (π⋯π interactions) and note that: (i) the symmetry point group of an idealized [8]CPP molecule is D8h, and thus the range of angles explored should be sufficient to infer any periodicity effects although the effective symmetry of the system (molecule plus nanoflake) would probably decrease; and (ii) we keep a rigid and flat graphene surface and thus neglect the influence of any out-of-plane (flexural) elastic deformation.
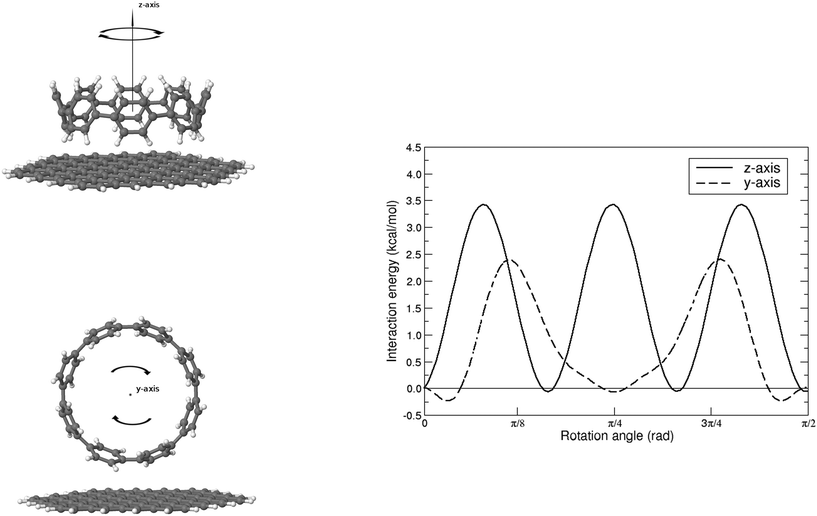 |
| Fig. 5 Interaction energy for the rotation of the weakly bound [8]CPP⋯C96H24 complex, as calculated at the HF-3c level, for both CH⋯π (top) and π⋯π (bottom) configurations. | |
For the case of CH⋯π interactions, the initial structure (shown in Fig. 6) corresponds to the angle of minimum energy, with the H atoms pointing mostly to the inner part of the benzene, and thus maximizing the quadrupole–quadrupole interactions.68 We indeed observe a periodic curve with an energy peak of 3.42 kcal mol−1 every
approximately, indicating a low energy barrier of only a few times the value of kBT at room temperature, and thus relatively easy to overcome as a function of temperature. Actually, for a value (T = 298 K) of kBT ≈ 0.6 kcal mol−1, we can observe a range of angles around 10° freely available for rotating the molecule once it was physisorbed. Note that the rotation of organic molecules, like C60 or PCBM derivatives, at room temperature and in bulk phase, is known to contribute to the energetic disorder of the samples.69 That peak of maximum energy corresponds to the relative orientation also displayed in Fig. 6, where the rotation originates larger repulsive interactions. Similar findings are found for the π⋯π interactions, with a even lower energy barrier of 2.64 kcal mol−1 for fully rotating the molecule and a larger
period between energy peaks, which allows a deeper understanding of the molecular friction mechanisms and anticipates possible design path of molecular machines based on [n]CPP molecules.
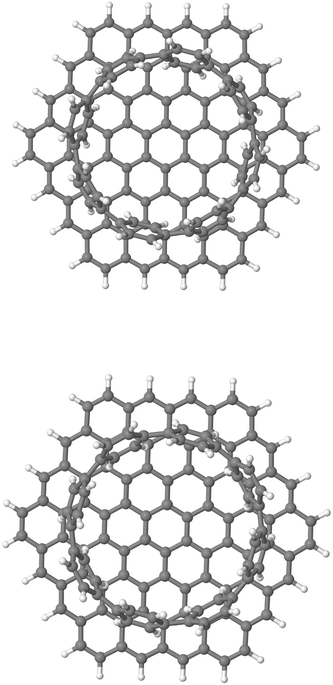 |
| Fig. 6 Chemical structure (top view) of the weakly bound [8]CPP⋯C96H24 complex at 0 (top) and (bottom) rotating angles along the z-axis perpendicular to the surface. | |
4 Conclusions
We have theoretically studied the non-covalent interactions between the [8]CPP molecule, a phenylene oligomer with cyclic topology, and a pair of nano-sized graphene flakes of increasing size, involving CH⋯π and π⋯π stacking depending on how the [8]CPP approaches to the flat surface. The dispersion-corrected and cost-effective HF-3c method has revealed as a reliable and robust theoretical tool for this kind of studies. Interestingly, the strength of the CH⋯π binding is at least twice as high than the π⋯π stacking, which might be exploited for the controlled and highly directional layer-by-layer growth of the samples.
The locality of the weak interactions is analyzed by adding a second graphene-like sheet, with a negligible influence on the results. On the other hand, we have also disclosed the influence of edge effects, which may be sizable if the dimensions of the [n]CPP molecule are close to that of the nano-sized graphene flake. Nonetheless, extending the length and width of the nano-sized graphene flake have allowed us to bracket the interaction energy in the case of having more extended graphene surfaces. Complementarily, the additive effect of weak forces in the CH⋯π stacking is probed by modelling a simpler case, a benzene molecule instead of the [8]CPP system, to infer a linear relationship between the binding energy and the number of benzene rings, which is not so pronounced in the case of the π⋯π stacking since the adjacent bent rings play also some role.
Furthermore, we have fitted the obtained data to a physically meaningful potential functions (the ILJ model) for non-covalent interactions. We have also investigated the mechanical behavior of the [8]CPP system at the nanoscale inspecting the friction isotropy and its strength, obtaining low energy barriers for rotating the molecule once it is physisorbed. Overall, we hope the present study contributes to the understanding and quantification of adhesive forces and interfacial phenomena in carbon-based materials, allowing thus to further model interfacial phenomena and/or for the growth of [n]CPP samples once the first monolayer is immobilized by the graphitic-like substrate, stimulating more efforts in the challenging field of the use of cyclic nanorings as templates for the controlled growth of SWCNTs and their subsequent applications.
Acknowledgements
This work is supported by the “Ministerio de Economía y Competitividad” of Spain and the “European Regional Development Fund” through project CTQ2014-55073-P. We kindly acknowledge L. Muccioli (U. Bologna, Italy) for a critical reading of the manuscript.
References
- H. Omachi, Y. Segawa and K. Itami, Acc. Chem. Res., 2012, 45, 1378 CrossRef CAS PubMed.
- S. Yamago, E. Kayahara and T. Iwamoto, Chem. Rec., 2014, 14, 84 CrossRef CAS PubMed.
- M. R. Golder and R. Jasti, Acc. Chem. Res., 2015, 48, 557 CrossRef CAS PubMed.
- S. E. Lewis, Chem. Soc. Rev., 2015, 44, 2221 RSC.
- Y. Segawa, A. Yagi, K. Matsui and K. Itami, Angew. Chem., Int. Ed., 2016, 55, 5136 CrossRef CAS PubMed.
- R. Jasti, J. Bhattacharjee, J. B. Neaton and C. R. Bertozzi, J. Am. Chem. Soc., 2008, 130, 17646 CrossRef CAS PubMed.
- H. Takaba, H. Omachi, Y. Yamamoto, J. Bouffard and K. Itami, Angew. Chem., Int. Ed., 2009, 48, 6112 CrossRef CAS PubMed.
- S. Yamago, Y. Watanabe and T. Iwamoto, Angew. Chem., Int. Ed., 2010, 49, 757 CrossRef CAS PubMed.
- E. Kayahara, V. K. Patel, J. Xia, R. Jasti and S. Yamago, Synlett, 2015, 26, 1615 CrossRef CAS.
- H. Omachi, T. Nakayama, E. Takahashi, Y. Segawa and K. Itami, Nat. Chem., 2013, 5, 572 CrossRef CAS PubMed.
- T. J. Sisto, S. Tian and X. R. Jasti, J. Org. Chem., 2012, 77, 5857 CrossRef CAS PubMed.
- T. Nishiuchi, X. Feng, V. Enkelmann, M. Wagner and K. Müllen, Chem. – Eur. J., 2012, 18, 16621 CrossRef CAS PubMed.
- F. E. Golling, M. Quernheim, M. Wagner, T. Nishiuchi and K. Müllen, Angew. Chem., Int. Ed., 2014, 53, 1525 CrossRef CAS PubMed.
- F. E. Golling, S. Osella, M. Quernheim, M. Wagner, D. Beljonne and K. Müllen, Chem. Sci., 2015, 6, 7072 RSC.
- J. Xia and R. Jasti, Angew. Chem., Int. Ed., 2012, 51, 2474 CrossRef CAS PubMed.
- M. Reche-Tamayo, M. Moral, A. J. Pérez-Jiménez and J. C. Sancho-García, J. Phys. Chem. C, 2016, 120, 22627 CAS.
- E. H. Fort and L. T. Scott, J. Mater. Chem., 2011, 21, 1373 RSC.
- H.-B. Li, A. J. Page, S. Irle and K. Morokuma, J. Phys. Chem. Lett., 2013, 4, 3176 CrossRef CAS.
- A. H. Castro Neto, F. Guinea, N. M. R. Peres, K. S. Novoselov and A. Geim, Rev. Mod. Phys., 2009, 81, 109 CrossRef CAS.
- A. K. Geim, Science, 2009, 324, 1530 CrossRef CAS PubMed.
- P. Lazar, F. Karlický, P. Jurečka, M. Kocman, E. Otyepková, K. Šafářová and M. Otyepka, J. Am. Chem. Soc., 2013, 135, 6372 CrossRef CAS PubMed.
- M. Pykal, P. Jurečka, F. Karlický and M. Otyepka, Phys. Chem. Chem. Phys., 2016, 18, 6351 RSC.
- E. Otyepková, P. Lazar, K. Cepe, O. Tomanec and M. Otyepka, Appl. Mater. Today, 2016, 5, 142 CrossRef.
- F. Karlicky, E. Otyepkova, R. Lo, M. Pitonak, P. Jurecka, M. Pykal, P. Hobza and M. Otyepka, J. Chem. Theory Comput., 2017, 13, 1328 CrossRef CAS PubMed.
- C. Rubio-Verdú, G. Sáenz-Arce, J. Martínez-Asencio, D. C. Milan, M. Moaied, J. J. Palacios, M. J. Caturla and C. Untiedt, Phys. Chem. Chem. Phys., 2017, 19, 8061 RSC.
- A. D. Becke, J. Chem. Phys., 1993, 98, 1372 CrossRef CAS.
- V. Barone and C. Adamo, Chem. Phys. Lett., 1994, 224, 432 CrossRef CAS.
- S. Grimme, J. Antony, S. Ehrlich and H. Krieg, J. Chem. Phys., 2010, 132, 154104 CrossRef PubMed.
- S. Grimme, S. Ehrlich and L. Goerigk, J. Comput. Chem., 2011, 32, 1456 CrossRef CAS PubMed.
- A. D. Becke and E. R. Johnson, J. Chem. Phys., 2005, 122, 154101 CrossRef PubMed.
- E. R. Johnson and A. D. Becke, J. Chem. Phys., 2005, 123, 024101 CrossRef PubMed.
- E. R. Johnson and A. D. Becke, J. Chem. Phys., 2006, 124, 174104 CrossRef PubMed.
- O. A. Vydrov and T. Van Voorhis, J. Chem. Phys., 2010, 133, 244103 CrossRef PubMed.
- W. Hujo and S. Grimme, J. Chem. Theory Comput., 2011, 7, 3866 CrossRef CAS PubMed.
- J. Aragó, E. Ortí and J. C. Sancho-García, J. Chem. Theory Comput., 2013, 9, 3437 CrossRef PubMed.
- J. Calbo, E. Ortí, J. C. Sancho-García and J. Aragó, Annu. Rep. Comput. Chem., 2015, 11, 37 CrossRef PubMed.
- R. Sure and S. Grimme, J. Comput. Chem., 2013, 34, 1672 CrossRef CAS PubMed.
- L. Goerigk, C. A. Collyer and J. R. Reimers, J. Phys. Chem. B, 2014, 118, 14612 CrossRef CAS PubMed.
- J. G. Brandenburg and S. Grimme, Top. Curr. Chem., 2014, 345, 1 CrossRef CAS PubMed.
- J. V. Climent-Medina, A. J. Pérez-Jiménez, M. Moral, E. San-Fabián and J. C. Sancho-García, ChemPhysChem, 2015, 16, 1520 CrossRef CAS PubMed.
- F. Neese, WIREs Comput. Mol. Sci., 2012, 2, 73 CrossRef CAS.
- F. Neese, F. Wennmohs, A. Hansen and U. Becker, Chem. Phys., 2009, 356, 98 CrossRef CAS.
- S. Grimme, C. Mück-Lichtenfeld and J. Antony, J. Phys. Chem. C, 2007, 111, 11199 CAS.
- E. Brémond, M. Savarese, N. Q. Su, Á. J. Pérez-Jiménez, X. Xu, J. C. Sancho-García and C. Adamo, J. Chem. Theory Comput., 2016, 12, 459 CrossRef PubMed.
- P.-P. Zhou and R.-Q. Zhang, Phys. Chem. Chem. Phys., 2015, 17, 12185 RSC.
- K. Yuan, R.-S. Zhao, J.-J. Zheng, H. Zheng, S. Nagase, S.-D. Zhao, Y.-Z. Liu and X. Zhao, J. Comput. Chem., 2017, 38, 730 CrossRef CAS PubMed.
- M. O. Sinnokrot and C. D. Sherrill, J. Phys. Chem. A, 2006, 110, 10656 CrossRef CAS PubMed.
- S. T. Schneebeli, A. D. Bochevarov and R. A. Friesner, J. Chem. Theory Comput., 2011, 7, 658 CrossRef CAS PubMed.
- K. Strutyński, M. Melle-Franco and J. A. N. F. Gomes, J. Phys. Chem. A, 2013, 117, 2844 CrossRef PubMed.
- L. Goerigk, J. Chem. Theory Comput., 2014, 10, 968 CrossRef CAS PubMed.
- K. Strutyński, J. A. N. F. Gomes and M. Melle-Franco, J. Phys. Chem. A, 2013, 118, 9561 CrossRef PubMed.
- R. Zacharia, H. Ulbricht and T. Hertel, Phys. Rev. B: Condens. Matter Mater. Phys., 2004, 69, 155406 CrossRef.
- O. A. von Lilienfeld and A. Tkatchenko, J. Chem. Phys., 2013, 132, 234109 CrossRef PubMed.
- J. C. Sancho-García, A. J. Pérez-Jiménez and Y. Olivier, J. Chem. Phys., 2015, 142, 054702 CrossRef PubMed.
- O. A. von Lilienfeld and D. Andrienko, J. Chem. Phys., 2006, 124, 054307 CrossRef CAS PubMed.
- L. Muccioli, G. D’Avino, R. Berardi, S. Orlandi, A. Pizzirusso, M. Ricci, O. M. Roscioni and C. Zannoni, Top. Curr. Chem., 2014, 352, 39 CrossRef CAS PubMed.
- M. Moral, W.-J. Son, J. C. Sancho-García, Y. Olivier and L. Muccioli, J. Chem. Theory Comput., 2015, 11, 3383 CrossRef CAS PubMed.
- F. Pirani, S. Brizi, L. F. Roncaratti, P. Casavecchia, D. Cappelletti and F. Vecchiocattivi, Phys. Chem. Chem. Phys., 2008, 10, 5489 RSC.
- Md. B. Yeamin, N. Faginas-Lago, M. Alberti, I. G. Cuesta, J. Sánchez-Marín and A. M. J. Sánchez de Merás, RSC Adv., 2014, 4, 54447 RSC.
- A. K. Rappé, C. J. Casewit, K. S. Colwell, W. A. Goddard III and W. M. Skiff, J. Am. Chem. Soc., 1992, 114, 10024 CrossRef.
- W. D. Cornell, P. Cieplak, C. I. Bayly, I. R. Gould, K. M. Merz Jr., D. M. Ferguson, D. C. Spellmeyer, T. Fox, J. W. Caldwell and P. A. Kollman, J. Am. Chem. Soc., 1995, 117, 5179 CrossRef CAS.
-
M. J. Frisch, G. W. Trucks, H. B. Schlegel, G. E. Scuseria, M. A. Robb, J. R. Cheeseman, G. Scalmani, V. Barone, B. Mennucci, G. A. Petersson, H. Nakatsuji, M. Caricato, X. Li, H. P. Hratchian, A. F. Izmaylov, J. Bloino, G. Zheng, J. L. Sonnenberg, M. Hada, M. Ehara, K. Toyota, R. Fukuda, J. Hasegawa, M. Ishida, T. Nakajima, Y. Honda, O. Kitao, H. Nakai, T. Vreven, J. A. Montgomery, Jr., J. E. Peralta, F. Ogliaro, M. Bearpark, J. J. Heyd, E. Brothers, K. N. Kudin, V. N. Staroverov, R. Kobayashi, J. Normand, K. Raghavachari, A. Rendell, J. C. Burant, S. S. Iyengar, J. Tomasi, M. Cossi, N. Rega, J. M. Millam, M. Klene, J. E. Knox, J. B. Cross, V. Bakken, C. Adamo, J. Jaramillo, R. Gomperts, R. E. Stratmann, O. Yazyev, A. J. Austin, R. Cammi, C. Pomelli, J. W. Ochterski, R. L. Martin, K. Morokuma, V. G. Zakrzewski, G. A. Voth, P. Salvador, J. J. Dannenberg, S. Dapprich, A. D. Daniels, Ö. Farkas, J. B. Foresman, J. V. Ortiz, J. Cioslowski and D. J. Fox, Gaussian 09, Revision D.03, Gaussian, Inc., Wallingford, CT, 2009 Search PubMed.
- A. K. Rappé, L. M. Bormann-Rochotte, D. C. Wiser, J. R. Hart, M. A. Pietsch, C. J. Casewit and W. M. Skiff, Mol. Phys., 2007, 105, 301 CrossRef.
- M. Bartolomei, F. Pirani and J. M. C. Marques, J. Comput. Chem., 2015, 36, 2291 CrossRef CAS PubMed.
- A. J. Weymouth, D. Meuer, P. Mutombo, T. Wutscher, M. Ondracek, P. Jelinek and F. J. Giessibl, Phys. Rev. Lett., 2013, 111, 126103 CrossRef CAS PubMed.
- C. M. Almeida, R. Prioli, B. Fragneaud, L. G. Cançado, R. Paupitz, D. S. Galvao, M. De Cicco, M. G. Menezes, C. A. Achete and R. B. Capaz, Sci. Rep., 2016, 6, 31569 CrossRef CAS PubMed.
- W. R. Browne and B. L. Feringa, Nat. Nanotechnol., 2006, 1, 25 CrossRef CAS PubMed.
- C. R. Martínez and B. L. Iverson, Chem. Sci., 2012, 3, 2191 RSC.
- G. D’Avino, Y. Olivier, L. Muccioli and D. Beljonne, J. Mater. Chem. C, 2016, 4, 3747 RSC.
|
This journal is © The Royal Society of Chemistry 2017 |
Click here to see how this site uses Cookies. View our privacy policy here.